Topic Editors
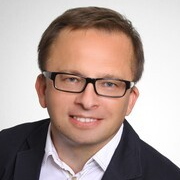
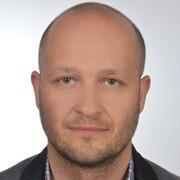
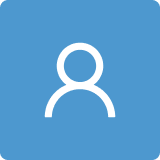
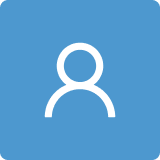
AI and Computational Methods for Modelling, Simulations and Optimizing of Advanced Systems: Innovations in Complexity
Topic Information
Dear Colleagues,
Due to the increasing computational capability of current data processing systems, new opportunities emerge in the modelling, simulations and optimization of complex systems and devices. Difficult-to-apply, highly demanding and time-consuming methods may now be considered when developing complete and sophisticated models in many areas of science and technology. Combining AI algorithms and computational methods, including numerical and other methods, allows for conducting multi-threaded analyses to solve advanced and interdisciplinary problems.
This article collection aims to bring together research on advances in the modelling, simulations and optimization issues of complex systems, considering the great interest received for the part I of the topic.
Original research, review articles and short communications focusing on (but not limited to) artificial intelligence and other computational methods are welcome.
Prof. Dr. Jaroslaw Krzywanski
Dr. Marcin Sosnowski
Dr. Karolina Grabowska
Dr. Dorian Skrobek
Dr. Ghulam Moeen Uddin
Topic Editors
Keywords
- artificial intelligence
- artificial neural networks
- deep learning
- genetic and evolutionary algorithms
- artificial immune systems
- fuzzy logic
- information theory
- expert systems
- bio-inspired methods
- CFD
- fractal and fractional problems
- fractional and fractal dynamics
- functional analysis
- quantum mechanics
- micro and nano-mechanics
- fluidics and nano-fluidics
- modelling
- simulation
- optimization
- complex systems
Participating Journals
Journal Name | Impact Factor | CiteScore | Launched Year | First Decision (median) | APC | |
---|---|---|---|---|---|---|
![]()
Algorithms
|
1.8 | 4.1 | 2008 | 15 Days | CHF 1600 | Submit |
![]()
Energies
|
3.0 | 6.2 | 2008 | 17.5 Days | CHF 2600 | Submit |
![]()
Entropy
|
2.1 | 4.9 | 1999 | 22.4 Days | CHF 2600 | Submit |
![]()
Fractal and Fractional
|
3.6 | 4.6 | 2017 | 20.9 Days | CHF 2700 | Submit |
![]()
Machine Learning and Knowledge Extraction
|
4.0 | 6.3 | 2019 | 27.1 Days | CHF 1800 | Submit |
![]()
Materials
|
3.1 | 5.8 | 2008 | 15.5 Days | CHF 2600 | Submit |
MDPI Topics is cooperating with Preprints.org and has built a direct connection between MDPI journals and Preprints.org. Authors are encouraged to enjoy the benefits by posting a preprint at Preprints.org prior to publication:
- Immediately share your ideas ahead of publication and establish your research priority;
- Protect your idea from being stolen with this time-stamped preprint article;
- Enhance the exposure and impact of your research;
- Receive feedback from your peers in advance;
- Have it indexed in Web of Science (Preprint Citation Index), Google Scholar, Crossref, SHARE, PrePubMed, Scilit and Europe PMC.