Journal Description
Informatics
Informatics
is an international, peer-reviewed, open access journal on information and communication technologies, human–computer interaction, and social informatics, and is published quarterly online by MDPI.
- Open Access— free for readers, with article processing charges (APC) paid by authors or their institutions.
- High Visibility: indexed within Scopus, ESCI (Web of Science), dblp, and other databases.
- Journal Rank: JCR - Q2 (Computer Science, Interdisciplinary Applications) / CiteScore - Q1 (Communication)
- Rapid Publication: manuscripts are peer-reviewed and a first decision is provided to authors approximately 33 days after submission; acceptance to publication is undertaken in 5.7 days (median values for papers published in this journal in the first half of 2024).
- Recognition of Reviewers: reviewers who provide timely, thorough peer-review reports receive vouchers entitling them to a discount on the APC of their next publication in any MDPI journal, in appreciation of the work done.
Impact Factor:
3.4 (2023);
5-Year Impact Factor:
3.1 (2023)
Latest Articles
AI Literacy and Intention to Use Text-Based GenAI for Learning: The Case of Business Students in Korea
Informatics 2024, 11(3), 54; https://doi.org/10.3390/informatics11030054 - 26 Jul 2024
Abstract
►
Show Figures
With the increasing use of large-scale language model-based AI tools in modern learning environments, it is important to understand students’ motivations, experiences, and contextual influences. These tools offer new support dimensions for learners, enhancing academic achievement and providing valuable resources, but their use
[...] Read more.
With the increasing use of large-scale language model-based AI tools in modern learning environments, it is important to understand students’ motivations, experiences, and contextual influences. These tools offer new support dimensions for learners, enhancing academic achievement and providing valuable resources, but their use also raises ethical and social issues. In this context, this study aims to systematically identify factors influencing the usage intentions of text-based GenAI tools among undergraduates. By modifying the core variables of the Unified Theory of Acceptance and Use of Technology (UTAUT) with AI literacy, a survey was designed to measure GenAI users’ intentions to collect participants’ opinions. The survey, conducted among business students at a university in South Korea, gathered 239 responses during March and April 2024. Data were analyzed using Partial Least Squares Structural Equation Modeling (PLS-SEM) with SmartPLS software (Ver. 4.0.9.6). The findings reveal that performance expectancy significantly affects the intention to use GenAI, while effort expectancy does not. In addition, AI literacy and social influence significantly influence performance, effort expectancy, and the intention to use GenAI. This study provides insights into determinants affecting GenAI usage intentions, aiding the development of effective educational strategies and policies to support ethical and beneficial AI use in academic settings.
Full article
Open AccessArticle
A Comparative Analysis of Virtual Education Technology, E-Learning Systems Research Advances, and Digital Divide in the Global South
by
Ikpe Justice Akpan, Onyebuchi Felix Offodile, Aloysius Chris Akpanobong and Yawo Mamoua Kobara
Informatics 2024, 11(3), 53; https://doi.org/10.3390/informatics11030053 - 23 Jul 2024
Abstract
►▼
Show Figures
This pioneering study evaluates the digital divide and advances in virtual education (VE) and e-learning research in the Global South Countries (GSCs). Using metadata from bibliographic and World Bank data on research and development (R&D), we conduct quantitative bibliometric performance analyses and evaluate
[...] Read more.
This pioneering study evaluates the digital divide and advances in virtual education (VE) and e-learning research in the Global South Countries (GSCs). Using metadata from bibliographic and World Bank data on research and development (R&D), we conduct quantitative bibliometric performance analyses and evaluate the connection between R&D expenditures on VE/e-learning research advances in GSCs. The results show that ‘East Asia and the Pacific’ (EAP) spent significantly more on (R&D) and achieved the highest scientific literature publication (SLP), with significant impacts. Other GSCs’ R&D expenditure was flat until 2020 (during COVID-19), when R&D funding increased, achieving a corresponding 42% rise in SLPs. About 67% of ‘Arab States’ (AS) SLPs and 60% of citation impact came from SLPs produced from global north and other GSCs regions, indicating high dependence. Also, 51% of high-impact SLPs were ‘Multiple Country Publications’, mainly from non-GSC institutions, indicating high collaboration impact. The EAP, AS, and ‘South Asia’ (SA) regions experienced lower disparity. In contrast, the less developed countries (LDCs), including ‘Sub-Sahara Africa’, ‘Latin America and the Caribbean’, and ‘Europe (Eastern) and Central Asia’, showed few dominant countries with high SLPs and higher digital divides. We advocate for increased educational research funding to enhance innovative R&D in GSCs, especially in LDCs.
Full article
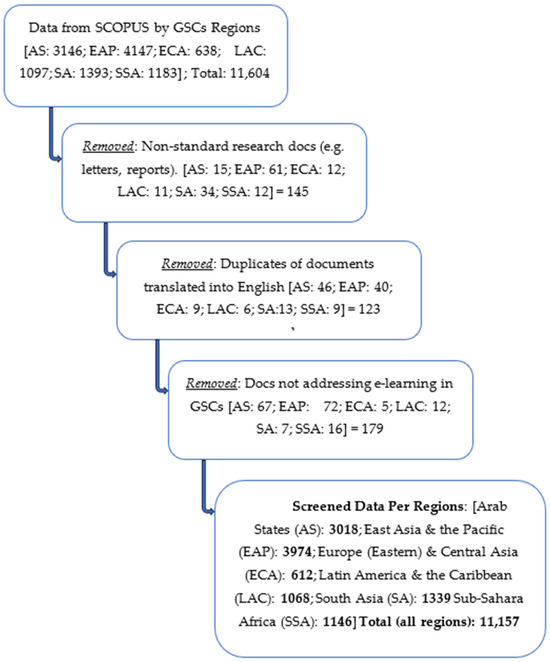
Figure 1
Open AccessArticle
Use of Chipless Radio Frequency Identification Technology for Smart Food Packaging: An Economic Analysis for an Australian Seafood Industry
by
Parya Fathi, Mita Bhattacharya, Sankar Bhattacharya and Nemai Karmakar
Informatics 2024, 11(3), 52; https://doi.org/10.3390/informatics11030052 - 22 Jul 2024
Abstract
►▼
Show Figures
Effective monitoring of perishable food products has become increasingly important for ensuring quality, enabling smart packaging to be a key consideration for food companies. Among the promising technologies available for transforming packaging into intelligent packaging, chipless radio frequency identification (RFID) sensors stand out.
[...] Read more.
Effective monitoring of perishable food products has become increasingly important for ensuring quality, enabling smart packaging to be a key consideration for food companies. Among the promising technologies available for transforming packaging into intelligent packaging, chipless radio frequency identification (RFID) sensors stand out. Despite the high initial implementation costs associated with chipless RFID technology, the potential benefits could outweigh the costs if electrical challenges can be overcome. We examine various economic methods to analyze the economic benefits of chipless RFID technology, evaluating the benefits of using this technology for the quality monitoring of seafood products of an Australian seafood producer, Tassal. The analysis considers three primary business drivers, viz. quality monitoring, operational efficiency, and tracking and tracing, using net present value and return on investment as the key indicators to assess the feasibility of implementing the technology. Based on sensitivity analysis, we suggest chipless RFID technology is currently best suited for large firms facing significant quality monitoring and operational efficiency challenges. However, as the cost of chipless RFID sensors decreases with further development, this technology may become a more viable option for small businesses in the future.
Full article
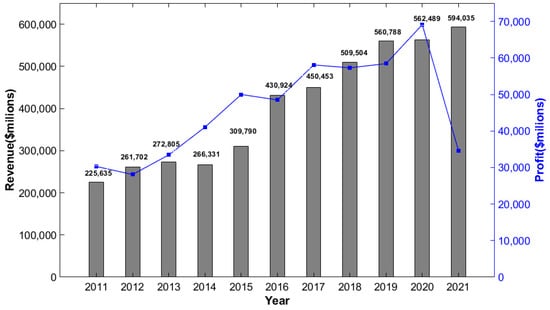
Figure 1
Open AccessArticle
Non-Invasive Diagnostic Approach for Diabetes Using Pulse Wave Analysis and Deep Learning
by
Hiruni Gunathilaka, Rumesh Rajapaksha, Thosini Kumarika, Dinusha Perera, Uditha Herath, Charith Jayathilaka, Janitha Liyanage and Sudath Kalingamudali
Informatics 2024, 11(3), 51; https://doi.org/10.3390/informatics11030051 - 19 Jul 2024
Abstract
The surging prevalence of diabetes globally necessitates advancements in non-invasive diagnostics, particularly for the early detection of cardiovascular anomalies associated with the condition. This study explores the efficacy of Pulse Wave Analysis (PWA) for distinguishing diabetic from non-diabetic individuals through morphological examination of
[...] Read more.
The surging prevalence of diabetes globally necessitates advancements in non-invasive diagnostics, particularly for the early detection of cardiovascular anomalies associated with the condition. This study explores the efficacy of Pulse Wave Analysis (PWA) for distinguishing diabetic from non-diabetic individuals through morphological examination of pressure pulse waveforms. The research unfolds in four phases: data accrual, preprocessing, Convolutional Neural Network (CNN) model construction, and performance evaluation. Data were procured using a multipara patient monitor, resulting in 2000 pulse waves equally divided between healthy individuals and those with diabetes. These were used to train, validate, and test three distinct CNN architectures: the conventional CNN, Visual Geometry Group (VGG16), and Residual Networks (ResNet18). The accuracy, precision, recall, and F1 score gauged each model’s proficiency. The CNN demonstrated a training accuracy of 82.09% and a testing accuracy of 80.6%. The VGG16, with its deeper structure, surpassed the baseline with training and testing accuracies of 90.2% and 86.57%, respectively. ResNet18 excelled, achieving a training accuracy of 92.50% and a testing accuracy of 92.00%, indicating its robustness in pattern recognition within pulse wave data. Deploying deep learning for diabetes screening marks progress, suggesting clinical use and future studies on bigger datasets for refinement.
Full article
(This article belongs to the Section Medical and Clinical Informatics)
►▼
Show Figures
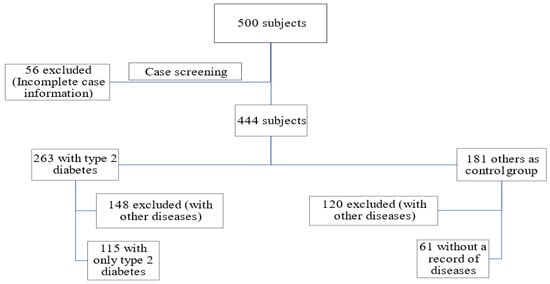
Figure 1
Open AccessArticle
Machine Learning to Estimate Workload and Balance Resources with Live Migration and VM Placement
by
Taufik Hidayat, Kalamullah Ramli, Nadia Thereza, Amarudin Daulay, Rushendra Rushendra and Rahutomo Mahardiko
Informatics 2024, 11(3), 50; https://doi.org/10.3390/informatics11030050 - 19 Jul 2024
Abstract
►▼
Show Figures
Currently, utilizing virtualization technology in data centers often imposes an increasing burden on the host machine (HM), leading to a decline in VM performance. To address this issue, live virtual migration (LVM) is employed to alleviate the load on the VM. This study
[...] Read more.
Currently, utilizing virtualization technology in data centers often imposes an increasing burden on the host machine (HM), leading to a decline in VM performance. To address this issue, live virtual migration (LVM) is employed to alleviate the load on the VM. This study introduces a hybrid machine learning model designed to estimate the direct migration of pre-copied migration virtual machines within the data center. The proposed model integrates Markov Decision Process (MDP), genetic algorithm (GA), and random forest (RF) algorithms to forecast the prioritized movement of virtual machines and identify the optimal host machine target. The hybrid models achieve a 99% accuracy rate with quicker training times compared to the previous studies that utilized K-nearest neighbor, decision tree classification, support vector machines, logistic regression, and neural networks. The authors recommend further exploration of a deep learning approach (DL) to address other data center performance issues. This paper outlines promising strategies for enhancing virtual machine migration in data centers. The hybrid models demonstrate high accuracy and faster training times than previous research, indicating the potential for optimizing virtual machine placement and minimizing downtime. The authors emphasize the significance of considering data center performance and propose further investigation. Moreover, it would be beneficial to delve into the practical implementation and dissemination of the proposed model in real-world data centers.
Full article
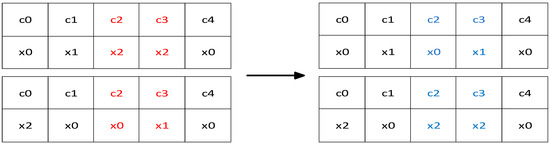
Figure 1
Open AccessArticle
AI Language Models: An Opportunity to Enhance Language Learning
by
Yan Cong
Informatics 2024, 11(3), 49; https://doi.org/10.3390/informatics11030049 - 19 Jul 2024
Abstract
AI language models are increasingly transforming language research in various ways. How can language educators and researchers respond to the challenge posed by these AI models? Specifically, how can we embrace this technology to inform and enhance second language learning and teaching? In
[...] Read more.
AI language models are increasingly transforming language research in various ways. How can language educators and researchers respond to the challenge posed by these AI models? Specifically, how can we embrace this technology to inform and enhance second language learning and teaching? In order to quantitatively characterize and index second language writing, the current work proposes the use of similarities derived from contextualized meaning representations in AI language models. The computational analysis in this work is hypothesis-driven. The current work predicts how similarities should be distributed in a second language learning setting. The results suggest that similarity metrics are informative of writing proficiency assessment and interlanguage development. Statistically significant effects were found across multiple AI models. Most of the metrics could distinguish language learners’ proficiency levels. Significant correlations were also found between similarity metrics and learners’ writing test scores provided by human experts in the domain. However, not all such effects were strong or interpretable. Several results could not be consistently explained under the proposed second language learning hypotheses. Overall, the current investigation indicates that with careful configuration and systematic metrics design, AI language models can be promising tools in advancing language education.
Full article
(This article belongs to the Topic AI Chatbots: Threat or Opportunity?)
►▼
Show Figures
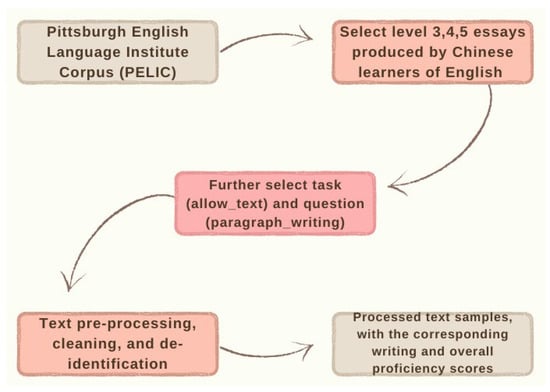
Figure 1
Open AccessReview
Machine Learning Applied to the Analysis of Prolonged COVID Symptoms: An Analytical Review
by
Paola Patricia Ariza-Colpas, Marlon Alberto Piñeres-Melo, Miguel Alberto Urina-Triana, Ernesto Barceló-Martinez, Camilo Barceló-Castellanos and Fabian Roman
Informatics 2024, 11(3), 48; https://doi.org/10.3390/informatics11030048 - 18 Jul 2024
Abstract
The COVID-19 pandemic continues to constitute a public health emergency of international importance, although the state of emergency declaration has indeed been terminated worldwide, many people continue to be infected and present different symptoms associated with the illness. Undoubtedly, solutions based on divergent
[...] Read more.
The COVID-19 pandemic continues to constitute a public health emergency of international importance, although the state of emergency declaration has indeed been terminated worldwide, many people continue to be infected and present different symptoms associated with the illness. Undoubtedly, solutions based on divergent technologies such as machine learning have made great contributions to the understanding, identification, and treatment of the disease. Due to the sudden appearance of this virus, many works have been carried out by the scientific community to support the detection and treatment processes, which has generated numerous publications, making it difficult to identify the status of current research and future contributions that can continue to be generated around this problem that is still valid among us. To address this problem, this article shows the result of a scientometric analysis, which allows the identification of the various contributions that have been generated from the line of automatic learning for the monitoring and treatment of symptoms associated with this pathology. The methodology for the development of this analysis was carried out through the implementation of two phases: in the first phase, a scientometric analysis was carried out, where the countries, authors, and magazines with the greatest production associated with this subject can be identified, later in the second phase, the contributions based on the use of the Tree of Knowledge metaphor are identified. The main concepts identified in this review are related to symptoms, implemented algorithms, and the impact of applications. These results provide relevant information for researchers in the field in the search for new solutions or the application of existing ones for the treatment of still-existing symptoms of COVID-19.
Full article
(This article belongs to the Special Issue Health Informatics: Feature Review Papers)
►▼
Show Figures
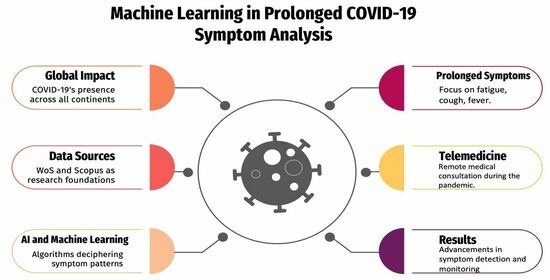
Graphical abstract
Open AccessSystematic Review
Healthcare and the Internet of Medical Things: Applications, Trends, Key Challenges, and Proposed Resolutions
by
Inas Al Khatib, Abdulrahim Shamayleh and Malick Ndiaye
Informatics 2024, 11(3), 47; https://doi.org/10.3390/informatics11030047 - 16 Jul 2024
Abstract
►▼
Show Figures
In recent years, the Internet of medical things (IoMT) has become a significant technological advancement in the healthcare sector. This systematic review aims to identify and summarize the various applications, key challenges, and proposed technical solutions within this domain, based on a comprehensive
[...] Read more.
In recent years, the Internet of medical things (IoMT) has become a significant technological advancement in the healthcare sector. This systematic review aims to identify and summarize the various applications, key challenges, and proposed technical solutions within this domain, based on a comprehensive analysis of the existing literature. This review highlights diverse applications of the IoMT, including mobile health (mHealth) applications, remote biomarker detection, hybrid RFID-IoT solutions for scrub distribution in operating rooms, IoT-based disease prediction using machine learning, and the efficient sharing of personal health records through searchable symmetric encryption, blockchain, and IPFS. Other notable applications include remote healthcare management systems, non-invasive real-time blood glucose measurement devices, distributed ledger technology (DLT) platforms, ultra-wideband (UWB) radar systems, IoT-based pulse oximeters, accident and emergency informatics (A&EI), and integrated wearable smart patches. The key challenges identified include privacy protection, sustainable power sources, sensor intelligence, human adaptation to sensors, data speed, device reliability, and storage efficiency. The proposed mitigations encompass network control, cryptography, edge-fog computing, and blockchain, alongside rigorous risk planning. The review also identifies trends and advancements in the IoMT architecture, remote monitoring innovations, the integration of machine learning and AI, and enhanced security measures. This review makes several novel contributions compared to the existing literature, including (1) a comprehensive categorization of IoMT applications, extending beyond the traditional use cases to include emerging technologies such as UWB radar systems and DLT platforms; (2) an in-depth analysis of the integration of machine learning and AI in IoMT, highlighting innovative approaches in disease prediction and remote monitoring; (3) a detailed examination of privacy and security measures, proposing advanced cryptographic solutions and blockchain implementations to enhance data protection; and (4) the identification of future research directions, providing a roadmap for addressing current limitations and advancing the scientific understanding of IoMT in healthcare. By addressing current limitations and suggesting future research directions, this work aims to advance scientific understanding of the IoMT in healthcare.
Full article
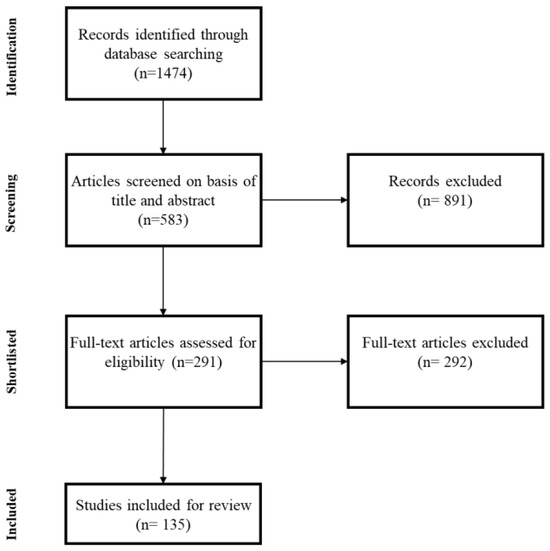
Figure 1
Open AccessArticle
Evaluating and Enhancing Artificial Intelligence Models for Predicting Student Learning Outcomes
by
Helia Farhood, Ibrahim Joudah, Amin Beheshti and Samuel Muller
Informatics 2024, 11(3), 46; https://doi.org/10.3390/informatics11030046 - 15 Jul 2024
Abstract
►▼
Show Figures
Predicting student outcomes is an essential task and a central challenge among artificial intelligence-based personalised learning applications. Despite several studies exploring student performance prediction, there is a notable lack of comprehensive and comparative research that methodically evaluates and compares multiple machine learning models
[...] Read more.
Predicting student outcomes is an essential task and a central challenge among artificial intelligence-based personalised learning applications. Despite several studies exploring student performance prediction, there is a notable lack of comprehensive and comparative research that methodically evaluates and compares multiple machine learning models alongside deep learning architectures. In response, our research provides a comprehensive comparison to evaluate and improve ten different machine learning and deep learning models, either well-established or cutting-edge techniques, namely, random forest, decision tree, support vector machine, K-nearest neighbours classifier, logistic regression, linear regression, and state-of-the-art extreme gradient boosting (XGBoost), as well as a fully connected feed-forward neural network, a convolutional neural network, and a gradient-boosted neural network. We implemented and fine-tuned these models using Python 3.9.5. With a keen emphasis on prediction accuracy and model performance optimisation, we evaluate these methodologies across two benchmark public student datasets. We employ a dual evaluation approach, utilising both k-fold cross-validation and holdout methods, to comprehensively assess the models’ performance. Our research focuses primarily on predicting student outcomes in final examinations by determining their success or failure. Moreover, we explore the importance of feature selection using the ubiquitous Lasso for dimensionality reduction to improve model efficiency, prevent overfitting, and examine its impact on prediction accuracy for each model, both with and without Lasso. This study provides valuable guidance for selecting and deploying predictive models for tabular data classification like student outcome prediction, which seeks to utilise data-driven insights for personalised education.
Full article
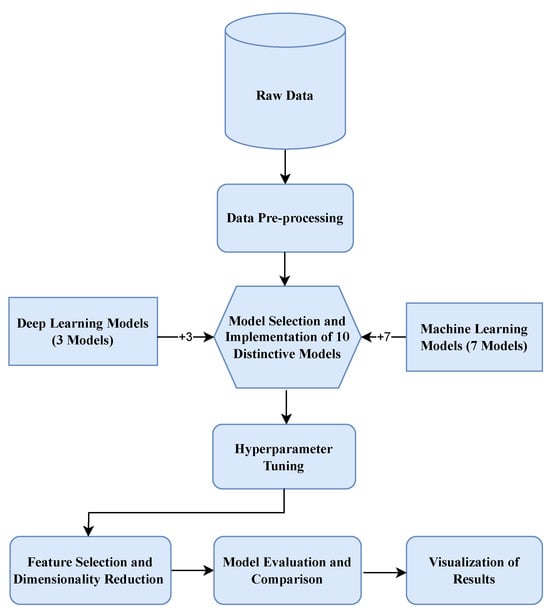
Figure 1
Open AccessArticle
GPTs or Grim Position Threats? The Potential Impacts of Large Language Models on Non-Managerial Jobs and Certifications in Cybersecurity
by
Raza Nowrozy
Informatics 2024, 11(3), 45; https://doi.org/10.3390/informatics11030045 - 11 Jul 2024
Abstract
ChatGPT, a Large Language Model (LLM) utilizing Natural Language Processing (NLP), has caused concerns about its impact on job sectors, including cybersecurity. This study assesses ChatGPT’s impacts in non-managerial cybersecurity roles using the NICE Framework and Technological Displacement theory. It also explores its
[...] Read more.
ChatGPT, a Large Language Model (LLM) utilizing Natural Language Processing (NLP), has caused concerns about its impact on job sectors, including cybersecurity. This study assesses ChatGPT’s impacts in non-managerial cybersecurity roles using the NICE Framework and Technological Displacement theory. It also explores its potential to pass top cybersecurity certification exams. Findings reveal ChatGPT’s promise to streamline some jobs, especially those requiring memorization. Moreover, this paper highlights ChatGPT’s challenges and limitations, such as ethical implications, LLM limitations, and Artificial Intelligence (AI) security. The study suggests that LLMs like ChatGPT could transform the cybersecurity landscape, causing job losses, skill obsolescence, labor market shifts, and mixed socioeconomic impacts. A shift in focus from memorization to critical thinking, and collaboration between LLM developers and cybersecurity professionals, is recommended.
Full article
(This article belongs to the Topic AI Chatbots: Threat or Opportunity?)
►▼
Show Figures
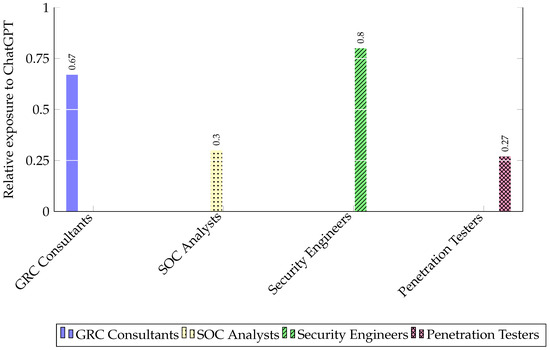
Figure 1
Open AccessArticle
A Framework for Antecedents to Health Information Systems Uptake by Healthcare Professionals: An Exploratory Study of Electronic Medical Records
by
Reza Torkman, Amir Hossein Ghapanchi and Reza Ghanbarzadeh
Informatics 2024, 11(3), 44; https://doi.org/10.3390/informatics11030044 - 9 Jul 2024
Abstract
Health information systems (HISs) are essential information systems used by organisations and individuals for various purposes. Past research has studied different types of HIS, such as rostering systems, Electronic Medical Records (EMRs), and Personal Health Records (PHRs). Although several past confirmatory studies have
[...] Read more.
Health information systems (HISs) are essential information systems used by organisations and individuals for various purposes. Past research has studied different types of HIS, such as rostering systems, Electronic Medical Records (EMRs), and Personal Health Records (PHRs). Although several past confirmatory studies have quantitatively examined EMR uptake by health professionals, there is a lack of exploratory and qualitative studies that uncover various drivers of healthcare professionals’ uptake of EMRs. Applying an exploratory and qualitative approach, this study introduces various antecedents of healthcare professionals’ uptake of EMRs. This study conducted 78 semi-structured, open-ended interviews with 15 groups of healthcare professional users of EMRs in two large Australian hospitals. Data analysis of qualitative data resulted in proposing a framework comprising 23 factors impacting healthcare professionals’ uptake of EMRs, which are categorised into ten main categories: perceived benefits of EMR, perceived difficulties, hardware/software compatibility, job performance uncertainty, ease of operation, perceived risk, assistance society, user confidence, organisational support, and technological support. Our findings have important implications for various practitioner groups, such as healthcare policymakers, hospital executives, hospital middle and line managers, hospitals’ IT departments, and healthcare professionals using EMRs. Implications of the findings for researchers and practitioners are provided herein in detail.
Full article
(This article belongs to the Topic Theories and Applications of Human-Computer Interaction)
►▼
Show Figures
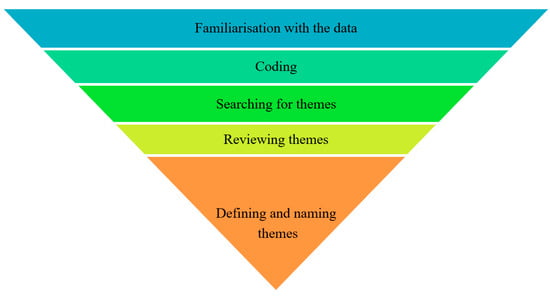
Figure 1
Open AccessArticle
Impact of Hospital Employees’ Awareness of the EMR System Certification on Interoperability Evaluation: Comparison of Public and Private Hospitals
by
Choyeal Park and Jikyeong Park
Informatics 2024, 11(3), 43; https://doi.org/10.3390/informatics11030043 - 3 Jul 2024
Abstract
This study examined the awareness of the EMR certification system among employees of public and private hospitals that have obtained EMR certification. It also assessed how this awareness impacted the evaluation of EMR interoperability. The objective of this study is to contribute to
[...] Read more.
This study examined the awareness of the EMR certification system among employees of public and private hospitals that have obtained EMR certification. It also assessed how this awareness impacted the evaluation of EMR interoperability. The objective of this study is to contribute to the stable adoption and further development of EMR system certification in Korea. Data were collected through 3600 questionnaires distributed over three years from 2021 to 2023. After excluding 24 questionnaires owing to missing values or insincere responses, 3576 responses were analyzed. The analysis involved descriptive statistics, cross-tabulation, t-tests, ANOVA, and multiple regression using SPSS 26.0. The significance level (α) for statistical tests was set at 0.05. This study revealed differences in awareness of EMR system certification and interoperability among hospital employees. In both public and private hospitals, awareness of the EMR system certification positively influences the evaluation of interoperability.
Full article
(This article belongs to the Section Health Informatics)
Open AccessArticle
The Mappability of Clinical Real-World Data of Patients with Melanoma to Oncological Fast Healthcare Interoperability Resources (FHIR) Profiles: A Single-Center Interoperability Study
by
Jessica Swoboda, Moritz Albert, Catharina Lena Beckmann, Georg Christian Lodde, Elisabeth Livingstone, Felix Nensa, Dirk Schadendorf and Britta Böckmann
Informatics 2024, 11(3), 42; https://doi.org/10.3390/informatics11030042 - 28 Jun 2024
Abstract
(1) Background: Tumor-specific standardized data are essential for AI-based progress in research, e.g., for predicting adverse events in patients with melanoma. Although there are oncological Fast Healthcare Interoperability Resources (FHIR) profiles, it is unclear how well these can represent malignant melanoma. (2) Methods:
[...] Read more.
(1) Background: Tumor-specific standardized data are essential for AI-based progress in research, e.g., for predicting adverse events in patients with melanoma. Although there are oncological Fast Healthcare Interoperability Resources (FHIR) profiles, it is unclear how well these can represent malignant melanoma. (2) Methods: We created a methodology pipeline to assess to what extent an oncological FHIR profile, in combination with a standard FHIR specification, can represent a real-world data set. We extracted Electronic Health Record (EHR) data from a data platform, and identified and validated relevant features. We created a melanoma data model and mapped its features to the oncological HL7 FHIR Basisprofil Onkologie [Basic Profile Oncology] and the standard FHIR specification R4. (3) Results: We identified 216 features. Mapping showed that 45 out of 216 (20.83%) features could be mapped completely or with adjustments using the Basisprofil Onkologie [Basic Profile Oncology], and 129 (60.85%) features could be mapped using the standard FHIR specification. A total of 39 (18.06%) new, non-mappable features could be identified. (4) Conclusions: Our tumor-specific real-world melanoma data could be partially mapped using a combination of an oncological FHIR profile and a standard FHIR specification. However, important data features were lost or had to be mapped with self-defined extensions, resulting in limited interoperability.
Full article
(This article belongs to the Section Health Informatics)
►▼
Show Figures
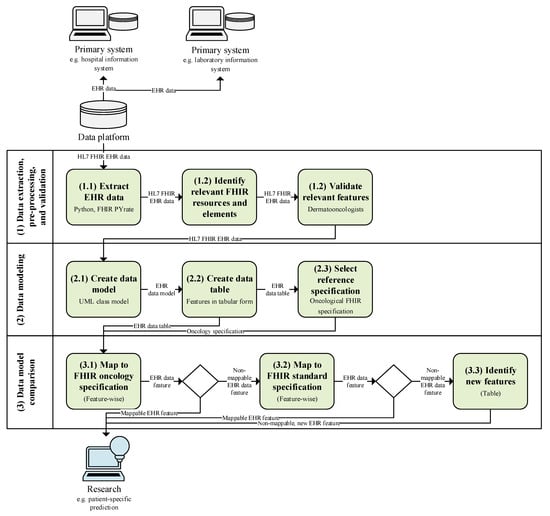
Figure 1
Open AccessReview
Identifying Long COVID Definitions, Predictors, and Risk Factors in the United States: A Scoping Review of Data Sources Utilizing Electronic Health Records
by
Rayanne A. Luke, George Shaw, Jr., Geetha Saarunya and Abolfazl Mollalo
Informatics 2024, 11(2), 41; https://doi.org/10.3390/informatics11020041 - 14 Jun 2024
Abstract
►▼
Show Figures
This scoping review explores the potential of electronic health records (EHR)-based studies to characterize long COVID. We screened all peer-reviewed publications in the English language from PubMed/MEDLINE, Scopus, and Web of Science databases until 14 September 2023, to identify the studies that defined
[...] Read more.
This scoping review explores the potential of electronic health records (EHR)-based studies to characterize long COVID. We screened all peer-reviewed publications in the English language from PubMed/MEDLINE, Scopus, and Web of Science databases until 14 September 2023, to identify the studies that defined or characterized long COVID based on data sources that utilized EHR in the United States, regardless of study design. We identified only 17 articles meeting the inclusion criteria. Respiratory conditions were consistently significant in all studies, followed by poor well-being features (n = 14, 82%) and cardiovascular conditions (n = 12, 71%). Some articles (n = 7, 41%) used a long COVID-specific marker to define the study population, relying mainly on ICD-10 codes and clinical visits for post-COVID-19 conditions. Among studies exploring plausible long COVID (n = 10, 59%), the most common methods were RT-PCR and antigen tests. The time delay for EHR data extraction post-test varied, ranging from four weeks to more than three months; however, most studies considering plausible long COVID used a waiting period of 28 to 31 days. Our findings suggest a limited utilization of EHR-derived data sources in defining long COVID, with only 59% of these studies incorporating a validation step.
Full article
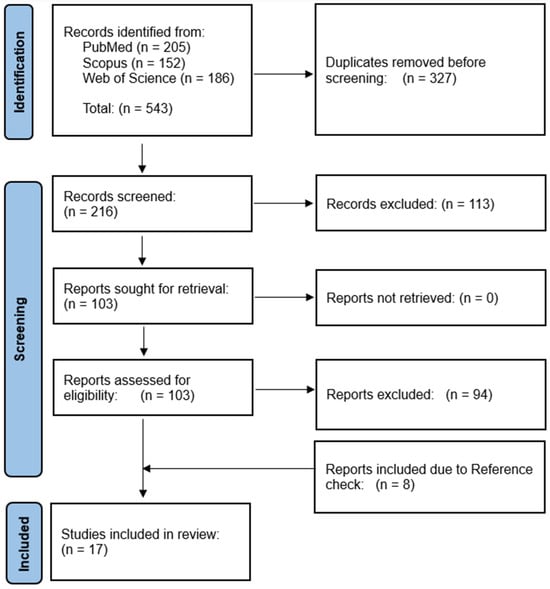
Figure 1
Open AccessArticle
Analysis of the Epidemic Curve of the Waves of COVID-19 Using Integration of Functions and Neural Networks in Peru
by
Oliver Amadeo Vilca Huayta, Adolfo Carlos Jimenez Chura, Carlos Boris Sosa Maydana and Alioska Jessica Martínez García
Informatics 2024, 11(2), 40; https://doi.org/10.3390/informatics11020040 - 7 Jun 2024
Abstract
The coronavirus (COVID-19) pandemic continues to claim victims. According to the World Health Organization, in the 28 days leading up to 25 February 2024 alone, the number of deaths from COVID-19 was 7141. In this work, we aimed to model the waves of
[...] Read more.
The coronavirus (COVID-19) pandemic continues to claim victims. According to the World Health Organization, in the 28 days leading up to 25 February 2024 alone, the number of deaths from COVID-19 was 7141. In this work, we aimed to model the waves of COVID-19 through artificial neural networks (ANNs) and the sigmoidal–Boltzmann model. The study variable was the global cumulative number of deaths according to days, based on the Peru dataset. Additionally, the variables were adapted to determine the correlation between social isolation measures and death rates, which constitutes a novel contribution. A quantitative methodology was used that implemented a non-experimental, longitudinal, and correlational design. The study was retrospective. The results show that the sigmoidal and ANN models were reasonably representative and could help to predict the spread of COVID-19 over the course of multiple waves. Furthermore, the results were precise, with a Pearson correlation coefficient greater than 0.999. The computational sigmoidal–Boltzmann model was also time-efficient. Moreover, the Spearman correlation between social isolation measures and death rates was 0.77, which is acceptable considering that the social isolation variable is qualitative. Finally, we concluded that social isolation measures had a significant effect on reducing deaths from COVID-19.
Full article
(This article belongs to the Section Health Informatics)
►▼
Show Figures
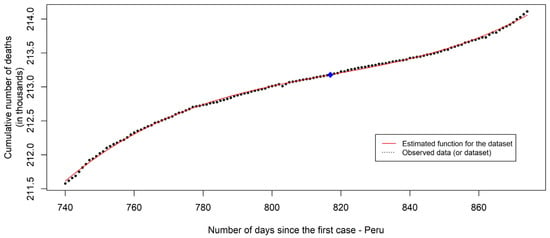
Figure 1
Open AccessArticle
MSProfileR: An Open-Source Software for Quality Control of Matrix-Assisted Laser Desorption Ionization–Time of Flight Spectra
by
Refka Ben Hamouda, Bertrand Estellon, Khalil Himet, Aimen Cherif, Hugo Marthinet, Jean-Marie Loreau, Gaëtan Texier, Samuel Granjeaud and Lionel Almeras
Informatics 2024, 11(2), 39; https://doi.org/10.3390/informatics11020039 - 6 Jun 2024
Abstract
In the early 2000s, matrix-assisted laser desorption ionization–time of flight mass spectrometry (MALDI-TOF MS) emerged as a performant and relevant tool for identifying micro-organisms. Since then, it has become practically essential for identifying bacteria in microbiological diagnostic laboratories. In the last decade, it
[...] Read more.
In the early 2000s, matrix-assisted laser desorption ionization–time of flight mass spectrometry (MALDI-TOF MS) emerged as a performant and relevant tool for identifying micro-organisms. Since then, it has become practically essential for identifying bacteria in microbiological diagnostic laboratories. In the last decade, it was successfully applied for arthropod identification, allowing researchers to distinguish vectors from non-vectors of infectious diseases. However, identification failures are not rare, hampering its wide use. Failure is generally attributed either to the absence of respective counter species MS spectra in the database or to the insufficient quality of query MS spectra (i.e., lower intensity and diversity of MS peaks detected). To avoid matching errors due to non-compliant spectra, the development of a strategy for detecting and excluding outlier MS profiles became compulsory. To this end, we created MSProfileR, an R package leading to a bioinformatics tool through a simple installation, integrating a control quality system of MS spectra and an analysis pipeline including peak detection and MS spectra comparisons. MSProfileR can also add metadata concerning the sample that the spectra are derived from. MSProfileR has been developed in the R environment and offers a user-friendly web interface using the R Shiny framework. It is available on Microsoft Windows as a web browser application by simple navigation using the link of the package on Github v.3.10.0. MSProfileR is therefore accessible to non-computer specialists and is freely available to the scientific community. We evaluated MSProfileR using two datasets including exclusively MS spectra from arthropods. In addition to coherent sample classification, outlier MS spectra were detected in each dataset confirming the value of MSProfileR.
Full article
(This article belongs to the Topic New Developments and Applications in Bioinformatics and Computational Biology)
►▼
Show Figures
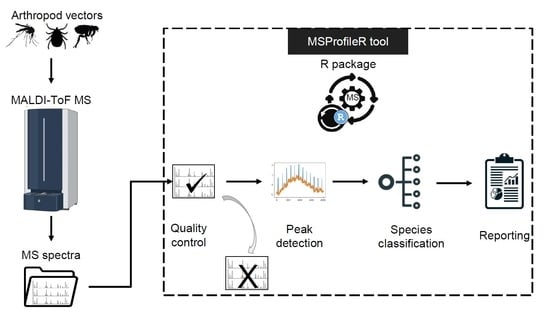
Graphical abstract
Open AccessArticle
Chatbot Technology Use and Acceptance Using Educational Personas
by
Fatima Ali Amer jid Almahri, David Bell and Zameer Gulzar
Informatics 2024, 11(2), 38; https://doi.org/10.3390/informatics11020038 - 3 Jun 2024
Abstract
Chatbots are computer programs that mimic human conversation using text or voice or both. Users’ acceptance of chatbots is highly influenced by their persona. Users develop a sense of familiarity with chatbots as they use them, so they become more approachable, and this
[...] Read more.
Chatbots are computer programs that mimic human conversation using text or voice or both. Users’ acceptance of chatbots is highly influenced by their persona. Users develop a sense of familiarity with chatbots as they use them, so they become more approachable, and this encourages them to interact with the chatbots more readily by fostering favorable opinions of the technology. In this study, we examine the moderating effects of persona traits on students’ acceptance and use of chatbot technology at higher educational institutions in the UK. We use an Extended Unified Theory of Acceptance and Use of Technology (Extended UTAUT2). Through a self-administrated survey using a questionnaire, data were collected from 431 undergraduate and postgraduate computer science students. This study employed a Likert scale to measure the variables associated with chatbot acceptance. To evaluate the gathered data, Structural Equation Modelling (SEM) coupled with multi-group analysis (MGA) using SmartPLS3 were used. The estimated Cronbach’s alpha highlighted the accuracy and legitimacy of the findings. The results showed that the emerging factors that influence students’ adoption and use of chatbot technology were habit, effort expectancy, and performance expectancy. Additionally, it was discovered that the Extended UTAUT2 model did not require grades or educational level to moderate the correlations. These results are important for improving user experience and they have implications for academics, researchers, and organizations, especially in the context of native chatbots.
Full article
(This article belongs to the Section Human-Computer Interaction)
►▼
Show Figures
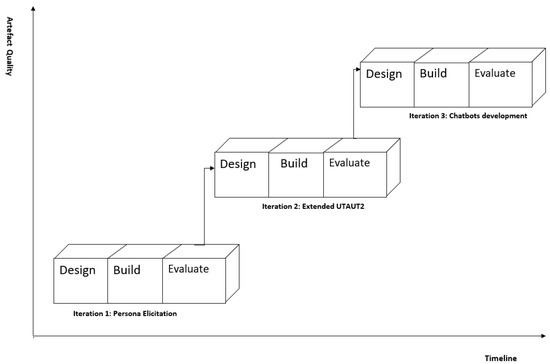
Figure 1
Open AccessArticle
Analysing the Impact of Generative AI in Arts Education: A Cross-Disciplinary Perspective of Educators and Students in Higher Education
by
Sara Sáez-Velasco, Mario Alaguero-Rodríguez, Vanesa Delgado-Benito and Sonia Rodríguez-Cano
Informatics 2024, 11(2), 37; https://doi.org/10.3390/informatics11020037 - 3 Jun 2024
Abstract
Generative AI refers specifically to a class of Artificial Intelligence models that use existing data to create new content that reflects the underlying patterns of real-world data. This contribution presents a study that aims to show what the current perception of arts educators
[...] Read more.
Generative AI refers specifically to a class of Artificial Intelligence models that use existing data to create new content that reflects the underlying patterns of real-world data. This contribution presents a study that aims to show what the current perception of arts educators and students of arts education is with regard to generative Artificial Intelligence. It is a qualitative research study using focus groups as a data collection technique in order to obtain an overview of the participating subjects. The research design consists of two phases: (1) generation of illustrations from prompts by students, professionals and a generative AI tool; and (2) focus groups with students (N = 5) and educators (N = 5) of artistic education. In general, the perception of educators and students coincides in the usefulness of generative AI as a tool to support the generation of illustrations. However, they agree that the human factor cannot be replaced by generative AI. The results obtained allow us to conclude that generative AI can be used as a motivating educational strategy for arts education.
Full article
(This article belongs to the Topic AI Chatbots: Threat or Opportunity?)
►▼
Show Figures
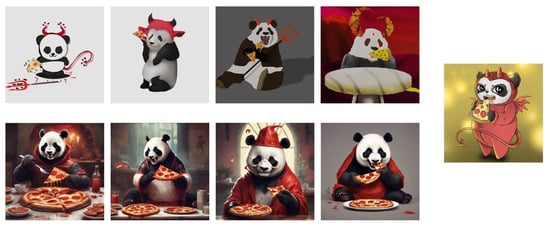
Figure 1
Open AccessCommunication
Safety of Human–Artificial Intelligence Systems: Applying Safety Science to Analyze Loopholes in Interactions between Human Organizations, Artificial Intelligence, and Individual People
by
Stephen Fox and Juan G. Victores
Informatics 2024, 11(2), 36; https://doi.org/10.3390/informatics11020036 - 29 May 2024
Abstract
Loopholes involve misalignments between rules about what should be done and what is actually done in practice. The focus of this paper is loopholes in interactions between human organizations’ implementations of task-specific artificial intelligence and individual people. The importance of identifying and addressing
[...] Read more.
Loopholes involve misalignments between rules about what should be done and what is actually done in practice. The focus of this paper is loopholes in interactions between human organizations’ implementations of task-specific artificial intelligence and individual people. The importance of identifying and addressing loopholes is recognized in safety science and in applications of AI. Here, an examination is provided of loophole sources in interactions between human organizations and individual people. Then, it is explained how the introduction of task-specific AI applications can introduce new sources of loopholes. Next, an analytical framework, which is well-established in safety science, is applied to analyses of loopholes in interactions between human organizations, artificial intelligence, and individual people. The example used in the analysis is human–artificial intelligence systems in gig economy delivery driving work.
Full article
(This article belongs to the Section Human-Computer Interaction)
►▼
Show Figures
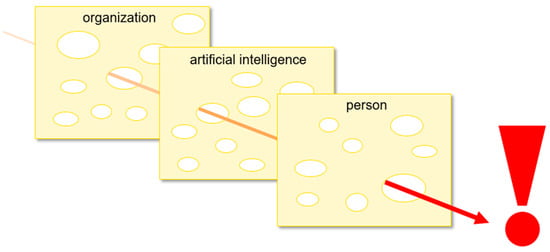
Figure 1
Open AccessArticle
Improving Minority Class Recall through a Novel Cluster-Based Oversampling Technique
by
Takorn Prexawanprasut and Thepparit Banditwattanawong
Informatics 2024, 11(2), 35; https://doi.org/10.3390/informatics11020035 - 28 May 2024
Abstract
In this study, we propose an approach to address the pressing issue of false negative errors by enhancing minority class recall within imbalanced data sets commonly encountered in machine learning applications. Through the utilization of a cluster-based oversampling technique in conjunction with an
[...] Read more.
In this study, we propose an approach to address the pressing issue of false negative errors by enhancing minority class recall within imbalanced data sets commonly encountered in machine learning applications. Through the utilization of a cluster-based oversampling technique in conjunction with an information entropy evaluation, our approach effectively targets areas of ambiguity inherent in the data set. An extensive evaluation across a diverse range of real-world data sets characterized by inter-cluster complexity demonstrates the superior performance of our method compared to that of existing oversampling techniques. Particularly noteworthy is its significant improvement within the Delinquency Telecom data set, where it achieves a remarkable increase of up to 30.54 percent in minority class recall compared to the original data set. This notable reduction in false negative errors underscores the importance of our methodology in accurately identifying and classifying instances from underrepresented classes, thereby enhancing model performance in imbalanced data scenarios.
Full article
(This article belongs to the Section Machine Learning)
►▼
Show Figures
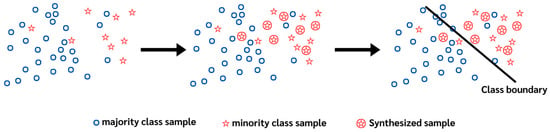
Figure 1
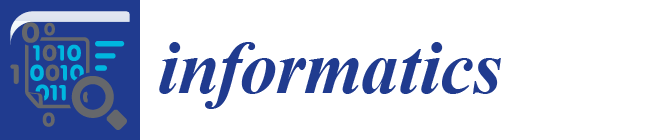
Journal Menu
► ▼ Journal Menu-
- Informatics Home
- Aims & Scope
- Editorial Board
- Reviewer Board
- Topical Advisory Panel
- Instructions for Authors
- Special Issues
- Topics
- Sections & Collections
- Article Processing Charge
- Indexing & Archiving
- Editor’s Choice Articles
- Most Cited & Viewed
- Journal Statistics
- Journal History
- Journal Awards
- Editorial Office
Journal Browser
► ▼ Journal BrowserHighly Accessed Articles
Latest Books
E-Mail Alert
News
Topics
Topic in
Applied Sciences, Electronics, Informatics, Information, Software
Software Engineering and Applications
Topic Editors: Sanjay Misra, Robertas Damaševičius, Bharti SuriDeadline: 31 October 2024
Topic in
Computers, Informatics, Information, Logistics, Mathematics, Algorithms
Decision Science Applications and Models (DSAM)
Topic Editors: Daniel Riera Terrén, Angel A. Juan, Majsa Ammuriova, Laura CalvetDeadline: 31 December 2024
Topic in
Brain Sciences, Healthcare, Informatics, IJERPH, JCM, Reports
Applications of Virtual Reality Technology in Rehabilitation
Topic Editors: Jorge Oliveira, Pedro GamitoDeadline: 30 June 2025

Conferences
Special Issues
Special Issue in
Informatics
Health Informatics: Feature Review Papers
Guest Editors: Jiang Bian, Yi GuoDeadline: 31 July 2024
Special Issue in
Informatics
New Advances in Semantic Recognition and Analysis
Guest Editors: Daniele Toti, Andrea Pozzi, Enrico BarbieratoDeadline: 31 August 2024
Special Issue in
Informatics
Digital Society: Interdisciplinary Insights and Applications of Wireless Connectivity
Guest Editors: Carolina Del Valle Soto, Ramiro VelázquezDeadline: 30 September 2024
Special Issue in
Informatics
The Smart Cities Continuum via Machine Learning and Artificial Intelligence
Guest Editors: Augusto Neto, Roger ImmichDeadline: 31 December 2024
Topical Collections
Topical Collection in
Informatics
Promotion of Computational Thinking and Informatics Education in Pre-University Studies
Collection Editor: Francisco José García-Peñalvo
Topical Collection in
Informatics
Uncertainty in Digital Humanities
Collection Editors: Roberto Theron, Eveline Wandl-Vogt, Jennifer Cizik Edmond, Cezary Mazurek