Topic Menu
► Topic MenuTopic Editors

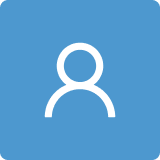
Computer Vision and Image Processing, 2nd Edition
Topic Information
Dear Colleagues,
The field of computer vision and image processing has advanced significantly in recent years, with new techniques and applications emerging constantly. Building on the success of our first edition, we are pleased to announce a second edition on this exciting topic. We invite researchers, academics, and practitioners to submit original research articles, reviews, or case studies that address the latest developments in computer vision and image processing. Topics of interest include but are not limited to:
- Deep learning for image classification and recognition
- Object detection and tracking
- Image segmentation and analysis
- 3D reconstruction and modeling
- Image and video compression
- Image enhancement and restoration
- Medical image processing and analysis
- Augmented and virtual reality
Submissions should be original and should not have been published or submitted elsewhere. All papers will be peer-reviewed by at least two experts in the field, and accepted papers will be published together on the topic website. To submit your paper, please visit the journal's website and follow the submission guidelines. For any queries, please contact the guest editors of the topic.
We look forward to receiving your submissions and sharing the latest advancements in computer vision and image processing with our readers.
Prof. Silvia Liberata Ullo
Prof. Dr. Li Zhang
Topic Editors
Keywords
- 3D acquisition, processing, and visualization
- scene understanding
- multimodal sensor processing and fusion
- multispectral, color, and greyscale image processing
- industrial quality inspection
- computer vision for robotics
- computer vision for surveillance
- airborne and satellite on-board image acquisition platforms
- computational models of vision
- imaging psychophysics
Participating Journals
Journal Name | Impact Factor | CiteScore | Launched Year | First Decision (median) | APC |
---|---|---|---|---|---|
![]()
Applied Sciences
|
2.5 | 5.3 | 2011 | 18.4 Days | CHF 2400 |
![]()
Electronics
|
2.6 | 5.3 | 2012 | 16.4 Days | CHF 2400 |
![]()
Journal of Imaging
|
2.7 | 5.9 | 2015 | 18.3 Days | CHF 1800 |
![]()
Mathematics
|
2.3 | 4.0 | 2013 | 18.3 Days | CHF 2600 |
![]()
Remote Sensing
|
4.2 | 8.3 | 2009 | 23.9 Days | CHF 2700 |
Preprints.org is a multidisciplinary platform offering a preprint service designed to facilitate the early sharing of your research. It supports and empowers your research journey from the very beginning.
MDPI Topics is collaborating with Preprints.org and has established a direct connection between MDPI journals and the platform. Authors are encouraged to take advantage of this opportunity by posting their preprints at Preprints.org prior to publication:
- Share your research immediately: disseminate your ideas prior to publication and establish priority for your work.
- Safeguard your intellectual contribution: Protect your ideas with a time-stamped preprint that serves as proof of your research timeline.
- Boost visibility and impact: Increase the reach and influence of your research by making it accessible to a global audience.
- Gain early feedback: Receive valuable input and insights from peers before submitting to a journal.
- Ensure broad indexing: Web of Science (Preprint Citation Index), Google Scholar, Crossref, SHARE, PrePubMed, Scilit and Europe PMC.