Topic Editors
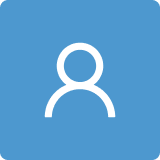

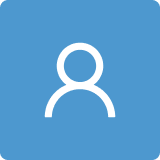
Challenges, Development and Frontiers of Smart Agriculture and Forestry—2nd Volume
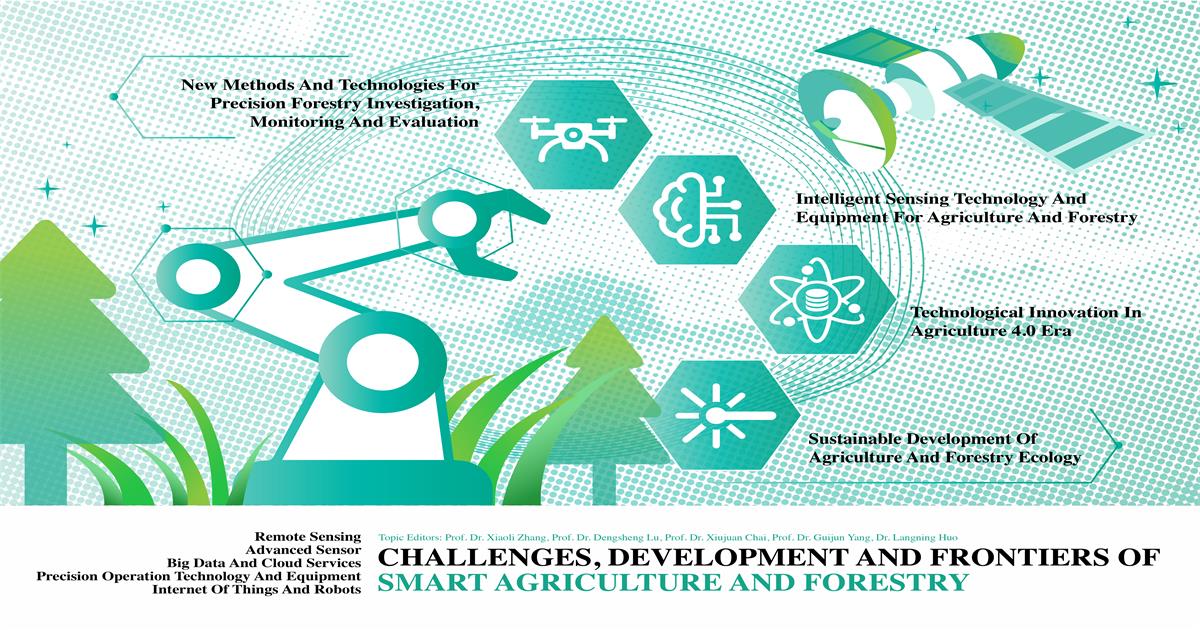
Topic Information
Dear Colleagues,
As agriculture and forestry enter the digital era, the digital technology related to these topics has started to become widespread. This is referred to as smart agriculture and forestry, which is characterized by “information + knowledge + intelligent equipment”, realizing the deep cross-domain integration of modern information technology and agriculture and forestry. In the future, the development of smart agriculture and forestry will focus on information and intelligent technology to build an integrated platform for smart perception, wireless transmission, smart decision-making and intelligent control, so as to realize the intelligent supervision of the entire agricultural and forestry industry chain (production, processing, operation, management and service), and finally achieve cost reduction, quality improvements and increased efficiency. The rapid development of artificial intelligence, UAV, remote sensing, big data analysis and other technologies provides a substantial support for agriculture and forestry to move forward to the intelligent era.
We are pleased to announce the opening of Volume II, “Challenges, Development and Frontiers of Smart Agriculture and Forestry-II”, which will follow on from Volume I. In this second edition, we aim to fully explore frontier technologies, including the internet of things, multi-modal and multi-angle stereo observation, cloud computing, big data, machine learning, intelligent decision-making, etc., thereby exploring their application potential in smart agriculture and forestry and accelerating the development of precision agriculture and forestry.
This Topic welcomes high-quality works that focus on the innovation of five core technologies, including remote sensing, advanced sensors, big data and cloud services, precision operation technology and equipment, internet of things and robots, as well as the solutions, strategies, pilot cases and examples of these frontier technologies applied in the fields of forest and farmland investigation and change monitoring, plant protection, disaster prevention, precision cultivation, visual management, ecological protection and reconstruction, and evaluation of ecological service value. Relevant themes include, but are not limited to, the following:
Intelligent sensing technology and equipment for agriculture and forestry
- Advanced agroforestry sensors and data analysis;
- Intelligent robot integrating cloud computing, big data and machine learning technology;
- Intelligent agricultural and forestry machinery equipment and technology;
- Development and test of agricultural and forestry intelligent equipment.
New methods and technologies for precision forestry investigation, monitoring and evaluation
- New methods and technology of forest resources investigation based on multi-source remote sensing;
- Forest change and disturbance detection using multi-modal remote sensing technology;
- Forest degradation-restoration monitoring combining remote sensing and geographic big data;
- Forest disaster monitoring and prediction integrating remote sensing and geographic big data;
- Precision estimation and modelling of forest structure and function parameters;
- Forest visualization and management.
Technological innovation in agriculture 4.0 era
- Innovative sensors and technologies for smart agriculture;
- Application of deep learning and geographic big data in smart breeding and germplasm resources;
- Smart technologies and decision support for precision orchard production;
- Multi technology integration for high-throughput plant phenotyping;
- Intelligent in situ monitoring and decision-making of crop typical stress.
Sustainable development of agriculture and forestry ecology
- Assessment of ecosystem service function based on geographic big data analysis;
- Precise estimation of agricultural and forestry carbon storage;
- Vegetation species diversity evaluation based on remote sensing and machine learning;
- Agriculture and forestry approaches and contributions to achieve carbon neutralization;
- Challenges and opportunities to the construction of smart agriculture and forestry.
Prof. Dr. Xiaoli Zhang
Prof. Dr. Dengsheng Lu
Prof. Dr. Xiujuan Chai
Prof. Dr. Guijun Yang
Dr. Langning Huo
Topic Editors
Keywords
- precision investigation
- smart agriculture and forestry
- intelligent perception
- artificial intelligence
- remote sensing
- big data
- decision-making
- carbon storage estimation
Participating Journals
Journal Name | Impact Factor | CiteScore | Launched Year | First Decision (median) | APC | |
---|---|---|---|---|---|---|
![]()
Agriculture
|
3.3 | 4.9 | 2011 | 19.2 Days | CHF 2600 | Submit |
![]()
Agronomy
|
3.3 | 6.2 | 2011 | 17.6 Days | CHF 2600 | Submit |
![]()
Forests
|
2.4 | 4.4 | 2010 | 16.2 Days | CHF 2600 | Submit |
![]()
Remote Sensing
|
4.2 | 8.3 | 2009 | 23.9 Days | CHF 2700 | Submit |
![]()
Sustainability
|
3.3 | 6.8 | 2009 | 19.7 Days | CHF 2400 | Submit |
Preprints.org is a multidisciplinary platform offering a preprint service designed to facilitate the early sharing of your research. It supports and empowers your research journey from the very beginning.
MDPI Topics is collaborating with Preprints.org and has established a direct connection between MDPI journals and the platform. Authors are encouraged to take advantage of this opportunity by posting their preprints at Preprints.org prior to publication:
- Share your research immediately: disseminate your ideas prior to publication and establish priority for your work.
- Safeguard your intellectual contribution: Protect your ideas with a time-stamped preprint that serves as proof of your research timeline.
- Boost visibility and impact: Increase the reach and influence of your research by making it accessible to a global audience.
- Gain early feedback: Receive valuable input and insights from peers before submitting to a journal.
- Ensure broad indexing: Web of Science (Preprint Citation Index), Google Scholar, Crossref, SHARE, PrePubMed, Scilit and Europe PMC.