Topic Menu
► Topic MenuTopic Editors
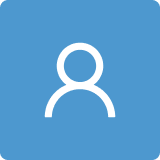
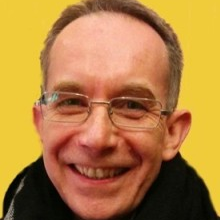


AI in Medical Imaging and Image Processing
Topic Information
Dear Colleagues,
In modern healthcare, the importance of computer-aided diagnosis is quickly becoming obvious, with clear benefits for the medical professionals and patients. Automatization of processes traditionally maintained by human professionals is also growing in importance. The process of image analysis can be supported by the use of networks that can carry out multilayer analyses of patterns—collectively called artificial intelligence (AI). If supported by large datasets of input data, computer networks can suggest the result with low error bias. Medical imaging focused on pattern detection is typically supported by AI algorithms. AI can be used as an important aid in three major steps of decision making in the medical imaging workflow: detection (image segmentation), recognition (assignment to the class), and result description (transformation of the result to natural language). The implementation of AI algorithms may participate in the diagnostic process standardization and markedly reduces the time needed to achieve pathology detection and description of the results. With AI support, medical specialists may work more effectively, which can improve healthcare quality. As AI has been a topic of interest for a while now, there are many approaches to and techniques for the implementation of AI based on different computing methods designed to work in various systems. The aim of this Special Issue in to present the current knowledge dedicated to the AI methods used in medical systems, with their applications in different fields of diagnostic imaging. Our goal is for this collection of works to contribute to the exchange of knowledge resulting in a better understanding of AI technical aspects and applications in modern radiology.
Dr. Karolina Nurzynska
Prof. Dr. Michał Strzelecki
Prof. Dr. Adam Piorkowski
Dr. Rafał Obuchowicz
Topic Editors
Keywords
- artificial intelligence
- computer-aided diagnosis
- medical imaging
- image analysis
- image processing
Participating Journals
Journal Name | Impact Factor | CiteScore | Launched Year | First Decision (median) | APC |
---|---|---|---|---|---|
![]()
BioMed
|
- | - | 2021 | 21.9 Days | CHF 1000 |
![]()
Cancers
|
4.5 | 8.8 | 2009 | 17.4 Days | CHF 2900 |
![]()
Diagnostics
|
3.0 | 5.9 | 2011 | 20.3 Days | CHF 2600 |
![]()
Journal of Clinical Medicine
|
3.0 | 5.2 | 2012 | 16 Days | CHF 2600 |
![]()
Tomography
|
2.2 | 3.5 | 2015 | 23.8 Days | CHF 2400 |
Preprints.org is a multidisciplinary platform offering a preprint service designed to facilitate the early sharing of your research. It supports and empowers your research journey from the very beginning.
MDPI Topics is collaborating with Preprints.org and has established a direct connection between MDPI journals and the platform. Authors are encouraged to take advantage of this opportunity by posting their preprints at Preprints.org prior to publication:
- Share your research immediately: disseminate your ideas prior to publication and establish priority for your work.
- Safeguard your intellectual contribution: Protect your ideas with a time-stamped preprint that serves as proof of your research timeline.
- Boost visibility and impact: Increase the reach and influence of your research by making it accessible to a global audience.
- Gain early feedback: Receive valuable input and insights from peers before submitting to a journal.
- Ensure broad indexing: Web of Science (Preprint Citation Index), Google Scholar, Crossref, SHARE, PrePubMed, Scilit and Europe PMC.
Related Topics
- Artificial Intelligence in Medical Imaging and Image Processing (35 articles)
- Machine Learning and Deep Learning in Medical Imaging (11 articles)