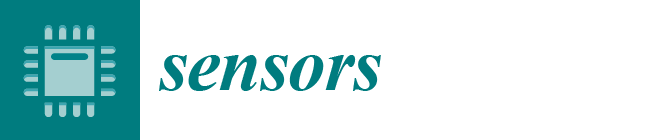
Journal Menu
► ▼ Journal Menu-
- Sensors Home
- Aims & Scope
- Editorial Board
- Reviewer Board
- Topical Advisory Panel
- Instructions for Authors
- Special Issues
- Topics
- Sections & Collections
- Article Processing Charge
- Indexing & Archiving
- Editor’s Choice Articles
- Most Cited & Viewed
- Journal Statistics
- Journal History
- Journal Awards
- Society Collaborations
- Conferences
- Editorial Office
Journal Browser
► ▼ Journal Browser-
arrow_forward_ios
Forthcoming issue
arrow_forward_ios Current issue - Vol. 24 (2024)
- Vol. 23 (2023)
- Vol. 22 (2022)
- Vol. 21 (2021)
- Vol. 20 (2020)
- Vol. 19 (2019)
- Vol. 18 (2018)
- Vol. 17 (2017)
- Vol. 16 (2016)
- Vol. 15 (2015)
- Vol. 14 (2014)
- Vol. 13 (2013)
- Vol. 12 (2012)
- Vol. 11 (2011)
- Vol. 10 (2010)
- Vol. 9 (2009)
- Vol. 8 (2008)
- Vol. 7 (2007)
- Vol. 6 (2006)
- Vol. 5 (2005)
- Vol. 4 (2004)
- Vol. 3 (2003)
- Vol. 2 (2002)
- Vol. 1 (2001)
Need Help?
Editor’s Choice Articles
Editor’s Choice articles are based on recommendations by the scientific editors of MDPI journals from around the world. Editors select a small number of articles recently published in the journal that they believe will be particularly interesting to readers, or important in the respective research area. The aim is to provide a snapshot of some of the most exciting work published in the various research areas of the journal.