Journal Description
Journal of Sensor and Actuator Networks
Journal of Sensor and Actuator Networks
is an international, peer-reviewed, open access journal on the science and technology of sensor and actuator networks, published bimonthly online by MDPI.
- Open Access— free for readers, with article processing charges (APC) paid by authors or their institutions.
- High Visibility: indexed within Scopus, ESCI (Web of Science), dblp, Inspec, and other databases.
- Journal Rank: CiteScore - Q1 (Control and Optimization)
- Rapid Publication: manuscripts are peer-reviewed and a first decision is provided to authors approximately 20.4 days after submission; acceptance to publication is undertaken in 5.7 days (median values for papers published in this journal in the second half of 2023).
- Recognition of Reviewers: reviewers who provide timely, thorough peer-review reports receive vouchers entitling them to a discount on the APC of their next publication in any MDPI journal, in appreciation of the work done.
Impact Factor:
3.5 (2022);
5-Year Impact Factor:
3.6 (2022)
Latest Articles
Data-Driven Position and Stiffness Control of Antagonistic Variable Stiffness Actuator Using Nonlinear Hammerstein Models
J. Sens. Actuator Netw. 2024, 13(2), 29; https://doi.org/10.3390/jsan13020029 - 19 Apr 2024
Abstract
In this paper, an optimal PID controller is introduced for an antagonistic variable stiffness actuator (AVSA) based on Hammerstein models. A set of Hammerstein models is developed for the AVSA using the voltage difference method. For each stiffness level, linear and nonlinear Hammerstein
[...] Read more.
In this paper, an optimal PID controller is introduced for an antagonistic variable stiffness actuator (AVSA) based on Hammerstein models. A set of Hammerstein models is developed for the AVSA using the voltage difference method. For each stiffness level, linear and nonlinear Hammerstein models are identified using the least squares method. Experimental results confirm that the outputs of the Hammerstein models fit the measured data better than linear models, as Hammerstein models can incorporate nonlinear effects such as friction. A genetic algorithm is utilized to find optimal PID gains for different stiffness levels and reference position amplitudes. The final gains are obtained by linearly interpolating the optimal gains obtained. To demonstrate the effectiveness of the proposed design, several scenarios with different reference positions and stiffness profiles are provided. Specifically, square, sinusoidal, and sawtooth waves are used for reference positions and stiffness values. The robustness of the proposed approach is further analyzed by applying a disturbance force on the actuator link. The results are compared with the linear method, showing that the proposed design can handle soft transitions in stiffness variation and ensure perfect tracking.
Full article
(This article belongs to the Section Actuators, Sensors and Devices)
►
Show Figures
Open AccessArticle
Level-Crossing Characteristics of an Actively Managed Buffer
by
Andrzej Chydzinski
J. Sens. Actuator Netw. 2024, 13(2), 28; https://doi.org/10.3390/jsan13020028 - 15 Apr 2024
Abstract
In this paper, we examine a buffer with active management that rejects packets basing on the buffer occupancy. Specifically, we derive several metrics characterizing how effectively the algorithm can prevent the queue of packets from becoming too long and how well it assists
[...] Read more.
In this paper, we examine a buffer with active management that rejects packets basing on the buffer occupancy. Specifically, we derive several metrics characterizing how effectively the algorithm can prevent the queue of packets from becoming too long and how well it assists in flushing the buffer quickly when necessary. First, we compute the probability that the size of the queue is kept below a predefined level L. Second, we calculate the distribution of the amount of time needed to cross level L, the buffer overflow probability, and the average time to buffer overflow. Third, we derive the distribution of the amount of time required to flush the buffer and its average value. A general modeling framework is used in derivations, with a general service time distribution, general rejection function, and a powerful model of the arrival process. The obtained formulas enable, among other things, the solving of many design problems, e.g., those connected with the design of wireless sensor nodes using the N-policy. Several numerical results are provided, including examples of design problems and other calculations.
Full article
(This article belongs to the Section Communications and Networking)
►▼
Show Figures
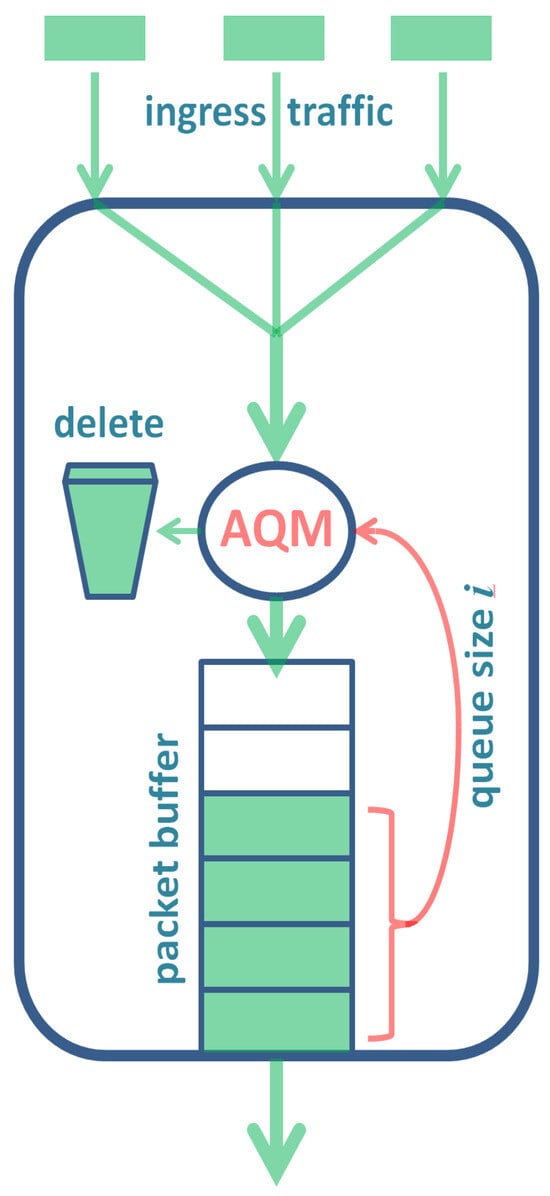
Figure 1
Open AccessArticle
Closed-Loop Optical Tracking of a Micro-Conveyor over a Smart Surface
by
Saly Malak, Hani Al Hajjar, Erwan Dupont, Muneeb-Ullah Khan, Christine Prelle and Frederic Lamarque
J. Sens. Actuator Netw. 2024, 13(2), 27; https://doi.org/10.3390/jsan13020027 - 13 Apr 2024
Abstract
►▼
Show Figures
In this work, a closed loop control system is developed to optically localize and track micro-robots with high precision. These micro-robots (i.e., micro-conveyors) are in motion simultaneously across a smart surface.The developed method’s primary objectives are to optimize their trajectories, avoid collisions between
[...] Read more.
In this work, a closed loop control system is developed to optically localize and track micro-robots with high precision. These micro-robots (i.e., micro-conveyors) are in motion simultaneously across a smart surface.The developed method’s primary objectives are to optimize their trajectories, avoid collisions between them, and control their position with micrometric resolution. This article presents and characterizes the tracking of a single micro-conveyor, and the method works similarly when multiple micro-robots move over the surface. Our tracking method starts with a scanning phase, where a 2D steering mirror, placed above the smart surface, reflects a laser beam toward the conveying surface seeking for the target. Localization occurs when this light beam reaches the micro-conveyor. By adding a retro-reflective element, that reflects the light in the same direction of the the incident light, onto the surface of the micro-conveyor, the light will be reflected towards a photodetector. Depending on the feedback from the photodetector, the steering mirror rotates to track the trajectory of the micro-conveyor. The tip-tilt angular values of the steering mirror allows the micro-conveyor position to be obtained via calibrated localization system. The aim of this work is to regulate the micro-conveyor, within a closed-loop control system, to reduce the positional error between the actual and desired position. The actual position value is measured in real-time application using our developed optical sensor. Results for tracking in the x-and y-axis have validated the proposed method, with an average tracking error less than 30 µm within a range 150 mm × 150 mm.
Full article
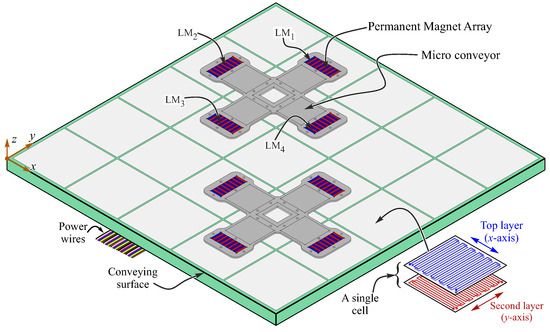
Figure 1
Open AccessReview
A Survey on Life-Cycle-Oriented Certificate Management in Industrial Networking Environments
by
Julian Göppert, Andreas Walz and Axel Sikora
J. Sens. Actuator Netw. 2024, 13(2), 26; https://doi.org/10.3390/jsan13020026 - 10 Apr 2024
Abstract
Driven by the Industry 4.0 paradigm and the resulting demand for connectivity in industrial networking, there is a convergence of formerly isolated operational technology and information technology networks. This convergence leads to attack surfaces on industrial networks. Therefore, a holistic approach of countermeasures
[...] Read more.
Driven by the Industry 4.0 paradigm and the resulting demand for connectivity in industrial networking, there is a convergence of formerly isolated operational technology and information technology networks. This convergence leads to attack surfaces on industrial networks. Therefore, a holistic approach of countermeasures is needed to protect against cyber attacks. One element of these countermeasures is the use of certificate-based authentication for industrial components communicating on the field level. This in turn requires the management of certificates, private keys, and trust anchors in the communication endpoints. The work at hand surveys the topic of certificate management in industrial networking environments throughout their life cycle, from manufacturing until their disposal. To the best of the authors’ knowledge, there is no work yet that surveys the topic of certificate management in industrial networking environments. The work at hand considers contributions from research papers, industrial communication standards, and contributions that originate from the IT domain. In total, 2042 results from IEEE Xplore, Science Direct, Scopus, and Springer Link were taken into account. After applying inclusion and exclusion criteria and title, abstract, and full-text analysis, 20 contributions from research papers were selected. In addition to the presentation of their key contributions, the work at hand provides a synopsis that compares the overarching aspects. This comprises different proposed entity architectures, certificate management functions, involvement of different stakeholders, and consideration of life cycle stages. Finally, research gaps that are to be filled by further work are identified. While the topic of certificate management has already been addressed by the IT domain, its incorporation into industrial communication standards began significantly later and is still the subject of research work.
Full article
(This article belongs to the Section Network Security and Privacy)
►▼
Show Figures
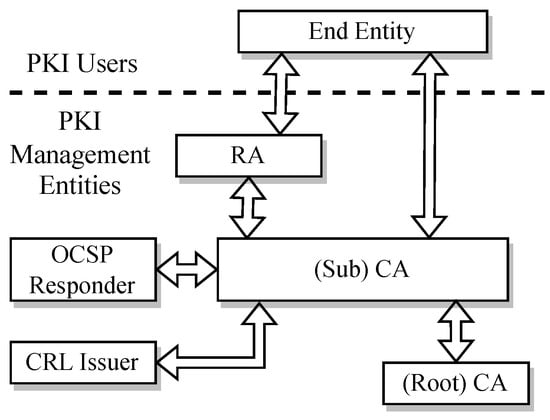
Figure 1
Open AccessArticle
Assessing the Acceptance of a Mid-Air Gesture Syntax for Smart Space Interaction: An Empirical Study
by
Ana M. Bernardos, Xian Wang, Luca Bergesio, Juan A. Besada and José R. Casar
J. Sens. Actuator Netw. 2024, 13(2), 25; https://doi.org/10.3390/jsan13020025 - 09 Apr 2024
Abstract
Mid-gesture interfaces have become popular for specific scenarios, such as interactions with augmented reality via head-mounted displays, specific controls over smartphones, or gaming platforms. This article explores the use of a location-aware mid-air gesture-based command triplet syntax to interact with a smart space.
[...] Read more.
Mid-gesture interfaces have become popular for specific scenarios, such as interactions with augmented reality via head-mounted displays, specific controls over smartphones, or gaming platforms. This article explores the use of a location-aware mid-air gesture-based command triplet syntax to interact with a smart space. The syntax, inspired by human language, is built as a vocative case with an imperative structure. In a sentence like “Light, please switch on!”, the object being activated is invoked via making a gesture that mimics its initial letter/acronym (vocative, coincident with the sentence’s elliptical subject). A geometrical or directional gesture then identifies the action (imperative verb) and may include an object feature or a second object with which to network (complement), which also represented by the initial or acronym letter. Technically, an interpreter relying on a trainable multidevice gesture recognition layer makes the pair/triplet syntax decoding possible. The recognition layer works on acceleration and position input signals from graspable (smartphone) and free-hand devices (smartwatch and external depth cameras), as well as a specific compiler. On a specific deployment at a Living Lab facility, the syntax has been instantiated via the use of a lexicon derived from English (with respect to the initial letters and acronyms). A within-subject analysis with twelve users has enabled the analysis of the syntax acceptance (in terms of usability, gesture agreement for actions over objects, and social acceptance) and technology preference of the gesture syntax within its three device implementations (graspable, wearable, and device-free ones). Participants express consensus regarding the simplicity of learning the syntax and its potential effectiveness in managing smart resources. Socially, participants favoured the Watch for outdoor activities and the Phone for home and work settings, underscoring the importance of social context in technology design. The Phone emerged as the preferred option for gesture recognition due to its efficiency and familiarity. The system, which can be adapted to different sensing technologies, addresses the scalability concerns (as it can be easily extended for new objects and actions) and allows for personalised interaction.
Full article
(This article belongs to the Special Issue Machine-Environment Interaction, Volume II)
►▼
Show Figures
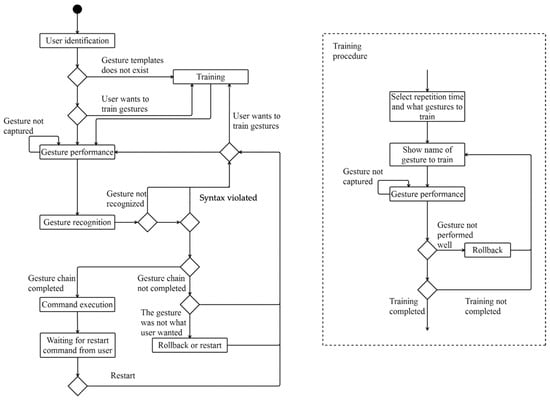
Figure 1
Open AccessArticle
Hybrid Adaptive Impedance and Admittance Control Based on the Sensorless Estimation of Interaction Joint Torque for Exoskeletons: A Case Study of an Upper Limb Rehabilitation Robot
by
Auwalu Muhammad Abdullahi, Ado Haruna and Ronnapee Chaichaowarat
J. Sens. Actuator Netw. 2024, 13(2), 24; https://doi.org/10.3390/jsan13020024 - 28 Mar 2024
Abstract
►▼
Show Figures
Physiotherapy is the treatment to recover a patient’s mobility and limb function after an injury, illness, or disability. Rehabilitation robots can be used to replace human physiotherapists. To ensure safety during robot physical therapy, the patient’s limb needs to be controlled to track
[...] Read more.
Physiotherapy is the treatment to recover a patient’s mobility and limb function after an injury, illness, or disability. Rehabilitation robots can be used to replace human physiotherapists. To ensure safety during robot physical therapy, the patient’s limb needs to be controlled to track a desired joint trajectory, and the torque due to interaction force/torque needs to be measured and regulated. Therefore, hybrid impedance and admittance with position control (HIPC) is required to track the trajectory and simultaneously regulate the contact torque. The literature describes two structures of HIPC: (1) a switched framework between admittance and impedance control operating in parallel (HIPCSW); and (2) a series connection between admittance and impedance control without switching. In this study, a hybrid adaptive impedance and position-based admittance control (HAIPC) in series is developed, which consists of a proportional derivative-based admittance position controller with gravitational torque compensation and an adaptive impedance controller. An extended state observer is used to estimate the interaction joint torque due to human stiff contact with the exoskeleton without the use of force/torque sensor, which is then used in the adaptive algorithm to update the stiffness and damping gains of the adaptive impedance controller. Simulation results obtained using MATLAB show that the proposed HAIPC significantly reduces the mean absolute values of the actuation torques (control inputs) required for the shoulder and elbow joints in comparison with HIPC and HIPCSW.
Full article
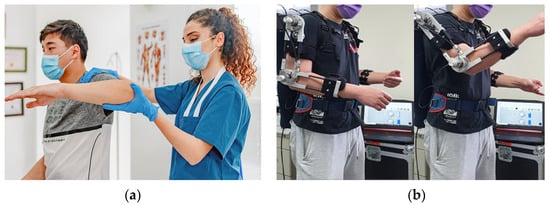
Figure 1
Open AccessArticle
Structured Data Ontology for AI in Industrial Asset Condition Monitoring
by
Jacob Hendriks, Mana Azarm and Patrick Dumond
J. Sens. Actuator Netw. 2024, 13(2), 23; https://doi.org/10.3390/jsan13020023 - 26 Mar 2024
Abstract
This paper proposes an ontology for prognostics and health management (PHM) applications involving sensor networks monitoring industrial machinery. Deep learning methods show promise for the development of autonomous PHM systems but require vast quantities of structured and representative data to realize their potential.
[...] Read more.
This paper proposes an ontology for prognostics and health management (PHM) applications involving sensor networks monitoring industrial machinery. Deep learning methods show promise for the development of autonomous PHM systems but require vast quantities of structured and representative data to realize their potential. PHM systems involve unique and specialized data characterized by time and context, and thus benefit from tailored data management systems. Furthermore, the use of dissimilar standards and practices with respect to database structure and data organization is a hinderance to interoperability. To address this, this paper presents a robust, structured data ontology and schema that is designed to accommodate a wide breadth of PHM applications. The inclusion of contextual and temporal data increases its value for developing and deploying enhanced ML-driven PHM systems. Challenges around balancing the competing priorities of structure and flexibility are discussed. The proposed schema provides the benefits of a relational schema with some provisions for noSQL-like flexibility in areas where PMH applications demand it. The selection of a database engine for implementation is also discussed, and the proposed ontology is demonstrated using a Postgres database. An instance of the database was loaded with large auto-generated fictitious data via multiple Python scripts. CRUD (create, read, update, delete) operations are demonstrated with several queries that answer common PHM questions.
Full article
(This article belongs to the Special Issue Fault Diagnosis in the Internet of Things Applications)
►▼
Show Figures
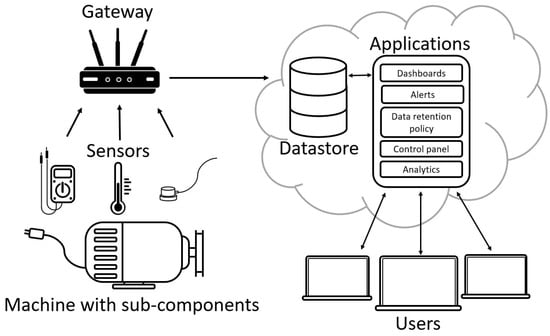
Figure 1
Open AccessArticle
An Optimized Link State Routing Protocol with a Blockchain Framework for Efficient Video-Packet Transmission and Security over Mobile Ad-Hoc Networks
by
Huda A. Ahmed and Hamid Ali Abed AL-Asadi
J. Sens. Actuator Netw. 2024, 13(2), 22; https://doi.org/10.3390/jsan13020022 - 11 Mar 2024
Abstract
A mobile ad-hoc network (MANET) necessitates appropriate routing techniques to enable optimal data transfer. The selection of appropriate routing protocols while utilizing the default settings is required to solve the existing problems. To enable effective video streaming in MANETs, this study proposes a
[...] Read more.
A mobile ad-hoc network (MANET) necessitates appropriate routing techniques to enable optimal data transfer. The selection of appropriate routing protocols while utilizing the default settings is required to solve the existing problems. To enable effective video streaming in MANETs, this study proposes a novel optimized link state routing (OLSR) protocol that incorporates a deep-learning model. Initially, the input videos are collected from the Kaggle dataset. Then, the black-hole node is detected using a novel twin-attention-based dense convolutional bidirectional gated network (SA_ DCBiGNet) model. Next, the neighboring nodes are analyzed using trust values, and routing is performed using the extended osprey-aided optimized link state routing protocol (EO_OLSRP) technique. Similarly, the extended osprey optimization algorithm (EOOA) selects the optimal feature based on parameters such as node stability and link stability. Finally, blockchain storage is included to improve the security of MANET data using interplanetary file system (IPFS) technology. Additionally, the proposed blockchain system is validated utilizing a consensus technique based on delegated proof-of-stake (DPoS). The proposed method utilizes Python and it is evaluated using data acquired from various mobile simulator models accompanied by the NS3 simulator. The proposed model performs better with a packet-delivery ratio (PDR) of 91.6%, average end delay (AED) of 23.6 s, and throughput of 2110 bytes when compared with the existing methods which have a PDR of 89.1%, AED of 22 s, and throughput of 1780 bytes, respectively.
Full article
(This article belongs to the Section Network Security and Privacy)
►▼
Show Figures
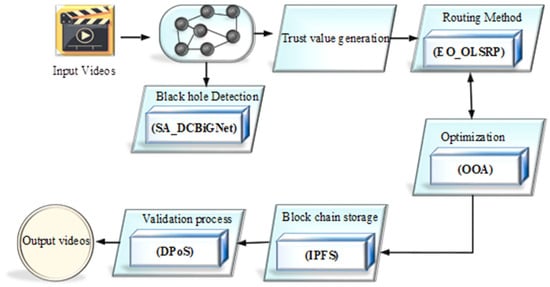
Figure 1
Open AccessArticle
Veritas: Layer-2 Scaling Solution for Decentralized Oracles on Ethereum Blockchain with Reputation and Real-Time Considerations
by
Moustafa Mowaffak Saad, Dalia Sobhy and Amani A. Saad
J. Sens. Actuator Netw. 2024, 13(2), 21; https://doi.org/10.3390/jsan13020021 - 07 Mar 2024
Abstract
►▼
Show Figures
Blockchainsand smart contracts are pivotal in transforming interactions between systems and individuals, offering secure, immutable, and transparent trust-building mechanisms without central oversight. However, Smart Contracts face limitations due to their reliance on blockchain-contained data, a gap addressed by ’Oracles’. These bridges to external
[...] Read more.
Blockchainsand smart contracts are pivotal in transforming interactions between systems and individuals, offering secure, immutable, and transparent trust-building mechanisms without central oversight. However, Smart Contracts face limitations due to their reliance on blockchain-contained data, a gap addressed by ’Oracles’. These bridges to external data sources introduce the ’Oracle problem’, where maintaining blockchain-like security and transparency becomes vital to prevent data integrity issues. This paper presents Veritas, a novel decentralized oracle system leveraging a layer-2 scaling solution, enhancing smart contracts’ efficiency and security on Ethereum blockchains. The proposed architecture, explored through simulation and experimental analyses, significantly reduces operational costs while maintaining robust security protocols. An innovative node selection process is also introduced to minimize the risk of malicious data entry, thereby reinforcing network security. Veritas offers a solution to the Oracle problem by aligning with blockchain principles of security and transparency, and demonstrates advancements in reducing operational costs and bolstering network integrity. While the study provides a promising direction, it also highlights potential areas for further exploration in blockchain technology and oracle system optimization.
Full article
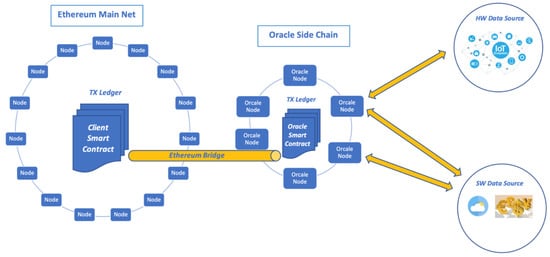
Figure 1
Open AccessReview
Interconnected Smart Transactive Microgrids—A Survey on Trading, Energy Management Systems, and Optimisation Approaches
by
Ipeleng L. Machele, Adeiza J. Onumanyi, Adnan M. Abu-Mahfouz and Anish M. Kurien
J. Sens. Actuator Netw. 2024, 13(2), 20; https://doi.org/10.3390/jsan13020020 - 01 Mar 2024
Abstract
The deployment of isolated microgrids has witnessed exponential growth globally, especially in the light of prevailing challenges faced by many larger power grids. However, these isolated microgrids remain separate entities, thus limiting their potential to significantly impact and improve the stability, efficiency, and
[...] Read more.
The deployment of isolated microgrids has witnessed exponential growth globally, especially in the light of prevailing challenges faced by many larger power grids. However, these isolated microgrids remain separate entities, thus limiting their potential to significantly impact and improve the stability, efficiency, and reliability of the broader electrical power system. Thus, to address this gap, the concept of interconnected smart transactive microgrids (ISTMGs) has arisen, facilitating the interconnection of these isolated microgrids, each with its unique attributes aimed at enhancing the performance of the broader power grid system. Furthermore, ISTMGs are expected to create more robust and resilient energy networks that enable innovative and efficient mechanisms for energy trading and sharing between individual microgrids and the centralized power grid. This paradigm shift has sparked a surge in research aimed at developing effective ISTMG networks and mechanisms. Thus, in this paper, we present a review of the current state-of-the-art in ISTMGs with a focus on energy trading, energy management systems (EMS), and optimization techniques for effective energy management in ISTMGs. We discuss various types of trading, architectures, platforms, and stakeholders involved in ISTMGs. We proceed to elucidate the suitable applications of EMS within such ISTMG frameworks, emphasizing its utility in various domains. This includes an examination of optimization tools and methodologies for deploying EMS in ISTMGs. Subsequently, we conduct an analysis of current techniques and their constraints, and delineate prospects for future research to advance the establishment and utilization of ISTMGs.
Full article
(This article belongs to the Section Network Services and Applications)
►▼
Show Figures
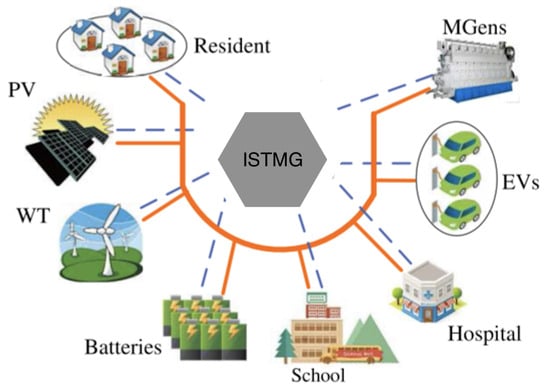
Figure 1
Open AccessArticle
Low-Cost Internet of Things Solution for Building Information Modeling Level 3B—Monitoring, Analysis and Management
by
Andrzej Szymon Borkowski
J. Sens. Actuator Netw. 2024, 13(2), 19; https://doi.org/10.3390/jsan13020019 - 29 Feb 2024
Abstract
►▼
Show Figures
The integration of the Internet of Things (IoT) and Building Information Modeling (BIM) is progressing. The use of microcontrollers and sensors in buildings is described as a level 3B maturity in the use of BIM. Design companies, contractors and building operators can use
[...] Read more.
The integration of the Internet of Things (IoT) and Building Information Modeling (BIM) is progressing. The use of microcontrollers and sensors in buildings is described as a level 3B maturity in the use of BIM. Design companies, contractors and building operators can use IoT solutions to monitor, analyze or manage processes. As a rule, solutions based on original Arduino boards are quite an expensive investment. The aim of this research was to find a low-cost IoT solution for monitoring, analysis and management, and integrate it with a BIM model. In the present study, an inexpensive NodeMCU microcontroller and a temperature and pressure sensor were used to study the thermal comfort of users in a single-family home. During the summer season, analysis of the monitored temperature can contribute to installation (HVAC) or retrofit work (for energy efficiency). The article presents a low-cost solution for studying the thermal comfort of users using a digital twin built-in BIM. Data obtained from sensors can support both the design and management processes. The main contribution of the article enables the design, construction and use of low-cost circuits (15.57 USD) even in small developments (single-family houses, semi-detached houses, terraced houses, atrium buildings). Combining IoT sensor telemetry with BIM (maturity level 3C) is a challenge that organizations will face in the near future.
Full article
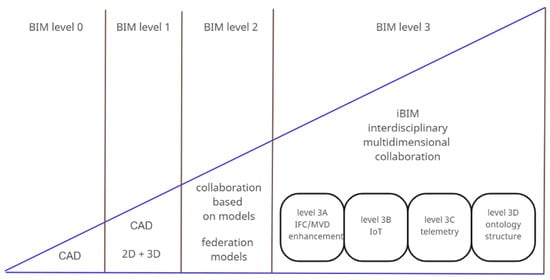
Figure 1
Open AccessFeature PaperArticle
UAV-Assisted Cooperative NOMA and OFDM Communication Systems: Analysis and Optimization
by
Thuc Kieu-Xuan and Anh Le-Thi
J. Sens. Actuator Netw. 2024, 13(1), 18; https://doi.org/10.3390/jsan13010018 - 19 Feb 2024
Abstract
►▼
Show Figures
Utilizing unmanned aerial vehicles (UAVs) to facilitate wireless communication has emerged as a viable and promising strategy to enhance current and prospective wireless systems. This approach offers many advantages by establishing line-of-sight connections, optimizing operational efficiency, and enabling flexible deployment capabilities in various
[...] Read more.
Utilizing unmanned aerial vehicles (UAVs) to facilitate wireless communication has emerged as a viable and promising strategy to enhance current and prospective wireless systems. This approach offers many advantages by establishing line-of-sight connections, optimizing operational efficiency, and enabling flexible deployment capabilities in various terrains. Thus, in this paper, we investigate UAV communication in a relaying network in which a UAV helps communication between a source and two destination users while flying to a location. To have a complete view of our proposed system, we consider both orthogonal multiple access, such as OFDMs and non-orthogonal multiple access (NOMA) scenarios. Moreover, we apply successive convex optimization (SCO) and the block-coordinate gradient descent (BCGD) for the sum-rate optimization problems to improve the system performance under constraints of total bandwidth and total power at the ground base station and UAV. The experimental results validate that the achievable secrecy rates are notably enhanced using our proposed algorithms and show optimal trends for critical parameters, such as transmit powers, the flight trajectory and speed of the UAV, and resource allocation of OFDM and NOMA.
Full article
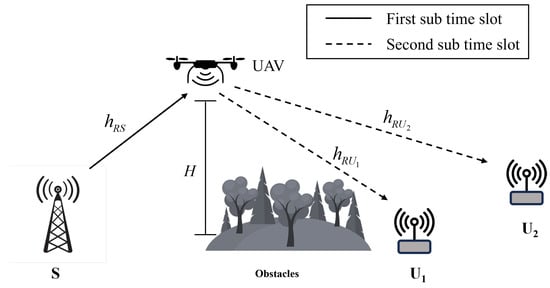
Figure 1
Open AccessArticle
Build–Launch–Consolidate Framework and Toolkit for Impact Analysis on Wireless Sensor Networks
by
Rakan Alghofaili, Hussah Albinali and Farag Azzedin
J. Sens. Actuator Netw. 2024, 13(1), 17; https://doi.org/10.3390/jsan13010017 - 18 Feb 2024
Abstract
►▼
Show Figures
The Internet of Things (IoT) and wireless sensor networks (WSNs) utilize their connectivity to enable solutions supporting a spectrum of industries in different and volatile environments. To effectively enhance the security and quality of the service of networks, empirical research should consider a
[...] Read more.
The Internet of Things (IoT) and wireless sensor networks (WSNs) utilize their connectivity to enable solutions supporting a spectrum of industries in different and volatile environments. To effectively enhance the security and quality of the service of networks, empirical research should consider a variety of factors and be reproducible. This will not only ensure scalability but also enable the verification of conclusions, leading to more reliable solutions. Cooja offers limited performance analysis capabilities of simulations, which are often extracted and calculated manually. In this paper, we introduce the Build–Launch–Consolidate (BLC) framework and a toolkit that enable researchers to conduct structured and conclusive experiments considering different factors and metrics, experiment design, and results analysis. Furthermore, the toolkit analyzes diverse network metrics across various scenarios. As a proof of concept, this paper studies the flooding attacks on the IoT and illustrates their impact on the network, utilizing the BLC framework and toolkit.
Full article
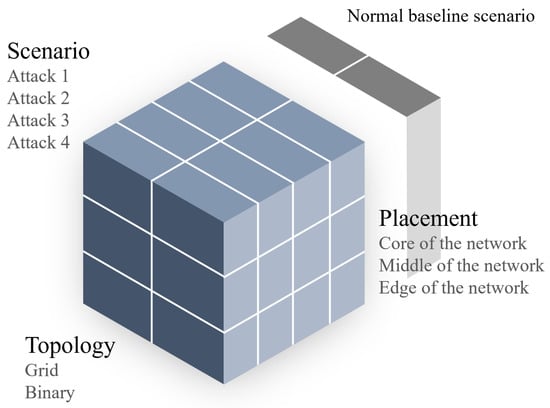
Figure 1
Open AccessReview
Reliability of LoRaWAN Communications in Mining Environments: A Survey on Challenges and Design Requirements
by
Sonile K. Musonda, Musa Ndiaye, Hastings M. Libati and Adnan M. Abu-Mahfouz
J. Sens. Actuator Netw. 2024, 13(1), 16; https://doi.org/10.3390/jsan13010016 - 09 Feb 2024
Abstract
While a robust and reliable communication network for monitoring the mining environment in a timely manner to take care of people, the planet Earth and profits is key, the mining environment is very challenging in terms of achieving reliable wireless transmission. This survey
[...] Read more.
While a robust and reliable communication network for monitoring the mining environment in a timely manner to take care of people, the planet Earth and profits is key, the mining environment is very challenging in terms of achieving reliable wireless transmission. This survey therefore investigates the reliability of LoRaWAN communication in the mining environment, identifying the challenges and design requirements. Bearing in mind that LoRaWAN is an IoT communication technology that has not yet been fully deployed in mining, the survey incorporates an investigation of LoRaWAN and other mining IoT communication technologies to determine their records of reliability, strengths and weaknesses and applications in mining. This aspect of the survey gives insight into the requirements of future mining IoT communication technologies and where LoRaWAN can be deployed in both underground and surface mining. Specific questions that the survey addresses are: (1) What is the record of reliability of LoRaWAN in mining environments? (2) What contributions have been made with regard to LoRa/LoRaWAN communication in general towards improving reliability? (3) What are the challenges and design requirements of LoRaWAN reliability in mining environments? (4) What research opportunities exist for achieving LoRaWAN communication in mining environments? In addition to recommending open research opportunities, the lessons learnt from the survey are also outlined.
Full article
(This article belongs to the Topic Electronic Communications, IOT and Big Data)
►▼
Show Figures
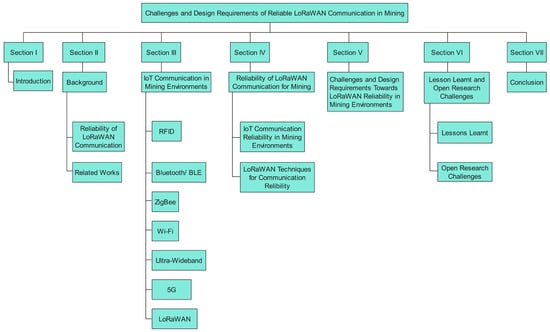
Figure 1
Open AccessArticle
IFGAN—A Novel Image Fusion Model to Fuse 3D Point Cloud Sensory Data
by
Henry Alexander Ignatious, Hesham El-Sayed and Salah Bouktif
J. Sens. Actuator Netw. 2024, 13(1), 15; https://doi.org/10.3390/jsan13010015 - 07 Feb 2024
Abstract
►▼
Show Figures
To enhance the level of autonomy in driving, it is crucial to ensure optimal execution of critical maneuvers in all situations. However, numerous accidents involving autonomous vehicles (AVs) developed by major automobile manufacturers in recent years have been attributed to poor decision making
[...] Read more.
To enhance the level of autonomy in driving, it is crucial to ensure optimal execution of critical maneuvers in all situations. However, numerous accidents involving autonomous vehicles (AVs) developed by major automobile manufacturers in recent years have been attributed to poor decision making caused by insufficient perception of environmental information. AVs employ diverse sensors in today’s technology-driven settings to gather this information. However, due to technical and natural factors, the data collected by these sensors may be incomplete or ambiguous, leading to misinterpretation by AVs and resulting in fatal accidents. Furthermore, environmental information obtained from multiple sources in the vehicular environment often exhibits multimodal characteristics. To address this limitation, effective preprocessing of raw sensory data becomes essential, involving two crucial tasks: data cleaning and data fusion. In this context, we propose a comprehensive data fusion engine that categorizes various sensory data formats and appropriately merges them to enhance accuracy. Specifically, we suggest a general framework to combine audio, visual, and textual data, building upon our previous research on an innovative hybrid image fusion model that fused multispectral image data. However, this previous model faced challenges when fusing 3D point cloud data and handling large volumes of sensory data. To overcome these challenges, our study introduces a novel image fusion model called Image Fusion Generative Adversarial Network (IFGAN), which incorporates a multi-scale attention mechanism into both the generator and discriminator of a Generative Adversarial Network (GAN). The primary objective of image fusion is to merge complementary data from various perspectives of the same scene to enhance the clarity and detail of the final image. The multi-scale attention mechanism serves two purposes: the first, capturing comprehensive spatial information to enable the generator to focus on foreground and background target information in the sensory data, and the second, constraining the discriminator to concentrate on attention regions rather than the entire input image. Furthermore, the proposed model integrates the color information retention concept from the previously proposed image fusion model. Furthermore, we propose simple and efficient models for extracting salient image features. We evaluate the proposed models using various standard metrics and compare them with existing popular models. The results demonstrate that our proposed image fusion model outperforms the other models in terms of performance.
Full article
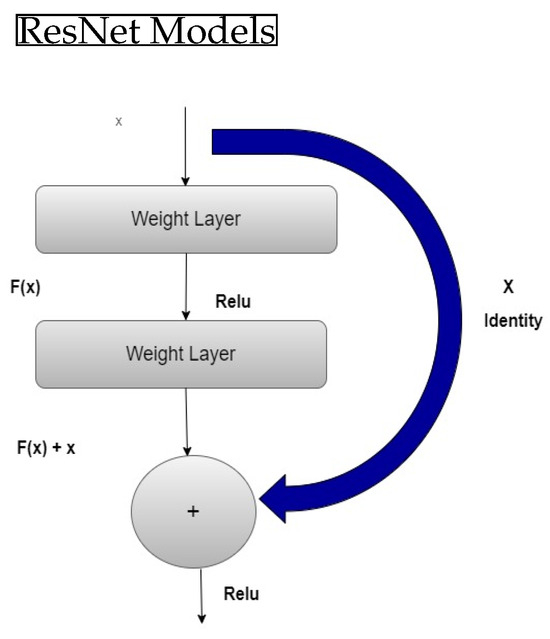
Figure 1
Open AccessArticle
Network Sliced Distributed Learning-as-a-Service for Internet of Vehicles Applications in 6G Non-Terrestrial Network Scenarios
by
David Naseh, Swapnil Sadashiv Shinde and Daniele Tarchi
J. Sens. Actuator Netw. 2024, 13(1), 14; https://doi.org/10.3390/jsan13010014 - 07 Feb 2024
Cited by 1
Abstract
In the rapidly evolving landscape of next-generation 6G systems, the integration of AI functions to orchestrate network resources and meet stringent user requirements is a key focus. Distributed Learning (DL), a promising set of techniques that shape the future of 6G communication systems,
[...] Read more.
In the rapidly evolving landscape of next-generation 6G systems, the integration of AI functions to orchestrate network resources and meet stringent user requirements is a key focus. Distributed Learning (DL), a promising set of techniques that shape the future of 6G communication systems, plays a pivotal role. Vehicular applications, representing various services, are likely to benefit significantly from the advances of 6G technologies, enabling dynamic management infused with inherent intelligence. However, the deployment of various DL methods in traditional vehicular settings with specific demands and resource constraints poses challenges. The emergence of distributed computing and communication resources, such as the edge-cloud continuum and integrated terrestrial and non-terrestrial networks (T/NTN), provides a solution. Efficiently harnessing these resources and simultaneously implementing diverse DL methods becomes crucial, and Network Slicing (NS) emerges as a valuable tool. This study delves into the analysis of DL methods suitable for vehicular environments alongside NS. Subsequently, we present a framework to facilitate DL-as-a-Service (DLaaS) on a distributed networking platform, empowering the proactive deployment of DL algorithms. This approach allows for the effective management of heterogeneous services with varying requirements. The proposed framework is exemplified through a detailed case study in a vehicular integrated T/NTN with diverse service demands from specific regions. Performance analysis highlights the advantages of the DLaaS approach, focusing on flexibility, performance enhancement, added intelligence, and increased user satisfaction in the considered T/NTN vehicular scenario.
Full article
(This article belongs to the Special Issue Advancing towards 6G Networks)
►▼
Show Figures
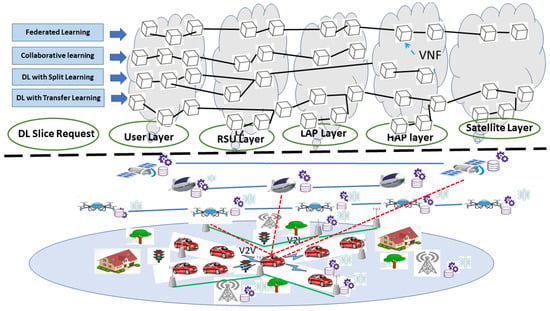
Figure 1
Open AccessArticle
A Secure Blockchain-Enabled Remote Healthcare Monitoring System for Home Isolation
by
Jongsuk Kongsen, Doungsuda Chantaradsuwan, Peeravit Koad, May Thu and Chanankorn Jandaeng
J. Sens. Actuator Netw. 2024, 13(1), 13; https://doi.org/10.3390/jsan13010013 - 05 Feb 2024
Abstract
This article presents a secure framework for remote healthcare monitoring in the context of home isolation, thereby addressing the concerns related to untrustworthy client connections to a hospital information system (HIS) within a secure network. Our proposed solution leverages a public blockchain network
[...] Read more.
This article presents a secure framework for remote healthcare monitoring in the context of home isolation, thereby addressing the concerns related to untrustworthy client connections to a hospital information system (HIS) within a secure network. Our proposed solution leverages a public blockchain network as a secure distributed database to buffer and transmit patient vital signs. The framework integrates an algorithm for the secure gathering and transmission of vital signs to the Ethereum network. Additionally, we introduce a publish/subscribe paradigm, thus enhancing security using the TLS channel to connect to the blockchain network. An analysis of the maintenance cost of the distributed database underscores the cost-effectiveness of our approach. In conclusion, our framework provides a highly secure and economical solution for remote healthcare monitoring in home isolation scenarios.
Full article
(This article belongs to the Section Actuators, Sensors and Devices)
►▼
Show Figures
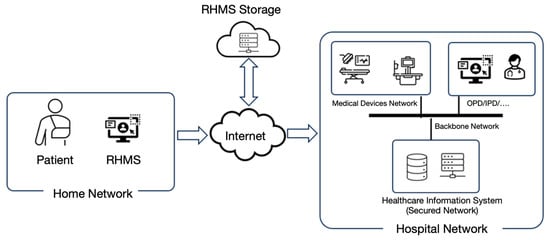
Figure 1
Open AccessArticle
Fast Multi-User Searchable Encryption with Forward and Backward Private Access Control
by
Salim Sabah Bulbul, Zaid Ameen Abduljabbar, Duaa Fadhel Najem, Vincent Omollo Nyangaresi, Junchao Ma and Abdulla J. Y. Aldarwish
J. Sens. Actuator Netw. 2024, 13(1), 12; https://doi.org/10.3390/jsan13010012 - 02 Feb 2024
Abstract
Untrusted servers are servers or storage entities lacking complete trust from the data owner or users. This characterization implies that the server hosting encrypted data may not enjoy full trust from data owners or users, stemming from apprehensions related to potential security breaches,
[...] Read more.
Untrusted servers are servers or storage entities lacking complete trust from the data owner or users. This characterization implies that the server hosting encrypted data may not enjoy full trust from data owners or users, stemming from apprehensions related to potential security breaches, unauthorized access, or other security risks. The security of searchable encryption has been put into question by several recent attacks. Currently, users can search for encrypted documents on untrusted cloud servers using searchable symmetric encryption (SSE). This study delves deeply into two pivotal concepts of privacy within dynamic searchable symmetric encryption (DSSE) schemes: forward privacy and backward privacy. The former serves as a safeguard against the linkage of recently added documents to previously conducted search queries, whereas the latter guarantees the irretrievability of deleted documents in subsequent search inquiries. However, the provision of fine-grained access control is complex in existing multi-user SSE schemes. SSE schemes may also incur high computation costs due to the need for fine-grained access control, and it is essential to support document updates and forward privacy. In response to these issues, this paper suggests a searchable encryption scheme that uses simple primitive tools. We present a multi-user SSE scheme that efficiently controls access to dynamically encrypted documents to resolve these issues, using an innovative approach that readily enhances previous findings. Rather than employing asymmetric encryption as in comparable systems, we harness low-complexity primitive encryption tools and inverted index-based DSSE to handle retrieving encrypted files, resulting in a notably faster system. Furthermore, we ensure heightened security by refreshing the encryption key after each search, meaning that users are unable to conduct subsequent searches with the same key and must obtain a fresh key from the data owner. An experimental evaluation shows that our scheme achieves forward and Type II backward privacy and has much faster search performance than other schemes. Our scheme can be considered secure, as proven in a random oracle model.
Full article
(This article belongs to the Special Issue Security and Smart Applications in IoT and Wireless Sensor and Actuator Networks)
►▼
Show Figures
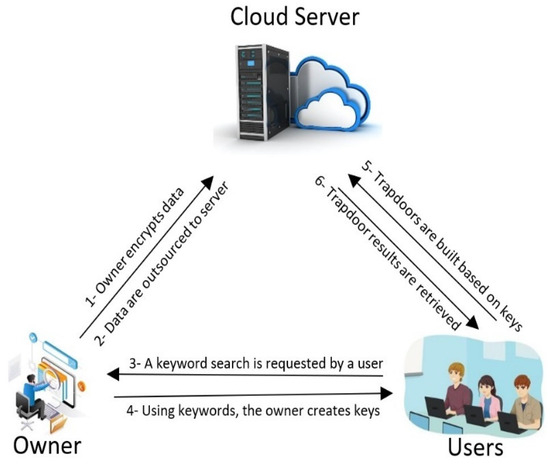
Figure 1
Open AccessEditorial
Featured Papers on Network Security and Privacy
by
Jordi Mongay Batalla
J. Sens. Actuator Netw. 2024, 13(1), 11; https://doi.org/10.3390/jsan13010011 - 01 Feb 2024
Abstract
There is an urgent need to introduce security-by-design in networks [...]
Full article
(This article belongs to the Special Issue Feature Papers in Network Security and Privacy)
Open AccessArticle
Service-Aware Hierarchical Fog–Cloud Resource Mappingfor e-Health with Enhanced-Kernel SVM
by
Alaa AlZailaa, Hao Ran Chi, Ayman Radwan and Rui L. Aguiar
J. Sens. Actuator Netw. 2024, 13(1), 10; https://doi.org/10.3390/jsan13010010 - 01 Feb 2024
Abstract
Fog–cloud-based hierarchical task-scheduling methods are embracing significant challenges to support e-Health applications due to the large number of users, high task diversity, and harsher service-level requirements. Addressing the challenges of fog–cloud integration, this paper proposes a new service/network-aware fog–cloud hierarchical resource-mapping scheme, which
[...] Read more.
Fog–cloud-based hierarchical task-scheduling methods are embracing significant challenges to support e-Health applications due to the large number of users, high task diversity, and harsher service-level requirements. Addressing the challenges of fog–cloud integration, this paper proposes a new service/network-aware fog–cloud hierarchical resource-mapping scheme, which achieves optimized resource utilization efficiency and minimized latency for service-level critical tasks in e-Health applications. Concretely, we develop a service/network-aware task classification algorithm. We adopt support vector machine as a backbone with fast computational speed to support real-time task scheduling, and we develop a new kernel, fusing convolution, cross-correlation, and auto-correlation, to gain enhanced specificity and sensitivity. Based on task classification, we propose task priority assignment and resource-mapping algorithms, which aim to achieve minimized overall latency for critical tasks and improve resource utilization efficiency. Simulation results showcase that the proposed algorithm is able to achieve average execution times for critical/non-critical tasks of 0.23/0.50 ms in diverse networking setups, which surpass the benchmark scheme by 73.88%/52.01%, respectively.
Full article
(This article belongs to the Topic Machine Learning in Communication Systems and Networks)
►▼
Show Figures
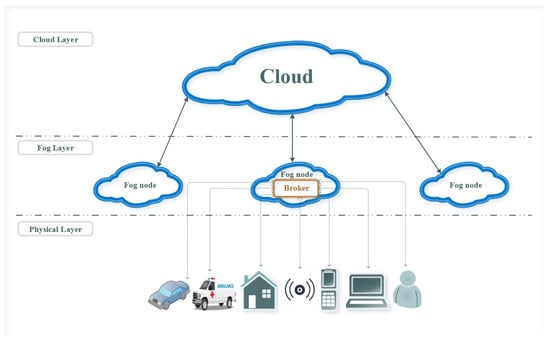
Figure 1
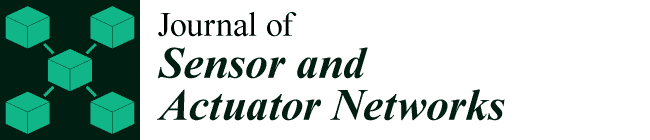
Journal Menu
► ▼ Journal Menu-
- JSAN Home
- Aims & Scope
- Editorial Board
- Reviewer Board
- Topical Advisory Panel
- Instructions for Authors
- Special Issues
- Topics
- Sections
- Article Processing Charge
- Indexing & Archiving
- Editor’s Choice Articles
- Most Cited & Viewed
- Journal Statistics
- Journal History
- Journal Awards
- Conferences
- Editorial Office
Journal Browser
► ▼ Journal BrowserHighly Accessed Articles
Latest Books
E-Mail Alert
News
Topics
Topic in
Coatings, Electronics, JSAN, Nanomaterials, Sensors
Modeling, Fabrication, and Characterization of Semiconductor Materials and Devices
Topic Editors: Andrei Avram, Ana-Maria Lepadatu, Florin Nastase, Martino AldrigoDeadline: 15 May 2024
Topic in
Applied Sciences, Computers, Electronics, JSAN, Technologies
Emerging AI+X Technologies including Selected Papers from ICGHIT 2024
Topic Editors: Byung-Seo Kim, Hyunsik Ahn, Kyu-Tae LeeDeadline: 31 October 2024
Topic in
Applied Sciences, Electronics, IoT, JSAN, Network, Sensors, Telecom, Technologies
Wireless Energy Harvesting and Power Transfer for Communications and Networks
Topic Editors: Yichuang Sun, Arooj Mubashara Siddiqui, Xiaojing Chen, Oluyomi SimpsonDeadline: 31 December 2024
Topic in
Applied Sciences, Electronics, JSAN, Photonics, Sensors, Telecom
Machine Learning in Communication Systems and Networks, 2nd Edition
Topic Editors: Yichuang Sun, Haeyoung Lee, Oluyomi SimpsonDeadline: 20 July 2025

Conferences
Special Issues
Special Issue in
JSAN
Recent Trends and Advancements in Location Fingerprinting
Guest Editors: Cristiano Pendão, Ivo Silva, Jianwei NiuDeadline: 31 July 2024
Special Issue in
JSAN
Security and Smart Applications in IoT and Wireless Sensor and Actuator Networks
Guest Editors: Donald Elmazi, Elis KullaDeadline: 25 November 2024