Journal Description
Remote Sensing
Remote Sensing
is an international, peer-reviewed, open access journal about the science and application of remote sensing technology, and is published semimonthly online by MDPI. The Remote Sensing Society of Japan (RSSJ) and the Japan Society of Photogrammetry and Remote Sensing (JSPRS) are affiliated with Remote Sensing, and their members receive a discount on the article processing charge.
- Open Access— free for readers, with article processing charges (APC) paid by authors or their institutions.
- High Visibility: indexed within Scopus, SCIE (Web of Science), Ei Compendex, PubAg, GeoRef, Astrophysics Data System, Inspec, dblp, and other databases.
- Journal Rank: JCR - Q1 (Geosciences, Multidisciplinary) / CiteScore - Q1 (General Earth and Planetary Sciences)
- Rapid Publication: manuscripts are peer-reviewed and a first decision is provided to authors approximately 24.9 days after submission; acceptance to publication is undertaken in 2.5 days (median values for papers published in this journal in the first half of 2025).
- Recognition of Reviewers: reviewers who provide timely, thorough peer-review reports receive vouchers entitling them to a discount on the APC of their next publication in any MDPI journal, in appreciation of the work done.
- Companion journal: Geomatics.
- Journal Cluster of Geospatial and Earth Sciences: Remote Sensing, Geosciences, Quaternary, Earth, Geographies, Geomatics and Fossil Studies.
Impact Factor:
4.1 (2024);
5-Year Impact Factor:
4.8 (2024)
Latest Articles
Driving Forces and Future Scenario Simulation of Urban Agglomeration Expansion in China: A Case Study of the Pearl River Delta Urban Agglomeration
Remote Sens. 2025, 17(14), 2455; https://doi.org/10.3390/rs17142455 (registering DOI) - 15 Jul 2025
Abstract
►
Show Figures
The remote sensing monitoring of land use changes and future scenario simulation hold crucial significance for accurately characterizing urban expansion patterns, optimizing urban land use configurations, and thereby promoting coordinated regional development. Through the integration of multi-source data, this study systematically analyzes the
[...] Read more.
The remote sensing monitoring of land use changes and future scenario simulation hold crucial significance for accurately characterizing urban expansion patterns, optimizing urban land use configurations, and thereby promoting coordinated regional development. Through the integration of multi-source data, this study systematically analyzes the spatiotemporal trajectories and driving forces of land use changes in the Pearl River Delta urban agglomeration (PRD) from 1990 to 2020 and further simulates the spatial patterns of urban land use under diverse development scenarios from 2025 to 2035. The results indicate the following: (1) During 1990–2020, urban expansion in the Pearl River Delta urban agglomeration exhibited a “stepwise growth” pattern, with an annual expansion rate of 3.7%. Regional land use remained dominated by forest (accounting for over 50%), while construction land surged from 6.5% to 21.8% of total land cover. The gravity center trajectory shifted southeastward. Concurrently, cropland fragmentation has intensified, accompanied by deteriorating connectivity of ecological lands. (2) Urban expansion in the PRD arises from synergistic interactions between natural and socioeconomic drivers. The Geographically and Temporally Weighted Regression (GTWR) model revealed that natural constraints—elevation (regression coefficients ranging −0.35 to −0.05) and river network density (−0.47 to −0.15)—exhibited significant spatial heterogeneity. Socioeconomic drivers dominated by year-end paved road area (0.26–0.28) and foreign direct investment (0.03–0.11) emerged as core expansion catalysts. Geographic detector analysis demonstrated pronounced interaction effects: all factor pairs exhibited either two-factor enhancement or nonlinear enhancement effects, with interaction explanatory power surpassing individual factors. (3) Validation of the Patch-generating Land Use Simulation (PLUS) model showed high reliability (Kappa coefficient = 0.9205, overall accuracy = 95.9%). Under the Natural Development Scenario, construction land would exceed the ecological security baseline, causing 408.60 km2 of ecological space loss; Under the Ecological Protection Scenario, mandatory control boundaries could reduce cropland and forest loss by 3.04%, albeit with unused land development intensity rising to 24.09%; Under the Economic Development Scenario, cross-city contiguous development zones along the Pearl River Estuary would emerge, with land development intensity peaking in Guangzhou–Foshan and Shenzhen–Dongguan border areas. This study deciphers the spatiotemporal dynamics, driving mechanisms, and scenario outcomes of urban agglomeration expansion, providing critical insights for formulating regionally differentiated policies.
Full article
Open AccessArticle
Performance Degradation in Monopulse Angle Measurement of Planar Phased-Array Due to Cross-Polarization Component
by
Yunhui Zhang, Bo Pang, Dahai Dai, Bo Chen and Zhengkuan Tan
Remote Sens. 2025, 17(14), 2454; https://doi.org/10.3390/rs17142454 (registering DOI) - 15 Jul 2025
Abstract
Due to the high-precision angle measurement performance, the monopulse technique plays a key role in fields such as remote sensing and space surveillance. The accuracy of monopulse angle measurement depends on the received amplitude and phase information, which is sensitive to the polarization
[...] Read more.
Due to the high-precision angle measurement performance, the monopulse technique plays a key role in fields such as remote sensing and space surveillance. The accuracy of monopulse angle measurement depends on the received amplitude and phase information, which is sensitive to the polarization component. Previous research has demonstrated that the performance of monopulse radar equipped with a parabolic antenna suffers from the cross-polarization component. However, it is not clear whether phased arrays (PAs) with higher degrees of freedom will also be affected by the cross-polarization component, and the parameter tolerance for performance degradation remains uncertain. In this paper, we establish a mathematical model of monopulse angle measurement in PA radar, which provides a comprehensive consideration of the cross-polarization component. Then, the received amplitude and phase patterns of PA radar are analyzed, and the theoretical angle errors caused by the cross-polarization jamming are derived. The experiments are conducted based on the measured amplitude-phase patterns of both co-polarization and cross-polarization. Experimental results are consistent with the theoretical analysis: the angle errors caused by cross-polarization jamming can reach half of the beamwidth in both azimuth and elevation dimensions, provided that the power of the cross-polarization and co-polarization components at the receiver is equal.
Full article
(This article belongs to the Special Issue Recent Advances in SAR: Signal Processing and Target Recognition)
Open AccessArticle
Soil Moisture Prediction Using the VIC Model Coupled with LSTMseq2seq
by
Xiuping Zhang, Xiufeng He, Rencai Lin, Xiaohua Xu, Yanping Shi and Zhenning Hu
Remote Sens. 2025, 17(14), 2453; https://doi.org/10.3390/rs17142453 (registering DOI) - 15 Jul 2025
Abstract
►▼
Show Figures
Soil moisture (SM) is a key variable in agricultural ecosystems and is crucial for drought prevention and control management. However, SM is influenced by underlying surface and meteorological conditions, and it changes rapidly in time and space. To capture the changes in SM
[...] Read more.
Soil moisture (SM) is a key variable in agricultural ecosystems and is crucial for drought prevention and control management. However, SM is influenced by underlying surface and meteorological conditions, and it changes rapidly in time and space. To capture the changes in SM and improve the accuracy of short-term and medium-to-long-term predictions on a daily scale, an LSTMseq2seq model driven by both observational data and mechanism models was constructed. This framework combines historical meteorological elements and SM, as well as the SM change characteristics output by the VIC model, to predict SM over a 90-day period. The model was validated using SMAP SM. The proposed model can accurately predict the spatiotemporal variations in SM in Jiangxi Province. Compared with classical machine learning (ML) models, traditional LSTM models, and advanced transformer models, the LSTMseq2seq model achieved R2 values of 0.949, 0.9322, 0.8839, 0.8042, and 0.7451 for the prediction of surface SM over 3 days, 7 days, 30 days, 60 days, and 90 days, respectively. The mean absolute error (MAE) ranged from 0.0118 m3/m3 to 0.0285 m3/m3. This study also analyzed the contributions of meteorological features and simulated future SM state changes to SM prediction from two perspectives: time importance and feature importance. The results indicated that meteorological and SM changes within a certain time range prior to the prediction have an impact on SM prediction. The dual-driven LSTMseq2seq model has unique advantages in predicting SM and is conducive to the integration of physical mechanism models with data-driven models for handling input features of different lengths, providing support for daily-scale SM time series prediction and drought dynamics prediction.
Full article
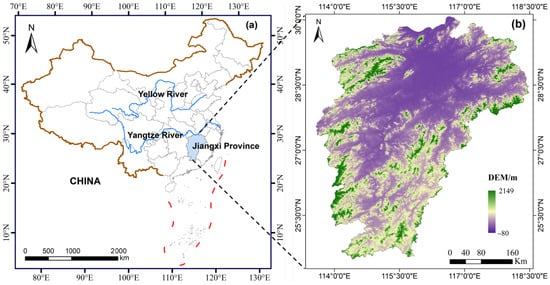
Figure 1
Open AccessArticle
Multimodal Distribution of Positioning Errors in NRTK GNSS CORSs: A Case Study in Sicily (Italy)
by
Antonino Maltese, Claudia Pipitone, Mario Mattia, Massimo Rossi, Valentina Bruno and Gino Dardanelli
Remote Sens. 2025, 17(14), 2452; https://doi.org/10.3390/rs17142452 (registering DOI) - 15 Jul 2025
Abstract
In the last 20 years, NRTK GNSS CORS networks have been designed, developed, and employed in Sicily (Italy) for research purposes. Following a comprehensive description of the CORS networks in this region, this study aims to compare results obtained from multiple reference points
[...] Read more.
In the last 20 years, NRTK GNSS CORS networks have been designed, developed, and employed in Sicily (Italy) for research purposes. Following a comprehensive description of the CORS networks in this region, this study aims to compare results obtained from multiple reference points with known coordinates. The analysis will not only account for the various CORS networks to which these points belong but also examine the different correction streams applied in NRTK surveys. The assumption of a normal distribution, which is often assumed for the positioning errors, is generally confirmed by applying the areal stream corrections (FKP, MAX, iMAX), but still, a multimodal distribution is evident when punctual corrections (Nearest and VRS) are applied. The representation of the results in a GIS environment allows for showing the different patterns for some differential correction streams. A statistical approach allowed confirming the trends of the different distribution frequencies of the residuals of the coordinates and quantifying separately the correlation with parameters involved in the analysis. The correlation retrieved is predominantly weak and very weak for all CORS, except for one of those analyzed, for which the correlation is moderate. The correlation became strong for the same CORS, if all parameters are included, for both planimetric and plano-altimetric components (r = 0.6 and r = 0.7, respectively).
Full article
(This article belongs to the Special Issue BDS/GNSS for Earth Observation (Third Edition))
►▼
Show Figures
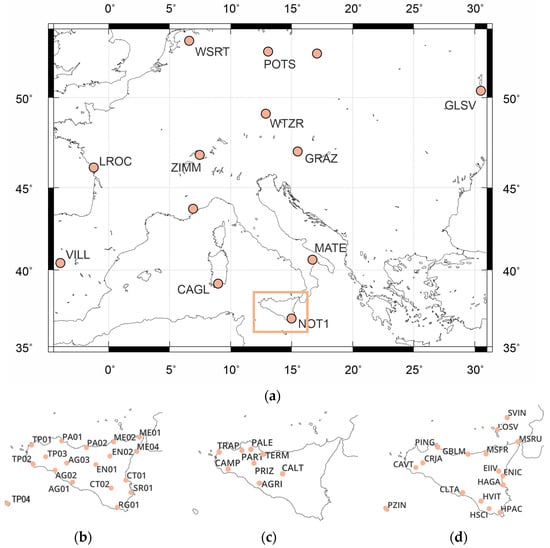
Figure 1
Open AccessArticle
Evaluating the Interferometric Performance of China’s Dual-Star SAR Satellite Constellation in Large Deformation Scenarios: A Case Study in the Jinchuan Mining Area, Gansu
by
Zixuan Ge, Wenhao Wu, Jiyuan Hu, Nijiati Muhetaer, Peijie Zhu, Jie Guo, Zhihui Li, Gonghai Zhang, Yuxing Bai and Weijia Ren
Remote Sens. 2025, 17(14), 2451; https://doi.org/10.3390/rs17142451 (registering DOI) - 15 Jul 2025
Abstract
Mining activities can trigger geological disasters, including slope instability and surface subsidence, posing a serious threat to the surrounding environment and miners’ safety. Consequently, the development of reasonable, effective, and rapid deformation monitoring methods in mining areas is essential. Traditional synthetic aperture radar(SAR)
[...] Read more.
Mining activities can trigger geological disasters, including slope instability and surface subsidence, posing a serious threat to the surrounding environment and miners’ safety. Consequently, the development of reasonable, effective, and rapid deformation monitoring methods in mining areas is essential. Traditional synthetic aperture radar(SAR) satellites are often limited by their revisiting period and image resolution, leading to unwrapping errors and decorrelation issues in the central mining area, which pose challenges in deformation monitoring in mining areas. In this study, persistent scatterer interferometric synthetic aperture radar (PS-InSAR) technology is used to monitor and analyze surface deformation of the Jinchuan mining area in Jinchang City, based on SAR images from the small satellites “Fucheng-1” and “Shenqi”, launched by the Tianyi Research Institute in Hunan Province, China. Notably, the dual-star constellation offers high-resolution SAR data with a spatial resolution of up to 3 m and a minimum revisit period of 4 days. We also assessed the stability of the dual-star interferometric capability, imaging quality, and time-series monitoring capability of the “Fucheng-1” and “Shenqi” satellites and performed a comparison with the time-series results from Sentinel-1A. The results show that the phase difference (SPD) and phase standard deviation (PSD) mean values for the “Fucheng-1” and “Shenqi” interferograms show improvements of 21.47% and 35.47%, respectively, compared to Sentinel-1A interferograms. Additionally, the processing results of the dual-satellite constellation exhibit spatial distribution characteristics highly consistent with those of Sentinel-1A, while demonstrating relatively better detail representation capabilities at certain measurement points. In the context of rapid deformation monitoring in mining areas, they show a higher revisit frequency and spatial resolution, demonstrating high practical value.
Full article
Open AccessArticle
SonarNet: Global Feature-Based Hybrid Attention Network for Side-Scan Sonar Image Segmentation
by
Juan Lei, Huigang Wang, Liming Fan, Qingyue Gu, Shaowei Rong and Huaxia Zhang
Remote Sens. 2025, 17(14), 2450; https://doi.org/10.3390/rs17142450 (registering DOI) - 15 Jul 2025
Abstract
With the rapid advancement of deep learning techniques, side-scan sonar image segmentation has become a crucial task in underwater scene understanding. However, the complex and variable underwater environment poses significant challenges for salient object detection, with traditional deep learning approaches often suffering from
[...] Read more.
With the rapid advancement of deep learning techniques, side-scan sonar image segmentation has become a crucial task in underwater scene understanding. However, the complex and variable underwater environment poses significant challenges for salient object detection, with traditional deep learning approaches often suffering from inadequate feature representation and the loss of global context during downsampling, thus compromising the segmentation accuracy of fine structures. To address these issues, we propose SonarNet, a Global Feature-Based Hybrid Attention Network specifically designed for side-scan sonar image segmentation. SonarNet features a dual-encoder architecture that leverages residual blocks and a self-attention mechanism to simultaneously capture both global structural and local contextual information. In addition, an adaptive hybrid attention module is introduced to effectively integrate channel and spatial features, while a global enhancement block fuses multi-scale global and spatial representations from the dual encoders, mitigating information loss throughout the network. Comprehensive experiments on a dedicated underwater sonar dataset demonstrate that SonarNet outperforms ten state-of-the-art saliency detection methods, achieving a mean absolute error as low as 2.35%. These results highlight the superior performance of SonarNet in challenging sonar image segmentation tasks.
Full article
(This article belongs to the Special Issue Ocean Remote Sensing Based on Radar, Sonar and Optical Techniques (Second Edition))
Open AccessArticle
Vegetation Baseline and Urbanization Development Level: Key Determinants of Long-Term Vegetation Greening in China’s Rapidly Urbanizing Region
by
Ke Zeng, Mengyao Ci, Shuyi Zhang, Ziwen Jin, Hanxin Tang, Hongkai Zhu, Rui Zhang, Yue Wang, Yiwen Zhang and Min Liu
Remote Sens. 2025, 17(14), 2449; https://doi.org/10.3390/rs17142449 (registering DOI) - 15 Jul 2025
Abstract
Urban vegetation shows significant spatial differences due to the combined effects of natural and human factors, yet fine-scale evolutionary patterns and their cross-scale feedback mechanisms remain limited. This study focuses on the Yangtze River Delta (YRD), the top economic area in China. By
[...] Read more.
Urban vegetation shows significant spatial differences due to the combined effects of natural and human factors, yet fine-scale evolutionary patterns and their cross-scale feedback mechanisms remain limited. This study focuses on the Yangtze River Delta (YRD), the top economic area in China. By integrating data from multiple Landsat sensors, we built a high—resolution framework to track vegetation dynamics from 1990 to 2020. It generates annual 30-m Enhanced Vegetation Index (EVI) data and uses a new Vegetation Green—Brown Balance Index (VBI) to measure changes between greening and browning. We combined Mann-Kendall trend analysis with machine—learning based attribution analysis to look into vegetation changes across different city types and urban—rural gradients. Over 30 years, the YRD’s annual EVI increased by 0.015/10 a, with greening areas 3.07 times larger than browning. Spatially, urban centers show strong greening, while peri—urban areas experience remarkable browning. Vegetation changes showed a city-size effect: larger cities had higher browning proportions but stronger urban cores’ greening trends. Cluster analysis finds four main evolution types, showing imbalances in grey—green infrastructure allocation. Vegetation baseline in 1990 is the main factor driving the long-term trend of vegetation greenness, while socioeconomic and climate drivers have different impacts depending on city size and position on the urban—rural continuum. In areas with low urbanization levels, climate factors matter more than human factors. These multi-scale patterns challenge traditional urban greening ideas, highlighting the need for vegetation governance that adapts to specific spatial conditions and city—unique evolution paths.
Full article
Open AccessArticle
UAV-Satellite Cross-View Image Matching Based on Adaptive Threshold-Guided Ring Partitioning Framework
by
Yushi Liao, Juan Su, Decao Ma and Chao Niu
Remote Sens. 2025, 17(14), 2448; https://doi.org/10.3390/rs17142448 (registering DOI) - 15 Jul 2025
Abstract
Cross-view image matching between UAV and satellite platforms is critical for geographic localization but remains challenging due to domain gaps caused by disparities in imaging sensors, viewpoints, and illumination conditions. To address these challenges, this paper proposes an Adaptive Threshold-guided Ring Partitioning Framework
[...] Read more.
Cross-view image matching between UAV and satellite platforms is critical for geographic localization but remains challenging due to domain gaps caused by disparities in imaging sensors, viewpoints, and illumination conditions. To address these challenges, this paper proposes an Adaptive Threshold-guided Ring Partitioning Framework (ATRPF) for UAV–satellite cross-view image matching. Unlike conventional ring-based methods with fixed partitioning rules, ATRPF innovatively incorporates heatmap-guided adaptive thresholds and learnable hyperparameters to dynamically adjust ring-wise feature extraction regions, significantly enhancing cross-domain representation learning through context-aware adaptability. The framework synergizes three core components: brightness-aligned preprocessing to reduce illumination-induced domain shifts, hybrid loss functions to improve feature discriminability across domains, and keypoint-aware re-ranking to refine retrieval results by compensating for neural networks’ localization uncertainty. Comprehensive evaluations on the University-1652 benchmark demonstrate the framework’s superiority; it achieves 82.50% Recall@1 and 84.28% AP for UAV→Satellite geo-localization, along with 90.87% Recall@1 and 80.25% AP for Satellite→UAV navigation. These results validate the framework’s capability to bridge UAV–satellite domain gaps while maintaining robust matching precision under heterogeneous imaging conditions, providing a viable solution for practical applications such as UAV navigation in GNSS-denied environments.
Full article
(This article belongs to the Special Issue Temporal and Spatial Analysis of Multi-Source Remote Sensing Images)
►▼
Show Figures
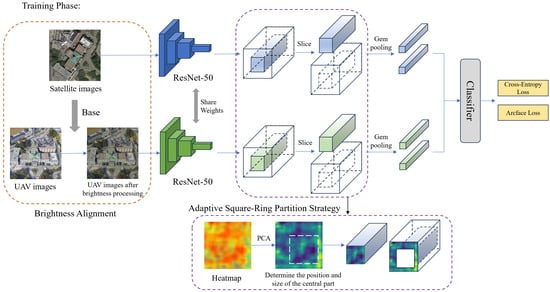
Figure 1
Open AccessArticle
Precipitation Governs Terrestrial Water Storage Anomaly Decline in the Hengduan Mountains Region, China, Amid Climate Change
by
Xuliang Li, Yayong Xue, Di Wu, Shaojun Tan, Xue Cao and Wusheng Zhao
Remote Sens. 2025, 17(14), 2447; https://doi.org/10.3390/rs17142447 (registering DOI) - 15 Jul 2025
Abstract
►▼
Show Figures
Climate change intensifies hydrological cycles, leading to an increased variability in terrestrial water storage anomalies (TWSAs) and a heightened drought risk. Understanding the spatiotemporal dynamics of TWSAs and their driving factors is crucial for sustainable water management. While previous studies have primarily attributed
[...] Read more.
Climate change intensifies hydrological cycles, leading to an increased variability in terrestrial water storage anomalies (TWSAs) and a heightened drought risk. Understanding the spatiotemporal dynamics of TWSAs and their driving factors is crucial for sustainable water management. While previous studies have primarily attributed TWSAs to regional factors, this study employs wavelet coherence, partial correlation analysis, and multiple linear regression to comprehensively analyze TWSA dynamics and their drivers in the Hengduan Mountains (HDM) region from 2003 to 2022, incorporating both regional and global influences. Additionally, dry–wet variations were quantified using the GRACE-based Drought Severity Index (GRACE-DSI). Key findings include the following: The annual mean TWSA showed a non-significant decreasing trend (−2.83 mm/y, p > 0.05), accompanied by increased interannual variability. Notably, approximately 36.22% of the pixels in the western HDM region exhibited a significantly decreasing trend. The Nujiang River Basin (NRB) (−17.17 mm/y, p < 0.01) and the Lancang (−17.17 mm/y, p < 0.01) River Basin experienced the most pronounced declines. Regional factors—particularly precipitation (PRE)—drove TWSA in 59% of the HDM region, followed by potential evapotranspiration (PET, 28%) and vegetation dynamics (13%). Among global factors, the North Atlantic Oscillation showed a weak correlation with TWSAs (r = −0.19), indirectly affecting it via winter PET (r = −0.56, p < 0.05). The decline in TWSAs corresponds to an elevated drought risk, notably in the NRB, which recorded the largest GRACE-DSI decline (slope = −0.011, p < 0.05). This study links TWSAs to climate drivers and drought risk, offering a framework for improving water resource management and drought preparedness in climate-sensitive mountain regions.
Full article
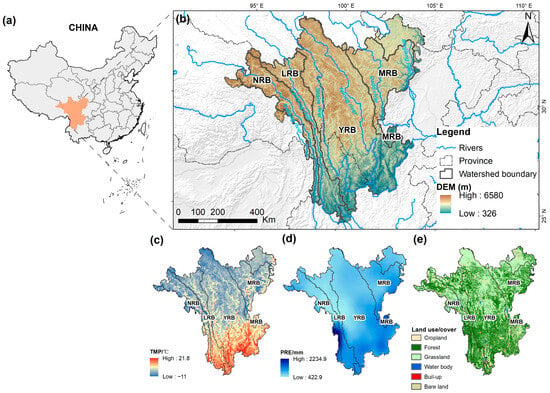
Figure 1
Open AccessArticle
Human Activities Dominantly Driven the Greening of China During 2001 to 2020
by
Xueli Chang, Zhangzhi Tian, Yepei Chen, Ting Bai, Zhina Song and Kaimin Sun
Remote Sens. 2025, 17(14), 2446; https://doi.org/10.3390/rs17142446 (registering DOI) - 15 Jul 2025
Abstract
Vegetation is a fundamental component of terrestrial ecosystems. Understanding how vegetation changes and what drives these evolutions is crucial for developing a high-quality ecological environment and addressing global climate change. Extensive evidence has shown that China has undergone substantial vegetation changes, characterized primarily
[...] Read more.
Vegetation is a fundamental component of terrestrial ecosystems. Understanding how vegetation changes and what drives these evolutions is crucial for developing a high-quality ecological environment and addressing global climate change. Extensive evidence has shown that China has undergone substantial vegetation changes, characterized primarily by greening. To quantify vegetation dynamics in China and assess the contributions of various drivers, we explored the spatiotemporal variations in the kernel Normalized Difference Vegetation Index (kNDVI) from 2001 to 2020, and quantitatively separated the influences of climate and human factors. The kNDVI time series were generated from the MCD19A1 v061 dataset based on the Google Earth Engine (GEE) platform. We employed the Theil-Sen trend analysis, the Mann-Kendall test, and the Hurst index to analyze the historical patterns and future trajectories of kNDVI. Residual analysis was then applied to determine the relative contributions of climate change and human activities to vegetation dynamics across China. The results show that from 2001 to 2020, vegetation in China showed a fluctuating but predominantly increasing trend, with a significant annual kNDVI growth rate of 0.002. The significant greening pattern was observed in over 48% of vegetated areas, exhibiting a clear spatial gradient with lower increases in the northwest and higher amplitudes in the southeast. Moreover, more than 60% of vegetation areas are projected to experience a sustained increase in the future. Residual analysis reveals that climate change contributed 21.89% to vegetation changes, while human activities accounted for 78.11%, being the dominant drivers of vegetation variation. This finding is further supported by partial correlation analysis between kNDVI and temperature, precipitation, and the human footprint. Vegetation dynamics were found to respond more strongly to human influences than to climate drivers, underscoring the leading role of human activities. Further analysis of tree cover fraction and cropping intensity data indicates that the greening in forests and croplands is primarily attributable to large-scale afforestation efforts and improved agricultural management.
Full article
(This article belongs to the Special Issue Quantitative Remote Sensing for Vegetation Phenology and Regional Landscape Patterns)
►▼
Show Figures
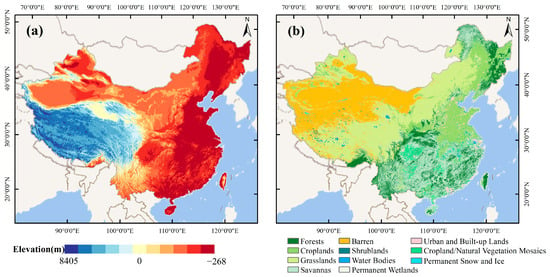
Figure 1
Open AccessArticle
A Joint Optimization Method for Power and Array of Multi-Point Sources System
by
Zhihao Cai, Shiqi Xing, Xinyuan Su, Junpeng Wang, Weize Meng and Ziwen Xiao
Remote Sens. 2025, 17(14), 2445; https://doi.org/10.3390/rs17142445 (registering DOI) - 14 Jul 2025
Abstract
In a multi-point source system, increasing the jamming power can expand the distribution area of the equivalent radiation center, but significantly increases the system exposure risk. Therefore, in order to achieve an optimal balance between the two, this paper proposes a joint optimization
[...] Read more.
In a multi-point source system, increasing the jamming power can expand the distribution area of the equivalent radiation center, but significantly increases the system exposure risk. Therefore, in order to achieve an optimal balance between the two, this paper proposes a joint optimization method for jamming power and an array of multi-point source systems. First, based on determining the spatial geometric relationship between the triplet antenna and the target, the distribution law of the equivalent radiation center of the triplet antenna under the condition of the target echo is derived. Second, by introducing the angle factor, the jamming power and equivalent radiation center distribution area are combined to construct the joint optimization model of jamming power and array in omnidirectional and non-omnidirectional situations. Third, based on the non-dominated sorting whale optimization algorithm (NSWOA), an adaptive inertia weight based on the cosine function and logistic chaotic map is introduced to obtain the optimal arrangement. The experimental results show that in the omnidirectional case, when the average jamming-to-signal ratio is 13.83 dB, the equilateral triangle array can achieve the goal of protecting the target while avoiding the exposure of the triplet antenna position. In the non-omnidirectional case, when the average jamming-to-signal ratio is 13.90 dB, the equilateral triangle array can achieve the optimal balance between the jamming power and the area of the distribution area of the equivalent radiation center, and control the distribution of the equivalent radiation center to strictly meet the preset angular domain constraints. Furthermore, the optimal JSR value was reduced by an average of 1.14 dB compared with that of the conventional selection scheme.
Full article
(This article belongs to the Special Issue Advancing Synthetic Aperture Radar: Imaging, Processing, and Applications in Remote Sensing)
►▼
Show Figures
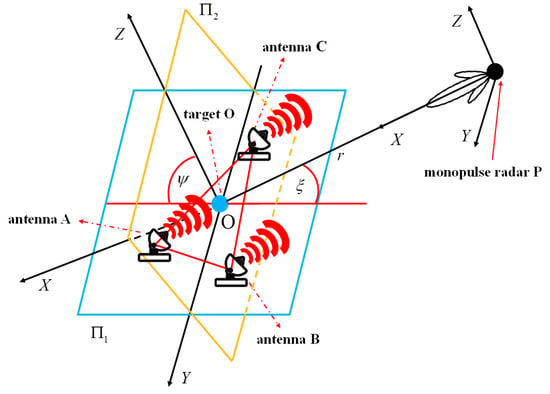
Figure 1
Open AccessArticle
Optimizing Hyperspectral Desertification Monitoring Through Metaheuristic-Enhanced Wavelet Packet Noise Reduction and Feature Band Selection
by
Weichao Liu, Jiapeng Xiao, Rongyuan Liu, Yan Liu, Yunzhu Tao, Tian Zhang, Fuping Gan, Ping Zhou, Yuanbiao Dong and Qiang Zhou
Remote Sens. 2025, 17(14), 2444; https://doi.org/10.3390/rs17142444 (registering DOI) - 14 Jul 2025
Abstract
Land desertification represents a significant and sensitive global ecological issue. In the Inner Mongolia region of China, soil desertification and salinization are widespread, resulting from the combined effects of extreme drought conditions and human activities. Using Gaofen 5B AHSI imagery as our data
[...] Read more.
Land desertification represents a significant and sensitive global ecological issue. In the Inner Mongolia region of China, soil desertification and salinization are widespread, resulting from the combined effects of extreme drought conditions and human activities. Using Gaofen 5B AHSI imagery as our data source, we collected spectral data for seven distinct land cover types: lush vegetation, yellow sand, white sand, saline soil, saline shell, saline soil with saline vegetation, and sandy soil. We applied Particle Swarm Optimization (PSO) to fine-tune the Wavelet Packet (WP) decomposition levels, thresholds, and wavelet basis function, ensuring optimal spectral decomposition and reconstruction. Subsequently, PSO was deployed to optimize key hyperparameters of the Random Forest algorithm and compare its performance with the ResNet-Transformer model. Our results indicate that PSO effectively automates the search for optimal WP decomposition parameters, preserving essential spectral information while efficiently reducing high-frequency spectral noise. The Genetic Algorithm (GA) was also found to be effective in extracting feature bands relevant to land desertification, which enhances the classification accuracy of the model. Among all the models, integrating wavelet packet denoising, genetic algorithm feature selection, the first-order differential (FD), and the hybrid architecture of the ResNet-Transformer, the WP-GA-FD-ResNet-Transformer model achieved the highest accuracy in extracting soil sandification and salinization, with Kappa coefficients and validation set accuracies of 0.9746 and 97.82%, respectively. This study contributes to the field by advancing hyperspectral desertification monitoring techniques and suggests that the approach could be valuable for broader ecological conservation and land management efforts.
Full article
(This article belongs to the Section Ecological Remote Sensing)
►▼
Show Figures
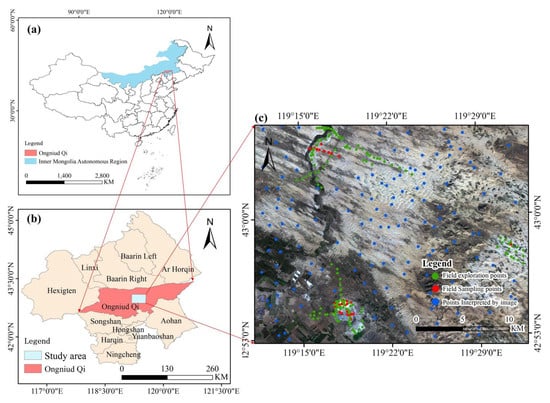
Figure 1
Open AccessArticle
AFNE-Net: Semantic Segmentation of Remote Sensing Images via Attention-Based Feature Fusion and Neighborhood Feature Enhancement
by
Ke Li, Hao Ji, Zhijiang Li, Zeyu Cui and Chengkai Liu
Remote Sens. 2025, 17(14), 2443; https://doi.org/10.3390/rs17142443 (registering DOI) - 14 Jul 2025
Abstract
Understanding remote sensing imagery is vital for object observation and planning. However, the acquisition of optical images is inevitably affected by shadows and occlusions, resulting in local discrepancies in object representation. To address these challenges, this paper proposes AFNE-Net, a general network architecture
[...] Read more.
Understanding remote sensing imagery is vital for object observation and planning. However, the acquisition of optical images is inevitably affected by shadows and occlusions, resulting in local discrepancies in object representation. To address these challenges, this paper proposes AFNE-Net, a general network architecture for remote sensing image segmentation. First, the model introduces an attention-based feature fusion module. Through the use of weighted fusion of multi-resolution features, this effectively expands the receptive field and enhances semantic associations between categories. Subsequently, a feature enhancement module based on the consistency of neighborhood semantic representation is introduced. This aims to improve the feature representation and reduce segmentation errors caused by local perturbations. Finally, evaluations are conducted on the ISPRS Potsdam, UAVid, and LoveDA datasets to verify the effectiveness of the proposed model.
Full article
(This article belongs to the Section AI Remote Sensing)
►▼
Show Figures
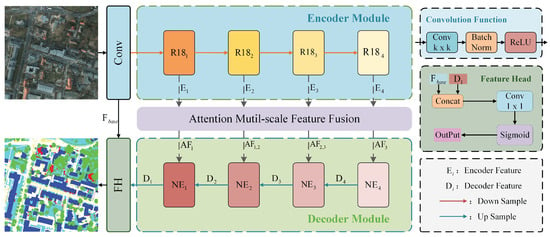
Figure 1
Open AccessArticle
A Multi-Task Learning Framework with Enhanced Cross-Level Semantic Consistency for Multi-Level Land Cover Classification
by
Shilin Tao, Haoyu Fu, Ruiqi Yang and Leiguang Wang
Remote Sens. 2025, 17(14), 2442; https://doi.org/10.3390/rs17142442 (registering DOI) - 14 Jul 2025
Abstract
►▼
Show Figures
The multi-scale characteristics of remote sensing imagery have an inherent correspondence with the hierarchical structure of land cover classification systems, providing a theoretical foundation for multi-level land cover classification. However, most existing methods treat classification tasks at different semantic levels as independent processes,
[...] Read more.
The multi-scale characteristics of remote sensing imagery have an inherent correspondence with the hierarchical structure of land cover classification systems, providing a theoretical foundation for multi-level land cover classification. However, most existing methods treat classification tasks at different semantic levels as independent processes, overlooking the semantic relationships among these levels, which leads to semantic inconsistencies and structural conflicts in classification results. We addressed this issue with a deep multi-task learning (MTL) framework, named MTL-SCH, which enables collaborative classification across multiple semantic levels. MTL-SCH employs a shared encoder combined with a feature cascade mechanism to boost information sharing and collaborative optimization between two levels. A hierarchical loss function is also embedded that explicitly models the semantic dependencies between levels, enhancing semantic consistency across levels. Two new evaluation metrics, namely Semantic Alignment Deviation (SAD) and Enhancing Semantic Alignment Deviation (ESAD), are also proposed to quantify the improvement of MTL-SCH in semantic consistency. In the experimental section, MTL-SCH is applied to different network models, including CNN, Transformer, and CNN-Transformer models. The results indicate that MTL-SCH improves classification accuracy in coarse- and fine-level segmentation tasks, significantly enhancing semantic consistency across levels and outperforming traditional flat segmentation methods.
Full article
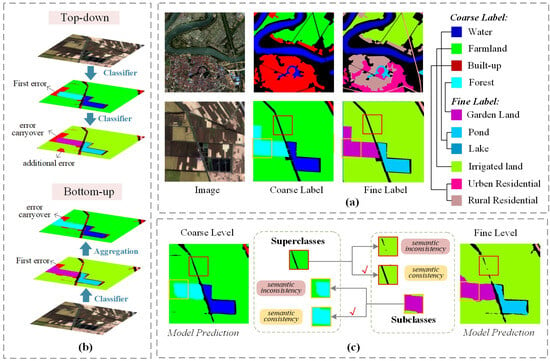
Figure 1
Open AccessArticle
SR-YOLO: Spatial-to-Depth Enhanced Multi-Scale Attention Network for Small Target Detection in UAV Aerial Imagery
by
Shasha Zhao, He Chen, Di Zhang, Yiyao Tao, Xiangnan Feng and Dengyin Zhang
Remote Sens. 2025, 17(14), 2441; https://doi.org/10.3390/rs17142441 (registering DOI) - 14 Jul 2025
Abstract
The detection of aerial imagery captured by Unmanned Aerial Vehicles (UAVs) is widely employed across various domains, including engineering construction, traffic regulation, and precision agriculture. However, aerial images are typically characterized by numerous small targets, significant occlusion issues, and densely clustered targets, rendering
[...] Read more.
The detection of aerial imagery captured by Unmanned Aerial Vehicles (UAVs) is widely employed across various domains, including engineering construction, traffic regulation, and precision agriculture. However, aerial images are typically characterized by numerous small targets, significant occlusion issues, and densely clustered targets, rendering traditional detection algorithms largely ineffective for such imagery. This work proposes a small target detection algorithm, SR-YOLO. It is specifically tailored to address these challenges in UAV-captured aerial images. First, the Space-to-Depth layer and Receptive Field Attention Convolution are combined, and the SR-Conv module is designed to replace the Conv module within the original backbone network. This hybrid module extracts more fine-grained information about small target features by converting image spatial information into depth information and the attention of the network to targets of different scales. Second, a small target detection layer and a bidirectional feature pyramid network mechanism are introduced to enhance the neck network, thereby strengthening the feature extraction and fusion capabilities for small targets. Finally, the model’s detection performance for small targets is improved by utilizing the Normalized Wasserstein Distance loss function to optimize the Complete Intersection over Union loss function. Empirical results demonstrate that the SR-YOLO algorithm significantly enhances the precision of small target detection in UAV aerial images. Ablation experiments and comparative experiments are conducted on the VisDrone2019 and RSOD datasets. Compared to the baseline algorithm YOLOv8s, our SR-YOLO algorithm has improved mAP@0.5 by 6.3% and 3.5% and mAP@0.5:0.95 by 3.8% and 2.3% on the datasets VisDrone2019 and RSOD, respectively. It also achieves superior detection results compared to other mainstream target detection methods.
Full article
(This article belongs to the Special Issue Recent Advances in Object Detection with Hyperspectral Remote Sensing Data)
►▼
Show Figures
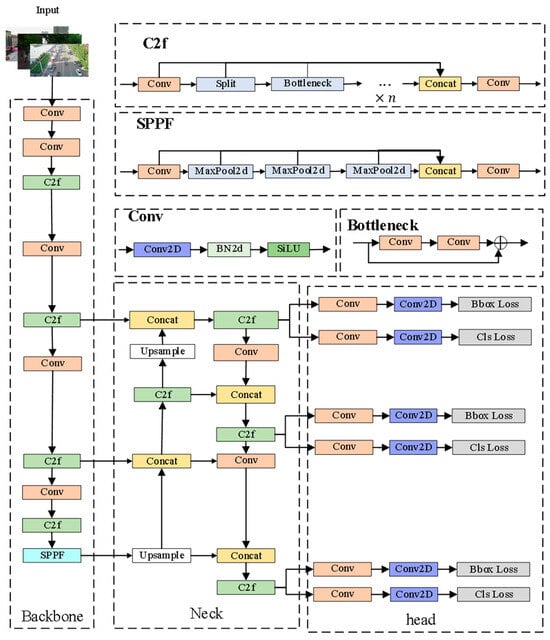
Figure 1
Open AccessArticle
Spatial and Temporal Expansion of Photovoltaic Sites and Thermal Environmental Effects in Ningxia Based on Remote Sensing and Deep Learning
by
Heao Xie, Peixian Li, Fang Shi, Chengting Han, Ximin Cui and Yuling Zhao
Remote Sens. 2025, 17(14), 2440; https://doi.org/10.3390/rs17142440 (registering DOI) - 14 Jul 2025
Abstract
►▼
Show Figures
Ningxia has emerged as a strategic hub for China’s photovoltaic (PV) industry by leveraging abundant solar energy resources and geoclimatic advantages. This study analyzed the spatiotemporal expansion trends and microclimatic impacts of PV installations (2015–2024) using Gaofen-1 (GF-1) and Landsat8 satellite imagery with
[...] Read more.
Ningxia has emerged as a strategic hub for China’s photovoltaic (PV) industry by leveraging abundant solar energy resources and geoclimatic advantages. This study analyzed the spatiotemporal expansion trends and microclimatic impacts of PV installations (2015–2024) using Gaofen-1 (GF-1) and Landsat8 satellite imagery with deep learning algorithms and multidimensional environmental metrics. Among semantic segmentation models, DeepLabV3+ had the best performance in PV extraction, and the Mean Intersection over Union, precision, and F1-score were 91.97%, 89.02%, 89.2%, and 89.11%, respectively, with accuracies close to 100% after manual correction. Subsequent land surface temperature inversion and spatial buffer analysis quantified the thermal environmental effects of PV installation. Localized cooling patterns may be influenced by albedo and vegetation dynamics, though further validation is needed. The total PV site area in Ningxia expanded from 59.62 km2 to 410.06 km2 between 2015 and 2024. Yinchuan and Wuzhong cities were primary growth hubs; Yinchuan alone added 99.98 km2 (2022–2023) through localized policy incentives. PV installations induced significant daytime cooling effects within 0–100 m buffers, reducing ambient temperatures by 0.19–1.35 °C on average. The most pronounced cooling occurred in western desert regions during winter (maximum temperature differential = 1.97 °C). Agricultural zones in central Ningxia exhibited weaker thermal modulation due to coupled vegetation–PV interactions. Policy-driven land use optimization was the dominant catalyst for PV proliferation. This study validates “remote sensing + deep learning” framework efficacy in renewable energy monitoring and provides empirical insights into eco-environmental impacts under “PV + ecological restoration” paradigms, offering critical data support for energy–ecology synergy planning in arid regions.
Full article
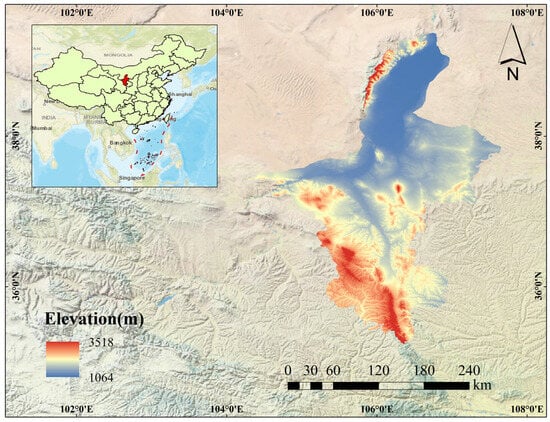
Figure 1
Open AccessArticle
Assessment of Aerosol Optical Depth, Cloud Fraction, and Liquid Water Path in CMIP6 Models Using Satellite Observations
by
Jiakun Liang and Jennifer D. Small Griswold
Remote Sens. 2025, 17(14), 2439; https://doi.org/10.3390/rs17142439 (registering DOI) - 14 Jul 2025
Abstract
Aerosols are critical to the Earth’s atmosphere, influencing climate through interactions with solar radiation and clouds. However, accurately replicating the interactions between aerosols and clouds remains challenging due to the complexity of the physical processes involved. This study evaluated the performance of Coupled
[...] Read more.
Aerosols are critical to the Earth’s atmosphere, influencing climate through interactions with solar radiation and clouds. However, accurately replicating the interactions between aerosols and clouds remains challenging due to the complexity of the physical processes involved. This study evaluated the performance of Coupled Model Intercomparison Project phase 6 (CMIP6) models in simulating aerosol optical depth (AOD), cloud fraction (CF), and liquid water path (LWP) by comparing them with satellite observations from MODIS and AMSR-E. Using 30 years of CMIP6 model simulations and available satellite observations during the satellite era, the results show that most CMIP6 models underestimate CF and LWP by 24.3% for LWP in the Northern Hemisphere. An assessment of spatial patterns indicates that models generally align more closely with observations in the Northern Hemisphere than in the Southern Hemisphere. Latitudinal profiles reveal that while most models capture the overall distribution patterns, they struggle to accurately reproduce observed magnitudes. A quantitative scoring system is applied to evaluate each model’s ability to replicate the spatial characteristics of multi-year mean aerosol and cloud properties. Overall, the findings suggest that CMIP6 models perform better in simulating AOD and CF than LWP, particularly in the Southern Hemisphere.
Full article
(This article belongs to the Special Issue Advances in Atmospheric Aerosol Monitoring Based on Lidar and Satellites)
►▼
Show Figures
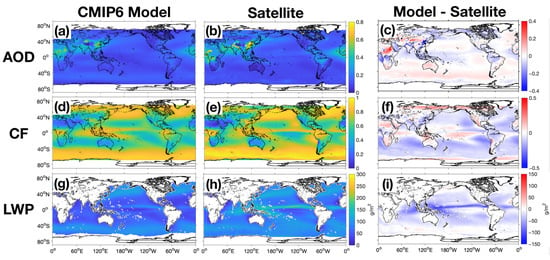
Figure 1
Open AccessArticle
VJDNet: A Simple Variational Joint Discrimination Network for Cross-Image Hyperspectral Anomaly Detection
by
Shiqi Wu, Xiangrong Zhang, Guanchun Wang, Puhua Chen, Jing Gu, Xina Cheng and Licheng Jiao
Remote Sens. 2025, 17(14), 2438; https://doi.org/10.3390/rs17142438 (registering DOI) - 14 Jul 2025
Abstract
►▼
Show Figures
To enhance the generalization of networks and avoid redundant training efforts, cross-image hyperspectral anomaly detection (HAD) based on deep learning has been gradually studied in recent years. Cross-image HAD aims to perform anomaly detection on unknown hyperspectral images after a single training process
[...] Read more.
To enhance the generalization of networks and avoid redundant training efforts, cross-image hyperspectral anomaly detection (HAD) based on deep learning has been gradually studied in recent years. Cross-image HAD aims to perform anomaly detection on unknown hyperspectral images after a single training process on the network, thereby improving detection efficiency in practical applications. However, the existing approaches may require additional supervised information or stacking of networks to improve model performance, which may impose high demands on data or hardware in practical applications. In this paper, a simple and lightweight unsupervised cross-image HAD method called Variational Joint Discrimination Network (VJDNet) is proposed. We leverage the reconstruction and distribution representation ability of the variational autoencoder (VAE), learning the global and local discriminability of anomalies jointly. To integrate these representations from the VAE, a probability distribution joint discrimination (PDJD) module is proposed. Through the PDJD module, the VJDNet can directly output the anomaly score mask of pixels. To further facilitate the unsupervised paradigm, a sample pair generation module is proposed, which is able to generate anomaly samples and background representation samples tailored for the cross-image HAD task. The experimental results show that the proposed method is able to maintain the detection accuracy with only a small number of parameters.
Full article
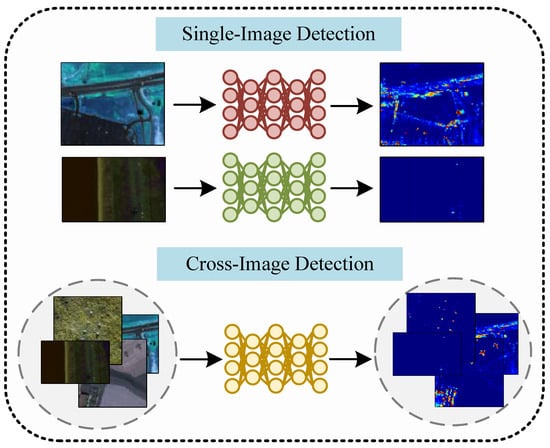
Figure 1
Open AccessArticle
Methodology for Evaluating Collision Avoidance Maneuvers Using Aerodynamic Control
by
Desiree González Rodríguez, Pedro Orgeira-Crespo, Jose M. Nuñez-Ortuño and Fernando Aguado-Agelet
Remote Sens. 2025, 17(14), 2437; https://doi.org/10.3390/rs17142437 (registering DOI) - 14 Jul 2025
Abstract
The increasing congestion of low Earth orbit (LEO) has raised the need for efficient collision avoidance strategies, especially for CubeSats without propulsion systems. This study proposes a methodology for evaluating passive collision avoidance maneuvers using aerodynamic control via a satellite’s Attitude Determination and
[...] Read more.
The increasing congestion of low Earth orbit (LEO) has raised the need for efficient collision avoidance strategies, especially for CubeSats without propulsion systems. This study proposes a methodology for evaluating passive collision avoidance maneuvers using aerodynamic control via a satellite’s Attitude Determination and Control System (ADCS). By adjusting orientation, the satellite modifies its exposed surface area, altering atmospheric drag and lift forces to shift its orbit. This new approach integrates atmospheric modeling (NRLMSISE-00), aerodynamic coefficient estimation using the ADBSat panel method, and orbital simulations in Systems Tool Kit (STK). The LUME-1 CubeSat mission is used as a reference case, with simulations at three altitudes (500, 460, and 420 km). Results show that attitude-induced drag modulation can generate significant orbital displacements—measured by Horizontal and Vertical Distance Differences (HDD and VDD)—sufficient to reduce collision risk. Compared to constant-drag models, the panel method offers more accurate, orientation-dependent predictions. While lift forces are minor, their inclusion enhances modeling fidelity. This methodology supports the development of low-resource, autonomous collision avoidance systems for future CubeSat missions, particularly in remote sensing applications where orbital precision is essential.
Full article
(This article belongs to the Special Issue Advances in CubeSat Missions and Applications in Remote Sensing)
►▼
Show Figures
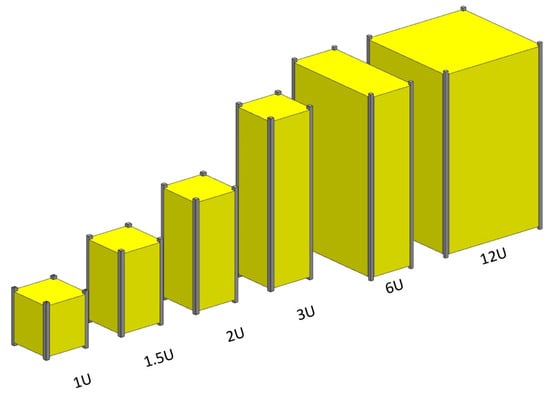
Figure 1
Open AccessArticle
Study on the Effect of Shortwave Radiation in Land Surface Temperature Downscaling over Rugged Terrain
by
Shumin Wang, Jie Cheng and Qiang Liu
Remote Sens. 2025, 17(14), 2436; https://doi.org/10.3390/rs17142436 - 14 Jul 2025
Abstract
Land surface temperature (LST) is an important parameter in the surface system with drastic variation in spatial and temporal domains. The protection of the ecological environment in mountainous areas and the monitoring of natural disasters require the support of surface temperature data with
[...] Read more.
Land surface temperature (LST) is an important parameter in the surface system with drastic variation in spatial and temporal domains. The protection of the ecological environment in mountainous areas and the monitoring of natural disasters require the support of surface temperature data with high spatiotemporal resolution. LST downscaling is an effective method to improve the spatial and temporal resolution of remote sensing LST data. However, at present, the LST downscaling research mainly focuses on plain and urban areas, while the area of rugged terrain is less studied, and the accuracy of LST in rugged terrain is lower than in plain and urban areas. In the few studies that discuss auxiliary parameters for LST downscaling in rugged terrain, only elevation is considered as an auxiliary parameter. In this study, we selected parameters that have evident correlation with LST as potential auxiliary factors and discussed the benefits of adding shortwave radiation to the LST downscaling process. We chose four scene images in the Beijing suburbs and the Loess Plateau and conducted the LST downscaling experiments. In this study, we used the Taylor expansion method for LST downscaling. We selected Landsat 8 and MODSI LST data as fine and coarse study datasets, respectively. The results show that the accuracy of LST downscaling in rugged terrain areas can be improved by using elevation and shortwave radiation as auxiliary factors, and the benefits of shortwave radiation is independent of that of elevation. Therefore, it is suggested that these two parameters be simultaneously used to achieve the best LST downscaling result over rugged terrain areas.
Full article
(This article belongs to the Special Issue Land Surface Temperature Estimation Using Remote Sensing II)
►▼
Show Figures
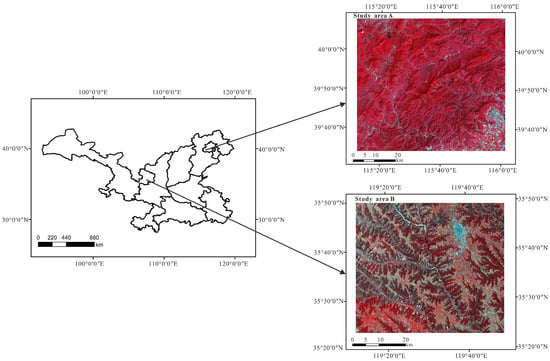
Figure 1
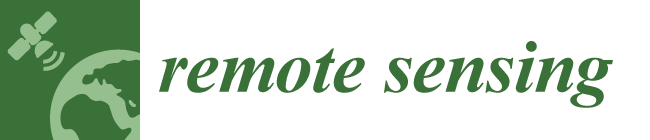
Journal Menu
► ▼ Journal Menu-
- Remote Sensing Home
- Aims & Scope
- Editorial Board
- Reviewer Board
- Topical Advisory Panel
- Photography Exhibition
- Instructions for Authors
- Special Issues
- Topics
- Sections & Collections
- Article Processing Charge
- Indexing & Archiving
- Editor’s Choice Articles
- Most Cited & Viewed
- Journal Statistics
- Journal History
- Journal Awards
- Society Collaborations
- Conferences
- Editorial Office
Journal Browser
► ▼ Journal BrowserHighly Accessed Articles
Latest Books
E-Mail Alert
News
Topics
Topic in
AI, BDCC, Fire, GeoHazards, Remote Sensing
AI for Natural Disasters Detection, Prediction and Modeling
Topic Editors: Moulay A. Akhloufi, Mozhdeh ShahbaziDeadline: 25 July 2025
Topic in
Aerospace, Automation, Drones, Remote Sensing, Sensors
Target Tracking, Guidance, and Navigation for Autonomous Systems, 2nd Edition
Topic Editors: Won-Sang Ra, Shaoming He, Ivan MasmitjaDeadline: 20 August 2025
Topic in
Drones, Electronics, Remote Sensing, Sensors, Smart Cities
Advanced Array Signal Processing for B5G/6G: Models, Algorithms, and Applications
Topic Editors: Fangqing Wen, Xianpeng Wang, Jin He, Liangtian Wan, Zhiyuan ZhaDeadline: 31 August 2025
Topic in
Conservation, Diversity, JMSE, Remote Sensing, Technologies
Technological Innovation to Support Reef Research and Conservation
Topic Editors: Atsuko Fukunaga, John BurnsDeadline: 15 September 2025

Conferences
Special Issues
Special Issue in
Remote Sensing
Geological Hazard Monitoring, Identify, Predict, and Risk Assessment Using Geographic Information Science and Remote Sensing
Guest Editors: Yan Zhao, Yongsheng Li, Yi ZhangDeadline: 25 July 2025
Special Issue in
Remote Sensing
Advances in Multimodal Remote Sensing Data: Processing, Fusion and Applications
Guest Editors: Luomei Yixiang, Fa-Ming FangDeadline: 25 July 2025
Special Issue in
Remote Sensing
Geomatics and Natural Hazards
Guest Editors: Lisa Borgatti, Enrico Corrado Borgogno Mondino, Alessandro Corsini, Daniele Giordan, Stefano Gandolfi, Mauro SoldatiDeadline: 25 July 2025
Special Issue in
Remote Sensing
Advances in Spectral Imagery and Methods for Fire and Smoke Detection
Guest Editor: Eriita JonesDeadline: 28 July 2025
Topical Collections
Topical Collection in
Remote Sensing
Feature Paper Special Issue on Forest Remote Sensing
Collection Editors: Zengyuan Li, Erxue Chen, Lin Cao
Topical Collection in
Remote Sensing
Sentinel-2: Science and Applications
Collection Editors: Clement Atzberger, Jadu Dash, Olivier Hagolle, Jochem Verrelst, Quinten Vanhellemont, Jordi Inglada, Tuomas Häme
Topical Collection in
Remote Sensing
Discovering A More Diverse Remote Sensing Discipline
Collection Editors: Karen Joyce, Meghan Halabisky, Cristina Gómez, Michelle Kalamandeen, Gopika Suresh, Kate C. Fickas
Topical Collection in
Remote Sensing
The VIIRS Collection: Calibration, Validation, and Application
Collection Editors: Xi Shao, Xiaoxiong Xiong, Changyong Cao