-
Application of Real-Life On-Road Driving Data for Simulating the Electrification of Long-Haul Transport Trucks
-
Predicting the Torque Demand of a Battery Electric Vehicle for Real-World Driving Maneuvers Using the NARX Technique
-
Comparison of EV Fast Charging Protocols and Impact of Sinusoidal Half-Wave Fast Charging Methods on Lithium-Ion Cells
-
Simulation-Based Assessment of Energy Consumption of Alternative Powertrains in Agricultural Tractors
Journal Description
World Electric Vehicle Journal
World Electric Vehicle Journal
is the first peer-reviewed, international, scientific journal that comprehensively covers all studies related to battery, hybrid, and fuel cell electric vehicles. The journal is owned by the World Electric Vehicle Association (WEVA) and its members, the European Association for e-Mobility (AVERE), Electric Drive Transportation Association (EDTA), and Electric Vehicle Association of Asia Pacific (EVAAP). It has been published monthly online by MDPI since Volume 9, Issue 1 (2018).
- Open Access— free for readers, with article processing charges (APC) paid by authors or their institutions.
- High Visibility: indexed within Scopus, ESCI (Web of Science), Ei Compendex, and other databases.
- Journal Rank: CiteScore - Q2 (Automotive Engineering)
- Rapid Publication: manuscripts are peer-reviewed and a first decision is provided to authors approximately 14.1 days after submission; acceptance to publication is undertaken in 3.7 days (median values for papers published in this journal in the second half of 2023).
- Recognition of Reviewers: reviewers who provide timely, thorough peer-review reports receive vouchers entitling them to a discount on the APC of their next publication in any MDPI journal, in appreciation of the work done.
Impact Factor:
2.3 (2022)
Latest Articles
Suppression of Initial Charging Torque for Electric Drive-Reconfigured On-Board Charger
World Electr. Veh. J. 2024, 15(5), 207; https://doi.org/10.3390/wevj15050207 - 9 May 2024
Abstract
This paper presents a new electric drive-reconfigured on-board charger and initial electromagnetic torque suppression method. This proposed reconfigured on-board charger does not need many components added to the original electric drive system: only a connector is needed, which is easy to add. Specifically,
[...] Read more.
This paper presents a new electric drive-reconfigured on-board charger and initial electromagnetic torque suppression method. This proposed reconfigured on-board charger does not need many components added to the original electric drive system: only a connector is needed, which is easy to add. Specifically, the inverter for propulsion is reconfigured as a buck chopper and a conduction path to match the reconfigured windings. Two of the machine phase windings serve as inductors, while the third phase winding is reutilized as a common-mode inductor. In addition, the initial charging torque is generated at the outset of the charging process, which may cause an instant shock or even rotational movement. In order to prevent vehicle movement, the reason for the charging torque and suppression method were analyzed. Further, predictive control of the model based on mutual inductance analysis was adopted, where the charging torque was directly used as a control object in the cost function. Finally, experimental performances were applied to verify the proposed reconfigured on-board charger under constant current and constant voltage charging.
Full article
(This article belongs to the Special Issue Emerging Topologies and Control of Electric-Drive-Reconstructed Onboard Charger for Electric Vehicles)
►
Show Figures
Open AccessArticle
Modeling an Investment Framework for BMTA Electric Bus Fleet Development
by
Sorawit Wanitanukul, Kuskana Kubaha and Roongrojana Songprakorp
World Electr. Veh. J. 2024, 15(5), 206; https://doi.org/10.3390/wevj15050206 - 9 May 2024
Abstract
►▼
Show Figures
In Thailand, diesel buses are notorious for their poor energy efficiency and contribution to air pollution. To combat these issues, battery electric buses (BEBs) have emerged as a promising alternative. However, their high initial costs have posed challenges for fleet management, especially for
[...] Read more.
In Thailand, diesel buses are notorious for their poor energy efficiency and contribution to air pollution. To combat these issues, battery electric buses (BEBs) have emerged as a promising alternative. However, their high initial costs have posed challenges for fleet management, especially for agencies such as the Bangkok Mass Transit Authority (BMTA). This study aims to revolutionize BEB fleet management by developing an energy model tailored to the BMTA’s needs. The methodology consists of two crucial steps: analyzing BMTA bus routes and designing fleet management and charging systems. Through this process, the study seeks to determine the maximum number of BEBs that can be operated on each route with the fewest chargers possible. The results reveal exciting possibilities. Within the city bus landscape, two out of five BMTA bus routes show potential for transitioning to BEBs, provided they meet a maximum energy requirement of 200 kWh every two rounds. This analysis identifies routes ripe for BEB adoption while considering the limitations of battery size. In the next step, the study unveils a game-changing strategy: a maximum of 13 BEBs can operate on two routes with just four chargers requiring 150 kW each. This means fewer chargers and more efficient operations. Plus, the charging profile peaks at 600 kW from 4:00 to 8:00 p.m., showing when and where the fleet needs power the most. However, the real eye-opener? Significant energy savings of THB 10.44 million per year compared to diesel buses, with an initial investment cost savings of over 37%. These findings underscore the potential for BEB fleet management to revolutionize public transportation and save money in the long run. However, there is more work to be done. The study highlights the need for real-time passenger considerations, the development of post-service charging strategies, and a deeper dive into total lifetime costs. These areas of improvement promise even greater strides in the future of sustainable urban transportation.
Full article
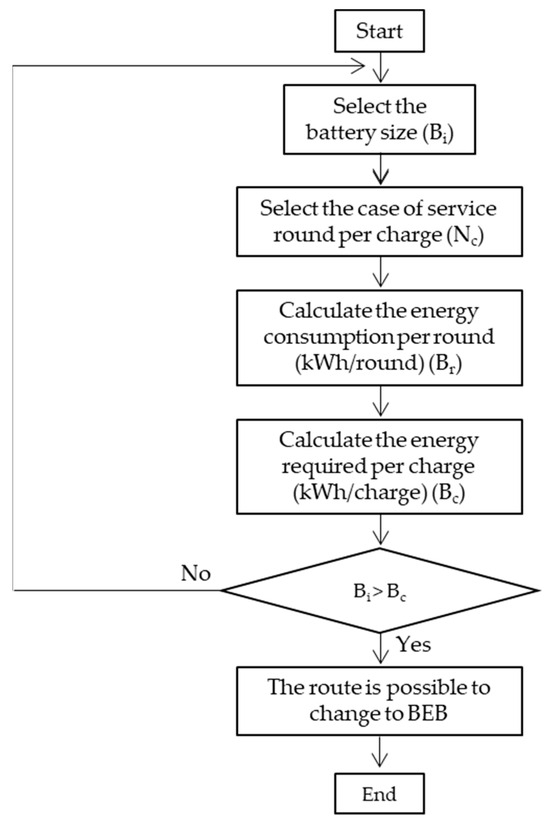
Figure 1
Open AccessArticle
An Effective Charging Torque Elimination Method for Dual-Channel Electric-Drive-Reconstructed Onboard Chargers
by
Xunhui Cheng, Feng Yu and Linhao Qiu
World Electr. Veh. J. 2024, 15(5), 205; https://doi.org/10.3390/wevj15050205 - 8 May 2024
Abstract
The idea of electric-drive-reconstructed onboard charger (EDROC) systems, along with the concept of dual-channel charging, offers a novel design, thought to enhance the integration and fault tolerance of the charging system of electric vehicles (EVs). This article investigates a dual-channel EDROC incorporating an
[...] Read more.
The idea of electric-drive-reconstructed onboard charger (EDROC) systems, along with the concept of dual-channel charging, offers a novel design, thought to enhance the integration and fault tolerance of the charging system of electric vehicles (EVs). This article investigates a dual-channel EDROC incorporating an asymmetrical six-phase permanent magnet synchronous machine (ASPMSM). A unique operation mode, called the unbalanced charging voltage operation mode, exists in this topology, in case the voltages of the two batteries are unequal. This unbalance results in different winding currents following through two channels, leading to an undesired charging torque in the machine. To ensure the safety of the system, an effective charging torque elimination method, based on dual-channel winding current balance, is proposed, which achieves a dot-shaped current path of torque generation-associated subspace (i.e., α–β subspace) by balancing the dual-channel charging power. Eventually, a controller is designed for the system and a prototype is created, to validate the effectiveness of the proposed method.
Full article
(This article belongs to the Special Issue Emerging Topologies and Control of Electric-Drive-Reconstructed Onboard Charger for Electric Vehicles)
Open AccessArticle
Online Fault Detection of Open-Circuit Faults in a DTP-PMSM Using Double DQ Current Prediction
by
Qiang Geng, Wenhao Du, Xuefeng Jin, Guozheng Zhang and Zhanqing Zhou
World Electr. Veh. J. 2024, 15(5), 204; https://doi.org/10.3390/wevj15050204 - 8 May 2024
Abstract
This research proposes a strategy to diagnose open-phase faults (OPF) and open-switching faults (OSF) in dual three-phase permanent magnet synchronous motor (DTP-PMSM) inverters. The method is based on the dual d–q predictive current model and involves establishing a mathematical model and utilizing the
[...] Read more.
This research proposes a strategy to diagnose open-phase faults (OPF) and open-switching faults (OSF) in dual three-phase permanent magnet synchronous motor (DTP-PMSM) inverters. The method is based on the dual d–q predictive current model and involves establishing a mathematical model and utilizing the finite control set model predictive current extraction technique to predict the motor current. It then analyzes the characteristics of the switching-tube current under both normal and fault conditions. Finally, a fault predictive current model is introduced and the residual is calculated based on the predicted fault current value and the actual measured current value to diagnose the inverter fault. The proposed method effectively overcomes misjudgment issues encountered in traditional open-circuit fault diagnosis of inverters. It enhances the system’s response speed during dynamic processes and strengthens the robustness of diagnosis algorithm parameters. The experimental results demonstrate that the proposed method can rapidly, effectively, and accurately diagnose open-circuit faults presented in this paper fastest within one-fifth of a current cycle. It achieves a diagnostic accuracy rate of 97% in the dual three-phase permanent magnet synchronous motor drive system.
Full article
(This article belongs to the Special Issue Temperature Field, Electromagnetic Field, and Operation Control of Permanent Magnet Motor for Electric Vehicles)
►▼
Show Figures
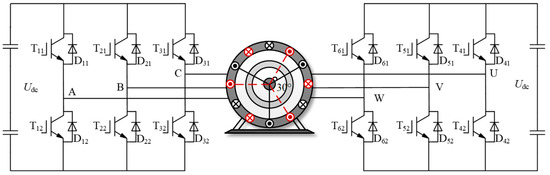
Figure 1
Open AccessArticle
Position Correction Control of Permanent-Magnet Brushless Motor Based on Commutation-Interval Current Symmetry
by
Yongwu Guo, Yun Zhang and Xiaowei Li
World Electr. Veh. J. 2024, 15(5), 203; https://doi.org/10.3390/wevj15050203 - 7 May 2024
Abstract
With the needs of environmental protection and the adjustment of energy structure, new energy vehicles are playing an increasingly important role in the field of transportation today. The permanent-magnet brushless direct-current motor has the characteristics of high efficiency, and can be used in
[...] Read more.
With the needs of environmental protection and the adjustment of energy structure, new energy vehicles are playing an increasingly important role in the field of transportation today. The permanent-magnet brushless direct-current motor has the characteristics of high efficiency, and can be used in the drive system of new energy vehicles or other auxiliary equipment. In the control process of the permanent-magnet brushless direct-current motor, based on a three-Hall position sensor, due to various factors, there are some errors in the Hall position signal, which must be corrected by appropriate measures. In this paper, the relationship between the position deviation in the commutation interval and the non-commutation-phase current is analyzed, and the current expressions in three different states are given. A new closed-loop compensation strategy for correcting the inaccurate commutation caused by the Hall signal error is proposed. Taking the position of a 30° electrical angle before and after the phase-change point as the H point, realizing the current symmetry within the 30° interval around the H point as the target and the sum of the slopes of the tangent lines at the two points symmetrical within the β (0 < β < 30) electrical angle around the H point as the deviation, a proportional-integral regulator is designed to correct the phase error of the phase-change signal. Finally, it is verified by experiments that the closed-loop compensation strategy proposed in this paper can effectively compensate the phase deviation of the commutation signal at a speed of about 2000 r/min, which improves the working efficiency of the motor to a certain extent.
Full article
(This article belongs to the Special Issue Design and Control of Electrical Machines in Electric Vehicles, 2nd Edition)
Open AccessArticle
Distributed-Drive Vehicle Lateral-Stability Coordinated Control Based on Phase-Plane Stability Region
by
Jun Liu and Ang Dai
World Electr. Veh. J. 2024, 15(5), 202; https://doi.org/10.3390/wevj15050202 - 7 May 2024
Abstract
The lateral stability control of vehicles is one of the most crucial aspects of vehicle safety. This article introduces a coordinated-control strategy designed to enhance the handling stability of distributed-drive electric vehicles. The upper controller uses active front steering and direct yaw moment-control
[...] Read more.
The lateral stability control of vehicles is one of the most crucial aspects of vehicle safety. This article introduces a coordinated-control strategy designed to enhance the handling stability of distributed-drive electric vehicles. The upper controller uses active front steering and direct yaw moment-control controllers designed based on sliding-mode control theory. The lower controller optimally allocates control inputs to the upper controller, considering factors such as load transfer and tire load rate. It divides the stability region by relying on the phase plane and develops a coordinated-control strategy based on the degree of deviation of the vehicle state from the stability region. The results of the simulation experiments demonstrate that the proposed control strategy effectively improves handling stability under extreme working conditions.
Full article
(This article belongs to the Special Issue Intelligent Electric Vehicle Control, Testing and Evaluation)
►▼
Show Figures
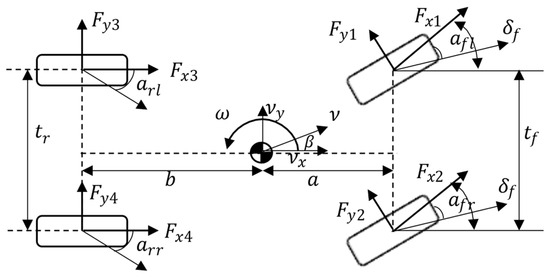
Figure 1
Open AccessArticle
Optimization Design of Variable Reluctance Resolver Based on Three-phase Symmetrical Winding
by
Xinmin Li, Jiannan Chen and Zhen Zhang
World Electr. Veh. J. 2024, 15(5), 201; https://doi.org/10.3390/wevj15050201 - 6 May 2024
Abstract
In order to ease the structure and manufacturing process of the variable reluctance (VR) resolver, the three-phase symmetrical single-layer winding commonly used in the stator winding of permanent magnet synchronous motors (PMSM) is applied to the VR resolver in this paper. The proposed
[...] Read more.
In order to ease the structure and manufacturing process of the variable reluctance (VR) resolver, the three-phase symmetrical single-layer winding commonly used in the stator winding of permanent magnet synchronous motors (PMSM) is applied to the VR resolver in this paper. The proposed resolver has the same winding direction and number of turns on all teeth. And the non-overlapping distribution of the three-phase windings of the resolver is ensured. For this novel resolver, the resolver-to-digital conversion (RDC) method references the ultra-high-frequency (UHF) signal injection method used when a PMSM is powered off and restarted. Instead of the need for the orthogonal envelope RDC required by conventional resolvers, the absolute position of the rotor can be obtained. In this paper, the prototype of the proposed resolver and the peripheral circuits are fabricated and compared with the position detected by the optical encoder, and the validity of the proposed resolver and the accuracy of the RDC are verified by the results of the comparison experiments.
Full article
(This article belongs to the Special Issue Advanced Electrical Machine and Power Electronics for the Charging and Drive System of Electric Vehicles (EVs))
►▼
Show Figures
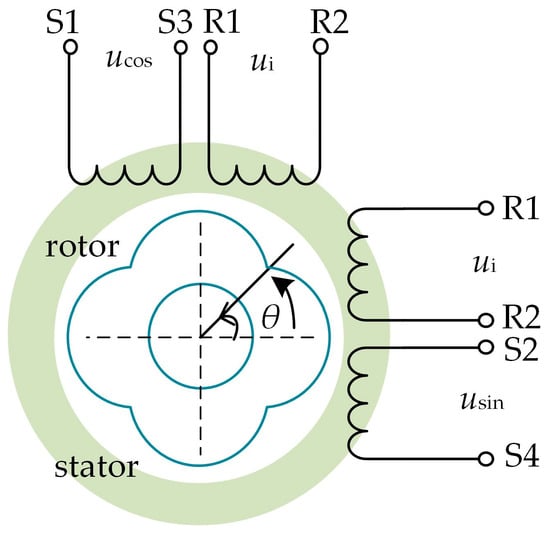
Figure 1
Open AccessArticle
Improved Equivalent Strain Method for Fatigue Life of Automobile Aluminum Alloy
by
Shanjie Zhi, Hejian Liu and Xintian Liu
World Electr. Veh. J. 2024, 15(5), 200; https://doi.org/10.3390/wevj15050200 - 6 May 2024
Abstract
Automotive parts are usually subjected to random loads with large mean tensile/compressive stresses under working conditions. It is important for automotive parts to have a long fatigue life under mean stress in practical engineering applications. An equivalent strain model is established here to
[...] Read more.
Automotive parts are usually subjected to random loads with large mean tensile/compressive stresses under working conditions. It is important for automotive parts to have a long fatigue life under mean stress in practical engineering applications. An equivalent strain model is established here to predict fatigue life considering the influence of mean strain and stress under asymmetric cycles. To predict the fatigue life more accurately, the coefficient of surface roughness and temperature correction is introduced in this model. The effectiveness of the improved equivalent strain (IES) model is verified by comparing it with multiple sets of experimental data. The IES is also compared with Smith–Watson–Topper (SWT), Manson–Coffin, and equivalent strain models. The results show that the developed model has a higher prediction accuracy than the other models. An improved fatigue strength exponent is introduced to modify the equivalent strain model, and the effectiveness of the model is verified by experimental data. The IES model demonstrates significantly reduced standard deviations under various strain ratios (−0.06, 0.06, 0.5), with measurements of 0.0936, 0.0721, and 0.0636, respectively. The method provides a certain reference for the life prediction of automotive parts.
Full article
(This article belongs to the Special Issue Electric Vehicle Networking and Traffic Control)
►▼
Show Figures
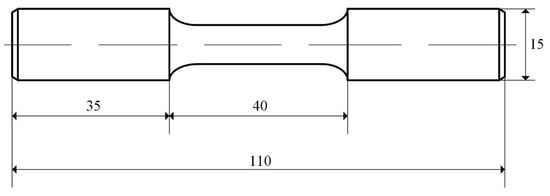
Figure 1
Open AccessArticle
Direct Torque Control of Dual Three-Phase Permanent Magnet Synchronous Motors Based on Master–Slave Virtual Vectors
by
Qiang Geng, Ziteng Qin, Xuefeng Jin, Guozheng Zhang and Zhanqing Zhou
World Electr. Veh. J. 2024, 15(5), 199; https://doi.org/10.3390/wevj15050199 - 4 May 2024
Abstract
In order to further reduce the torque, flux-linkage fluctuation, and current harmonic content of dual three-phase permanent magnet synchronous motors, this paper proposes a direct torque control strategy combined with a master–slave virtual vector duty cycle assignment. Two types of virtual voltage vectors
[...] Read more.
In order to further reduce the torque, flux-linkage fluctuation, and current harmonic content of dual three-phase permanent magnet synchronous motors, this paper proposes a direct torque control strategy combined with a master–slave virtual vector duty cycle assignment. Two types of virtual voltage vectors with different amplitudes are used to form a harmonic suppression switching table. The virtual vectors are classified into master and slave virtual vectors according to the degree of influence on the torque and the flux-linkage. Then, the duty cycle of the master and slave virtual vectors is recalculated and allocated through the evaluation function to achieve accurate control of the torque and the flux-linkage. Finally, the switching sequences of the master and slave virtual vectors that act together in one control cycle are rearranged into a symmetrical waveform. It is experimentally verified that the phase current THD of the proposed strategy is reduced by 69.4%, the 5th and 7th current harmonics content is significantly reduced, and the torque fluctuation and flux-linkage fluctuation can also be effectively suppressed, which provides better dynamic performance and steady-state performance.
Full article
(This article belongs to the Special Issue Temperature Field, Electromagnetic Field, and Operation Control of Permanent Magnet Motor for Electric Vehicles)
►▼
Show Figures
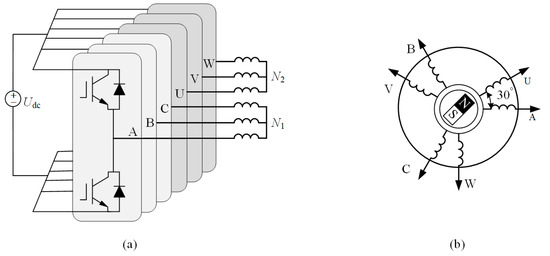
Figure 1
Open AccessArticle
Personalized Path-Tracking Approach Based on Reference Vector Field for Four-Wheel Driving and Steering Wire-Controlled Chassis
by
Changhua Dai, Changfu Zong, Dong Zhang, Hongyu Zheng, Chuyo Kaku, Dingheng Wang and Kai Zhao
World Electr. Veh. J. 2024, 15(5), 198; https://doi.org/10.3390/wevj15050198 - 3 May 2024
Abstract
It is essential and forward-thinking to investigate the personalized use of four-wheel driving and steering wire-controlled unmanned chassis. This paper introduces a personalized path-tracking approach designed to adapt the vehicle’s control system to human-like characteristics, enhancing the fit and maximizing the potential of
[...] Read more.
It is essential and forward-thinking to investigate the personalized use of four-wheel driving and steering wire-controlled unmanned chassis. This paper introduces a personalized path-tracking approach designed to adapt the vehicle’s control system to human-like characteristics, enhancing the fit and maximizing the potential of the chassis’ multi-directional driving and steering capabilities. By modifying the classic vehicle motion controller design, this approach aligns with individual driving habits, significantly improving upon traditional path-tracking control methods that rely solely on reference vector fields. First, the classic reference vector field’s logic was expanded upon, and it is shown that a personalized upgrade is feasible. Then, driving behavior data from multiple drivers were collected using a driving simulator. The fuzzy c-means clustering method was used to categorize drivers based on typical states that match vehicle path-tracking performance. Additionally, the random forest algorithm was used as the method for recognizing driving style. Subsequently, a personalized path-tracking control strategy based on the reference vector field was developed and a distributed execution architecture for four-wheel driving and steering wire-controlled unmanned chassis was established. Finally, the proposed personalized path-tracking approach was validated using a driving simulator. The results of the experimental tests demonstrated that the personalized path-tracking control approach not only fits well with various driving styles but also delivers high accuracy in driving style identification, making it highly suitable for application in four-wheel driving and steering wire-controlled chassis.
Full article
(This article belongs to the Special Issue Dynamics Modelling and Control of Electrified Chassis for Intelligent Vehicles)
►▼
Show Figures
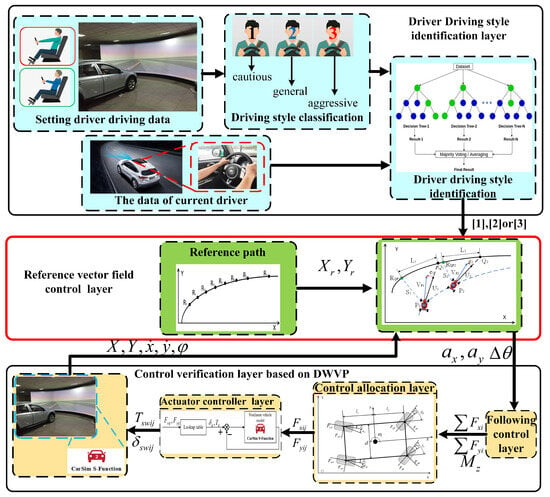
Figure 1
Open AccessArticle
Flexible Charging to Energy Saving—Strategies Assessment with Big Data Analysis for PHEVs Private Cars
by
Natascia Andrenacci, Giancarlo Giuli, Antonino Genovese and Giovanni Pede
World Electr. Veh. J. 2024, 15(5), 197; https://doi.org/10.3390/wevj15050197 - 3 May 2024
Abstract
In road transport, most vehicles today still rely on internal combustion engines. However, these engines have lower efficiency and generate higher pollution levels compared to electric motors. Consequently, there is a growing interest in the transition from conventional vehicles to electric ones. However,
[...] Read more.
In road transport, most vehicles today still rely on internal combustion engines. However, these engines have lower efficiency and generate higher pollution levels compared to electric motors. Consequently, there is a growing interest in the transition from conventional vehicles to electric ones. However, the transition to an electrified road transport system is not without challenges. Among these, the impact that electric vehicle charging will have on the electricity grid is of particular concern. This paper analyzes different charging scenarios for plug-in hybrid electric vehicles (PHEVs) and proposes charging strategies to minimize their impact on the electricity grid. The analysis is based on a large dataset of trips in urban areas in Italy. The study shows that smart charging of PHEVs can be implemented to minimize the impact on the electricity grid. The implementation of optimized charging strategies can contribute to making PHEVs a valid, eco-sustainable alternative to conventional vehicles while also promoting the stability and efficiency of the electricity grid. The study aims to verify the effectiveness and efficiency of the flexible charging strategy by comparing the common charging operation (first in–first out) with other, less impactful charging schemes.
Full article
(This article belongs to the Special Issue Smart Charging Strategies for Plug-In Electric Vehicles)
►▼
Show Figures
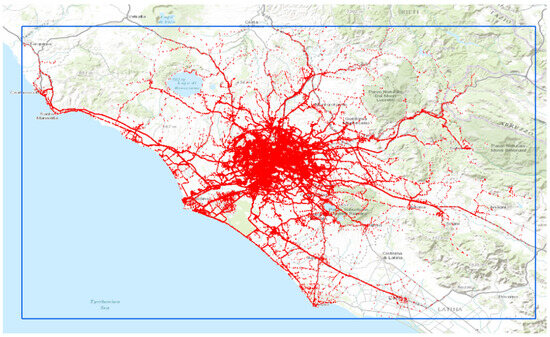
Figure 1
Open AccessArticle
Mutual Inductance Identification and Bilateral Cooperation Control Strategy for MCR-BE System
by
Ke Li, Yuanmeng Liu, Xiaodong Sun and Xiang Tian
World Electr. Veh. J. 2024, 15(5), 196; https://doi.org/10.3390/wevj15050196 - 2 May 2024
Abstract
Considering that the excitation method of an electric excitation synchronous motor has the disadvantages of the brush and slip ring, this article proposes a new brushless excitation system, which includes two parts: a wireless charging system and a motor. To meet the requirements
[...] Read more.
Considering that the excitation method of an electric excitation synchronous motor has the disadvantages of the brush and slip ring, this article proposes a new brushless excitation system, which includes two parts: a wireless charging system and a motor. To meet the requirements of maximum transmission efficiency and constant voltage output of the system, a bilateral cooperation control strategy is proposed. For the strategy, the buck converter in the receiving side of the system can maintain maximum transmission efficiency through impedance matching, while the inverter in the transmitting side can keep the output voltage constant through phase shift modulation. In the control process, considering that the offset of coupling coils will affect the control results, a grey wolf optimization–particle swarm optimization algorithm is proposed to identify mutual inductance. Simulation and experimental results show that this identification algorithm can improve the identification accuracy and maximize the avoidance of falling into local optima. The final experimental result shows that the bilateral cooperation control strategy can maintain the output voltage around 48 V and the transmission efficiency around 84.5%, which meets the expected requirements.
Full article
(This article belongs to the Special Issue Permanent Magnet Motors and Driving Control for Electric Vehicles)
►▼
Show Figures
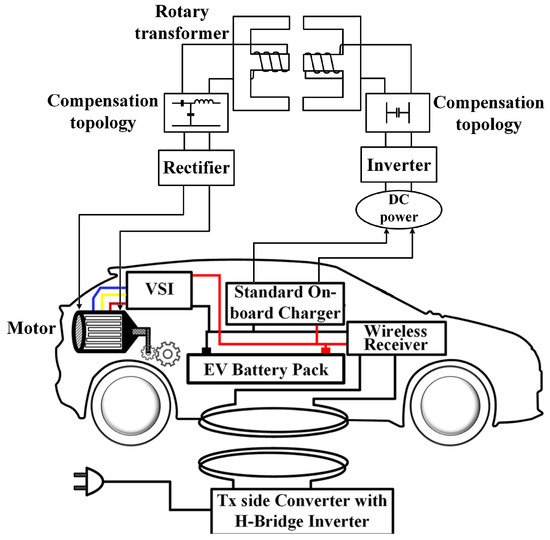
Figure 1
Open AccessArticle
A Double-Threshold Cooperative Spectrum Sensing Algorithm in the Internet of Vehicles
by
Hong Du and Yuhan Wang
World Electr. Veh. J. 2024, 15(5), 195; https://doi.org/10.3390/wevj15050195 - 2 May 2024
Abstract
►▼
Show Figures
To address the shortage of wireless spectrum resources caused by the rapid development of the Internet of Vehicles, spectrum sensing technology in cognitive radio is employed to tackle this issue. In pursuit of superior outcomes, a double-threshold cooperative spectrum sensing algorithm is introduced.
[...] Read more.
To address the shortage of wireless spectrum resources caused by the rapid development of the Internet of Vehicles, spectrum sensing technology in cognitive radio is employed to tackle this issue. In pursuit of superior outcomes, a double-threshold cooperative spectrum sensing algorithm is introduced. This algorithm enhances traditional energy detection technology to mitigate the high sensitivity to noise interference in the Internet of Vehicles environment. A double-threshold judgment mechanism can be established based on the uncertainty of noise. Varying fusion rules are implemented in the collaborative spectrum sensing scheme according to the density of vehicles and the spectrum resource demand. Simulation results demonstrate that the performance of the double-threshold cooperative spectrum sensing algorithm surpasses that of the traditional single-threshold energy detection scheme, particularly evident under lower Signal-to-Noise Ratio (SNR) conditions. Moreover, the proposed algorithm exhibits superior sensing performance in environments characterized by higher noise uncertainty.
Full article
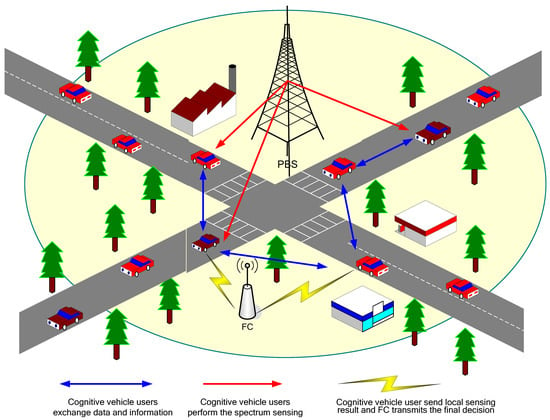
Figure 1
Open AccessArticle
Improvement of Commercial Vehicle Seat Suspension Employing a Mechatronic Inerter Element
by
Xiaofeng Yang, Shuilan Bi, Yanling Liu, Yi Yang, Changning Liu and Jiahao Qin
World Electr. Veh. J. 2024, 15(5), 194; https://doi.org/10.3390/wevj15050194 - 30 Apr 2024
Abstract
To further improve the ride comfort of commercial vehicles, a seat ISD (Inerter–Spring–Damper) suspension utilizing a mechatronic inerter is proposed in this paper. Firstly, a five-DOF (degree-of-freedom) commercial vehicle seat ISD model was built. Then, the positive real network constraint conditions of a
[...] Read more.
To further improve the ride comfort of commercial vehicles, a seat ISD (Inerter–Spring–Damper) suspension utilizing a mechatronic inerter is proposed in this paper. Firstly, a five-DOF (degree-of-freedom) commercial vehicle seat ISD model was built. Then, the positive real network constraint conditions of a biquadratic impedance transfer function were determined, and the meta-heuristic intelligent optimization algorithm was used to solve the parameters. According to the solution, the impedance transfer function was obtained and the specific network structure was realized by network synthesis. Lastly, this study compares the vibration isolation performance of the mechatronic ISD suspension of the vehicle seat with that of a passive suspension. In comparison to passive seat suspension, the seat mechatronic ISD suspension reduces seat vibration transmissibility by 16.33% and vertical acceleration by 16.78%. Results indicate that the new suspension system can be an effective improvement in ride comfort.
Full article
(This article belongs to the Special Issue Advanced Vehicle System Dynamics and Control)
►▼
Show Figures
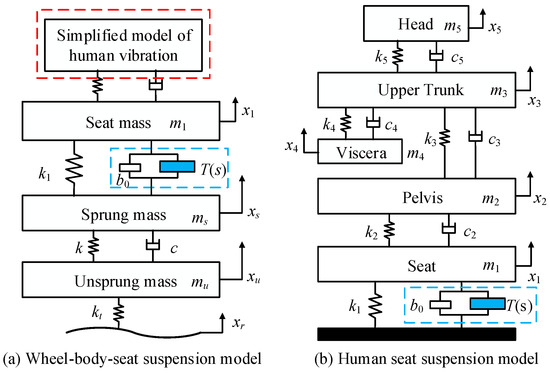
Figure 1
Open AccessArticle
Battery Research and Innovation—A Study of Patents and Papers
by
Hans Pohl and Måns Marklund
World Electr. Veh. J. 2024, 15(5), 193; https://doi.org/10.3390/wevj15050193 - 29 Apr 2024
Abstract
This study of patent applications and scientific publications related to batteries is unique as it includes the volume of as well as qualitative indicators for both types of publications. Using carefully elaborated strategies to identify publications relating to batteries, this study provides data
[...] Read more.
This study of patent applications and scientific publications related to batteries is unique as it includes the volume of as well as qualitative indicators for both types of publications. Using carefully elaborated strategies to identify publications relating to batteries, this study provides data to discuss the critical balance to strike between investments in research and the more innovation-related aspects. The results show that China’s dominance in publication volumes increases and that research with Chinese involvement is highly cited, whereas patent applications are slightly less valued than the world average. Quality-related indicators for Canada and the United States are very high for both scientific publications and patent applications. National differences in the proportions of patent applications and scientific publications are large, with Japan at one end with three patent applications per scientific paper and Canada at the other with almost seven scientific papers per patent application. On an actor level, data for Sweden indicate how the automotive industry started to file many patent applications in the decade starting in 2010. Finally, it is noted that this new approach to study a technological field appears promising as it gives new perspectives of relevance for policy actors and others.
Full article
(This article belongs to the Special Issue EVS36—International Electric Vehicle Symposium and Exhibition (California, USA))
►▼
Show Figures
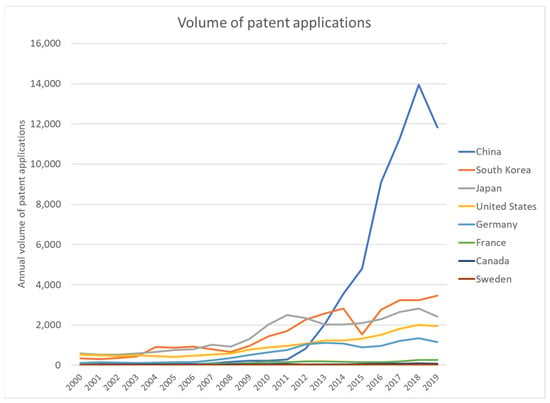
Figure 1
Open AccessArticle
Design and Aerodynamic Characteristics Analysis of an Electric Racecar Body Based on CFD
by
Jixiong Li, Fengbi Liu and Lei Wang
World Electr. Veh. J. 2024, 15(5), 192; https://doi.org/10.3390/wevj15050192 - 29 Apr 2024
Abstract
This study focuses on the development of a body for an electric racecar, utilizing CAD software for the design. A simplified full-vehicle geometric model was constructed. Based on fundamental theories of computational fluid dynamics and using CAE software platforms, the shear stress transport
[...] Read more.
This study focuses on the development of a body for an electric racecar, utilizing CAD software for the design. A simplified full-vehicle geometric model was constructed. Based on fundamental theories of computational fluid dynamics and using CAE software platforms, the shear stress transport (SST) k-ω physical model was chosen to establish a three-dimensional computational model of the racecar’s external flow field. Simulations were conducted to analyze the pressure, airflow streamlines, and velocity distribution around the body and its surrounding flow field, elucidating the impact of body shape structure on aerodynamic characteristics. Finally, a manufacturing process for the body was designed, and a prototype was produced and integrated into the complete vehicle for road testing. The results indicate that the designed electric racecar body maintained consistent airflow over its surface, meeting the basic requirements of aerodynamics.
Full article
(This article belongs to the Topic Advanced Electrical Machine Design and Optimization Ⅱ)
►▼
Show Figures
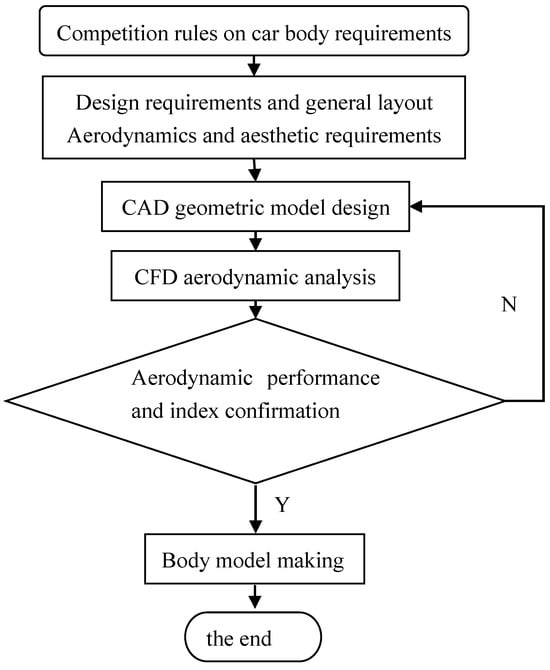
Figure 1
Open AccessReview
OCPP Interoperability: A Unified Future of Charging
by
Silke R. Kirchner
World Electr. Veh. J. 2024, 15(5), 191; https://doi.org/10.3390/wevj15050191 - 29 Apr 2024
Abstract
Electric vehicle (EV) adoption grows steadily on a global scale, yet there is no consistent experience for EV drivers to charge their vehicles, which hinders the important EV mass market adoption. The Open Charge Point Protocol (OCPP) is the solution to this challenge,
[...] Read more.
Electric vehicle (EV) adoption grows steadily on a global scale, yet there is no consistent experience for EV drivers to charge their vehicles, which hinders the important EV mass market adoption. The Open Charge Point Protocol (OCPP) is the solution to this challenge, as it provides standardization and open communication between EV infrastructure components. The interplay of the OCPP with open cross-functional communication standards boosters driver experience on the one hand, while the charging station itself is integrated into a renewable energy ecosystem. This paper presents a deep dive into the combination of the OCPP with the OpenADR protocol, the Open Smart Charging Protocol (OSCP), the ISO 15118, and eRoaming protocols to explore possibilities and limitations. Furthermore, we suggest LoRa communication as an alternative to IP-based communication for deep-in building applications. Hence, this paper reveals the next important steps towards a successful EV mass market transition powered by user-friendliness and green energy.
Full article
(This article belongs to the Special Issue EVS36—International Electric Vehicle Symposium and Exhibition (California, USA))
►▼
Show Figures
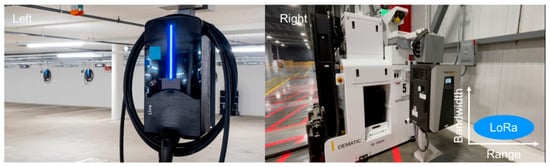
Figure 1
Open AccessReview
Control Strategies, Economic Benefits, and Challenges of Vehicle-to-Grid Applications: Recent Trends Research
by
Guangjie Chen and Zhaoyun Zhang
World Electr. Veh. J. 2024, 15(5), 190; https://doi.org/10.3390/wevj15050190 - 28 Apr 2024
Abstract
With the rapid growth in the number of EVs, a huge number of EVs are connected to the power grid for charging, which places a great amount of pressure on the stable operation of the power grid. This paper focuses on the development
[...] Read more.
With the rapid growth in the number of EVs, a huge number of EVs are connected to the power grid for charging, which places a great amount of pressure on the stable operation of the power grid. This paper focuses on the development of V2G applications, based on the current research status of V2G technology. Firstly, the standards on V2G applications and some pilot projects involving more representative V2G systems are introduced. Comparing V2G applications with ordered charging and unordered charging, the social and economic benefits of V2G applications are highlighted. Analysis of the social benefits of V2G applications concerns three points: the grid demand response, personalized charging, and the coordination of renewable energy sources. And analysis of the economic benefits of V2G applications is divided into three parties: the grid, the aggregator, and individuals. From the perspective of innovative EVs expanding the application scenarios through V2G technology, V2G applications for commercial EVs, emergency power applications, and vehicle-to-vehicle energy trading are introduced. The current challenges related to V2G applications are presented: users’ willingness to participate in V2G applications, battery loss, charging and discharging tariffs, privacy and security, and power loss. Finally, some research recommendations for the development of V2G applications are given and the current state of research in regard to those recommendations is presented.
Full article
(This article belongs to the Special Issue Electric Vehicles and Smart Grid Interaction)
►▼
Show Figures
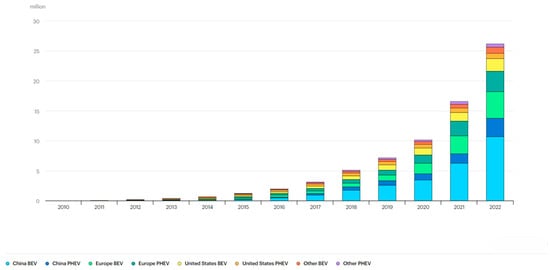
Figure 1
Open AccessArticle
Short-Term Charging Load Prediction of Electric Vehicles with Dynamic Traffic Information Based on a Support Vector Machine
by
Qipei Zhang, Jixiang Lu, Wenteng Kuang, Lin Wu and Zhaohui Wang
World Electr. Veh. J. 2024, 15(5), 189; https://doi.org/10.3390/wevj15050189 - 28 Apr 2024
Abstract
This study proposes a charging demand forecasting model for electric vehicles (EVs) that takes into consideration the characteristics of EVs with transportation and mobile load. The model utilizes traffic information to evaluate the influence of traffic systems on driving and charging behavior, specifically
[...] Read more.
This study proposes a charging demand forecasting model for electric vehicles (EVs) that takes into consideration the characteristics of EVs with transportation and mobile load. The model utilizes traffic information to evaluate the influence of traffic systems on driving and charging behavior, specifically focusing on the characteristics of EVs with transportation and mobile load. Additionally, it evaluates the effect of widespread charging on the distribution network. An urban traffic network model is constructed based on the multi-intersection features, and a traffic network–distribution network interaction model is determined according to the size of the urban road network. Type classification simplifies the charging and discharging characteristics of EVs, enabling efficient aggregation of EVs. The authors have built a singular EV transportation model and an EV charging queue model is established. The EV charging demand is forecasted and then used as an input in the support vector machine (SVM) model. The final projection value for EV charging load is determined by taking into account many influencing elements. Compared to the real load, the proposed method’s feasibility and effectiveness are confirmed.
Full article
(This article belongs to the Special Issue Fast-Charging Station for Electric Vehicles: Challenges and Issues)
►▼
Show Figures
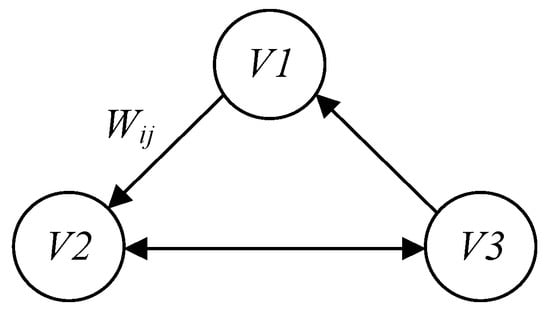
Figure 1
Open AccessArticle
Research on Power Optimization for Energy System of Hydrogen Fuel Cell Wheel-Driven Electric Tractor
by
Jingyun Zhang, Buyuan Wang, Junjiang Zhang, Liyou Xu and Kai Zhang
World Electr. Veh. J. 2024, 15(5), 188; https://doi.org/10.3390/wevj15050188 - 28 Apr 2024
Abstract
Hydrogen fuel cell tractors are emerging as a new power source for tractors. Currently, there is no mature energy management control method available. Existing methods mostly rely on engineers’ experience to determine the output power of the fuel cell and the power battery,
[...] Read more.
Hydrogen fuel cell tractors are emerging as a new power source for tractors. Currently, there is no mature energy management control method available. Existing methods mostly rely on engineers’ experience to determine the output power of the fuel cell and the power battery, resulting in relatively low energy utilization efficiency of the energy system. To address the aforementioned problems, a power optimization method for the energy system of hydrogen fuel cell wheel-driven electric tractor was proposed. A dynamic model of tractor ploughing conditions was established based on the system dynamics theory. From this, based on the equivalent hydrogen consumption theory, the charging and discharging of the power battery were equivalent to the fuel consumption of the hydrogen fuel cell, forming an equivalent hydrogen consumption model for the tractor. Using the state of charge (SOC) of the power battery as a constraint, and with the minimum equivalent hydrogen consumption as the objective function, an instantaneously optimized power allocation method based on load demand in the energy system is proposed by using a traversal algorithm. The optimization method was simulated and tested based on the MATLAB simulation platform, and the results showed under ploughing conditions, compared with the rule-based control strategy, the proposed energy system power optimization method optimized the power output of hydrogen fuel cells and power batteries, allowing the energy system to work in a high-efficiency range, reducing the equivalent hydrogen consumption of the tractor by 7.79%, and solving the energy system power distribution problem.
Full article
(This article belongs to the Special Issue New Energy Special Vehicle, Tractor and Agricultural Machinery)
►▼
Show Figures
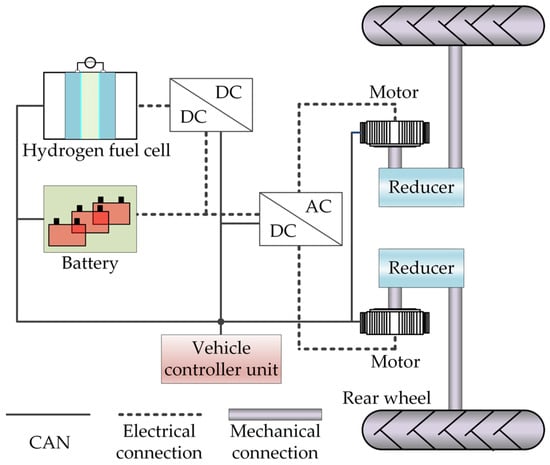
Figure 1
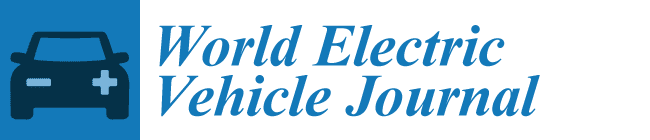
Journal Menu
► ▼ Journal Menu-
- WEVJ Home
- Aims & Scope
- Editorial Board
- Topical Advisory Panel
- Instructions for Authors
- Special Issues
- Topics
- Article Processing Charge
- Indexing & Archiving
- Editor’s Choice Articles
- Most Cited & Viewed
- Journal Statistics
- Journal History
- Journal Awards
- Society Collaborations
- Conferences
- Editorial Office
Journal Browser
► ▼ Journal BrowserHighly Accessed Articles
Latest Books
E-Mail Alert
News
Topics
Topic in
Energies, Materials, Electronics, Machines, WEVJ
Advanced Electrical Machine Design and Optimization Ⅱ
Topic Editors: Youguang Guo, Gang Lei, Xin BaDeadline: 31 May 2024
Topic in
Energies, Processes, Electronics, Applied Sciences, WEVJ
Energy Management and Efficiency in Electric Motors, Drives, Power Converters and Related Systems
Topic Editors: Mario Marchesoni, Alfonso DamianoDeadline: 15 October 2024
Topic in
Applied Sciences, Batteries, Electricity, Electronics, Sensors, WEVJ, Technologies, Chips
Advanced Wireless Charging Technology
Topic Editors: Chong Zhu, Kailong LiuDeadline: 31 October 2024
Topic in
Computation, Electronics, Energies, Sensors, Sustainability, WEVJ
Modern Power Systems and Units
Topic Editors: Jan Taler, Ali Cemal Benim, Sławomir Grądziel, Marek Majdak, Moghtada Mobedi, Tomasz Sobota, Dawid Taler, Bohdan WęglowskiDeadline: 30 November 2024

Conferences
Special Issues
Special Issue in
WEVJ
Recent Advances in Electric Motor Drives for Electrified Mobility
Guest Editors: Mohamed Diab, Xing Zhao, Ayman Abdel-KhalikDeadline: 31 May 2024
Special Issue in
WEVJ
Dynamic Control of Traction Motors for EVs
Guest Editors: Rezkallah Miloud, Ambrish ChandraDeadline: 20 June 2024
Special Issue in
WEVJ
Data Exchange between Vehicle and Power System for Optimal Charging
Guest Editors: Jennifer Leijon, Boel Ekergård, Valeria CastellucciDeadline: 30 June 2024
Special Issue in
WEVJ
Dynamics Modelling and Control of Electrified Chassis for Intelligent Vehicles
Guest Editors: Junnian Wang, Hongqing ChuDeadline: 31 July 2024