Journal Description
Data
- Open Access— free for readers, with article processing charges (APC) paid by authors or their institutions.
- High Visibility: indexed within Scopus, ESCI (Web of Science), Ei Compendex, dblp, Inspec, RePEc, and other databases.
- Journal Rank: JCR - Q2 (Multidisciplinary Sciences) / CiteScore - Q2 (Information Systems and Management)
- Rapid Publication: manuscripts are peer-reviewed and a first decision is provided to authors approximately 26.8 days after submission; acceptance to publication is undertaken in 3.6 days (median values for papers published in this journal in the second half of 2024).
- Recognition of Reviewers: reviewers who provide timely, thorough peer-review reports receive vouchers entitling them to a discount on the APC of their next publication in any MDPI journal, in appreciation of the work done.
Latest Articles
Highly Accessed Articles
Latest Books
E-Mail Alert
News
MDPI INSIGHTS: The CEO's Letter #21 - Annual Report, Swiss Consortium, IWD, ICARS, Serbia
Meet Us at the 52nd CAA International Conference (Digital Horizons: Embracing Heritage in an Evolving World), 5–9 May 2025, Athens, Greece
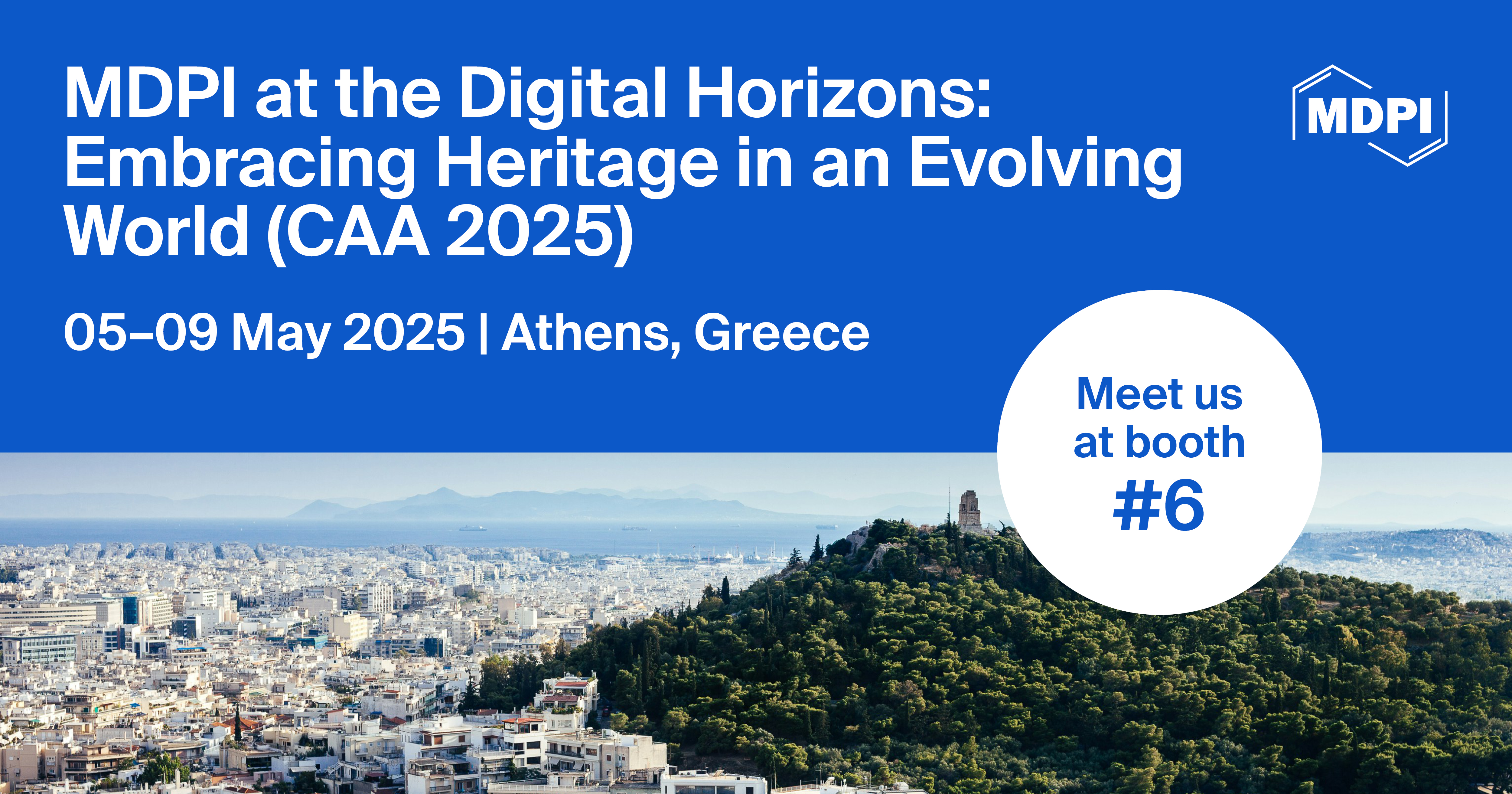
Topics
Deadline: 31 July 2025
Deadline: 30 September 2025
Deadline: 30 November 2025
Deadline: 30 December 2025
Conferences
Special Issues
Deadline: 30 April 2025
Deadline: 30 June 2025
Deadline: 31 July 2025
Deadline: 20 August 2025