-
A Novel Method to Compute the Contact Surface Area Between an Organ and Cancer Tissue
-
Sowing, Monitoring, Detecting: A Possible Solution to Improve the Visibility of Cropmarks in Cultivated Fields
-
A Method for Estimating Fluorescence Emission Spectra from the Image Data of Plant Grain and Leaves Without a Spectrometer
-
SAVE: Self-Attention on Visual Embedding for Zero-Shot Generic Object Counting
Journal Description
Journal of Imaging
Journal of Imaging
is an international, multi/interdisciplinary, peer-reviewed, open access journal of imaging techniques published online monthly by MDPI.
- Open Access— free for readers, with article processing charges (APC) paid by authors or their institutions.
- High Visibility: indexed within Scopus, ESCI (Web of Science), PubMed, PMC, dblp, Inspec, Ei Compendex, and other databases.
- Journal Rank: CiteScore - Q1 (Computer Graphics and Computer-Aided Design)
- Rapid Publication: manuscripts are peer-reviewed and a first decision is provided to authors approximately 18.3 days after submission; acceptance to publication is undertaken in 3.3 days (median values for papers published in this journal in the second half of 2024).
- Recognition of Reviewers: reviewers who provide timely, thorough peer-review reports receive vouchers entitling them to a discount on the APC of their next publication in any MDPI journal, in appreciation of the work done.
Impact Factor:
2.7 (2023);
5-Year Impact Factor:
3.0 (2023)
Latest Articles
SwinTCS: A Swin Transformer Approach to Compressive Sensing with Non-Local Denoising
J. Imaging 2025, 11(5), 139; https://doi.org/10.3390/jimaging11050139 - 29 Apr 2025
Abstract
In the era of the Internet of Things (IoT), the rapid growth of interconnected devices has intensified the demand for efficient data acquisition and processing techniques. Compressive Sensing (CS) has emerged as a promising approach for simultaneous signal acquisition and dimensionality reduction, particularly
[...] Read more.
In the era of the Internet of Things (IoT), the rapid growth of interconnected devices has intensified the demand for efficient data acquisition and processing techniques. Compressive Sensing (CS) has emerged as a promising approach for simultaneous signal acquisition and dimensionality reduction, particularly in multimedia applications. In response to the challenges presented by traditional CS reconstruction methods, such as boundary artifacts and limited robustness, we propose a novel hierarchical deep learning framework, SwinTCS, for CS-aware image reconstruction. Leveraging the Swin Transformer architecture, SwinTCS integrates a hierarchical feature representation strategy to enhance global contextual modeling while maintaining computational efficiency. Moreover, to better capture local features of images, we introduce an auxiliary convolutional neural network (CNN). Additionally, for suppressing noise and improving reconstruction quality in high-compression scenarios, we incorporate a Non-Local Means Denoising module. The experimental results on multiple public benchmark datasets indicate that SwinTCS surpasses State-of-the-Art (SOTA) methods across various evaluation metrics, thereby confirming its superior performance.
Full article
(This article belongs to the Topic Intelligent Image Processing Technology)
Open AccessArticle
Design of a Novel Conditional Noise Predictor for Image Super-Resolution Reconstruction Based on DDPM
by
Jiyan Zhang, Hua Sun, Haiyang Fan, Yujie Xiong and Jiaqi Zhang
J. Imaging 2025, 11(5), 138; https://doi.org/10.3390/jimaging11050138 - 29 Apr 2025
Abstract
Image super-resolution (SR) reconstruction is a critical task aimed at enhancing low-quality images to obtain high-quality counterparts. Existing denoising diffusion models have demonstrated commendable performance in handling image SR reconstruction tasks; however, they often require thousands—or even more—diffusion sampling steps, significantly prolonging the
[...] Read more.
Image super-resolution (SR) reconstruction is a critical task aimed at enhancing low-quality images to obtain high-quality counterparts. Existing denoising diffusion models have demonstrated commendable performance in handling image SR reconstruction tasks; however, they often require thousands—or even more—diffusion sampling steps, significantly prolonging the training duration for the denoising diffusion model. Conversely, reducing the number of diffusion steps may lead to the loss of intricate texture features in the generated images, resulting in overly smooth outputs despite improving the training efficiency. To address these challenges, we introduce a novel diffusion model named RapidDiff. RapidDiff uses a state-of-the-art conditional noise predictor (CNP) to predict the noise distribution at a level that closely resembles the real noise properties, thereby reducing the problem of high-variance noise produced by U-Net decoders during the noise prediction stage. Additionally, RapidDiff enhances the efficiency of image SR reconstruction by focusing on the residuals between high-resolution (HR) and low-resolution (LR) images. Experimental analyses confirm that our proposed RapidDiff model achieves performance that is either superior or comparable to that of the most advanced models that are currently available, as demonstrated on both the ImageNet dataset and the Alsat-2b dataset.
Full article
(This article belongs to the Section Image and Video Processing)
►▼
Show Figures
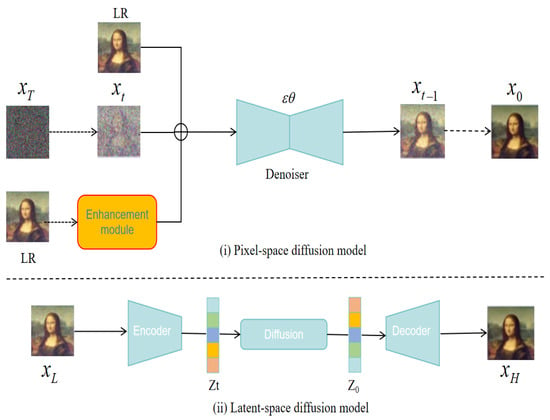
Figure 1
Open AccessArticle
Deep-Learning Approaches for Cervical Cytology Nuclei Segmentation in Whole Slide Images
by
Andrés Mosquera-Zamudio, Sandra Cancino, Guillermo Cárdenas-Montoya, Juan D. Garcia-Arteaga, Carlos Zambrano-Betancourt and Rafael Parra-Medina
J. Imaging 2025, 11(5), 137; https://doi.org/10.3390/jimaging11050137 - 29 Apr 2025
Abstract
Whole-slide imaging (WSI) in cytopathology poses challenges related to segmentation accuracy, computational efficiency, and image acquisition artifacts. This study aims to evaluate the performance of deep-learning models for instance segmentation in cervical cytology, benchmarking them against state-of-the-art methods on both public and institutional
[...] Read more.
Whole-slide imaging (WSI) in cytopathology poses challenges related to segmentation accuracy, computational efficiency, and image acquisition artifacts. This study aims to evaluate the performance of deep-learning models for instance segmentation in cervical cytology, benchmarking them against state-of-the-art methods on both public and institutional datasets. We tested three architectures—U-Net, vision transformer (ViT), and Detectron2—and evaluated their performance on the ISBI 2014 and CNseg datasets using panoptic quality (PQ), dice similarity coefficient (DSC), and intersection over union (IoU). All models were trained on CNseg and tested on an independent institutional dataset. Data preprocessing involved manual annotation using QuPath, patch extraction guided by GeoJSON files, and exclusion of regions containing less than 60% cytologic material. Our models achieved superior segmentation performance on public datasets, reaching up to 98% PQ. Performance decreased on the institutional dataset, likely due to differences in image acquisition and the presence of blurred nuclei. Nevertheless, the models were able to detect blurred nuclei, highlighting their robustness in suboptimal imaging conditions. In conclusion, the proposed models offer an accurate and efficient solution for instance segmentation in cytology WSI. These results support the development of reliable AI-powered tools for digital cytology, with potential applications in automated screening and diagnostic workflows.
Full article
(This article belongs to the Special Issue Deep Learning in Biomedical Image Segmentation and Classification: Advancements, Challenges and Applications)
►▼
Show Figures
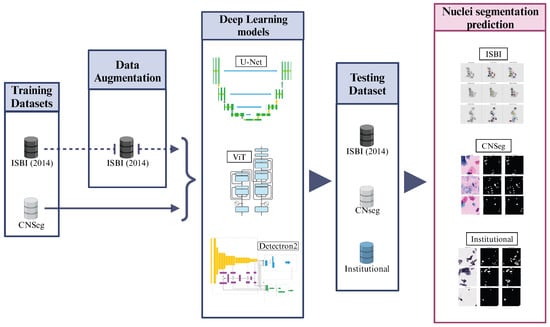
Figure 1
Open AccessArticle
Minimizing Bleed-Through Effect in Medieval Manuscripts with Machine Learning and Robust Statistics
by
Adriano Ettari, Massimo Brescia, Stefania Conte, Yahya Momtaz and Guido Russo
J. Imaging 2025, 11(5), 136; https://doi.org/10.3390/jimaging11050136 - 28 Apr 2025
Abstract
Over the last decades, plenty of ancient manuscripts have been digitized all over the world, and particularly in Europe. The fruition of these huge digital archives is often limited by the bleed-through effect due to the acid nature of the inks used, resulting
[...] Read more.
Over the last decades, plenty of ancient manuscripts have been digitized all over the world, and particularly in Europe. The fruition of these huge digital archives is often limited by the bleed-through effect due to the acid nature of the inks used, resulting in very noisy images. Several authors have recently worked on bleed-through removal, using different approaches. With the aim of developing a bleed-through removal tool, capable of batch application on a large number of images, of the order of hundred thousands, we used machine learning and robust statistical methods with four different methods, and applied them to two medieval manuscripts. The methods used are (i) non-local means (NLM); (ii) Gaussian mixture models (GMMs); (iii) biweight estimation; and (iv) Gaussian blur. The application of these methods to the two quoted manuscripts shows that these methods are, in general, quite effective in bleed-through removal, but the selection of the method has to be performed according to the characteristics of the manuscript, e.g., if there is no ink fading and the difference between bleed-through pixels and the foreground text is clear, we can use a stronger model without the risk of losing important information. Conversely, if the distinction between bleed-through and foreground pixels is less pronounced, it is better to use a weaker model to preserve useful details.
Full article
(This article belongs to the Section Document Analysis and Processing)
►▼
Show Figures
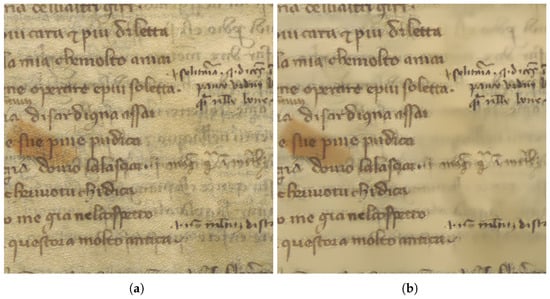
Figure 1
Open AccessArticle
Breast Lesion Detection Using Weakly Dependent Customized Features and Machine Learning Models with Explainable Artificial Intelligence
by
Simona Moldovanu, Dan Munteanu, Keka C. Biswas and Luminita Moraru
J. Imaging 2025, 11(5), 135; https://doi.org/10.3390/jimaging11050135 - 28 Apr 2025
Abstract
This research proposes a novel strategy for accurate breast lesion classification that combines explainable artificial intelligence (XAI), machine learning (ML) classifiers, and customized weakly dependent features from ultrasound (BU) images. Two new weakly dependent feature classes are proposed to improve the diagnostic accuracy
[...] Read more.
This research proposes a novel strategy for accurate breast lesion classification that combines explainable artificial intelligence (XAI), machine learning (ML) classifiers, and customized weakly dependent features from ultrasound (BU) images. Two new weakly dependent feature classes are proposed to improve the diagnostic accuracy and diversify the training data. These are based on image intensity variations and the area of bounded partitions and provide complementary rather than overlapping information. ML classifiers such as Random Forest (RF), Extreme Gradient Boosting (XGB), Gradient Boosting Classifiers (GBC), and LASSO regression were trained with both customized feature classes. To validate the reliability of our study and the results obtained, we conducted a statistical analysis using the McNemar test. Later, an XAI model was combined with ML to tackle the influence of certain features, the constraints of feature selection, and the interpretability capabilities across various ML models. LIME (Local Interpretable Model-Agnostic Explanations) and SHAP (SHapley Additive exPlanations) models were used in the XAI process to enhance the transparency and interpretation in clinical decision-making. The results revealed common relevant features for the malignant class, consistently identified by all of the classifiers, and for the benign class. However, we observed variations in the feature importance rankings across the different classifiers. Furthermore, our study demonstrates that the correlation between dependent features does not impact explainability.
Full article
(This article belongs to the Special Issue Celebrating the 10th Anniversary of the Journal of Imaging)
►▼
Show Figures
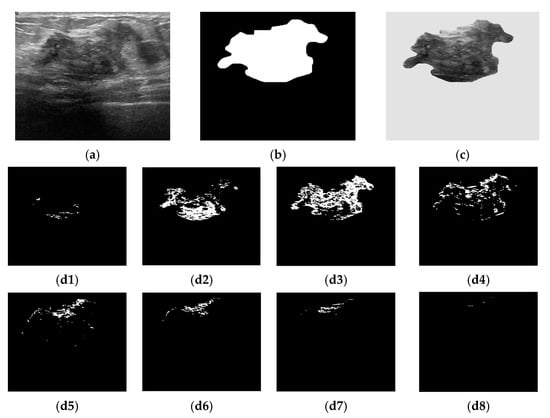
Figure 1
Open AccessArticle
Bilingual Sign Language Recognition: A YOLOv11-Based Model for Bangla and English Alphabets
by
Nawshin Navin, Fahmid Al Farid, Raiyen Z. Rakin, Sadman S. Tanzim, Mashrur Rahman, Shakila Rahman, Jia Uddin and Hezerul Abdul Karim
J. Imaging 2025, 11(5), 134; https://doi.org/10.3390/jimaging11050134 - 27 Apr 2025
Abstract
Communication through sign language effectively helps both hearing- and speaking-impaired individuals connect. However, there are problems with the interlingual communication between Bangla Sign Language (BdSL) and English Sign Language (ASL) due to the absence of a unified system. This study aims to introduce
[...] Read more.
Communication through sign language effectively helps both hearing- and speaking-impaired individuals connect. However, there are problems with the interlingual communication between Bangla Sign Language (BdSL) and English Sign Language (ASL) due to the absence of a unified system. This study aims to introduce a detection system that incorporates these two sign languages to enhance the flow of communication for those who use these forms of sign language. This study developed and tested a deep learning-based sign-language detection system that can recognize both BdSL and ASL alphabets concurrently in real time. The approach uses a YOLOv11 object detection architecture that has been trained with an open-source dataset on a set of 9556 images containing 64 different letter signs from both languages. Data preprocessing was applied to enhance the performance of the model. Evaluation criteria, including the precision, recall, mAP, and other parameter values were also computed to evaluate the model. The performance analysis of the proposed method shows a precision of 99.12% and average recall rates of 99.63% in 30 epochs. The studies show that the proposed model outperforms the current techniques in sign language recognition (SLR) and can be used in communicating assistive technologies and human–computer interaction systems.
Full article
(This article belongs to the Section Computer Vision and Pattern Recognition)
►▼
Show Figures
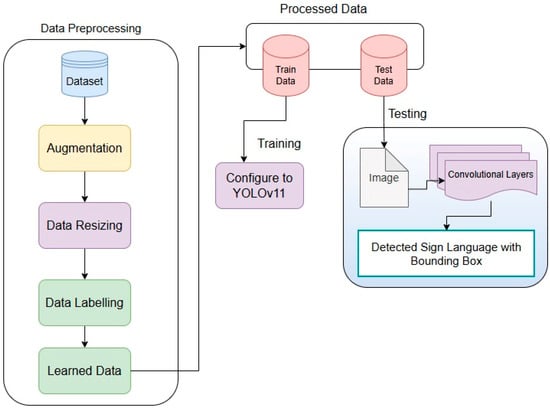
Figure 1
Open AccessReview
A Comprehensive Review on Document Image Binarization
by
Bilal Bataineh, Mohamed Tounsi, Nuha Zamzami, Jehan Janbi, Waleed Abdel Karim Abu-ain, Tarik AbuAin and Shaima Elnazer
J. Imaging 2025, 11(5), 133; https://doi.org/10.3390/jimaging11050133 - 26 Apr 2025
Abstract
In today’s digital age, the conversion of hardcopy documents into digital formats is widespread. This process involves electronically scanning and storing large volumes of documents. These documents come from various sources, including records and reports, camera-captured text and screen snapshots, official documents, newspapers,
[...] Read more.
In today’s digital age, the conversion of hardcopy documents into digital formats is widespread. This process involves electronically scanning and storing large volumes of documents. These documents come from various sources, including records and reports, camera-captured text and screen snapshots, official documents, newspapers, medical reports, music scores, and more. In the domain of document analysis techniques, an essential step is document image binarization. Its goal is to eliminate unnecessary data from images and preserve only the text. Despite the existence of multiple techniques for binarization, the presence of degradation in document images can hinder their efficacy. The objective of this work is to provide an extensive review and analysis of the document binarization field, emphasizing its importance and addressing the challenges encountered during the image binarization process. Additionally, it provides insights into techniques and methods employed for image binarization. The current paper also introduces benchmark datasets for evaluating binarization accuracy, model training, evaluation metrics, and the effectiveness of recent methods.
Full article
(This article belongs to the Section Document Analysis and Processing)
►▼
Show Figures
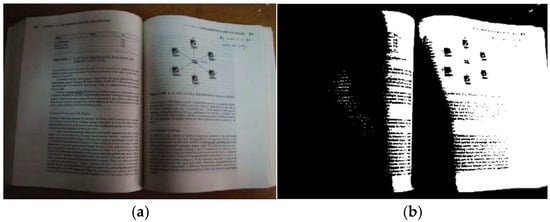
Figure 1
Open AccessArticle
Unveiling the Ultimate Meme Recipe: Image Embeddings for Identifying Top Meme Templates from r/Memes
by
Jan Sawicki
J. Imaging 2025, 11(5), 132; https://doi.org/10.3390/jimaging11050132 - 23 Apr 2025
Abstract
Meme analysis, particularly identifying top meme templates, is crucial for understanding digital culture, communication trends, and the spread of online humor, as memes serve as units of cultural transmission that shape public discourse. Tracking popular templates enables researchers to examine their role in
[...] Read more.
Meme analysis, particularly identifying top meme templates, is crucial for understanding digital culture, communication trends, and the spread of online humor, as memes serve as units of cultural transmission that shape public discourse. Tracking popular templates enables researchers to examine their role in social engagement, ideological framing, and viral dynamics within digital ecosystems. This study explored the viral nature of memes by analyzing a large dataset of over 1.5 million meme submissions from Reddit’s r/memes subreddit, spanning from January 2021 to July 2024. The focus was on uncovering the most popular meme templates by applying advanced image processing techniques. Apart from building an overall understanding of the memesphere, the main contribution was a selection of top meme templates providing a recipe for the best meme template for the meme creators (memesters). Using Vision Transformer (ViT) models, visual features of memes were analyzed without the influence of text, and memes were grouped into 1000 clusters that represented distinct templates. By combining image captioning and keyword extraction methods, key characteristics of the templates were identified, highlighting those with the most visual consistency. A deeper examination of the most popular memes revealed that factors like timing, cultural relevance, and references to current events played a significant role in their virality. Although user identity had limited influence on meme success, a closer look at contributors revealed an interesting pattern of a bot account and two prominent users. Ultimately, the study pinpointed the ten most popular meme templates, many of which were based on pop culture, offering insights into what makes a meme likely to go viral in today’s digital culture.
Full article
(This article belongs to the Section Image and Video Processing)
►▼
Show Figures
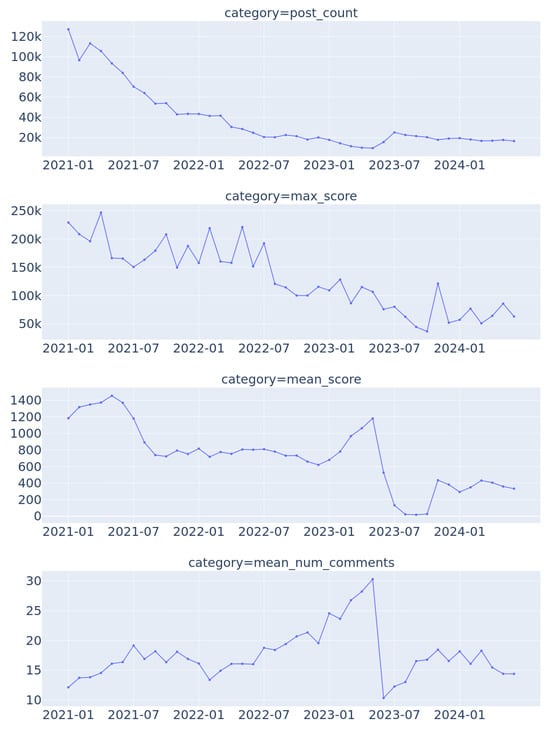
Figure 1
Open AccessCommunication
Improvements in Image Registration, Segmentation, and Artifact Removal in ThermOcular Imaging System
by
Navid Shahsavari, Ehsan Zare Bidaki, Alexander Wong and Paul J. Murphy
J. Imaging 2025, 11(5), 131; https://doi.org/10.3390/jimaging11050131 - 23 Apr 2025
Abstract
The assessment of ocular surface temperature (OST) plays a pivotal role in the diagnosis and management of various ocular diseases. This paper introduces significant enhancements to the ThermOcular system, initially developed for precise OST measurement using infrared (IR) thermography. These advancements focus on
[...] Read more.
The assessment of ocular surface temperature (OST) plays a pivotal role in the diagnosis and management of various ocular diseases. This paper introduces significant enhancements to the ThermOcular system, initially developed for precise OST measurement using infrared (IR) thermography. These advancements focus on accuracy improvements that reduce user dependency and increase the system’s diagnostic capabilities. A novel addition to the system includes the use of EyeTags, which assist clinicians in selecting control points more easily, thus reducing errors associated with manual selection. Furthermore, the integration of state-of-the-art semantic segmentation models trained on the newest dataset is explored. Among these, the OCRNet-HRNet-w18 model achieved a segmentation accuracy of 96.21% MIOU, highlighting the effectiveness of the improved pipeline. Additionally, the challenge of eliminating eyelashes in IR frames, which cause artifactual measurement errors in OST assessments, is addressed. Through a newly developed method, the influence of eyelashes is eliminated, thereby enhancing the precision of temperature readings. Moreover, an algorithm for blink detection and elimination is implemented, significantly improving upon the basic methods previously utilized. These innovations not only enhance the reliability of OST measurements, but also contribute to the system’s efficiency and diagnostic accuracy, marking a significant step forward in ocular health monitoring and diagnostics.
Full article
(This article belongs to the Section Image and Video Processing)
►▼
Show Figures
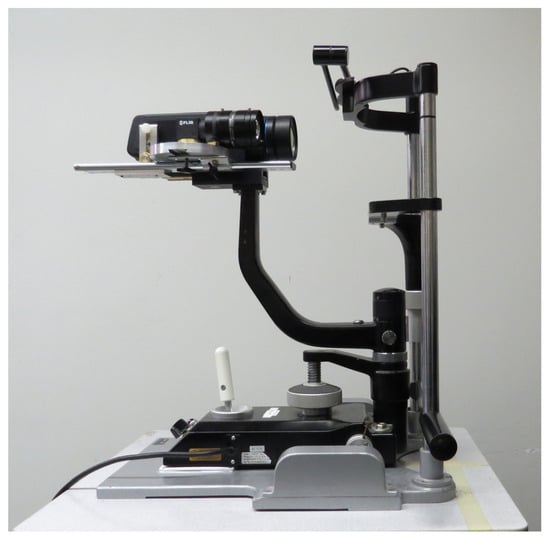
Figure 1
Open AccessArticle
Correlation Between SCORE2-Diabetes and Coronary Artery Calcium Score in Patients with Type 2 Diabetes Mellitus: A Cross-Sectional Study in Vietnam
by
Hung Phi Truong, Hoang Minh Tran, Thuan Huynh, Dung N. Q. Nguyen, Dung Thuong Ho, Cuong Cao Tran, Sang Van Nguyen and Tuan Minh Vo
J. Imaging 2025, 11(5), 130; https://doi.org/10.3390/jimaging11050130 - 23 Apr 2025
Abstract
(1) Background: The SCORE2-Diabetes model has been developed as an effective tool to estimate the 10-year cardiovascular risk in patients with diabetes. Coronary computed tomography angiography (CCTA) and its derived Coronary Artery Calcium Score (CACS) are widely used non-invasive imaging tools for assessing
[...] Read more.
(1) Background: The SCORE2-Diabetes model has been developed as an effective tool to estimate the 10-year cardiovascular risk in patients with diabetes. Coronary computed tomography angiography (CCTA) and its derived Coronary Artery Calcium Score (CACS) are widely used non-invasive imaging tools for assessing coronary artery disease (CAD). This study aimed to evaluate the correlation between CACS and SCORE2-Diabetes in patients with T2DM. (2) Methods: A cross-sectional study was conducted from October 2023 to May 2024. We included patients aged 40 to 69 years with T2DM who underwent a coronary multislice CT scan due to atypical angina. The correlation between CACS and SCORE2-Diabetes was analyzed using Spearman’s rank correlation coefficient. (3) Results: A total of 100 patients met the inclusion criteria, including 71 males and 29 females, with a mean age of 61.9 ± 5.4 years. The differences in CACS and SCORE2-Diabetes among different degrees of coronary artery stenosis were statistically significant (p < 0.05). A statistically significant but weak positive correlation was observed between CACS and SCORE2-Diabetes across all risk categories, with Spearman’s rank correlation coefficients ranging from 0.27 to 0.28 (p < 0.01). (4) Conclusions: Despite the weak correlation between CACS and SCORE2-Diabetes, understanding their relationship and independent associations with disease severity is valuable. The combination of these two tools may warrant investigation in future studies to potentially enhance cardiovascular risk assessment in T2DM patients.
Full article
(This article belongs to the Section Medical Imaging)
►▼
Show Figures
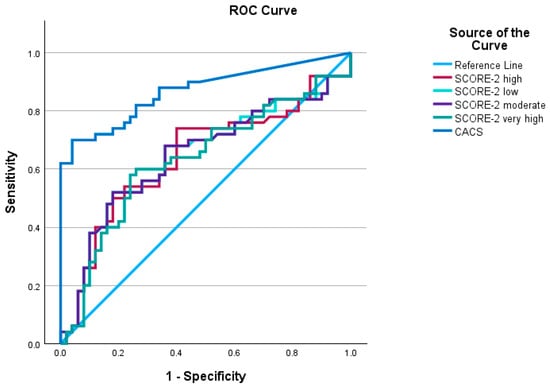
Figure 1
Open AccessArticle
Method for Indoor Seismic Intensity Assessment Based on Image Processing Techniques
by
Jingsong Yang, Guoxing Lu, Yanxiong Wu and Fumin Peng
J. Imaging 2025, 11(5), 129; https://doi.org/10.3390/jimaging11050129 - 22 Apr 2025
Abstract
The seismic intensity experienced indoors directly reflects the degree of damage to the internal structure of the building. The current classification of indoor strength relies on manual surveys and qualitative descriptions of macro phenomena, which are subjective, unable to capture real-time dynamic changes
[...] Read more.
The seismic intensity experienced indoors directly reflects the degree of damage to the internal structure of the building. The current classification of indoor strength relies on manual surveys and qualitative descriptions of macro phenomena, which are subjective, unable to capture real-time dynamic changes in the room, and lack quantitative indicators. In this paper, we present the Image Evaluation of Seismic Intensity (IESI) method, which is based on image processing technology. This method mainly evaluates the degree of responses from objects by identifying the percentage of movement of different types of objects in images taken before and after an earthquake. In order to further improve the recognition accuracy, we combined the camera vibration degree and the object displacement between images to correct the generated earthquake intensity level estimation, so as to achieve the rapid assessment of an earthquake’s intensity indoors. We took, as an example, 29 sets of seismic data from different scenarios. We used the IESI method to evaluate the seismic intensity of these scenarios. Compared with the seismic intensity evaluation results obtained by the Post-disaster Sensor-based Condition Assessment of Buildings (PSAB) and the Image-based Seismic Damage Assessment System (IDEAS) methods, the accuracy of the IESI method was higher by more than 30%, and its accuracy reached 97%. The universality of the IESI method in different indoor scenarios was demonstrated. In a low-intensity evaluation experiment, the accuracy of the IESI method also reached 91%, which verifies the reliability of the IESI method in low-intensity regions.
Full article
(This article belongs to the Section Image and Video Processing)
►▼
Show Figures
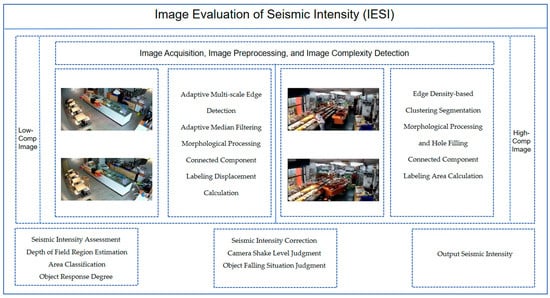
Figure 1
Open AccessArticle
Revisiting Wölfflin in the Age of AI: A Study of Classical and Baroque Composition in Generative Models
by
Adrien Deliege, Maria Giulia Dondero and Enzo D’Armenio
J. Imaging 2025, 11(5), 128; https://doi.org/10.3390/jimaging11050128 - 22 Apr 2025
Abstract
This study explores how contemporary text-to-image models interpret and generate Classical and Baroque styles under Wölfflin’s framework—two categories that are atemporal and transversal across media. Our goal is to see whether generative AI can replicate the nuanced stylistic cues that art historians attribute
[...] Read more.
This study explores how contemporary text-to-image models interpret and generate Classical and Baroque styles under Wölfflin’s framework—two categories that are atemporal and transversal across media. Our goal is to see whether generative AI can replicate the nuanced stylistic cues that art historians attribute to them. We prompted two popular models (DALL•E and Midjourney) using explicit style labels (e.g., “baroque” and “classical”) as well as more implicit cues (e.g., “dynamic”, “static”, or reworked Wölfflin descriptors). We then collected expert ratings and conducted broader qualitative reviews to assess how each output aligned with Wölfflin’s characteristics. Our findings suggest that the term “baroque” usually evokes features recognizable in typically historical Baroque artworks, while “classical” often yields less distinct results, particularly when a specified genre (portrait, still life) imposes a centered, closed-form composition. Removing explicit style labels may produce highly abstract images, revealing that Wölfflin’s descriptors alone may be insufficient to convey Classical or Baroque styles efficiently. Interestingly, the term “dynamic” gives rather chaotic images, yet this chaos is somehow ordered, centered, and has an almost Classical feel. Altogether, these observations highlight the complexity of bridging canonical stylistic frameworks and contemporary AI training biases, underscoring the need to update or refine Wölfflin’s atemporal categories to accommodate how generative models—and modern visual culture—reinterpret Classical and Baroque.
Full article
(This article belongs to the Special Issue Celebrating the 10th Anniversary of the Journal of Imaging)
►▼
Show Figures
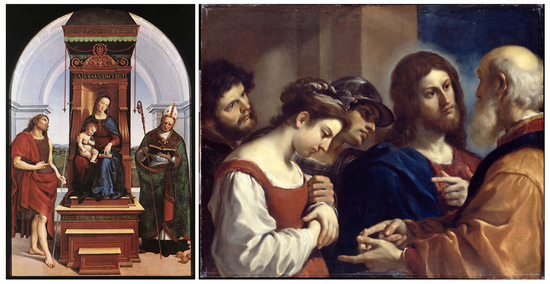
Figure 1
Open AccessArticle
Reliability of Quadriceps and Hamstring Soft Tissue Measures Using Dual-Energy X-Ray Absorptiometry (DXA) Scans
by
Trey R. Naylor, Mariana V. Jacobs, Cameron J. Elder, Michael A. Samaan and Jody L. Clasey
J. Imaging 2025, 11(5), 127; https://doi.org/10.3390/jimaging11050127 - 22 Apr 2025
Abstract
Purpose: The purpose of this study was to determine the intra- and interrater reliability of quadriceps and hamstring soft tissue measures using DXA scans. Methods: A total of 44 subjects (23 males) participated in this study. The first total body DXA scan was
[...] Read more.
Purpose: The purpose of this study was to determine the intra- and interrater reliability of quadriceps and hamstring soft tissue measures using DXA scans. Methods: A total of 44 subjects (23 males) participated in this study. The first total body DXA scan was performed in the standard anterior/posterior scanning position, followed by two additional total body scans while the subjects were lying on their left and right sides with the leg of interest extended and the contralateral leg bent. Unique regions of interest were created for analyses of mineral-free lean masses (MFL) using custom analysis software with manual tracing (by two investigators) of the quadriceps (QUADS) and hamstrings (HAMS) of the right and left thighs. Between–within repeated measure analysis of variance (ANOVA) was used to determine if there were significant differences among the MFL measures, while intraclass correlation coefficients (ICC) and coefficients of variation (CV) were used to assess the intra- and interrater reliability. Results: Between-group analyses revealed that Investigator 2 had small yet significantly higher mean differences for right QUADS (2346.6 ± 602.4 g vs. 2327.4 ± 587.9 g), left QUADS (2337.3 ± 581.9 g vs. 2312.8 ± 581.2 g), right HAMS (2655.9 ± 626.3 g vs. 2543.0 ± 593.5 g), and left HAMS (2686.1 ± 628.1 g vs. 2562.8 ± 596.5 g) when compared to Investigator 1. Intraclass correlation coefficients between (≥0.984) and within (≥0.992) raters were high for all MFL measures, with low variation across all MFL measures (≤1.62%). Conclusions: Despite having significant group mean differences, our results revealed strong and significant reliability, and we recommend that a single investigator analyze the scans twice and that the mean of the two measures be used for final reporting within a given study.
Full article
(This article belongs to the Section Medical Imaging)
►▼
Show Figures
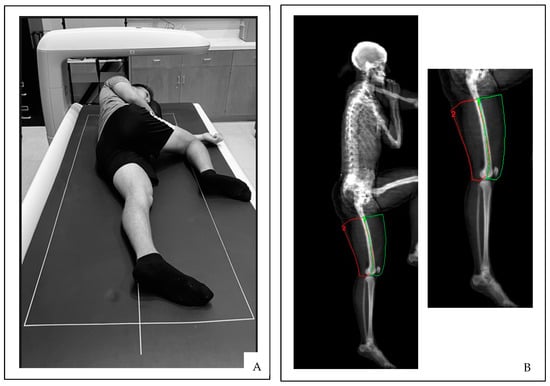
Figure 1
Open AccessArticle
Real-Time Volume-Rendering Image Denoising Based on Spatiotemporal Weighted Kernel Prediction
by
Xinran Xu, Chunxiao Xu and Lingxiao Zhao
J. Imaging 2025, 11(4), 126; https://doi.org/10.3390/jimaging11040126 - 21 Apr 2025
Abstract
Volumetric Path Tracing (VPT) based on Monte Carlo (MC) sampling often requires numerous samples for high-quality images, but real-time applications limit samples to maintain interaction rates, leading to significant noise. Traditional real-time denoising methods use radiance and geometric features as neural network inputs,
[...] Read more.
Volumetric Path Tracing (VPT) based on Monte Carlo (MC) sampling often requires numerous samples for high-quality images, but real-time applications limit samples to maintain interaction rates, leading to significant noise. Traditional real-time denoising methods use radiance and geometric features as neural network inputs, but lightweight networks struggle with temporal stability and complex mapping relationships, causing blurry results. To address these issues, a spatiotemporal lightweight neural network is proposed to enhance the denoising performance of VPT-rendered images with low samples per pixel. First, the reprojection technique was employed to obtain features from historical frames. Next, a dual-input convolutional neural network architecture was designed to predict filtering kernels. Radiance and geometric features were encoded independently. The encoding of geometric features guided the pixel-wise fitting of radiance feature filters. Finally, learned weight filtering kernels were applied to images’ spatiotemporal filtering to produce denoised results. The experimental results across multiple denoising datasets demonstrate that this approach outperformed the baseline models in terms of feature extraction and detail representation capabilities while effectively suppressing noise with superior performance and enhanced temporal stability.
Full article
(This article belongs to the Topic Visual Computing and Understanding: New Developments and Trends)
►▼
Show Figures
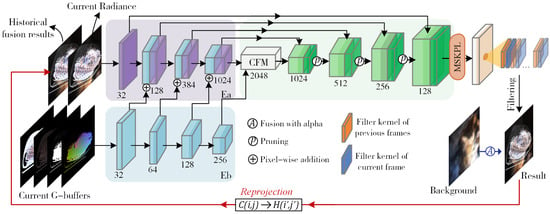
Figure 1
Open AccessReview
Low-Light Image and Video Enhancement for More Robust Computer Vision Tasks: A Review
by
Mpilo M. Tatana, Mohohlo S. Tsoeu and Rito C. Maswanganyi
J. Imaging 2025, 11(4), 125; https://doi.org/10.3390/jimaging11040125 - 21 Apr 2025
Abstract
Computer vision aims to enable machines to understand the visual world. Computer vision encompasses numerous tasks, namely action recognition, object detection and image classification. Much research has been focused on solving these tasks, but one that remains relatively uncharted is light enhancement (LE).
[...] Read more.
Computer vision aims to enable machines to understand the visual world. Computer vision encompasses numerous tasks, namely action recognition, object detection and image classification. Much research has been focused on solving these tasks, but one that remains relatively uncharted is light enhancement (LE). Low-light enhancement (LLE) is crucial as computer vision tasks fail in the absence of sufficient lighting, having to rely on the addition of peripherals such as sensors. This review paper will shed light on this (focusing on video enhancement) subfield of computer vision, along with the other forementioned computer vision tasks. The review analyzes both traditional and deep learning-based enhancers and provides a comparative analysis on recent models in the field. The review also analyzes how popular computer vision tasks are improved and made more robust when coupled with light enhancement algorithms. Results show that deep learners outperform traditional enhancers, with supervised learners obtaining the best results followed by zero-shot learners, while computer vision tasks are improved with light enhancement coupling. The review concludes by highlighting major findings such as that although supervised learners obtain the best results, due to a lack of real-world data and robustness to new data, a shift to zero-shot learners is required.
Full article
(This article belongs to the Section Computer Vision and Pattern Recognition)
►▼
Show Figures
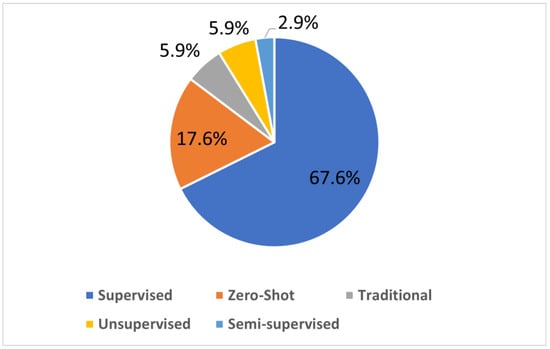
Figure 1
Open AccessArticle
Evolution of Lung Disease Studied by Computed Tomography in Adults with Cystic Fibrosis Treated with Elexacaftor/Tezacaftor/Ivacaftor
by
Susana Hernández-Muñiz, Paloma Caballero, Adrián Peláez, Marta Solís-García, Carmen de Benavides, Javier Collada, Ignacio Díaz-Lorenzo, Cristina Zorzo, Rosa Mar Gómez-Punter and Rosa María Girón
J. Imaging 2025, 11(4), 124; https://doi.org/10.3390/jimaging11040124 - 21 Apr 2025
Abstract
Elexacaftor–tezacaftor–ivacaftor (ETI) has shown clinical and spirometric benefits in cystic fibrosis (CF). CT remains a vital tool for diagnosing and monitoring structural lung disease. This study aimed to assess the evolution of lung disease, as evaluated through CT, in adults with CF after
[...] Read more.
Elexacaftor–tezacaftor–ivacaftor (ETI) has shown clinical and spirometric benefits in cystic fibrosis (CF). CT remains a vital tool for diagnosing and monitoring structural lung disease. This study aimed to assess the evolution of lung disease, as evaluated through CT, in adults with CF after at least one year of ETI treatment. This ambispective observational analysis assessed lung CT scans performed before initiating ETI and after at least one year of treatment, using the modified Bhalla scoring system. For those patients with an earlier CT scan, a pre-treatment phase analysis was performed. Epidemiological, clinical, and functional parameters were evaluated. Results: Sixty-two patients were included (35 males, median age 30.4 ± 7.87 years). After at least one year of ETI, significant improvements were observed in the global CT Bhalla score (12.2 ± 2.8 vs. 14.0 ± 2.8), peribronchial thickening (1.4 ± 0.6 vs. 1.0 ± 0.4), and mucus plugging (1.6 ± 0.7 vs. 0.8 ± 0.6) (p < 0.001). Spirometry parameters increased significantly: the percentage of the predicted forced expiratory volume in the first second (ppFEV1) increased from 66.5 ± 19.8 to 77.0 ± 20.4 (p = 0.005) and forced vital capacity (ppFVC) from 80.6 ± 16.4 to 91.6 ± 14.1 (p < 0.001). Additionally, body mass index showed a significant increase. A moderate correlation was found between the Bhalla score and spirometry results. In the pre-treatment phase (n = 52), mucus plugging demonstrated a significant worsening, whereas global CT score, other subscores, and spirometry did not change significantly. Conclusions: In adults with CF, after at least one year of ETI, a significant improvement in structural lung disease was achieved, as reflected by the CT Bhalla score.
Full article
(This article belongs to the Special Issue Tools and Techniques for Improving Radiological Imaging Applications)
►▼
Show Figures
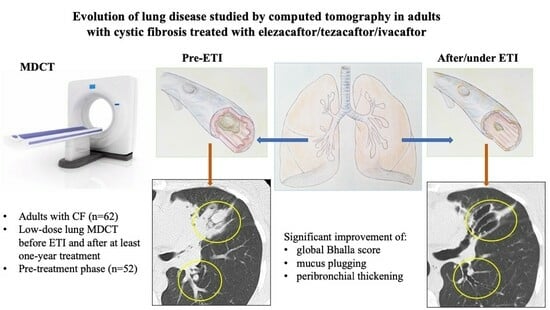
Graphical abstract
Open AccessArticle
Evolutionary-Driven Convolutional Deep Belief Network for the Classification of Macular Edema in Retinal Fundus Images
by
Rafael A. García-Ramírez, Ivan Cruz-Aceves, Arturo Hernández-Aguirre, Gloria P. Trujillo-Sánchez and Martha A. Hernandez-González
J. Imaging 2025, 11(4), 123; https://doi.org/10.3390/jimaging11040123 - 21 Apr 2025
Abstract
Early detection of diabetic retinopathy is critical for preserving vision in diabetic patients. The classification of lesions in Retinal fundus images, particularly macular edema, is an essential diagnostic tool, yet it presents a significant learning curve for both novice and experienced ophthalmologists. To
[...] Read more.
Early detection of diabetic retinopathy is critical for preserving vision in diabetic patients. The classification of lesions in Retinal fundus images, particularly macular edema, is an essential diagnostic tool, yet it presents a significant learning curve for both novice and experienced ophthalmologists. To address this challenge, a novel Convolutional Deep Belief Network (CDBN) is proposed to classify image patches into three distinct categories: two types of macular edema—microhemorrhages and hard exudates—and a healthy category. The method leverages high-level feature extraction to mitigate issues arising from the high similarity of low-level features in noisy images. Additionally, a Real-Coded Genetic Algorithm optimizes the parameters of Gabor filters and the network, ensuring optimal feature extraction and classification performance. Experimental results demonstrate that the proposed CDBN outperforms comparative models, achieving an F1 score of 0.9258. These results indicate that the architecture effectively overcomes the challenges of lesion classification in retinal images, offering a robust tool for clinical application and paving the way for advanced clinical decision support systems in diabetic retinopathy management.
Full article
(This article belongs to the Special Issue Advances in Biomedical Image Processing and Artificial Intelligence for Computer-Aided Diagnosis in Medicine)
►▼
Show Figures
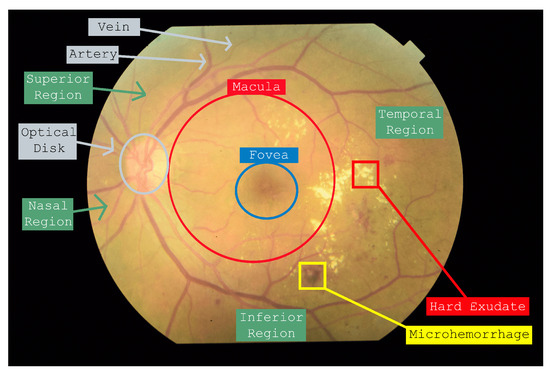
Figure 1
Open AccessArticle
Classification of Parotid Tumors with Robust Radiomic Features from DCE- and DW-MRI
by
Francesca Angelone, Silvia Tortora, Francesca Patella, Maria Chiara Bonanno, Maria Teresa Contaldo, Mario Sansone, Gianpaolo Carrafiello, Francesco Amato and Alfonso Maria Ponsiglione
J. Imaging 2025, 11(4), 122; https://doi.org/10.3390/jimaging11040122 - 17 Apr 2025
Abstract
This study aims to evaluate the role of MRI-based radiomic analysis and machine learning using both DWI with multiple B-values and dynamic contrast-enhanced T1-weighted sequences to differentiate benign (B) and malignant (M) parotid tumors. Patients underwent DCE- and DW-MRI. An expert radiologist performed
[...] Read more.
This study aims to evaluate the role of MRI-based radiomic analysis and machine learning using both DWI with multiple B-values and dynamic contrast-enhanced T1-weighted sequences to differentiate benign (B) and malignant (M) parotid tumors. Patients underwent DCE- and DW-MRI. An expert radiologist performed the manual selection of 3D ROIs. Classification of malignant vs. benign parotid tumors was based on radiomic features extracted from DCE-based and DW-based parametric maps. Care was taken in robustness evaluation and the no-bias selection of features. Several classifiers were employed. Sensitivity and specificity ranged from 0.6 to 0.8. The combination of LASSO + neural networks achieved the highest performance (0.76 sensitivity and 0.75 specificity). Our study identified a few robust DCE-based radiomic features with respect to ROI selection that can effectively be adopted in classifying malignant vs. benign parotid tumors.
Full article
(This article belongs to the Section Medical Imaging)
►▼
Show Figures
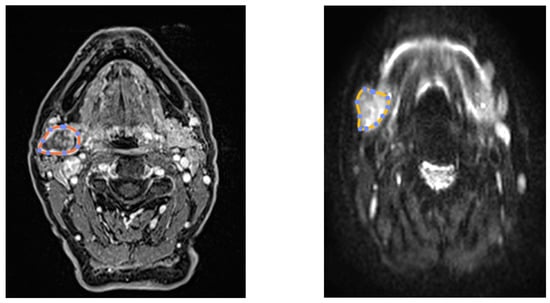
Figure 1
Open AccessArticle
Implementation of Chaotic Synchronization and Artificial Neural Networks in Modified OTP Scheme for Image Encryption
by
Hristina Stoycheva, Georgi Mihalev, Stanimir Sadinov and Krasen Angelov
J. Imaging 2025, 11(4), 121; https://doi.org/10.3390/jimaging11040121 - 17 Apr 2025
Abstract
This paper presents a modified image encryption scheme based on the OTP (One-Time Pad) algorithm, consisting of chaotic synchronization and artificial neural networks (ANNs) for improved security and efficiency. The scheme uses chaotic synchronization based on feedback control to create complex and unique
[...] Read more.
This paper presents a modified image encryption scheme based on the OTP (One-Time Pad) algorithm, consisting of chaotic synchronization and artificial neural networks (ANNs) for improved security and efficiency. The scheme uses chaotic synchronization based on feedback control to create complex and unique encryption keys. Additionally, ANNs are used to approximate time functions, creating a neural encoding key, which adds an additional layer of complexity to the encryption process. The proposed scheme integrates static, chaotic, and neural keys in a multilayer structure, providing high resistance against statistical and cryptographic attacks. The results show that the proposed methodology achieves entropy values close to the theoretical maximum, effectively destroys the correlation between pixels, and demonstrates high sensitivity to variations in the input data. The proposed scheme shows very good feasibility in terms of both security and efficiency, which gives a reliable solution for secure image transmission and storage. This is proven by a study of resistance to various crypto–graphic attacks such as brute force attack, differential attack, noise and data cut attacks, key sensitivity, and computational complexity.
Full article
(This article belongs to the Section Image and Video Processing)
►▼
Show Figures
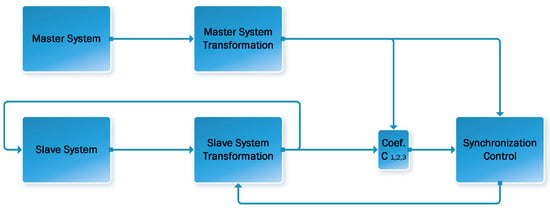
Figure 1
Open AccessArticle
RGB Color Space-Enhanced Training Data Generation for Cucumber Classification
by
Hotaka Hoshino, Takuya Shindo, Takefumi Hiraguri and Nobuhiko Itoh
J. Imaging 2025, 11(4), 120; https://doi.org/10.3390/jimaging11040120 - 17 Apr 2025
Abstract
Cucumber farmers classify harvested cucumbers based on specific criteria before they are introduced to the market. During peak harvesting periods, farmers must process a large volume of cucumbers; however, the classification task requires specialized knowledge and experience. This expertise-dependent process poses a significant
[...] Read more.
Cucumber farmers classify harvested cucumbers based on specific criteria before they are introduced to the market. During peak harvesting periods, farmers must process a large volume of cucumbers; however, the classification task requires specialized knowledge and experience. This expertise-dependent process poses a significant challenge, as it prevents untrained individuals, including hired workers, from effectively assisting in classification, thereby necessitating that farmers perform the task themselves. To address this issue, this study aims to develop a classification system that enables individuals, regardless of their level of expertise, to accurately classify cucumbers. The proposed system employs a convolutional neural network (CNN) to process cucumber images and generate classification results. The CNN used in this study consists of a total of 11 layers: 2 convolution layers, 2 pooling layers, 3 dense layers, and 4 dropout layers. To facilitate the widespread adoption of this system, improving classification accuracy is imperative. In this paper, we propose a method for embedding information related to cucumber length, bend, and thickness into the background space of cucumber images when creating training data. Specifically, this method encodes these attributes into the RGB color space, allowing the background color to vary based on the cucumber’s length, bend, and thickness. The effectiveness of the proposed method is validated through an evaluation of multi-class classification metrics, including accuracy, recall, precision, and F-measure, using cucumbers classified based on the criteria established by an actual agricultural cooperative. The experimental results demonstrate that the proposed method improves these evaluation metrics, thereby enhancing the overall performance of the system.Specifically, the proposed method achieved 79.1% accuracy, while the method without RGB color space achieved 70.1% accuracy. This indicates that the proposed method achieves 1.1 times better performance than the conventional method.
Full article
(This article belongs to the Section Image and Video Processing)
►▼
Show Figures
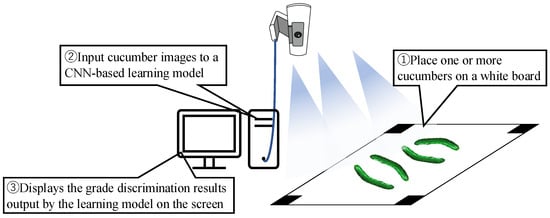
Figure 1
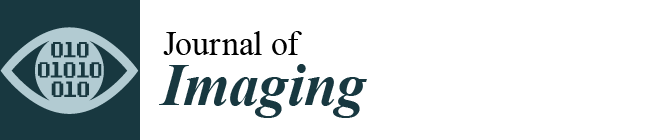
Journal Menu
► ▼ Journal Menu-
- J. Imaging Home
- Aims & Scope
- Editorial Board
- Reviewer Board
- Topical Advisory Panel
- Instructions for Authors
- Special Issues
- Topics
- Sections
- Article Processing Charge
- Indexing & Archiving
- Most Cited & Viewed
- Journal Statistics
- Journal History
- Journal Awards
- Conferences
- Editorial Office
- 10th Anniversary
Journal Browser
► ▼ Journal BrowserHighly Accessed Articles
Latest Books
E-Mail Alert
News
28 April 2025
Meet Us at the 16th International Symposium on Measurement Technology and Intelligent Instruments (ISMTII 2025) and the 3rd International Conference of Optical Imaging and Measurement (ICOIM 2025), 25–28 May 2025, Wuhan, China
Meet Us at the 16th International Symposium on Measurement Technology and Intelligent Instruments (ISMTII 2025) and the 3rd International Conference of Optical Imaging and Measurement (ICOIM 2025), 25–28 May 2025, Wuhan, China
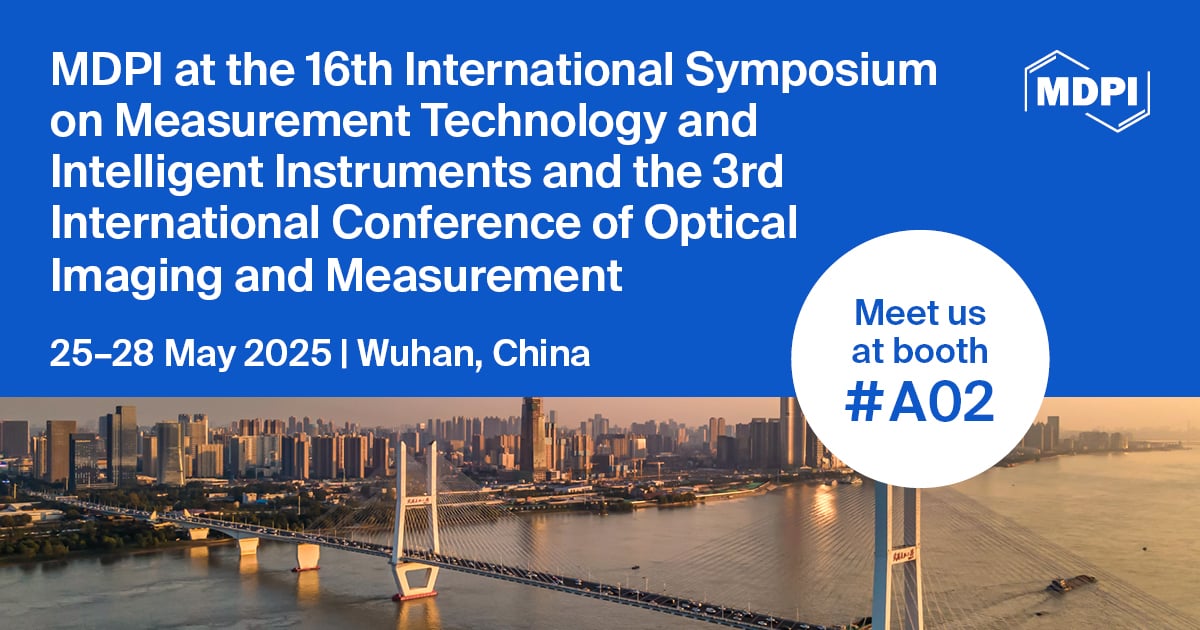
2 April 2025
MDPI INSIGHTS: The CEO's Letter #21 - Annual Report, Swiss Consortium, IWD, ICARS, Serbia
MDPI INSIGHTS: The CEO's Letter #21 - Annual Report, Swiss Consortium, IWD, ICARS, Serbia
Topics
Topic in
Applied Sciences, Computation, Entropy, J. Imaging, Optics
Color Image Processing: Models and Methods (CIP: MM)
Topic Editors: Giuliana Ramella, Isabella TorcicolloDeadline: 30 July 2025
Topic in
Applied Sciences, Bioengineering, Diagnostics, J. Imaging, Signals
Signal Analysis and Biomedical Imaging for Precision Medicine
Topic Editors: Surbhi Bhatia Khan, Mo SaraeeDeadline: 31 August 2025
Topic in
Animals, Computers, Information, J. Imaging, Veterinary Sciences
AI, Deep Learning, and Machine Learning in Veterinary Science Imaging
Topic Editors: Vitor Filipe, Lio Gonçalves, Mário GinjaDeadline: 31 October 2025
Topic in
Applied Sciences, Electronics, MAKE, J. Imaging, Sensors
Applied Computer Vision and Pattern Recognition: 2nd Edition
Topic Editors: Antonio Fernández-Caballero, Byung-Gyu KimDeadline: 31 December 2025

Conferences
Special Issues
Special Issue in
J. Imaging
Object Detection in Video Surveillance Systems
Guest Editors: Jesús Ruiz-Santaquiteria Alegre, Juan Antonio Álvarez García, Harbinder SinghDeadline: 30 April 2025
Special Issue in
J. Imaging
Recent Advancements in 3D Imaging
Guest Editor: Guofeng MeiDeadline: 30 April 2025
Special Issue in
J. Imaging
Explainable AI in Computer Vision
Guest Editor: Bas Van der VeldenDeadline: 30 April 2025
Special Issue in
J. Imaging
Novel Approaches to Image Quality Assessment
Guest Editors: Luigi Celona, Hanhe LinDeadline: 30 April 2025