Journal Description
Remote Sensing
Remote Sensing
is an international, peer-reviewed, open access journal about the science and application of remote sensing technology, and is published semimonthly online by MDPI. The Remote Sensing Society of Japan (RSSJ) and the Japan Society of Photogrammetry and Remote Sensing (JSPRS) are affiliated with Remote Sensing, and their members receive a discount on the article processing charge.
- Open Access— free for readers, with article processing charges (APC) paid by authors or their institutions.
- High Visibility: indexed within Scopus, SCIE (Web of Science), Ei Compendex, PubAg, GeoRef, Astrophysics Data System, Inspec, dblp, and other databases.
- Journal Rank: JCR - Q1 (Geosciences, Multidisciplinary) / CiteScore - Q1 (General Earth and Planetary Sciences)
- Rapid Publication: manuscripts are peer-reviewed and a first decision is provided to authors approximately 23.9 days after submission; acceptance to publication is undertaken in 2.5 days (median values for papers published in this journal in the second half of 2024).
- Recognition of Reviewers: reviewers who provide timely, thorough peer-review reports receive vouchers entitling them to a discount on the APC of their next publication in any MDPI journal, in appreciation of the work done.
- Companion journal: Geomatics
Impact Factor:
4.2 (2023);
5-Year Impact Factor:
4.9 (2023)
Latest Articles
Synergistic Semantic Segmentation and Height Estimation for Monocular Remote Sensing Images via Cross-Task Interaction
Remote Sens. 2025, 17(9), 1637; https://doi.org/10.3390/rs17091637 - 5 May 2025
Abstract
Semantic segmentation and height estimation in remote sensing imagery are two pivotal tasks for scene understanding, and they are highly interrelated. Although deep learning methods have achieved remarkable progress in these tasks in recent years, several challenges remain. Recent studies have shown that
[...] Read more.
Semantic segmentation and height estimation in remote sensing imagery are two pivotal tasks for scene understanding, and they are highly interrelated. Although deep learning methods have achieved remarkable progress in these tasks in recent years, several challenges remain. Recent studies have shown that multi-task learning methods can enhance the complementarity of task-related features, thus maximizing the prediction accuracy of multiple tasks at a low computational cost. However, due to factors such as complex semantic categories and the inconsistent spatial scales of remotely sensed images, existing multi-task learning methods often fail to achieve better results on these two tasks. To address this issue, we propose CTME-Net, a novel architecture termed the Cross-Task Mutual Enhancement Network, designed to jointly perform height estimation and semantic segmentation tasks on remote sensing imagery. Firstly, to generate discriminative initial features for each task branch and activate dedicated pathways for cross-task feature disentanglement, we design a universal initial feature embedding module for each downstream task. Secondly, to address the impact of redundancy in general features during global–local fusion, we develop an Adaptive Task-specific Feature Distillation Module that enhances the model’s ability to acquire task-specific features. Finally, we propose a task feature interaction module to optimize features across tasks through mutual optimization, maximizing task-specific feature expression. We conduct extensive experiments on the ISPRS Vaihingen and Potsdam datasets to validate the effectiveness of our approach. The results demonstrate that our proposed method outperforms existing methods in both height estimation and semantic segmentation.
Full article
(This article belongs to the Special Issue Advances in High-Resolution Satellite Remote Sensing Image Processing and Classification)
Open AccessArticle
Significant Improvement in Short-Term Green-Tide Transport Predictions Using the XGBoost Model
by
Menghao Ji and Chengyi Zhao
Remote Sens. 2025, 17(9), 1636; https://doi.org/10.3390/rs17091636 - 5 May 2025
Abstract
Accurately predicting the drift trajectory of green tides is crucial for assessing potential risks and implementing effective countermeasures. This paper proposes a short-term green-tide drift prediction method that combines green-tide patch characteristics, 1 h interval drift distances from GOCI-II images, and driving-factor data
[...] Read more.
Accurately predicting the drift trajectory of green tides is crucial for assessing potential risks and implementing effective countermeasures. This paper proposes a short-term green-tide drift prediction method that combines green-tide patch characteristics, 1 h interval drift distances from GOCI-II images, and driving-factor data using the XGBoost machine learning model to enhance prediction accuracy. The results demonstrate that the proposed method outperforms the traditional OpenDrift model in short-term predictions. Specifically, at time intervals of 3, 5, and 7 h, the root mean square errors (RMSEs) of the OpenDrift model in the zonal direction are 1.81 km, 2.89 km, and 3.55 km, respectively, whereas the RMSEs of the proposed method are 0.80 km, 0.98 km, and 1.20 km, respectively; in the meridional direction, the RMSEs of the OpenDrift model are 1.77 km, 2.67 km, and 3.10 km, while the RMSEs for the proposed method are 0.82 km, 1.10 km, and 1.25 km, respectively. Furthermore, the proposed XGBoost method more-accurately tracks the actual positions of green-tide patches compared to the OpenDrift model. Specifically, at the 25 h interval, the proposed method continues to accurately predict patch positions, while the OpenDrift model exhibits significant deviations. This study demonstrates that the proposed method, by learning drift patterns from historical data, effectively predicts the short-term drift process of green tides. It provides valuable support for early warning systems, thereby helping to mitigate the ecological and economic impacts of green-tide disasters.
Full article
(This article belongs to the Section Remote Sensing in Geology, Geomorphology and Hydrology)
►▼
Show Figures
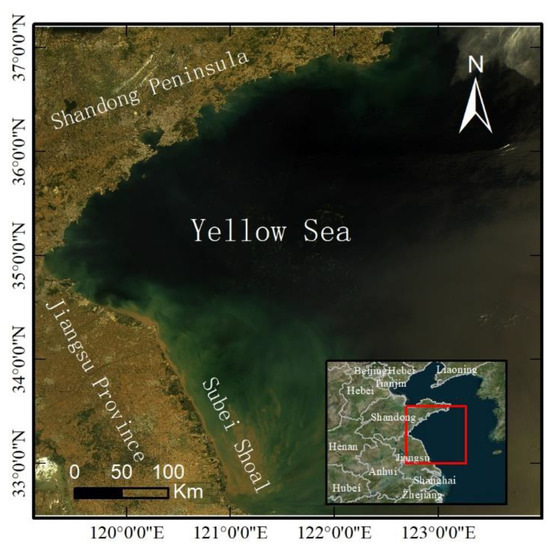
Figure 1
Open AccessArticle
Assimilation of Moderate-Resolution Imaging Spectroradiometer Level Two Cloud Products for Typhoon Analysis and Prediction
by
Haomeng Zhang, Yubao Liu, Yu Qin, Zheng Xiang, Yueqin Shi and Zhaoyang Huo
Remote Sens. 2025, 17(9), 1635; https://doi.org/10.3390/rs17091635 - 5 May 2025
Abstract
A novel data assimilation technique is developed to assimilate MODIS (Moderate Resolution Imaging Spectroradiometer) level two (L2) cloud products, including cloud optical thickness (COT), cloud particle effective radius (Re), cloud water path (CWP), and cloud top pressure (CTP), into the Weather Research and
[...] Read more.
A novel data assimilation technique is developed to assimilate MODIS (Moderate Resolution Imaging Spectroradiometer) level two (L2) cloud products, including cloud optical thickness (COT), cloud particle effective radius (Re), cloud water path (CWP), and cloud top pressure (CTP), into the Weather Research and Forecast (WRF) model. Its impact on the analysis and forecast of Typhoon Talim in 2023 at its initial developing stage is demonstrated. First, the conditional generative adversarial networks–bidirectional ensemble binned probability fusion (CGAN-BEBPF) model ) is applied to retrieve three-dimensional (3D) CloudSat CPR (cloud profiling radar) equivalent W-band (94 Ghz) radar reflectivity factor for the typhoons Talim and Chaba using the MODIS L2 data. Next, a W-band to S-band radar reflectivity factor mapping algorithm (W2S) is developed based on the collocated measurements of the retrieved W-band radar and ground-based S-band (4 Ghz) radar data for Typhoon Chaba at its landfall time. Then, W2S is utilized to project the MODIS-retrieved 3D W-band radar reflectivity factor of Typhoon Talim to equivalent ground-based S-band reflectivity factors. Finally, data assimilation and forecast experiments are conducted by using the WRF Hydrometeor and Latent Heat Nudging (HLHN) radar data assimilation technique. Verification of the simulation results shows that assimilating the MODIS L2 cloud products dramatically improves the initialization and forecast of the cloud and precipitation fields of Typhoon Talim. In comparison to the experiment without assimilation of the MODIS data, the Threat Score (TS) for general cloud areas and major precipitation areas is increased by 0.17 (from 0.46 to 0.63) and 0.28 (from 0.14 to 0.42), respectively. The fraction skill score (FSS) for the 5 mm precipitation threshold is increased by 0.43. This study provides an unprecedented data assimilation method to initialize 3D cloud and precipitation hydrometeor fields with the MODIS imagery payloads for numerical weather prediction models.
Full article
(This article belongs to the Section Atmospheric Remote Sensing)
►▼
Show Figures
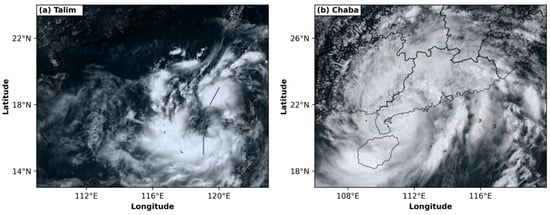
Figure 1
Open AccessArticle
The Study on Landslide Hazards Based on Multi-Source Data and GMLCM Approach
by
Zhifang Zhao, Zhengyu Li, Penghui Lv, Fei Zhao and Lei Niu
Remote Sens. 2025, 17(9), 1634; https://doi.org/10.3390/rs17091634 - 5 May 2025
Abstract
The southwest region of China is characterized by numerous rugged mountains and valleys, which create favorable conditions for landslide disasters. The landslide-influencing factors show different sensitivities regionally, which induces the occurrence of disasters to different degrees, especially in small sample areas. This study
[...] Read more.
The southwest region of China is characterized by numerous rugged mountains and valleys, which create favorable conditions for landslide disasters. The landslide-influencing factors show different sensitivities regionally, which induces the occurrence of disasters to different degrees, especially in small sample areas. This study constructs a framework for the identification, analysis, and evaluation of landslide hazards in complex mountainous regions within small sample areas. This study utilizes small baseline subset interferometric synthetic aperture radar (SBAS-InSAR) technology and high-resolution optical imagery for a comprehensive interpretation to identify landslide hazards. A geodetector is employed to analyze disaster-inducing factors, and machine-learning models such as random forest (RF), gradient boosting decision tree (GBDT), categorical boosting (CatBoost), logistic regression (LR), and stacking ensemble strategies (Stacking) are applied for landslide sensitivity evaluation. GMLCM stands for geodetector–machine-learning-coupled modeling. The results indicate the following: (1) 172 landslide hazards were identified, primarily concentrated along the banks of the Lancang River. (2) A geodetector analysis shows that the key disaster-inducing factors for landslides include a digital elevation model (DEM) (1321–1857 m), rainfall (1181–1290 mm/a), the distance from roads (0–1285 m), and geological rock formation (soft rock formation). (3) Based on the application of the K-means clustering algorithm and the Bayesian optimization algorithm, the GD-CatBoost model shows excellent performance. High-sensitivity zones were predominantly concentrated along the Lancang River, accounting for 24.2% in the study area. The method for identifying landslide hazards and small-sample sensitivity evaluation can provide guidance and insights for landslide monitoring and harnessing in similar geological environments.
Full article
(This article belongs to the Special Issue Geological Hazard Monitoring, Identify, Predict, and Risk Assessment Using Geographic Information Science and Remote Sensing)
►▼
Show Figures
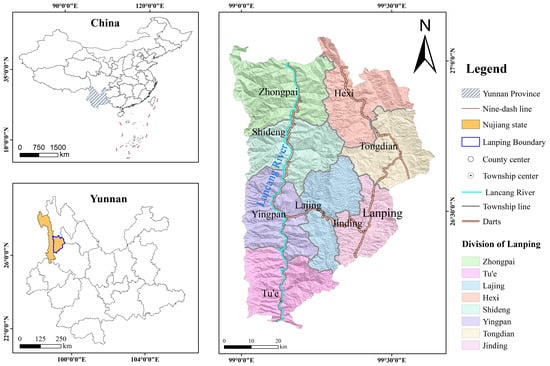
Figure 1
Open AccessArticle
Sea Breeze-Driven Variations in Planetary Boundary Layer Height over Barrow: Insights from Meteorological and Lidar Observations
by
Hui Li, Wei Gong, Boming Liu, Yingying Ma, Shikuan Jin, Weiyan Wang, Ruonan Fan, Shuailong Jiang, Yujie Wang and Zhe Tong
Remote Sens. 2025, 17(9), 1633; https://doi.org/10.3390/rs17091633 - 5 May 2025
Abstract
The planetary boundary layer height (PBLH) in coastal Arctic regions is influenced by sea breeze circulation. However, the specific mechanisms through which sea breeze affects PBLH evolution remain insufficiently explored. This study uses meteorological data, micro-pulse lidar (MPL) data, and sounding profiles from
[...] Read more.
The planetary boundary layer height (PBLH) in coastal Arctic regions is influenced by sea breeze circulation. However, the specific mechanisms through which sea breeze affects PBLH evolution remain insufficiently explored. This study uses meteorological data, micro-pulse lidar (MPL) data, and sounding profiles from 2014 to 2021 to investigate the annual and polar day PBLH evolution driven by sea breezes in the Barrow region of Alaska, as well as the specific mechanisms. The results show that sea breeze events significantly suppress PBLH, especially during the polar day, when prolonged solar radiation intensifies the thermal contrast between land and ocean. The cold, moist sea breeze stabilizes the atmospheric conditions, reducing net radiation and sensible heat flux. All these factors inhibit turbulent mixing and PBLH development. Lidar and sounding analyses further reveal that PBLH is lower during sea breeze events compared to non-sea-breeze conditions, with the peak of its probability density distribution occurring at a lower PBLH range. The variable importance in projection (VIP) analysis identifies relative humidity (VIP = 1.95) and temperature (VIP = 1.1) as the primary factors controlling PBLH, highlighting the influence of atmospheric stability in regulating PBLH. These findings emphasize the crucial role of sea breeze in modulating PBL dynamics in the Arctic, with significant implications for improving climate models and studies on pollutant dispersion in polar regions.
Full article
(This article belongs to the Special Issue Advanced Lidar Remote Sensing for Atmosphere, Vegetation, and Ocean Observations)
►▼
Show Figures
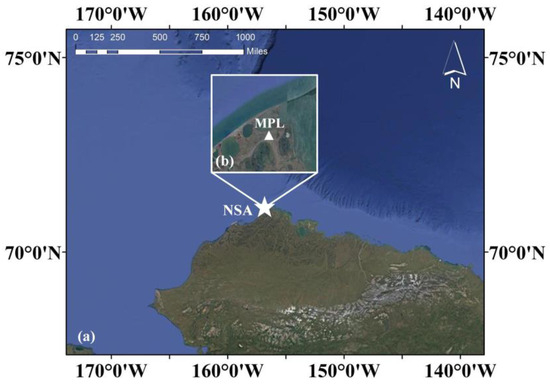
Figure 1
Open AccessArticle
Long-Term (2015–2024) Daily PM2.5 Estimation in China by Using XGBoost Combining Empirical Orthogonal Function Decomposition
by
Jiacheng Jiang, Jiaxin Dong, Yu Ding, Wenjia Ni, Jie Yang and Siwei Li
Remote Sens. 2025, 17(9), 1632; https://doi.org/10.3390/rs17091632 - 4 May 2025
Abstract
Fine particulate matter (PM2.5) has garnered significant scientific and public health concern owing to its capacity for deep penetration into the human respiratory system, presenting significant health risks. Despite the implementation of strict environmental policies in China over the past decade
[...] Read more.
Fine particulate matter (PM2.5) has garnered significant scientific and public health concern owing to its capacity for deep penetration into the human respiratory system, presenting significant health risks. Despite the implementation of strict environmental policies in China over the past decade to reduce PM2.5 levels, long-term public health concerns remain a serious issue. Our study aims to provide a high-quality, seamless daily PM2.5 dataset for China covering the years 2015 to 2024. A two-step PM2.5 estimation model is established based on a machine learning algorithm and a spatio-temporal decomposition method. First, we utilize the machine learning algorithm XGBoost (EXtreme Gradient Boosting) to address gaps in the daily MAIAC (Multi-Angle Implementation of Atmospheric Correction) AOD (Aerosol Optical Depth), with R2/RMSE (coefficient of determination/Root Mean Square Error) of 0.67/0.2678 compared to AERONET (Aerosol Robotic Network) AOD. Then, a novel approach by integrating XGBoost with EOF (Empirical Orthogonal Function) decomposition is introduced for PM2.5 estimation. The integration of EOF allows for the incorporation of entire meteorological field information into the PM2.5 estimation model, significantly enhancing its accuracy: spatial CV (cross-validation)-R2 improved from 0.8340 to 0.8935, and spatial CV-RMSE reduced from 13.8177 to 11.0668. Leveraging the newly produced dataset, we analyze the spatio-temporal variations of PM2.5 across China with EOF decomposition, particularly noting that PM2.5 levels in the eastern anthropogenic intensive regions continuously declined from 2015 to 2020, and fluctuated steadily during 2020–2024. This research underscores the critical need for sustained and effective air quality management strategies in China.
Full article
(This article belongs to the Special Issue Satellite Remote Sensing of Atmospheric Aerosols for Air Quality Applications (Second Edition))
►▼
Show Figures
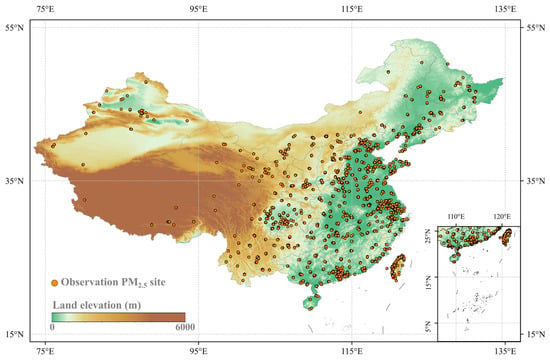
Figure 1
Open AccessArticle
Structural Similarity-Guided Siamese U-Net Model for Detecting Changes in Snow Water Equivalent
by
Karim Malik and Colin Robertson
Remote Sens. 2025, 17(9), 1631; https://doi.org/10.3390/rs17091631 - 4 May 2025
Abstract
Snow water equivalent (SWE), the amount of water generated when a snowpack melts, has been used to study the impacts of climate change on the cryosphere processes and snow cover dynamics during the winter season. In most analyses, high-temporal-resolution SWE and SD data
[...] Read more.
Snow water equivalent (SWE), the amount of water generated when a snowpack melts, has been used to study the impacts of climate change on the cryosphere processes and snow cover dynamics during the winter season. In most analyses, high-temporal-resolution SWE and SD data are aggregated into monthly and yearly averages to detect and characterize changes. Aggregating snow measurements, however, can magnify the modifiable aerial unit problem, resulting in differing snow trends at different temporal resolutions. Time series analysis of gridded SWE data holds the potential to unravel the impacts of climate change and global warming on daily, weekly, and monthly changes in snow during the winter season. Consequently, this research presents a high-temporal-resolution analysis of changes in the SWE across the cold regions of Canada. A Siamese UNet (Si-UNet) was developed by modifying the model’s last layer to incorporate the structural similarity (SSIM) index. The similarity values from the SSIM index are passed to a contrastive loss function, where the optimization process maximizes SSIM index values for pairs of similar SWE images and minimizes the values for pairs of dissimilar SWE images. A comparison of different model architectures, loss functions, and similarity metrics revealed that the SSIM index and the contrastive loss improved the Si-UNet’s accuracy by 16%. Using our Si-UNet, we found that interannual SWE declined steadily from 1979 to 2018, with March being the month in which the most significant changes occurred (R2 = 0.1, p-value < 0.05). We conclude with a discussion on the implications of the findings from our study of snow dynamics and climate variables using gridded SWE data, computer vision metrics, and fully convolutional deep neural networks.
Full article
(This article belongs to the Special Issue Recent Progress on Remote Sensing Change Detection and Application Driven by AI)
►▼
Show Figures
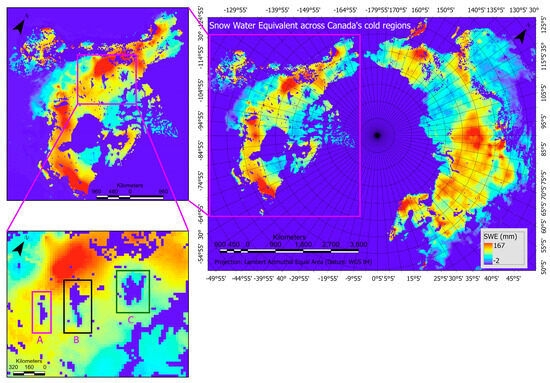
Figure 1
Open AccessArticle
Research on Monitoring Oceanic Precipitable Water Vapor and Short-Term Rainfall Forecasting Using Low-Cost Global Navigation Satellite System Buoy
by
Maosheng Zhou, Pengcheng Wang, Zelu Ji, Yunzhou Li, Dingfeng Yu, Zengzhou Hao, Min Li and Delu Pan
Remote Sens. 2025, 17(9), 1630; https://doi.org/10.3390/rs17091630 (registering DOI) - 4 May 2025
Abstract
This study utilizes a low-cost Global Navigation Satellite System (GNSS) buoy platform, combined with multi-system GNSS data, to investigate the impact of GNSS signal quality and multipath effects on the accuracy of atmospheric precipitable water vapor (PWV) retrievals. It also explores the methods
[...] Read more.
This study utilizes a low-cost Global Navigation Satellite System (GNSS) buoy platform, combined with multi-system GNSS data, to investigate the impact of GNSS signal quality and multipath effects on the accuracy of atmospheric precipitable water vapor (PWV) retrievals. It also explores the methods for oceanic rainfall event forecasting and precipitation prediction based on GNSS-PWV. By analyzing the data quality from various GNSS systems and using the European Centre for Medium-Range Weather Forecasts (ECMWF) ERA5 dataset as a reference, the study assesses the accuracy of PWV retrievals in dynamic marine environments. The results show that the GNSS-derived PWV from the buoy platform is highly consistent with ERA5 data in both trend and characteristics, with an RMSE of 3.8 mm for the difference between GNSS-derived PWV and ERA5 PWV. To enhance rainfall forecasting accuracy, a balanced threshold selection (BTS) method is proposed, significantly improving the balance between the probability of detection (POD) and false alarm rate (FAR). Furthermore, a Random Forest model based on multiple meteorological parameters optimizes precipitation forecasting, especially in reducing false alarms. Additionally, a particle swarm optimization (PSO)-based BP Neural Network model for rainfall prediction achieves high precision, with an R2 of 97.8%, an average absolute error of 0.08 mm, and an RMSE of 0.1 mm. The findings demonstrate the potential of low-cost GNSS buoy for monitoring atmospheric water vapor and short-term rainfall forecasting in dynamic marine environments.
Full article
(This article belongs to the Special Issue Remote Sensing in Space Geodesy and Cartography Methods (Third Edition))
►▼
Show Figures
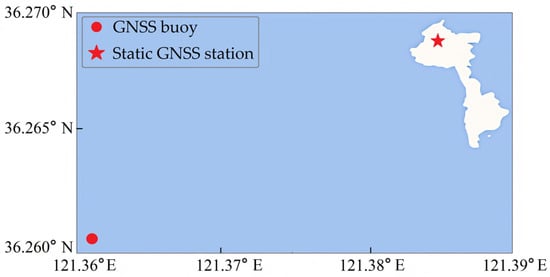
Figure 1
Open AccessArticle
Analysis of Ionospheric Disturbances in China During the December 2023 Geomagnetic Storm Using Multi-Instrument Data
by
Jun Tang, Sheng Wang, Jintao Wang, Mingxian Hu and Chaoqian Xu
Remote Sens. 2025, 17(9), 1629; https://doi.org/10.3390/rs17091629 - 4 May 2025
Abstract
This study investigates the ionospheric response over China during the geomagnetic storm that occurred on 1–2 December 2023. The data used include GPS measurements from the Crustal Movement Observation Network of China, BDS-GEO satellite data from IGS MEGX stations, [O]/[N2] ratio
[...] Read more.
This study investigates the ionospheric response over China during the geomagnetic storm that occurred on 1–2 December 2023. The data used include GPS measurements from the Crustal Movement Observation Network of China, BDS-GEO satellite data from IGS MEGX stations, [O]/[N2] ratio information obtained by the TIMED/GUVI, and electron density (Ne) observations from Swarm satellites. The Prophet time series forecasting model is employed to detect ionospheric anomalies. VTEC variations reveal significant daytime increases in GNSS stations such as GAMG, URUM, and CMUM after the onset of the geomagnetic storm on 1 December, indicating a dayside positive ionospheric response primarily driven by prompt penetration electric fields (PPEF). In contrast, the stations JFNG and CKSV show negative responses, reflecting regional differences. The [O]/[N2] ratio increased significantly in the southern region between 25°N and 40°N, suggesting that atmospheric gravity waves (AGWs) induced thermospheric compositional changes, which played a crucial role in the ionospheric disturbances. Ne observations from Swarm A and C satellites further confirmed that the intense ionospheric perturbations were consistent with changes in VTEC and [O]/[N2], indicating the medium-scale traveling ionospheric disturbance was driven by atmospheric gravity waves. Precise point positioning (PPP) analysis reveals that ionospheric variations during the geomagnetic storm significantly impact GNSS positioning precision, with various effects across different stations. Station GAMG experienced disturbances in the U direction (vertical positioning error) at the onset of the storm but quickly stabilized; station JFNG showed significant fluctuations in the U direction around 13:00 UT; and station CKSV experienced similar fluctuations during the same period; station CMUM suffered minor disturbances in the U direction; while station URUM maintained relatively stable positioning throughout the storm, corresponding to steady VTEC variations. These findings demonstrate the substantial impact of ionospheric disturbances on GNSS positioning accuracy in southern and central China during the geomagnetic storm. This study reveals the complex and dynamic processes of ionospheric disturbances over China during the 1–2 December 2023 storm, highlighting the importance of ionospheric monitoring and high-precision positioning corrections during geomagnetic storms. The results provide scientific implications for improving GNSS positioning stability in mid- and low-latitude regions.
Full article
(This article belongs to the Special Issue BDS/GNSS for Earth Observation: Part II)
►▼
Show Figures
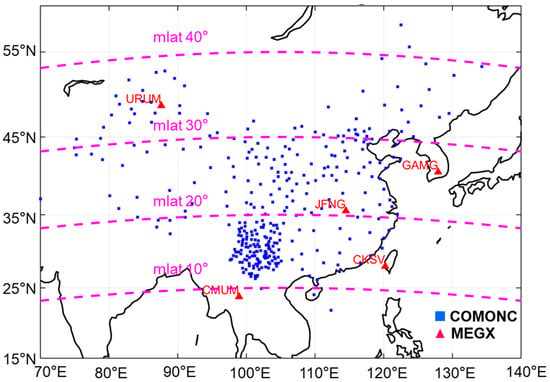
Figure 1
Open AccessArticle
Study on Class Imbalance in Land Use Classification for Soil Erosion in Dry–Hot Valley Regions
by
Yuzhuang Deng, Guokun Chen, Bohui Tang, Xingwu Duan, Lijun Zuo and Haijuan Zhao
Remote Sens. 2025, 17(9), 1628; https://doi.org/10.3390/rs17091628 - 4 May 2025
Abstract
The inherent spatial heterogeneity of land types often leads to a class imbalance in remote sensing-based classification, reducing the accuracy of minority class detection. Consequently, current land use datasets are often inadequate for the specific needs of soil erosion studies. In response to
[...] Read more.
The inherent spatial heterogeneity of land types often leads to a class imbalance in remote sensing-based classification, reducing the accuracy of minority class detection. Consequently, current land use datasets are often inadequate for the specific needs of soil erosion studies. In response to the need for soil conservation in dry–hot valley regions, this study integrated multi-source remote sensing imagery and constructed three high-precision imbalanced sample datasets on the Google Earth Engine (GEE) platform to perform land use classification. The degree of class imbalance was quantified using the imbalance ratio (IR), and the impact of sample imbalance on the classification accuracy of different land use types in a typical dry–hot valley was analyzed. The results show that (1) Feature selection significantly improved both classification accuracy and computational efficiency. The period from February to April each year, between 2018 and 2023, was identified as the optimal time window for land use classification in dry–hot valleys. (2) Constructing composite images over longer time scales enhanced classification performance: using a 2020 annual composite image combined with a Gradient Tree Boosting classifier yielded the highest accuracy, indicating that longer temporal synthesis improves classification results. (3) The effect of class imbalance on classification accuracy varied by land type: woodland (the majority class) was least affected by imbalance, whereas minority classes such as cultivated land, garden plantations, and grassland were highly sensitive to imbalance. In imbalanced scenarios, minority classes are prone to omission errors, leading to notable accuracy declines; producer’s accuracy (PA) decreased by 46%, 42%, and 25% for cultivated land, garden plantations, and grassland, respectively, as IR increased (with PA dropping faster than user’s accuracy, UA). Cultivated land was especially sensitive and frequently overlooked under high imbalance conditions compared to gardens and grasslands. Despite overall accuracy improving with higher IR, the accuracy of these minority classes dropped significantly, underscoring the importance of addressing the class imbalance in land use classification for erosion-prone areas.
Full article
(This article belongs to the Special Issue Remote Sensing Measurements of Land Use and Land Cover)
►▼
Show Figures
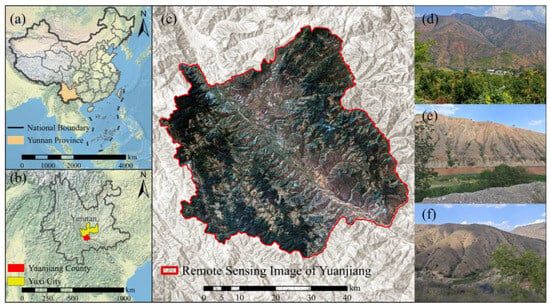
Figure 1
Open AccessArticle
Forecasting Cumulonimbus Clouds: Evaluation of New Operational Convective Index Using Lightning and Precipitation Data
by
Margarida Belo-Pereira
Remote Sens. 2025, 17(9), 1627; https://doi.org/10.3390/rs17091627 - 3 May 2025
Abstract
Deep convective clouds, such as towering cumulus and Cumulonimbus, can endanger lives and property, also being a major hazard to aviation. This study presents the convective index (IndexCON) used operationally at the Portuguese Meteorological Watch Office. Moreover, IndexCON is evaluated against
[...] Read more.
Deep convective clouds, such as towering cumulus and Cumulonimbus, can endanger lives and property, also being a major hazard to aviation. This study presents the convective index (IndexCON) used operationally at the Portuguese Meteorological Watch Office. Moreover, IndexCON is evaluated against lightning and precipitation data for two years, between January 2022 and December 2023, over mainland Portugal and its surrounding areas. This index combines several European Center for Medium-Range Weather Forecasts (ECMWF) prognostic variables, such as stability indices, cloud water content, relative humidity and vertical velocity, using a fuzzy-logic approach. IndexCON performs well in the warm season (May–October), with a probability of detection (POD) of 70%, a false alarm ratio (FAR) of 30% and a probability of false detection (POFD) less than 5%, leading to a Critical Success Index (CSI) above 0.55. However, IndexCON performs worse in the cold season (November–April), when dynamical drivers are more relevant, mainly due to overestimating the convective activity, resulting in CSI and Heidke Skill Score (HSS) values below 0.3. Optimizing the membership functions partially reduces this overestimation. Finally, the added value of IndexCON was illustrated in detail for a thunderstorm episode, using satellite products, lightning and precipitation data.
Full article
(This article belongs to the Special Issue Cloud Remote Sensing: Current Status and Perspective)
►▼
Show Figures
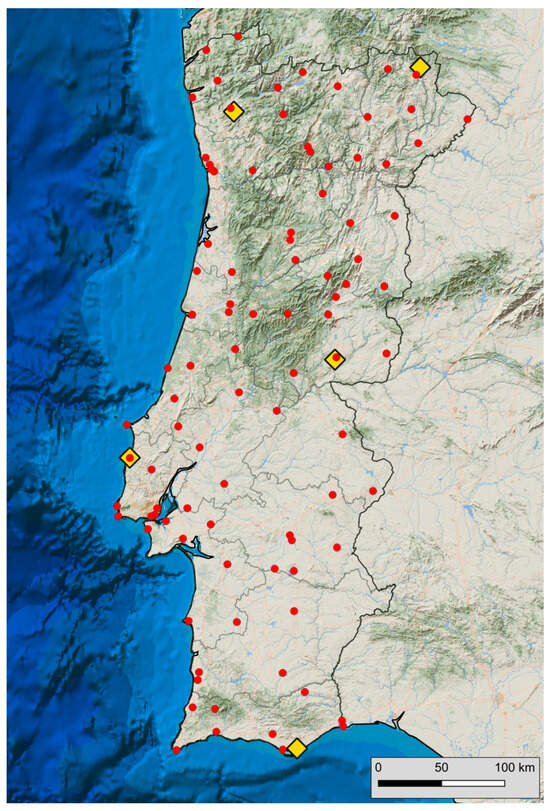
Figure 1
Open AccessArticle
One-Dimensional Convolutional Neural Network for Automated Kimchi Cabbage Downy Mildew Detection Using Aerial Hyperspectral Images
by
Yang Lyu, Lukas Wiku Kuswidiyanto, Pingan Wang, Hyun-Ho Noh, Hee-Young Jung and Xiongzhe Han
Remote Sens. 2025, 17(9), 1626; https://doi.org/10.3390/rs17091626 - 3 May 2025
Abstract
Downy mildew poses a significant threat to kimchi cabbage, a vital agricultural product in Korea, adversely affecting its yield and quality. Traditional disease detection methods based on visual inspection are labor intensive and time consuming. This study proposes a non-destructive, field-scale disease detection
[...] Read more.
Downy mildew poses a significant threat to kimchi cabbage, a vital agricultural product in Korea, adversely affecting its yield and quality. Traditional disease detection methods based on visual inspection are labor intensive and time consuming. This study proposes a non-destructive, field-scale disease detection approach using unmanned aerial vehicle (UAV)-based hyperspectral imaging. Hyperspectral images of the kimchi cabbage field were preprocessed, segmented at the pixel level, and classified into four categories: background, healthy, early-stage disease, and late-stage disease. Spectral analysis of the late and early stages of downy mildew infection revealed notable differences in the red-edge band, with infected plants exhibiting increased red-edge reflectance. To automate disease detection, various machine learning models, including Random Forest (RF), 1D Convolutional Neural Network (1D-CNN), 1D Residual Network (1D-ResNet), and 1D Inception Network (1D-InceptionNet), were developed. These models were trained based on a 0.2 sampling dataset, achieving overall accuracy scores of 0.907, 0.901, 0.909, and 0.914, along with F1 scores of 0.876, 0.845, 0.897, and 0.899, respectively. Overall, the results of this study revealed that the red-edge band reliably signaled the presence of downy mildew, and the 1D-InceptionNet model demonstrated the most effective performance for automatic disease detection.
Full article
(This article belongs to the Special Issue Proximal and Remote Sensing for Precision Crop Management II)
►▼
Show Figures
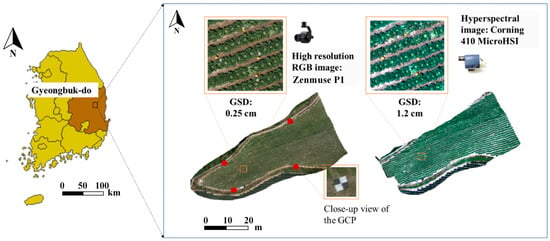
Figure 1
Open AccessArticle
Specific Responses to Environmental Factors Cause Discrepancy in the Link Between Solar-Induced Chlorophyll Fluorescence and Transpiration in Three Plantations
by
Meijun Hu, Shoujia Sun, Xiangfen Cheng, Qingmei Pan, Jinsong Zhang, Xin Wang, Chongfan Guan, Zhipeng Li and Xiang Gao
Remote Sens. 2025, 17(9), 1625; https://doi.org/10.3390/rs17091625 - 3 May 2025
Abstract
Vegetation transpiration (Tr) is crucial for the water cycle, regional water balance, and plant growth but remains challenging to estimate at large scales. Sun-induced chlorophyll fluorescence (SIF) provides a novel method for estimating Tr, but its effectiveness is limited by species specificity, requiring
[...] Read more.
Vegetation transpiration (Tr) is crucial for the water cycle, regional water balance, and plant growth but remains challenging to estimate at large scales. Sun-induced chlorophyll fluorescence (SIF) provides a novel method for estimating Tr, but its effectiveness is limited by species specificity, requiring continuous tower-based observations for comprehensive analysis across diverse ecosystems. In this study, SIF and Tr were simultaneously monitored in Chinese cork oak (ring-porous), poplar (diffuse-porous), and arborvitae (non-porous) plantations in northern China, and the SIF–Tr relationship was further analyzed. The results showed that SIF and Tr shared similar diurnal dynamics, although Tr exhibited midday saturation. SIF and Tr were closely correlated, and the correlation strengthened as the temporal scale aggregated. Environmental factors had nonlinear impacts on SIF and Tr. Therefore, the SIF–Tr relationship deteriorated to some extent at midday, with short-term stress reducing the correlation by 0.1–0.23. Compared to the linear empirical model, the inclusion of environmental factors improved the accuracy of SIF-based Tr estimation, increasing the R2 value by 0.12 to 0.37. At the same level of accuracy, the number of environmental variables required was higher at the half-hour scale than at the daily scale. This study demonstrated the species-specific influence of environmental factors on SIF and Tr in different plantations, enhanced the understanding of the SIF–Tr relationship, and provided theoretical and data support for future large-scale Tr predictions using satellite-based SIF.
Full article
Open AccessArticle
The Response Mechanism of Ecosystem Service Trade-Offs Along an Aridity Gradient in Humid and Semi-Humid Regions: A Case Study of Northeast China
by
Yuetong Liu, Zhen Zhen and Yinghui Zhao
Remote Sens. 2025, 17(9), 1624; https://doi.org/10.3390/rs17091624 - 3 May 2025
Abstract
►▼
Show Figures
In the context of global climate change, the sensitivity of ecosystem services (ESs) and their relationships to aridity are increasing, and the areas affected by aridity continue to expand. Previous research has largely focused on ES changes in arid regions under intensified aridity
[...] Read more.
In the context of global climate change, the sensitivity of ecosystem services (ESs) and their relationships to aridity are increasing, and the areas affected by aridity continue to expand. Previous research has largely focused on ES changes in arid regions under intensified aridity conditions and has overlooked humid and semi-humid regions, which are more sensitive to water loss. In this study, we applied a generalized additive model (GAM) to analyze the nonlinear responses of ES trade-offs to aridity in humid and semi-humid regions. The results indicated that at an aridity index (AI) of approximately 0.26, the trade-off intensity of two ES pairs—carbon sequestration vs. water yield and habitat quality vs. water yield—reached a peak. Additionally, structural equation modeling (SEM) based on threshold partitioning revealed significant shifts in ES trade-off drivers below and above the AI threshold. In areas with AI < 0.26, worsening meteorological and soil conditions exacerbated ES trade-offs, whereas in areas with AI > 0.26, competition for water resources between human activities and vegetation became the primary driver of intensified trade-offs. These findings highlight the need for targeted management strategies to maintain ecosystem stability in humid and semi-humid regions and provide valuable guidance for responding to increasing aridity risks.
Full article
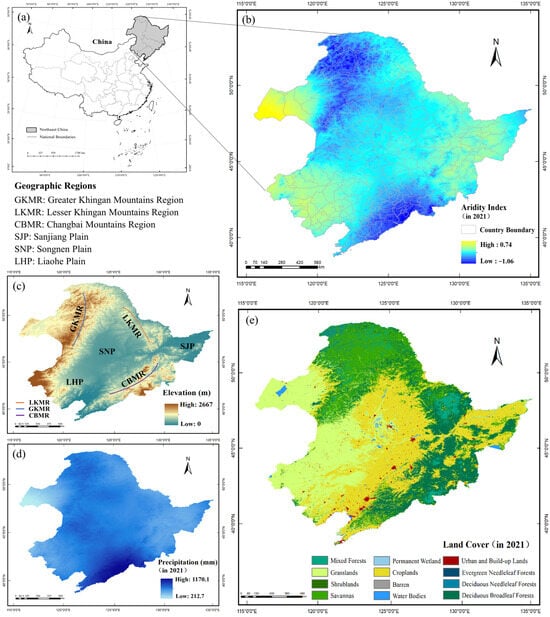
Figure 1
Open AccessArticle
Triple Graph Convolutional Network for Hyperspectral Image Feature Fusion and Classification
by
Maryam Imani and Daniele Cerra
Remote Sens. 2025, 17(9), 1623; https://doi.org/10.3390/rs17091623 - 3 May 2025
Abstract
Most graph-based networks utilize superpixel generation methods as a preprocessing step, considering superpixels as graph nodes. In the case of hyperspectral images having high variability in spectral features, considering an image region as a graph node may degrade the class discrimination ability of
[...] Read more.
Most graph-based networks utilize superpixel generation methods as a preprocessing step, considering superpixels as graph nodes. In the case of hyperspectral images having high variability in spectral features, considering an image region as a graph node may degrade the class discrimination ability of networks for pixel-based classification. Moreover, most graph-based networks focus on global feature extraction, while both local and global information are important for pixel-based classification. To deal with these challenges, superpixel-based graphs are overruled in this work, and a Graph-based Feature Fusion (GF2) method relying on three different graphs is proposed instead. A local patch is considered around each pixel under test, and at the same time, global anchors with the highest informational content are selected from the entire scene. While the first graph explores relationships between neighboring pixels in the local patch and the global anchors, the second and third graphs use the global anchors and pixels of the local patch as nodes, respectively. These graphs are processed using graph convolutional networks, and their results are fused using a cross-attention mechanism. The experiments on three hyperspectral benchmark datasets show that the GF2 network has high classification performance compared to state-of-the-art methods, while imposing a reasonable number of learnable parameters.
Full article
(This article belongs to the Special Issue Machine Learning Approaches for Semantic and Instance Segmentation in Remote Sensing)
►▼
Show Figures
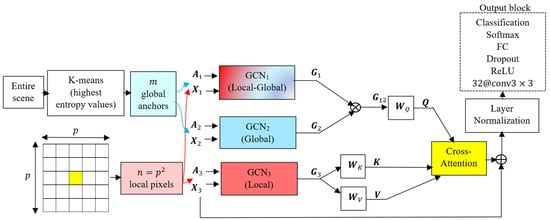
Figure 1
Open AccessArticle
SFRADNet: Object Detection Network with Angle Fine-Tuning Under Feature Matching
by
Keliang Liu, Yantao Xi, Donglin Jing, Xue Zhang and Mingfei Xu
Remote Sens. 2025, 17(9), 1622; https://doi.org/10.3390/rs17091622 - 2 May 2025
Abstract
Due to the distant acquisition and bird’s-eye perspective of remote sensing images, ground objects are distributed in arbitrary scales and multiple orientations. Existing detectors often utilize feature pyramid networks (FPN) and deformable (or rotated) convolutions to adapt to variations in object scale and
[...] Read more.
Due to the distant acquisition and bird’s-eye perspective of remote sensing images, ground objects are distributed in arbitrary scales and multiple orientations. Existing detectors often utilize feature pyramid networks (FPN) and deformable (or rotated) convolutions to adapt to variations in object scale and orientation. However, these methods solve scale and orientation issues separately and ignore their deeper coupling relationships. When the scale features extracted by the network are significantly mismatched with the object, it is difficult for the detection head to effectively capture orientation of object, resulting in misalignment between object and bounding box. Therefore, we propose a one-stage detector—Scale First Refinement-Angle Detection Network (SFRADNet), which aims to fine-tune the rotation angle under precise scale feature matching. We introduce the Group Learning Large Kernel Network (GL2KNet) as the backbone of SFRADNet and employ a Shape-Aware Spatial Feature Extraction Module (SA-SFEM) as the primary component of the detection head. Specifically, within GL2KNet, we construct diverse receptive fields with varying dilation rates to capture features across different spatial coverage ranges. Building on this, we utilize multi-scale features within the layers and apply weighted aggregation based on a Scale Selection Matrix (SSMatrix). The SSMatrix dynamically adjusts the receptive field coverage according to the target size, enabling more refined selection of scale features. Based on precise scale features captured, we first design a Directed Guiding Box (DGBox) within the SA-SFEM, using its shape and position information to supervise the sampling points of the convolution kernels, thereby fitting them to deformations of object. This facilitates the extraction of orientation features near the object region, allowing for accurate refinement of both scale and orientation. Experiments show that our network achieves a mAP of 80.10% on the DOTA-v1.0 dataset, while reducing computational complexity compared to the baseline model.
Full article
(This article belongs to the Special Issue Remote Sensing of Target Object Detection and Identification (Third Edition))
►▼
Show Figures
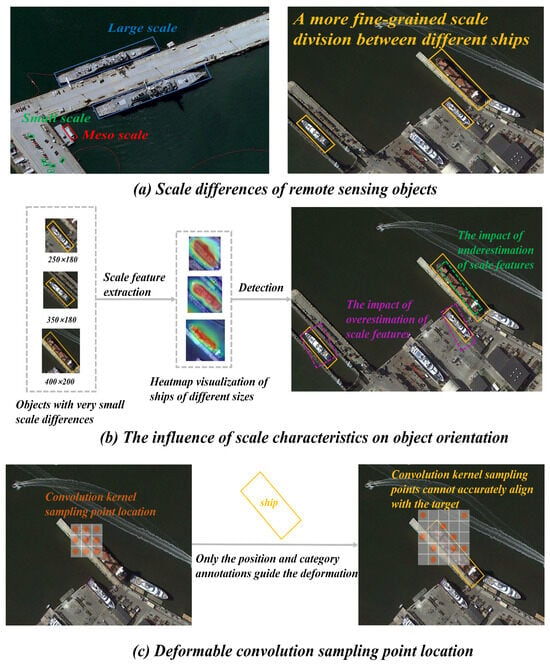
Figure 1
Open AccessArticle
Performance Evaluation of Inherent Optical Property Algorithms and Identification of Potential Water Quality Indicators Using GCOM-C Data in Eutrophic Lake Kasumigaura, Japan
by
Misganaw Choto, Hiroto Higa, Salem Ibrahim Salem, Eko Siswanto, Takayuki Suzuki and Martin Mäll
Remote Sens. 2025, 17(9), 1621; https://doi.org/10.3390/rs17091621 - 2 May 2025
Abstract
Lake Kasumigaura, one of Japan’s largest lakes, presents significant challenges for remote sensing due to its eutrophic conditions and complex optical properties. Although the Global Change Observation Mission-Climate (GCOM-C)/Second-generation Global Imager (SGLI)-derived inherent optical properties (IOPs) offer water quality monitoring potential, their performance
[...] Read more.
Lake Kasumigaura, one of Japan’s largest lakes, presents significant challenges for remote sensing due to its eutrophic conditions and complex optical properties. Although the Global Change Observation Mission-Climate (GCOM-C)/Second-generation Global Imager (SGLI)-derived inherent optical properties (IOPs) offer water quality monitoring potential, their performance in such turbid inland waters remains inadequately validated. This study evaluated five established IOP retrieval algorithms, including the quasi-analytical algorithm (QAA_V6), Garver–Siegel–Maritorena (GSM), generalized IOP (GIOP-DC), Plymouth Marine Laboratory (PML), and linear matrix inversion (LMI), using measured remote sensing reflectance (Rrs) and corresponding IOPs between 2017–2018. The results demonstrated that the QAA had the highest performance for retrieving absorption of particles (ap) with a Pearson correlation (r) = 0.98, phytoplankton (aph) with r = 0.97, and non-algal particles (anap) with r = 0.85. In contrast, the GSM algorithm exhibited the best accuracy for estimating absorption by colored dissolved organic matter (aCDOM), with r = 0.87, along with the lowest mean absolute percentage error (MAPE) and root mean square error (RMSE). Additionally, a strong correlation (r = 0.81) was observed between SGLI satellite-derived remote-sensing reflectance (Rrs) and in situ measurements. Notably, a high correlation was observed between the aph (443 nm) and the chlorophyll a (Chl-a) concentration (r = 0.84), as well as between the backscattering coefficient (bbp) at 443 nm and inorganic suspended solids (r = 0.64), confirming that IOPs are reliable water quality assessment indicators. Furthermore, the use of IOPs as variables for estimating water quality parameters such as Chl-a and suspended solids showed better performance compared to empirical methods.
Full article
(This article belongs to the Special Issue Remote Sensing Band Ratios for the Assessment of Water Quality)
►▼
Show Figures
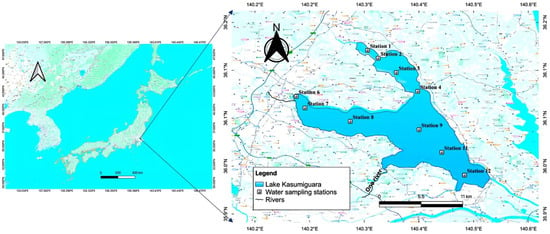
Figure 1
Open AccessArticle
Explainability Feature Bands Adaptive Selection for Hyperspectral Image Classification
by
Jirui Liu, Jinhui Lan, Yiliang Zeng, Wei Luo, Zhixuan Zhuang and Jinlin Zou
Remote Sens. 2025, 17(9), 1620; https://doi.org/10.3390/rs17091620 - 2 May 2025
Abstract
Hyperspectral remote sensing images are widely used in resource exploration, urban planning, natural disaster assessment, and feature classification. Aiming at the problems of poor interpretability of feature classification algorithms for hyperspectral images, multiple feature dimensions, and difficulty in effectively improving classification accuracy, this
[...] Read more.
Hyperspectral remote sensing images are widely used in resource exploration, urban planning, natural disaster assessment, and feature classification. Aiming at the problems of poor interpretability of feature classification algorithms for hyperspectral images, multiple feature dimensions, and difficulty in effectively improving classification accuracy, this paper proposes a feature band adaptive selection method for hyperspectral images. The proposed feature band adaptive selection model focuses on the joint salient feature regions of the hyperspectral image, visualizes the feature contribution of the bands, more intuitively reveals the selection basis of the feature bands of the hyperspectral features in the process of deep learning, and selects the feature bands with high contribution to carry out classification experiments for verification. Quantitative evaluations on four hyperspectral benchmarks (Pavia University/Centre, Washington DC, GF-5) demonstrate that EFBASN achieves state-of-the-art classification accuracy, with an overall accuracy (OA) of 97.68% on Pavia U, surpassing 12 recent methods including SSCFA (94.48%) and CNCMN (93.12%). Crucially, the attention weights of critical bands (e.g., Band 26 at 672 nm for iron oxide detection) are 3.2 times higher than redundant bands, providing physically interpretable selection criteria.
Full article
(This article belongs to the Special Issue Advances of Remote Sensing in Land Cover and Land Use Mapping)
►▼
Show Figures
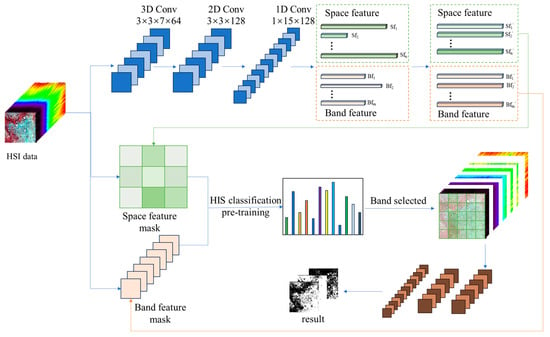
Figure 1
Open AccessArticle
Optimization Simulation and Comprehensive Evaluation Coupled with CNN-LSTM and PLUS for Multi-Scenario Land Use in Cultivated Land Reserve Resource Area
by
Shaner Li, Chao Zhang, Chang Chen, Cuicui Yang, Lihua Zhao and Xuechuan Bai
Remote Sens. 2025, 17(9), 1619; https://doi.org/10.3390/rs17091619 - 2 May 2025
Abstract
The scientific development and utilization of cultivated land reserve resource areas is an important basis for realizing national food security and regional ecological protection. This paper focuses on land use optimization simulations to explore the paths of sustainable land use in cultivated land
[...] Read more.
The scientific development and utilization of cultivated land reserve resource areas is an important basis for realizing national food security and regional ecological protection. This paper focuses on land use optimization simulations to explore the paths of sustainable land use in cultivated land reserve resources areas. Deep learning technology was introduced to calculate the growth probability of each land use type. A land use change simulation method coupling CNN-LSTM and PLUS was constructed to dynamically simulate the land use pattern, and the spatial accuracy of the simulation was improved. Markov chains and multi-objective planning (MOP) model were used to set historical development (HD) scenarios, ecological conservation (EP) scenarios, land consolidation (LC) scenarios, and sustainable development (SD) scenarios. The comprehensive impact of land use change on ecosystem service value (ESV), agricultural production benefits (APBs), and carbon balance (CB) was evaluated by systematically analyzing the quantitative and spatial distribution characteristics of land use change in different scenarios from 2020 to 2030. Da’an City, Jilin province, China was selected as the study area. The results of this study show the following: (1) The CNN-LSTM coupled with the PLUS model was designed to capture the dynamic change characteristics of land use, which achieves high accuracy (Kappa of 0.8119). (2) In the EP scenario, the increase in ESV was 4.36%, but the increase in APB was only 7.33%. In the LC scenario, APB increased by 22.11%, while ESV decreased by 3.44%. In the SD scenario, a dynamic balance was achieved between ESV and APB, and it was the optimal path for sustainable development. (3) The SD scenario performed best, with a CB of 5,532,100 tons, while the EP scenario was the lowest, at only 1,493,500 tons. The SD scenario shows the optimal potential of combining carbon reduction and agricultural development. In this paper, deep learning and spatial modeling for multi-scenario simulation were integrated, and a scientific basis for the planning and management of cultivated land reserve resource areas was provided.
Full article
(This article belongs to the Special Issue Remote Sensing and Associated Artificial Intelligence in Agricultural Applications (2nd Edition))
►▼
Show Figures
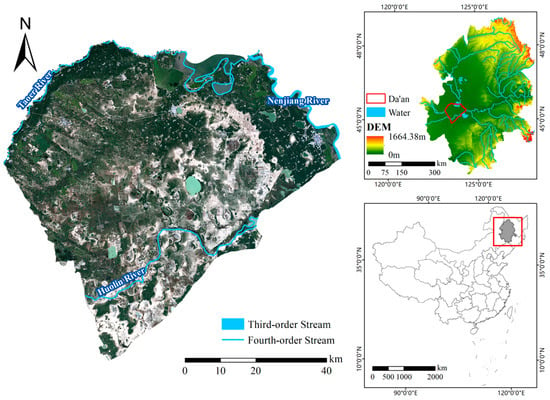
Figure 1
Open AccessArticle
Modeling Dynamics of Water Balance for Lakes in the Northwest Tibetan Plateau with Satellite-Based Observations
by
Jiaheng Yan, Yanhong Wu, Yongkang Ren, Siqi Zheng, Hao Chen and Xuankai Teng
Remote Sens. 2025, 17(9), 1618; https://doi.org/10.3390/rs17091618 - 2 May 2025
Abstract
The hydrological cycle in the Tibetan Plateau is experiencing notable changes in recent decades under a changing climate. The hydrological changes, however, are not well investigated due to the limitations in the availability of ground-based observations. In this study, by incorporating satellite-based observations
[...] Read more.
The hydrological cycle in the Tibetan Plateau is experiencing notable changes in recent decades under a changing climate. The hydrological changes, however, are not well investigated due to the limitations in the availability of ground-based observations. In this study, by incorporating satellite-based observations into a hydrological modeling framework, seasonal and inter-annual dynamics of water balance for lakes in the northwest Tibetan Plateau are examined systematically for the period of 1990 to 2022. Satellite-based observations, including lake water area and water level, have been used to calibrate the hydrological model and to estimate lake water storage. The hydrological model performs satisfactorily, with the Nash–Sutcliffe efficiency coefficient (NSE) exceeding 0.5 for all 15 studied lakes. It is found that inflow contributes over 70% of annual water gain for most lakes, while percolation accounts for a larger portion (>60%) of total water loss than evaporation. The studied lakes have expanded substantially, with regional average increasing rates in lake level and water storage of 0.38 m/a and 3.12 × 108 m3/a, respectively. Some lakes transitioned from shrinking to expanding around 1999, and expansion in most lakes has further accelerated since around 2012, primarily because of increased precipitation over the lake catchments, leading to greater inflow to the lakes. These findings provide important insights into understanding and predicting responses of lake water balance to climate change as well as for developing adaptative strategies.
Full article
(This article belongs to the Special Issue Hydrological Modelling Based on Satellite Observations)
►▼
Show Figures
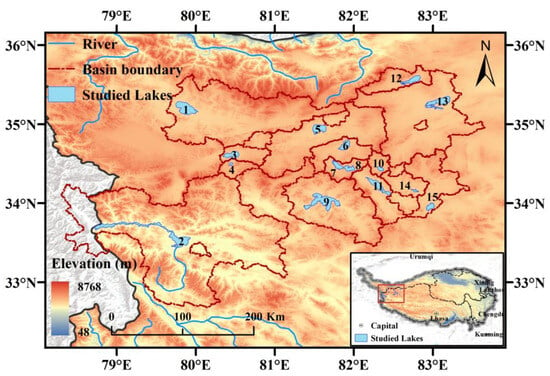
Figure 1
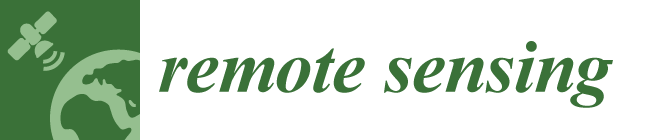
Journal Menu
► ▼ Journal Menu-
- Remote Sensing Home
- Aims & Scope
- Editorial Board
- Reviewer Board
- Topical Advisory Panel
- Photography Exhibition
- Instructions for Authors
- Special Issues
- Topics
- Sections & Collections
- Article Processing Charge
- Indexing & Archiving
- Editor’s Choice Articles
- Most Cited & Viewed
- Journal Statistics
- Journal History
- Journal Awards
- Society Collaborations
- Conferences
- Editorial Office
Journal Browser
► ▼ Journal BrowserHighly Accessed Articles
Latest Books
E-Mail Alert
News
Topics
Topic in
Applied Sciences, Inventions, JMSE, Oceans, Remote Sensing, Sensors
Ship Dynamics, Stability and Safety
Topic Editors: Zaojian Zou, Weilin LuoDeadline: 20 May 2025
Topic in
Sustainability, Buildings, Sensors, Remote Sensing, Land, Climate, Atmosphere
Advances in Low-Carbon, Climate-Resilient, and Sustainable Built Environment
Topic Editors: Baojie He, Stephen Siu Yu Lau, Deshun Zhang, Andreas Matzarakis, Fei GuoDeadline: 31 May 2025
Topic in
Earth, GeoHazards, IJGI, Land, Remote Sensing, Smart Cities, Infrastructures, Automation
Machine Learning and Big Data Analytics for Natural Disaster Reduction and Resilience
Topic Editors: Isam Shahrour, Marwan Alheib, Anna Brdulak, Fadi Comair, Carlo Giglio, Xiongyao Xie, Yasin Fahjan, Salah ZidiDeadline: 30 June 2025
Topic in
Agronomy, Climate, Earth, Remote Sensing, Water
Advances in Crop Simulation Modelling
Topic Editors: Mavromatis Theodoros, Thomas Alexandridis, Vassilis AschonitisDeadline: 15 July 2025

Conferences
Special Issues
Special Issue in
Remote Sensing
Quantitative Inversion and Validation of Satellite Remote Sensing Products
Guest Editors: Xingfa Gu, Jian Yang, Xiangqin Wei, Hailiang Gao, Fernando CamachoDeadline: 10 May 2025
Special Issue in
Remote Sensing
Oceanographic Lidar in the Study of Marine Systems
Guest Editors: Peng Chen, Cédric Jamet, Junwu Tang, Martin A. MontesDeadline: 10 May 2025
Special Issue in
Remote Sensing
SAR for Forest Mapping III
Guest Editors: Michele Martone, Armando MarinoDeadline: 10 May 2025
Special Issue in
Remote Sensing
Advances in High-Resolution Satellite Remote Sensing Image Processing and Classification
Guest Editors: Yingdong Pi, Wenli Huang, Qingwei Zhuang, Arnab MuhuriDeadline: 10 May 2025
Topical Collections
Topical Collection in
Remote Sensing
Feature Paper Special Issue on Forest Remote Sensing
Collection Editors: Zengyuan Li, Erxue Chen, Lin Cao
Topical Collection in
Remote Sensing
Google Earth Engine Applications
Collection Editors: Lalit Kumar, Onisimo Mutanga
Topical Collection in
Remote Sensing
The VIIRS Collection: Calibration, Validation, and Application
Collection Editors: Xi Shao, Xiaoxiong Xiong, Changyong Cao
Topical Collection in
Remote Sensing
Current, Planned, and Future Satellite Missions: Guidelines for Data Exploitation by the Remote Sensing Community
Collection Editors: Jose Moreno, Magaly Koch, Robert Wang