Journal Description
Processes
- Open Access— free for readers, with article processing charges (APC) paid by authors or their institutions.
- High Visibility: indexed within Scopus, SCIE (Web of Science), Ei Compendex, Inspec, AGRIS, and other databases.
- Journal Rank: JCR - Q2 (Engineering, Chemical) / CiteScore - Q2 (Chemical Engineering (miscellaneous))
- Rapid Publication: manuscripts are peer-reviewed and a first decision is provided to authors approximately 14.9 days after submission; acceptance to publication is undertaken in 2.7 days (median values for papers published in this journal in the second half of 2024).
- Recognition of Reviewers: reviewers who provide timely, thorough peer-review reports receive vouchers entitling them to a discount on the APC of their next publication in any MDPI journal, in appreciation of the work done.
Latest Articles
E-Mail Alert
News
MDPI INSIGHTS: The CEO's Letter #21 - Annual Report, Swiss Consortium, IWD, ICARS, Serbia
Meet Us at the XVI International Mineral Processing and Recycling Conference, 28–30 May 2025, Belgrade, Serbia
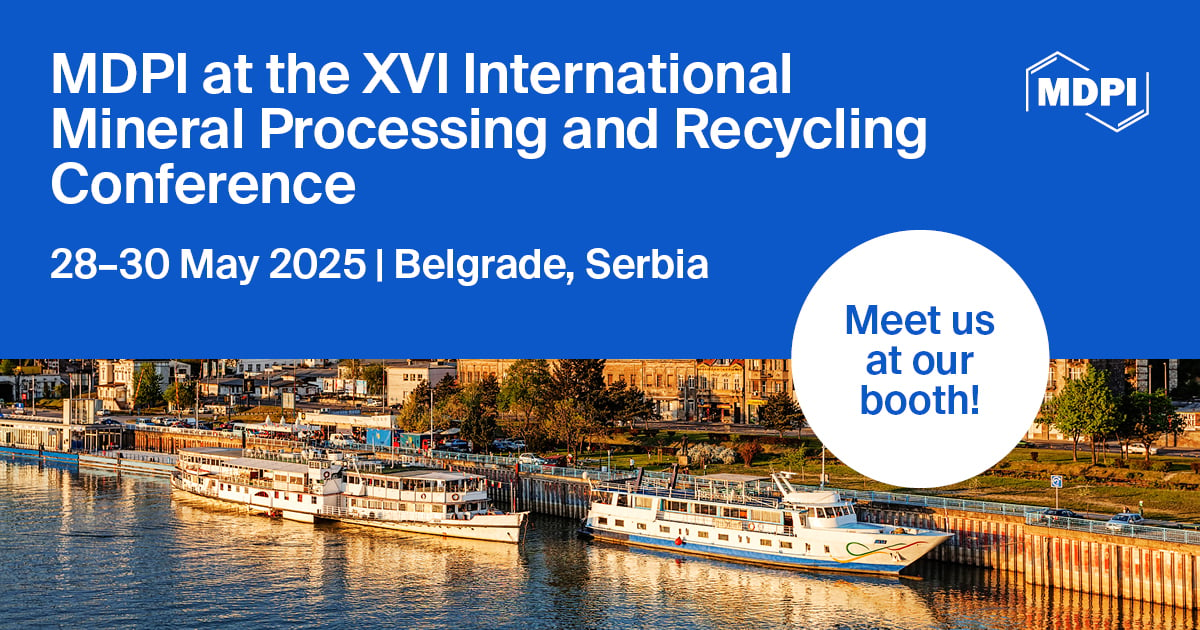
Topics
Deadline: 15 April 2025
Deadline: 30 April 2025
Deadline: 31 May 2025
Deadline: 9 June 2025
Conferences
Special Issues
Deadline: 5 April 2025
Deadline: 5 April 2025
Deadline: 10 April 2025
Deadline: 10 April 2025