Topic Menu
► Topic MenuTopic Editors



2. Czech Institute of Informatics, Robotics and Cybernetics, Czech Technical University in Prague, 166 36 Prague, Czech Republic
Machine Learning and Biomedical Sensors
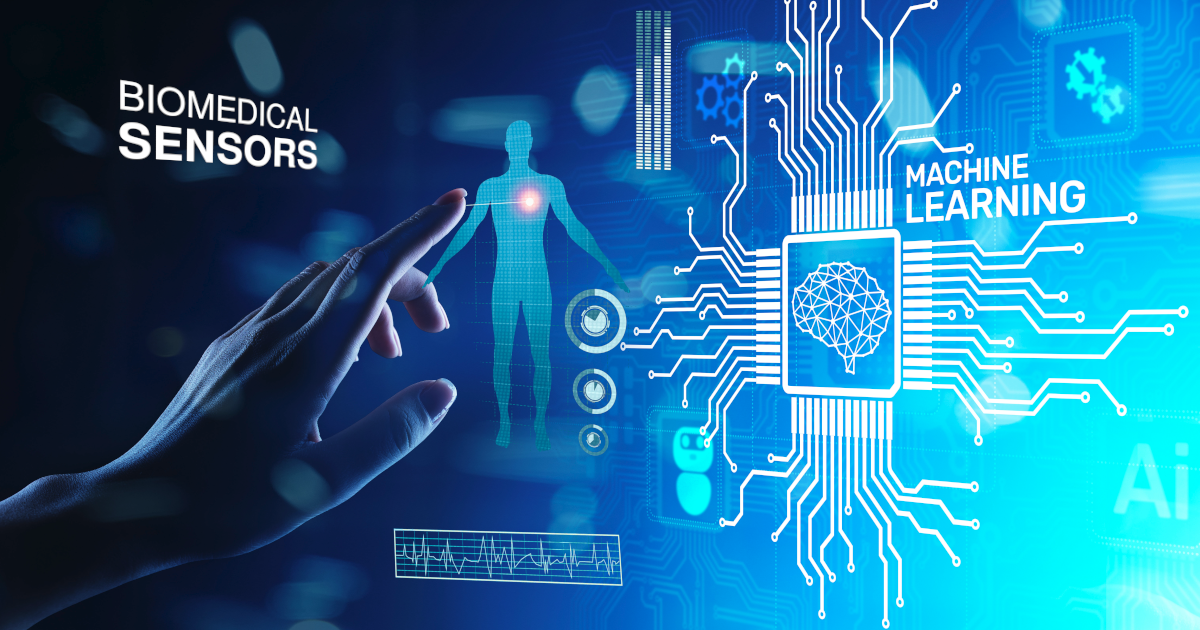
Topic Information
Dear Colleagues,
The increase in the information collected by an ever-growing number of biomedical sensors connected to the internet, together with the availability of a network of services recording any biological, physical, clinical and other kinds of data, has made the soil fertile for the significant use of machine learning (ML). It has become possible to use the various biomedical devices in the most diverse environments (at the depths of the seas, high altitudes, in space) in hospitals as well at home or outdoors, opening up new scenarios that were not previously conceivable. In this context, machine learning has shown great potential and might provide solutions to many issues concerning life on our planet. This Topic aims to present the most recent and innovative solutions leveraging the interplay between biomedical sensors and machine learning. Advanced and modern data-driven methods and learning approaches are sought to correlate and understand heterogeneous data in providing accurate classifications and predictions. The perspective opened by pervasive and edge computing should be properly transferred to the biomedical domain by devising novel activity monitoring and physiological computing paradigms. From the point of view of innovative sensing technologies, new transducers coupled with embedded computing for obtaining smart and possibly miniaturized or minimally invasive devices should be investigated. In such a panorama, the role of machine learning and artificial intelligence, more generally, should be adequately understood together with the issues related to their perception. In addition, user acceptance and privacy issues are important aspects to be assessed in real experimentation, which is necessary for clinical validation of the proposed technological solutions. The Topic, through its participating journals, is therefore seeking contributions that explore multifaced aspects of the convergence between biomedical sensors and machine learning: from fundamental elements related to computing over sensor networks and federated learning to innovative sensing principles and technologies for smart devices, from clinical experimentation and validation in healthcare scenarios to general application in ambient assisted living, contextually with the concurrent assessment of privacy and user acceptance factors.
- Human physiology & physiological computing
- Multimedia data analysis
- Digital signal and image processing
- Computer vision in biomedical sensing
- New materials and approaches for smart biomedical sensors
- Artificial intelligence over networks of biomedical sensors
- Protocols and middleware for smart biomedical sensors
- Sensors for Active and Healthy Ageing
- Internet of Biomedical Things (IoBT)
- Pilot studies and clinical validation
- User experience and acceptance of Artificial Intelligence in biomedical sensors
- Privacy and security issues
- Big Data
- Teleassistance & telemedicine
- Signals analysis & statistics methods
Dr. Massimo Martinelli
Dr. Davide Moroni
Prof. Dr. Aleš Procházka
Topic Editors
Keywords
- machine learning
- artificial intelligence
- biomedicine
- decision support systems & recommendation systems
- pervasive and mobile computing
- embedded computing
- monitoring systems based on smart sensors
- personalized services for wellbeing
- wearable smart sensors
- smartphone applications
- contactless smart sensors
- learning schemes for smart biomedical sensing
- incremental learning
- reinforcement learning
- physical activities monitoring
Participating Journals
Journal Name | Impact Factor | CiteScore | Launched Year | First Decision (median) | APC |
---|---|---|---|---|---|
![]()
Bioengineering
|
3.8 | 5.3 | 2014 | 16.4 Days | CHF 2700 |
![]()
Healthcare
|
2.4 | 4.7 | 2013 | 20.3 Days | CHF 2700 |
![]()
Journal of Clinical Medicine
|
3.0 | 5.2 | 2012 | 16 Days | CHF 2600 |
![]()
Journal of Sensor and Actuator Networks
|
3.3 | 9.4 | 2012 | 19 Days | CHF 2000 |
![]()
Sensors
|
3.4 | 8.2 | 2001 | 18.6 Days | CHF 2600 |
![]()
Applied Sciences
|
2.5 | 5.5 | 2011 | 18.4 Days | CHF 2400 |
![]()
Biosensors
|
4.9 | 9.8 | 2011 | 18.9 Days | CHF 2200 |
![]()
Inventions
|
2.1 | 4.9 | 2016 | 18.5 Days | CHF 1800 |
Preprints.org is a multidisciplinary platform offering a preprint service designed to facilitate the early sharing of your research. It supports and empowers your research journey from the very beginning.
MDPI Topics is collaborating with Preprints.org and has established a direct connection between MDPI journals and the platform. Authors are encouraged to take advantage of this opportunity by posting their preprints at Preprints.org prior to publication:
- Share your research immediately: disseminate your ideas prior to publication and establish priority for your work.
- Safeguard your intellectual contribution: Protect your ideas with a time-stamped preprint that serves as proof of your research timeline.
- Boost visibility and impact: Increase the reach and influence of your research by making it accessible to a global audience.
- Gain early feedback: Receive valuable input and insights from peers before submitting to a journal.
- Ensure broad indexing: Web of Science (Preprint Citation Index), Google Scholar, Crossref, SHARE, PrePubMed, Scilit and Europe PMC.