-
A Novel Method to Compute the Contact Surface Area Between an Organ and Cancer Tissue
-
Sowing, Monitoring, Detecting: A Possible Solution to Improve the Visibility of Cropmarks in Cultivated Fields
-
A Method for Estimating Fluorescence Emission Spectra from the Image Data of Plant Grain and Leaves Without a Spectrometer
-
SAVE: Self-Attention on Visual Embedding for Zero-Shot Generic Object Counting
Journal Description
Journal of Imaging
Journal of Imaging
is an international, multi/interdisciplinary, peer-reviewed, open access journal of imaging techniques published online monthly by MDPI.
- Open Access— free for readers, with article processing charges (APC) paid by authors or their institutions.
- High Visibility: indexed within Scopus, ESCI (Web of Science), PubMed, PMC, dblp, Inspec, Ei Compendex, and other databases.
- Journal Rank: CiteScore - Q1 (Computer Graphics and Computer-Aided Design)
- Rapid Publication: manuscripts are peer-reviewed and a first decision is provided to authors approximately 18.3 days after submission; acceptance to publication is undertaken in 3.3 days (median values for papers published in this journal in the second half of 2024).
- Recognition of Reviewers: reviewers who provide timely, thorough peer-review reports receive vouchers entitling them to a discount on the APC of their next publication in any MDPI journal, in appreciation of the work done.
Impact Factor:
2.7 (2023);
5-Year Impact Factor:
3.0 (2023)
Latest Articles
Noise Suppressed Image Reconstruction for Quanta Image Sensors Based on Transformer Neural Networks
J. Imaging 2025, 11(5), 160; https://doi.org/10.3390/jimaging11050160 (registering DOI) - 17 May 2025
Abstract
The photon detection capability of quanta image sensors make them an optimal choice for low-light imaging. To address Possion noise in QIS reconstruction caused by spatio-temporal oversampling characteristic, a deep learning-based noise suppression reconstruction method is proposed in this paper. The proposed neural
[...] Read more.
The photon detection capability of quanta image sensors make them an optimal choice for low-light imaging. To address Possion noise in QIS reconstruction caused by spatio-temporal oversampling characteristic, a deep learning-based noise suppression reconstruction method is proposed in this paper. The proposed neural network integrates convolutional neural networks and Transformers. Its architecture combines the Anscombe transformation with serial and parallel modules to enhance denoising performance and adaptability across various scenarios. Experimental results demonstrate that the proposed method effectively suppresses noise in QIS image reconstruction. Compared with representative methods such as TD-BM3D, QIS-Net and DPIR, our approach achieves up to 1.2 dB improvement in PSNR, demonstrating superior reconstruction quality.
Full article
(This article belongs to the Section Image and Video Processing)
►
Show Figures
Open AccessArticle
A Transfer Learning-Based VGG-16 Model for COD Detection in UV–Vis Spectroscopy
by
Jingwei Li, Iqbal Muhammad Tauqeer, Zhiyu Shao and Haidong Yu
J. Imaging 2025, 11(5), 159; https://doi.org/10.3390/jimaging11050159 (registering DOI) - 17 May 2025
Abstract
Chemical oxygen demand (COD) serves as a key indicator of organic pollution in water bodies, and its rapid and accurate detection is crucial for environmental protection. Recently, ultraviolet–visible (UV–Vis) spectroscopy has gained popularity for COD detection due to its convenience and the absence
[...] Read more.
Chemical oxygen demand (COD) serves as a key indicator of organic pollution in water bodies, and its rapid and accurate detection is crucial for environmental protection. Recently, ultraviolet–visible (UV–Vis) spectroscopy has gained popularity for COD detection due to its convenience and the absence of chemical reagents. Meanwhile, deep learning has emerged as an effective approach for automatically extracting spectral features and predicting COD. This paper proposes transforming one-dimensional spectra into two-dimensional spectrum images and employing convolutional neural networks (CNNs) to extract features and model automatically. However, training such deep learning models requires a vast dataset of water samples, alongside the complex task of labeling this data. To address these challenges, we introduce a transfer learning model based on VGG-16 for spectrum images. In this approach, parameters in the initial layers of the model are frozen, while those in the later layers are fine-tuned with the spectrum images. The effectiveness of this method is demonstrated through experiments conducted on our dataset, where the results indicate that it significantly enhances the accuracy of COD prediction compared to traditional methods and other deep learning methods such as partial least squares regression (PLSR), support vector machine (SVM), artificial neural network (ANN), and CNN-based methods.
Full article
(This article belongs to the Section Image and Video Processing)
►▼
Show Figures
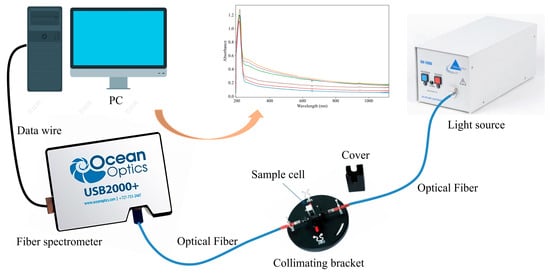
Figure 1
Open AccessArticle
Real-Time Far-Field BCSDF Filtering
by
Junjie Wei and Ying Song
J. Imaging 2025, 11(5), 158; https://doi.org/10.3390/jimaging11050158 (registering DOI) - 16 May 2025
Abstract
The real-time rendering of large-scale curve-based surfaces (e.g., hair, fabrics) requires efficient handling of bidirectional curve-scattering distribution functions (BCSDFs). While curve-based material models are essential for capturing anisotropic reflectance characteristics, conventional prefiltering techniques encounter challenges in jointly resolving micro-scale BCSDFs variations with tangent
[...] Read more.
The real-time rendering of large-scale curve-based surfaces (e.g., hair, fabrics) requires efficient handling of bidirectional curve-scattering distribution functions (BCSDFs). While curve-based material models are essential for capturing anisotropic reflectance characteristics, conventional prefiltering techniques encounter challenges in jointly resolving micro-scale BCSDFs variations with tangent distribution functions (TDFs) at pixel-level accuracy. This paper presents a real-time BCSDF filtering framework that achieves high-fidelity rendering without precomputation. Our key insight lies in formulating each pixel’s scattering response as a mixture of von Mises–Fisher (vMF) distributions, enabling analytical convolution between micro-scale BCSDFs and TDFs. Furthermore, we derive closed-form expressions for the integral of TDF-BCSDF products, avoiding the need for numerical approximation and heavy precomputation. Our method demonstrates state-of-the-art performance, achieving results comparable to 1000 spp Monte Carlo simulations under parallax-free conditions, where it improves the mean squared error (MSE) by one to two orders of magnitude over baseline methods. Qualitative comparisons and error analysis confirm both visual fidelity and computational efficiency.
Full article
(This article belongs to the Section Visualization and Computer Graphics)
►▼
Show Figures
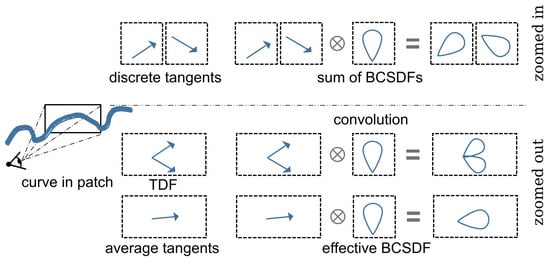
Figure 1
Open AccessArticle
Learning AI-Driven Automated Blood Cell Anomaly Detection: Enhancing Diagnostics and Telehealth in Hematology
by
Sami Naouali and Oussama El Othmani
J. Imaging 2025, 11(5), 157; https://doi.org/10.3390/jimaging11050157 - 16 May 2025
Abstract
Hematology plays a critical role in diagnosing and managing a wide range of blood-related disorders. The manual interpretation of blood smear images, however, is time-consuming and highly dependent on expert availability. Moreover, it is particularly challenging in remote and resource-limited settings. In this
[...] Read more.
Hematology plays a critical role in diagnosing and managing a wide range of blood-related disorders. The manual interpretation of blood smear images, however, is time-consuming and highly dependent on expert availability. Moreover, it is particularly challenging in remote and resource-limited settings. In this study, we present an AI-driven system for automated blood cell anomaly detection, combining computer vision and machine learning models to support efficient diagnostics in hematology and telehealth contexts. Our architecture integrates segmentation (YOLOv11), classification (ResNet50), transfer learning, and zero-shot learning to identify and categorize cell types and abnormalities from blood smear images. Evaluated on real annotated samples, the system achieved high performance, with a precision of 0.98, recall of 0.99, and F1 score of 0.98. These results highlight the potential of the proposed system to enhance remote diagnostic capabilities and support clinical decision making in underserved regions.
Full article
(This article belongs to the Special Issue Advances in Medical Imaging and Machine Learning)
►▼
Show Figures
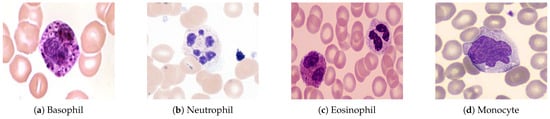
Figure 1
Open AccessArticle
Unleashing the Potential of Residual and Dual-Stream Transformers for the Remote Sensing Image Analysis
by
Priya Mittal, Vishesh Tanwar, Bhisham Sharma and Dhirendra Prasad Yadav
J. Imaging 2025, 11(5), 156; https://doi.org/10.3390/jimaging11050156 - 15 May 2025
Abstract
The categorization of remote sensing satellite imagery is crucial for various applications, including environmental monitoring, urban planning, and disaster management. Convolutional Neural Networks (CNNs) and Vision Transformers (ViTs) have exhibited exceptional performance among deep learning techniques, excelling in feature extraction and representational learning.
[...] Read more.
The categorization of remote sensing satellite imagery is crucial for various applications, including environmental monitoring, urban planning, and disaster management. Convolutional Neural Networks (CNNs) and Vision Transformers (ViTs) have exhibited exceptional performance among deep learning techniques, excelling in feature extraction and representational learning. This paper presents a hybrid dual-stream ResV2ViT model that combines the advantages of ResNet50 V2 and Vision Transformer (ViT) architectures. The dual-stream approach allows the model to extract both local spatial features and global contextual information by processing data through two complementary pathways. The ResNet50V2 component is utilized for hierarchical feature extraction and captures short-range dependencies, whereas the ViT module efficiently models long-range dependencies and global contextual information. After position embedding in the hybrid model, the tokens are bifurcated into two parts: q1 and q2. q1 is passed into the convolutional block to refine local spatial details, and q2 is given to the Transformer to provide global attention to the spatial feature. Combining these two architectures allows the model to acquire low-level and high-level feature representations, improving classification performance. We assess the proposed ResV2ViT model using the RSI-CB256 dataset and another dataset with 21 classes. The proposed model attains an average accuracy of 99.91%, with precision and F1 score of 99.90% for the first dataset and 98.75% accuracy for the second dataset, illustrating its efficacy in satellite image classification. The findings demonstrate that the dual-stream hybrid ResV2ViT model surpasses traditional CNN and Transformer-based models, establishing it as a formidable framework for remote sensing applications.
Full article
(This article belongs to the Special Issue Advanced Hyperspectral Imaging: Techniques, Data Analysis, and Applications)
►▼
Show Figures
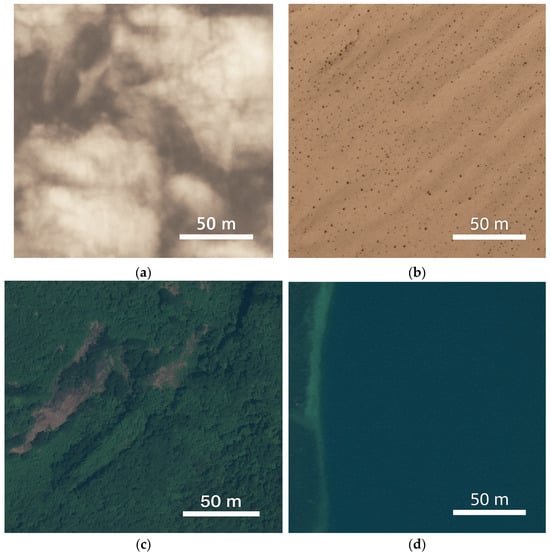
Figure 1
Open AccessArticle
Beyond Handcrafted Features: A Deep Learning Framework for Optical Flow and SLAM
by
Kamran Kazi, Arbab Nighat Kalhoro, Farida Memon, Azam Rafique Memon and Muddesar Iqbal
J. Imaging 2025, 11(5), 155; https://doi.org/10.3390/jimaging11050155 - 15 May 2025
Abstract
This paper presents a novel approach for visual Simultaneous Localization and Mapping (SLAM) using Convolution Neural Networks (CNNs) for robust map creation. Traditional SLAM methods rely on handcrafted features, which are susceptible to viewpoint changes, occlusions, and illumination variations. This work proposes a
[...] Read more.
This paper presents a novel approach for visual Simultaneous Localization and Mapping (SLAM) using Convolution Neural Networks (CNNs) for robust map creation. Traditional SLAM methods rely on handcrafted features, which are susceptible to viewpoint changes, occlusions, and illumination variations. This work proposes a method that leverages the power of CNNs by extracting features from an intermediate layer of a pre-trained model for optical flow estimation. We conduct an extensive search for optimal features by analyzing the offset error across thousands of combinations of layers and filters within the CNN. This analysis reveals a specific layer and filter combination that exhibits minimal offset error while still accounting for viewpoint changes, occlusions, and illumination variations. These features, learned by the CNN, are demonstrably robust to environmental challenges that often hinder traditional handcrafted features in SLAM tasks. The proposed method is evaluated on six publicly available datasets that are widely used for bench-marking map estimation and accuracy. Our method consistently achieved the lowest offset error compared to traditional handcrafted feature-based approaches on all six datasets. This demonstrates the effectiveness of CNN-derived features for building accurate and robust maps in diverse environments.
Full article
(This article belongs to the Section Visualization and Computer Graphics)
►▼
Show Figures
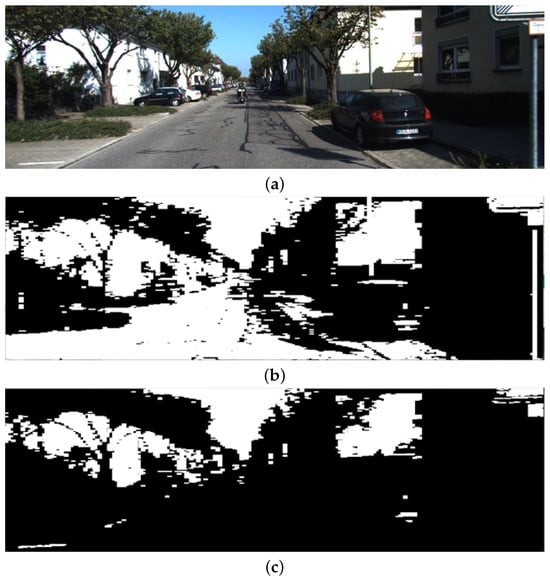
Figure 1
Open AccessArticle
A Multitask Network for the Diagnosis of Autoimmune Gastritis
by
Yuqi Cao, Yining Zhao, Xinao Jin, Jiayuan Zhang, Gangzhi Zhang, Pingjie Huang, Guangxin Zhang and Yuehua Han
J. Imaging 2025, 11(5), 154; https://doi.org/10.3390/jimaging11050154 - 15 May 2025
Abstract
Autoimmune gastritis (AIG) has a strong correlation with gastric neuroendocrine tumors (NETs) and gastric cancer, making its timely and accurate diagnosis crucial for tumor prevention. The endoscopic manifestations of AIG differ from those of gastritis caused by Helicobacter pylori (H. pylori)
[...] Read more.
Autoimmune gastritis (AIG) has a strong correlation with gastric neuroendocrine tumors (NETs) and gastric cancer, making its timely and accurate diagnosis crucial for tumor prevention. The endoscopic manifestations of AIG differ from those of gastritis caused by Helicobacter pylori (H. pylori) infection in terms of the affected gastric anatomical regions and the pathological characteristics observed in biopsy samples. Therefore, when diagnosing AIG based on endoscopic images, it is essential not only to distinguish between normal and atrophic gastric mucosa but also to accurately identify the anatomical region in which the atrophic mucosa is located. In this study, we propose a patient-based multitask gastroscopy image classification network that analyzes all images obtained during the endoscopic procedure. First, we employ the Scale-Invariant Feature Transform (SIFT) algorithm for image registration, generating an image similarity matrix. Next, we use a hierarchical clustering algorithm to group images based on this matrix. Finally, we apply the RepLKNet model, which utilizes large-kernel convolution, to each image group to perform two tasks: anatomical region classification and lesion recognition. Our method achieves an accuracy of 93.4 ± 0.5% (95% CI) and a precision of 92.6 ± 0.4% (95% CI) in the anatomical region classification task, which categorizes images into the fundus, body, and antrum. Additionally, it attains an accuracy of 90.2 ± 1.0% (95% CI) and a precision of 90.5 ± 0.8% (95% CI) in the lesion recognition task, which identifies the presence of gastric mucosal atrophic lesions in gastroscopy images. These results demonstrate that the proposed multitask patient-based gastroscopy image analysis method holds significant practical value for advancing computer-aided diagnosis systems for atrophic gastritis and enhancing the diagnostic accuracy and efficiency of AIG.
Full article
(This article belongs to the Section Medical Imaging)
►▼
Show Figures
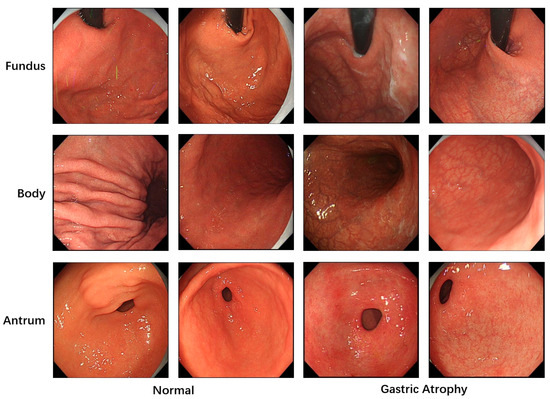
Figure 1
Open AccessArticle
Machine Learning Algorithms of Remote Sensing Data Processing for Mapping Changes in Land Cover Types over Central Apennines, Italy
by
Polina Lemenkova
J. Imaging 2025, 11(5), 153; https://doi.org/10.3390/jimaging11050153 - 12 May 2025
Abstract
►▼
Show Figures
This work presents the use of remote sensing data for land cover mapping with a case of Central Apennines, Italy. The data include 8 Landsat 8-9 Operational Land Imager/Thermal Infrared Sensor (OLI/TIRS) satellite images in six-year period (2018–2024). The operational workflow included satellite
[...] Read more.
This work presents the use of remote sensing data for land cover mapping with a case of Central Apennines, Italy. The data include 8 Landsat 8-9 Operational Land Imager/Thermal Infrared Sensor (OLI/TIRS) satellite images in six-year period (2018–2024). The operational workflow included satellite image processing which were classified into raster maps with automatically detected 10 classes of land cover types over the tested study. The approach was implemented by using a set of modules in Geographic Resources Analysis Support System (GRASS) Geographic Information System (GIS). To classify remote sensing (RS) data, two types of approaches were carried out. The first is unsupervised classification based on the MaxLike approach and clustering which extracted Digital Numbers (DN) of landscape feature based on the spectral reflectance of signals, and the second is supervised classification performed using several methods of Machine Learning (ML), technically realised in GRASS GIS scripting software. The latter included four ML algorithms embedded from the Python’s Scikit-Learn library. These classifiers have been implemented to detect subtle changes in land cover types as derived from the satellite images showing different vegetation conditions in spring and autumn periods in central Apennines, northern Italy.
Full article
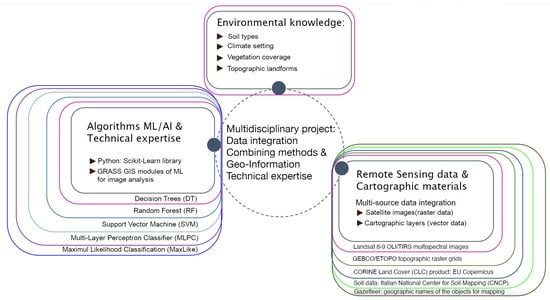
Figure 1
Open AccessArticle
Frequency-Aware Diffusion Model for Multi-Modal MRI Image Synthesis
by
Mingfeng Jiang, Peihang Jia, Xin Huang, Zihan Yuan, Dongsheng Ruan, Feng Liu and Ling Xia
J. Imaging 2025, 11(5), 152; https://doi.org/10.3390/jimaging11050152 - 11 May 2025
Abstract
Magnetic Resonance Imaging (MRI) is a widely used, non-invasive imaging technology that plays a critical role in clinical diagnostics. Multi-modal MRI, which combines images from different modalities, enhances diagnostic accuracy by offering comprehensive tissue characterization. Meanwhile, multi-modal MRI enhances downstream tasks, like brain
[...] Read more.
Magnetic Resonance Imaging (MRI) is a widely used, non-invasive imaging technology that plays a critical role in clinical diagnostics. Multi-modal MRI, which combines images from different modalities, enhances diagnostic accuracy by offering comprehensive tissue characterization. Meanwhile, multi-modal MRI enhances downstream tasks, like brain tumor segmentation and image reconstruction, by providing richer features. While recent advances in diffusion models (DMs) show potential for high-quality image translation, existing methods still struggle to preserve fine structural details and ensure accurate image synthesis in medical imaging. To address these challenges, we propose a Frequency-Aware Diffusion Model (FADM) for generating high-quality target modality MRI images from source modality images. The FADM incorporates a discrete wavelet transform within the diffusion model framework to extract both low- and high-frequency information from MRI images, enhancing the capture of tissue structural and textural features. Additionally, a wavelet downsampling layer and supervision module are incorporated to improve frequency awareness and optimize high-frequency detail extraction. Experimental results on the BraTS 2021 dataset and a 1.5T–3T MRI dataset demonstrate that the FADM outperforms existing generative models, particularly in preserving intricate brain structures and tumor regions while generating high-quality MRI images.
Full article
(This article belongs to the Special Issue Advances in Medical Imaging and Machine Learning)
►▼
Show Figures
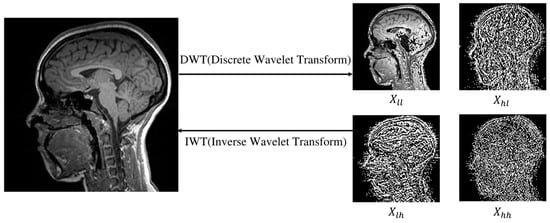
Figure 1
Open AccessArticle
A Weighted Facial Expression Analysis for Pain Level Estimation
by
Parkpoom Chaisiriprasert and Nattapat Patchsuwan
J. Imaging 2025, 11(5), 151; https://doi.org/10.3390/jimaging11050151 - 9 May 2025
Abstract
Accurate assessment of pain intensity is critical, particularly for patients who are unable to verbally express their discomfort. This study proposes a novel weighted analytical framework that integrates facial expression analysis through action units (AUs) with a facial feature-based weighting mechanism to enhance
[...] Read more.
Accurate assessment of pain intensity is critical, particularly for patients who are unable to verbally express their discomfort. This study proposes a novel weighted analytical framework that integrates facial expression analysis through action units (AUs) with a facial feature-based weighting mechanism to enhance the estimation of pain intensity. The proposed method was evaluated on a dataset comprising 4084 facial images from 25 individuals and demonstrated an average accuracy of 92.72% using the weighted pain level estimation model, in contrast to 83.37% achieved using conventional approaches. The observed improvements are primarily attributed to the strategic utilization of AU zones and expression-based weighting, which enable more precise differentiation between pain-related and non-pain-related facial movements. These findings underscore the efficacy of the proposed model in enhancing the accuracy and reliability of automated pain detection, especially in contexts where verbal communication is impaired or absent.
Full article
(This article belongs to the Topic Applications of Image and Video Processing in Medical Imaging)
►▼
Show Figures

Figure 1
Open AccessArticle
Reduction of Aerial Image Misalignment in Face-to-Face 3D Aerial Display
by
Atsutoshi Kurihara and Yue Bao
J. Imaging 2025, 11(5), 150; https://doi.org/10.3390/jimaging11050150 - 9 May 2025
Abstract
►▼
Show Figures
A Micromirror Array Plate (MMAP) has been proposed as a type of aerial display that allows users to directly touch the floating image. However, the aerial images generated by this optical element have a limited viewing angle, making them difficult to use in
[...] Read more.
A Micromirror Array Plate (MMAP) has been proposed as a type of aerial display that allows users to directly touch the floating image. However, the aerial images generated by this optical element have a limited viewing angle, making them difficult to use in face-to-face interactions. Conventional methods enable face-to-face usability by displaying multiple aerial images corresponding to different viewpoints. However, because these images are two-dimensional, they cannot be displayed at the same position due to the inherent characteristics of MMAP. An omnidirectional 3D autostereoscopic aerial display has been developed to address this issue, but it requires multiple expensive and specially shaped MMAPs to generate aerial images. To overcome this limitation, this study proposes a method that combines a single MMAP with integral photography (IP) to produce 3D aerial images with depth while reducing image misalignment. The experimental results demonstrate that the proposed method successfully displays a 3D aerial image using a single MMAP and reduces image misalignment to 1.1 mm.
Full article
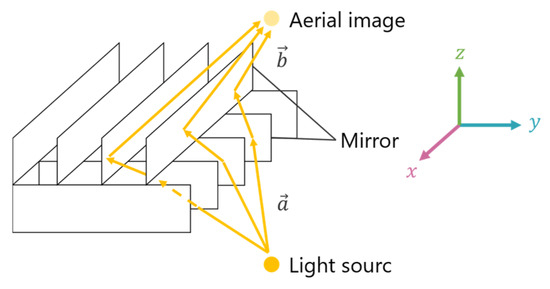
Figure 1
Open AccessArticle
Adaptive High-Precision 3D Reconstruction of Highly Reflective Mechanical Parts Based on Optimization of Exposure Time and Projection Intensity
by
Ci He, Rong Lai, Jin Sun, Kazuhiro Izui, Zili Wang, Xiaojian Liu and Shuyou Zhang
J. Imaging 2025, 11(5), 149; https://doi.org/10.3390/jimaging11050149 - 8 May 2025
Abstract
This article is used to reconstruct mechanical parts with highly reflective surfaces. Three-dimensional reconstruction based on Phase Measuring Profilometry (PMP) is a key technology in non-contact optical measurement and is widely applied in the intelligent inspection of mechanical components. Due to the high
[...] Read more.
This article is used to reconstruct mechanical parts with highly reflective surfaces. Three-dimensional reconstruction based on Phase Measuring Profilometry (PMP) is a key technology in non-contact optical measurement and is widely applied in the intelligent inspection of mechanical components. Due to the high reflectivity of metallic parts, direct utilization of the captured high-dynamic-range images often results in significant information loss in the oversaturated areas and excessive noise in the dark regions, leading to geometric defects and reduced accuracy in the reconstructed point clouds. Many image-fusion-based solutions have been proposed to solve these problems. However, unknown geometric structures and reflection characteristics of mechanical parts lead to the lack of effective guidance for the design of important imaging parameters. Therefore, an adaptive high-precision 3D reconstruction method of highly reflective mechanical parts based on optimization of exposure time and projection intensity is proposed in this article. The projection intensity is optimized to adapt the captured images to the linear dynamic range of the hardware. Image sequence under the obtained optimal intensities is fused using an integration of Genetic Algorithm and Stochastic Adam optimizer to maximize the image information entropy. Then, histogram-based analysis is employed to segment regions with similar reflective properties and determine the optimal exposure time. Experimental validation was carried out on three sets of typical mechanical components with diverse geometric characteristics and varying complexity. Compared with both non-saturated single-exposure techniques and conventional image fusion methods employing fixed attenuation steps, the proposed method reduced the average whisker range of reconstruction error by 51.18% and 25.09%, and decreased the median error by 42.48% and 25.42%, respectively. These experimental results verified the effectiveness and precision performance of the proposed method.
Full article
(This article belongs to the Special Issue Geometry Reconstruction from Images (2nd Edition))
►▼
Show Figures
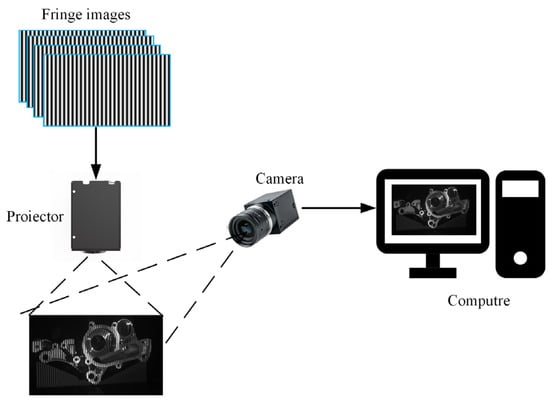
Figure 1
Open AccessArticle
3D-NASE: A Novel 3D CT Nasal Attention-Based Segmentation Ensemble
by
Alessandro Pani, Luca Zedda, Davide Antonio Mura, Andrea Loddo and Cecilia Di Ruberto
J. Imaging 2025, 11(5), 148; https://doi.org/10.3390/jimaging11050148 - 7 May 2025
Abstract
Accurate segmentation of the nasal cavity and paranasal sinuses in CT scans is crucial for disease assessment, treatment planning, and surgical navigation. It also facilitates the advanced computational modeling of airflow dynamics and enhances endoscopic surgery preparation. This work presents a novel ensemble
[...] Read more.
Accurate segmentation of the nasal cavity and paranasal sinuses in CT scans is crucial for disease assessment, treatment planning, and surgical navigation. It also facilitates the advanced computational modeling of airflow dynamics and enhances endoscopic surgery preparation. This work presents a novel ensemble framework for 3D nasal CT segmentation that synergistically combines CNN-based and transformer-based architectures, 3D-NASE. By integrating 3D U-Net, UNETR, Swin UNETR, SegResNet, DAF3D, and V-Net with majority and soft voting strategies, our approach leverages both local details and global context to improve segmentation accuracy and robustness. Results on the NasalSeg dataset demonstrate that the proposed ensemble method surpasses previous state-of-the-art results by achieving a improvement in the DICE score and reducing the standard deviation by . These promising results highlight the potential of our method to advance clinical workflows in diagnosis, treatment planning, and surgical navigation while also promoting further research into computationally efficient and highly accurate segmentation techniques.
Full article
(This article belongs to the Special Issue Advances in Biomedical Image Processing and Artificial Intelligence for Computer-Aided Diagnosis in Medicine)
►▼
Show Figures
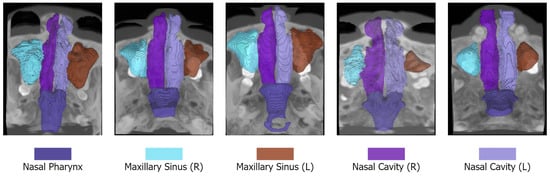
Figure 1
Open AccessArticle
Towards the Performance Characterization of a Robotic Multimodal Diagnostic Imaging System
by
George Papaioannou, Christos Mitrogiannis, Mark Schweitzer, Nikolaos Michailidis, Maria Pappa, Pegah Khosravi, Apostolos Karantanas, Sean Starling and Christian Ruberg
J. Imaging 2025, 11(5), 147; https://doi.org/10.3390/jimaging11050147 - 7 May 2025
Abstract
Characterizing imaging performance requires a multidisciplinary approach that evaluates various interconnected parameters, including dosage optimization and dynamic accuracy. Radiation dose and dynamic accuracy are challenged by patient motion that results in poor image quality. These challenges are more prevalent in the brain/cardiac pediatric
[...] Read more.
Characterizing imaging performance requires a multidisciplinary approach that evaluates various interconnected parameters, including dosage optimization and dynamic accuracy. Radiation dose and dynamic accuracy are challenged by patient motion that results in poor image quality. These challenges are more prevalent in the brain/cardiac pediatric patient imaging, as they relate to excess radiation dose that may be associated with various complications. Scanning vulnerable pediatric patients ought to eliminate anesthesia due to critical risks associated in some cases with intracranial hemorrhages, brain strokes, and congenital heart disease. Some pediatric imaging, however, requires prolonged scanning under anesthesia. It can often be a laborious, suboptimal process, with limited field of view and considerable dose. High dynamic accuracy is also necessary to diagnose tissue’s dynamic behavior beyond its static structural morphology. This study presents several performance characterization experiments from a new robotic multimodal imaging system using specially designed calibration methods at different system configurations. Additional musculoskeletal imaging and imaging from a pediatric brain stroke patient without anesthesia are presented for comparisons. The findings suggest that the system’s large dynamically controlled gantry enables scanning at full patient movement and with important improvements in scan times, accuracy, radiation dose, and the ability to image brain structures without anesthesia. This could position the system as a potential transformative tool in the pediatric interventional imaging landscape.
Full article
(This article belongs to the Special Issue Celebrating the 10th Anniversary of the Journal of Imaging)
►▼
Show Figures
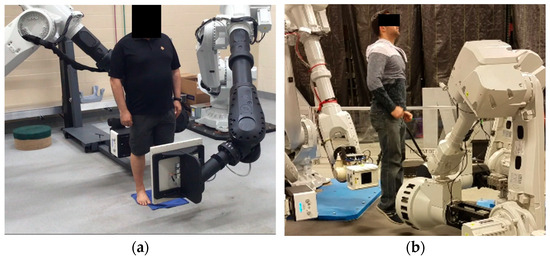
Figure 1
Open AccessArticle
Quantitative Ultrasound Texture Analysis of Breast Tumors: A Comparison of a Cart-Based and a Wireless Ultrasound Scanner
by
David Alberico, Lakshmanan Sannachi, Maria Lourdes Anzola Pena, Joyce Yip, Laurentius O. Osapoetra, Schontal Halstead, Daniel DiCenzo, Sonal Gandhi, Frances Wright, Michael Oelze and Gregory J. Czarnota
J. Imaging 2025, 11(5), 146; https://doi.org/10.3390/jimaging11050146 - 6 May 2025
Abstract
Previous work has demonstrated quantitative ultrasound (QUS) analysis techniques for extracting features and texture features from ultrasound radiofrequency data which can be used to distinguish between benign and malignant breast masses. It is desirable that there be good agreement between estimates of such
[...] Read more.
Previous work has demonstrated quantitative ultrasound (QUS) analysis techniques for extracting features and texture features from ultrasound radiofrequency data which can be used to distinguish between benign and malignant breast masses. It is desirable that there be good agreement between estimates of such features acquired using different ultrasound devices. Handheld ultrasound imaging systems are of particular interest as they are compact, relatively inexpensive, and highly portable. This study investigated the agreement between QUS parameters and texture features estimated from clinical ultrasound images of breast tumors acquired using two different ultrasound scanners: a traditional cart-based system and a wireless handheld ultrasound system. The 28 patients who participated were divided into two groups (benign and malignant). The reference phantom technique was used to produce functional estimates of the normalized power spectra and backscatter coefficient for each image. Root mean square differences of feature estimates were calculated for each cohort to quantify the level of feature variation attributable to tissue heterogeneity and differences in system imaging parameters. Cross-system statistical testing using the Mann–Whitney U test was performed on benign and malignant patient cohorts to assess the level of feature estimate agreement between systems, and the Bland–Altman method was employed to assess feature sets for systematic bias introduced by differences in imaging method. The range of p-values was 1.03 × 10−4 to 0.827 for the benign cohort and 3.03 × 10−10 to 0.958 for the malignant cohort. For both cohorts, all five of the primary QUS features (MBF, SS, SI, ASD, AAC) were found to be in agreement at the 5% confidence level. A total of 13 of the 20 QUS texture features (65%) were determined to exhibit statistically significant differences in the sample medians of estimates between systems at the 5% confidence level, with the remaining 7 texture features being in agreement. The results showed a comparable magnitude of feature variation between tissue heterogeneity and system effects, as well as a moderate level of statistical agreement between feature sets.
Full article
(This article belongs to the Section Medical Imaging)
►▼
Show Figures
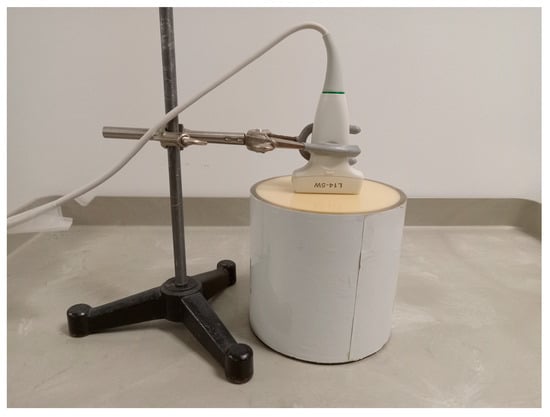
Figure 1
Open AccessArticle
Shear Wave Elastography for Parotid Glands: Quantitative Analysis of Shear Elastic Modulus in Relation to Age, Gender, and Internal Architecture in Patients with Oral Cancer
by
Yuka Tanabe, Ai Shirai and Ichiro Ogura
J. Imaging 2025, 11(5), 145; https://doi.org/10.3390/jimaging11050145 - 4 May 2025
Abstract
Background: Recently, shear wave elastography (SWE) has been recognized as an effective tool for evaluating Sjögren’s syndrome (SS) patients. The purpose of this study was to assess the parotid glands with SWE, especially for quantitative analysis of shear elastic modulus in relation to
[...] Read more.
Background: Recently, shear wave elastography (SWE) has been recognized as an effective tool for evaluating Sjögren’s syndrome (SS) patients. The purpose of this study was to assess the parotid glands with SWE, especially for quantitative analysis of shear elastic modulus in relation to age, gender, and internal architecture in patients with oral cancer to collect control data for SS. Methods: In total, 124 parotid glands of 62 patients with oral cancer were evaluated with SWE. The parotid glands were examined for the internal architecture (homogeneous or heterogeneous) on B-mode. The SWE allowed the operator to place regions of interest (ROIs) for parotid glands, and displayed automatically shear elastic modulus data (kPa) for each ROI. Gender and internal architecture were compared with the shear elastic modulus of the parotid glands by Mann–Whitney U-test. The comparison of age and shear elastic modulus was assessed using Spearman’s correlation coefficient. p < 0.05 was considered statistically significant. Results: The shear elastic modulus of the parotid glands was not significantly different for according to gender (males, 7.70 ± 2.22 kPa and females, 7.67 ± 2.41 kPa, p = 0.973) or internal architecture (homogeneous: 7.69 ± 2.25 kPa and heterogeneous: 7.72 ± 2.74 kPa, p = 0.981). Furthermore, the shear elastic modulus was not correlated with age (n = 124, R = −0.133, p = 0.139). Conclusion: Our study showed the control data of the shear elastic modulus of the parotid glands for SS. SWE is useful for the quantitative evaluation of the parotid glands.
Full article
(This article belongs to the Section Medical Imaging)
►▼
Show Figures
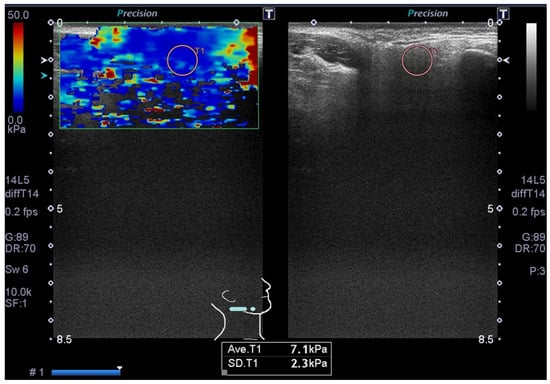
Figure 1
Open AccessArticle
Motion-Perception Multi-Object Tracking (MPMOT): Enhancing Multi-Object Tracking Performance via Motion-Aware Data Association and Trajectory Connection
by
Weijun Meng, Shuaipeng Duan, Sugang Ma and Bin Hu
J. Imaging 2025, 11(5), 144; https://doi.org/10.3390/jimaging11050144 - 3 May 2025
Abstract
Multiple Object Tracking (MOT) aims to detect and track multiple targets across consecutive video frames while preserving consistent object identities. While appearance-based approaches have achieved notable success, they often struggle in challenging conditions such as occlusions, motion blur, and the presence of visually
[...] Read more.
Multiple Object Tracking (MOT) aims to detect and track multiple targets across consecutive video frames while preserving consistent object identities. While appearance-based approaches have achieved notable success, they often struggle in challenging conditions such as occlusions, motion blur, and the presence of visually similar objects, resulting in identity switches and fragmented trajectories. To address these limitations, we propose Motion-Perception Multi-Object Tracking (MPMOT), a motion-aware tracking framework that emphasizes robust motion modeling and adaptive association. MPMOT incorporates three core components: (1) a Gain Kalman Filter (GKF) that adaptively adjusts detection noise based on confidence scores, stabilizing motion prediction during uncertain observations; (2) an Adaptive Cost Matrix (ACM) that dynamically fuses motion and appearance cues during track–detection association, improving robustness under ambiguity; and (3) a Global Connection Model (GCM) that reconnects fragmented tracklets by modeling spatio-temporal consistency. Extensive experiments on the MOT16, MOT17, and MOT20 benchmarks demonstrate that MPMOT consistently outperforms state-of-the-art trackers, achieving IDF1 scores of 72.8% and 72.6% on MOT16 and MOT17, respectively, surpassing the widely used FairMOT baseline by 1.1% and 1.3%. Additionally, rigorous statistical validation through post hoc analysis confirms that MPMOT’s improvements in tracking accuracy and identity preservation are statistically significant across all datasets. MPMOT delivers these gains while maintaining real-time performance, making it a scalable and reliable solution for multi-object tracking in dynamic and crowded environments.
Full article
(This article belongs to the Section Computer Vision and Pattern Recognition)
►▼
Show Figures
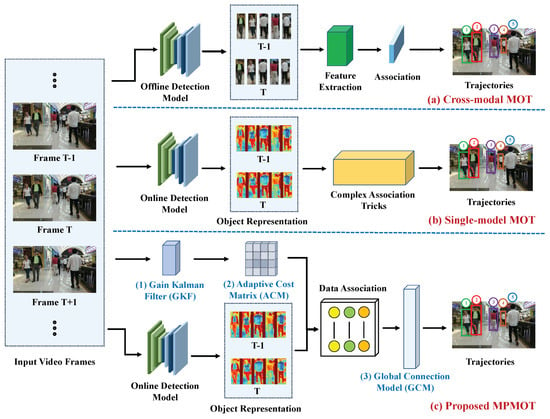
Figure 1
Open AccessReview
Cardiac Magnetic Resonance in the Assessment of Atrial Cardiomyopathy and Pulmonary Vein Isolation Planning for Atrial Fibrillation
by
Nicola Pegoraro, Serena Chiarello, Riccardo Bisi, Giuseppe Muscogiuri, Matteo Bertini, Aldo Carnevale, Melchiore Giganti and Alberto Cossu
J. Imaging 2025, 11(5), 143; https://doi.org/10.3390/jimaging11050143 - 2 May 2025
Abstract
Atrial fibrillation (AF) is the most frequently observed type of arrhythmia among adults, and its absolute prevalence is steadily rising in close association with the aging of the population, with its prevalence varying from 2% in the general population to 10–12% among the
[...] Read more.
Atrial fibrillation (AF) is the most frequently observed type of arrhythmia among adults, and its absolute prevalence is steadily rising in close association with the aging of the population, with its prevalence varying from 2% in the general population to 10–12% among the elderly. The relatively new concepts of ‘atrial cardiomyopathy’ and “AF-related atrial cardiomyopathy”, along with the growing body of knowledge regarding remodeling, function, and tissue characterization, highlight the need for novel approaches to the diagnostic process as well as in the therapeutic guidance and monitoring of atrial arrhythmias. Advanced imaging techniques, particularly cardiac magnetic resonance (CMR) imaging, have emerged as pivotal in the detailed assessment of atrial structure and function. CMR facilitates the precise measurement of left atrial volume and morphology, which are critical predictors of AF recurrence post-intervention. Furthermore, it enables the evaluation of atrial fibrosis using late gadolinium enhancement (LGE), offering a non-invasive method to assess the severity and distribution of fibrotic tissue. The possibility of an accurate CMR pulmonary vein anatomy mapping enhances the precision of pulmonary vein isolation procedures, potentially improving outcomes in AF management. This review underlines the integration of novel diagnostic tools in enhancing the understanding and management of AF, advocating for a shift towards more personalized and effective therapeutic programs.
Full article
(This article belongs to the Special Issue Clinical and Pathological Imaging in the Era of Artificial Intelligence: New Insights and Perspectives—2nd Edition)
►▼
Show Figures
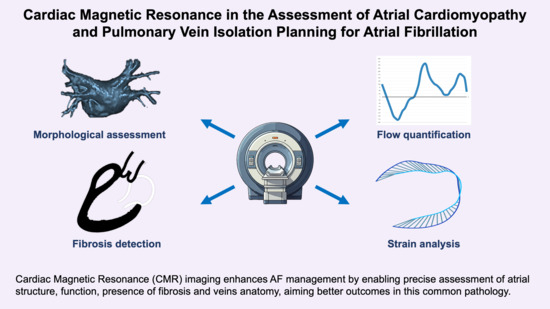
Graphical abstract
Open AccessArticle
ARAN: Age-Restricted Anonymized Dataset of Children Images and Body Measurements
by
Hezha H. MohammedKhan, Cascha Van Wanrooij, Eric O. Postma, Çiçek Güven, Marleen Balvert, Heersh Raof Saeed and Chenar Omer Ali Al Jaf
J. Imaging 2025, 11(5), 142; https://doi.org/10.3390/jimaging11050142 - 30 Apr 2025
Abstract
►▼
Show Figures
Precisely estimating a child’s body measurements and weight from a single image is useful in pediatrics for monitoring growth and detecting early signs of malnutrition. The development of estimation models for this task is hampered by the unavailability of a labeled image dataset
[...] Read more.
Precisely estimating a child’s body measurements and weight from a single image is useful in pediatrics for monitoring growth and detecting early signs of malnutrition. The development of estimation models for this task is hampered by the unavailability of a labeled image dataset to support supervised learning. This paper introduces the “Age-Restricted Anonymized” (ARAN) dataset, the first labeled image dataset of children with body measurements approved by an ethics committee under the European General Data Protection Regulation guidelines. The ARAN dataset consists of images of 512 children aged 16 to 98 months, each captured from four different viewpoints, i.e., 2048 images in total. The dataset is anonymized manually on the spot through a face mask and includes each child’s height, weight, age, waist circumference, and head circumference measurements. The dataset is a solid foundation for developing prediction models for various tasks related to these measurements; it addresses the gap in computer vision tasks related to body measurements as it is significantly larger than any other comparable dataset of children, along with diverse viewpoints. To create a suitable reference, we trained state-of-the-art deep learning algorithms on the ARAN dataset to predict body measurements from the images. The best results are obtained by a DenseNet121 model achieving competitive estimates for the body measurements, outperforming state-of-the-art results on similar tasks. The ARAN dataset is developed as part of a collaboration to create a mobile app to measure children’s growth and detect early signs of malnutrition, contributing to the United Nations Sustainable Development Goals.
Full article
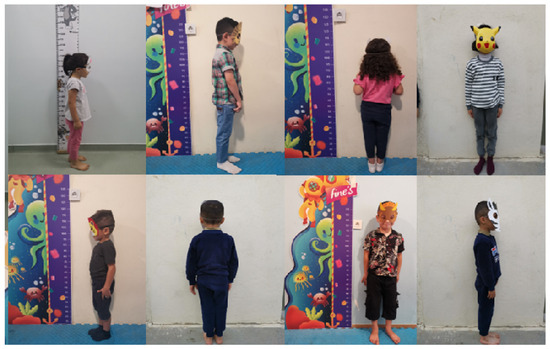
Figure 1
Open AccessReview
AI-Powered Object Detection in Radiology: Current Models, Challenges, and Future Direction
by
Abdussalam Elhanashi, Sergio Saponara, Qinghe Zheng, Nawal Almutairi, Yashbir Singh, Shiba Kuanar, Farzana Ali, Orhan Unal and Shahriar Faghani
J. Imaging 2025, 11(5), 141; https://doi.org/10.3390/jimaging11050141 - 30 Apr 2025
Abstract
Artificial intelligence (AI)-based object detection in radiology can assist in clinical diagnosis and treatment planning. This article examines the AI-based object detection models currently used in many imaging modalities, including X-ray Magnetic Resonance Imaging (MRI), Computed Tomography (CT), and Ultrasound (US). The key
[...] Read more.
Artificial intelligence (AI)-based object detection in radiology can assist in clinical diagnosis and treatment planning. This article examines the AI-based object detection models currently used in many imaging modalities, including X-ray Magnetic Resonance Imaging (MRI), Computed Tomography (CT), and Ultrasound (US). The key models from the convolutional neural network (CNN) as well as the contemporary transformer and hybrid models are analyzed based on their ability to detect pathological features, such as tumors, lesions, and tissue abnormalities. In addition, this review offers a closer look at the strengths and weaknesses of these models in terms of accuracy, robustness, and speed in real clinical settings. The common issues related to these models, including limited data, annotation quality, and interpretability of AI decisions, are discussed in detail. Moreover, the need for strong applicable models across different populations and imaging modalities are addressed. The importance of privacy and ethics in general data use as well as safety and regulations for healthcare data are emphasized. The future potential of these models lies in their accessibility in low resource settings, usability in shared learning spaces while maintaining privacy, and improvement in diagnostic accuracy through multimodal learning. This review also highlights the importance of interdisciplinary collaboration among artificial intelligence researchers, radiologists, and policymakers. Such cooperation is essential to address current challenges and to fully realize the potential of AI-based object detection in radiology.
Full article
(This article belongs to the Special Issue Learning and Optimization for Medical Imaging)
►▼
Show Figures
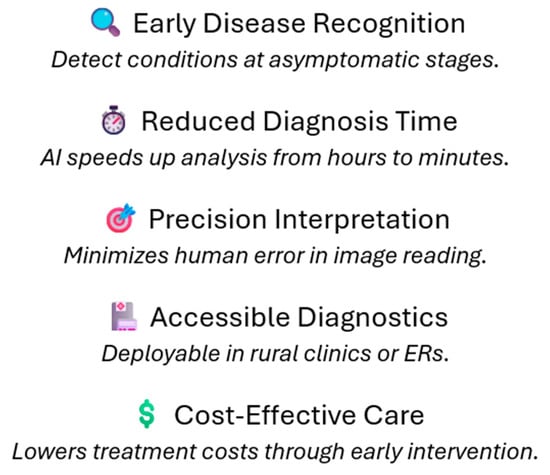
Figure 1
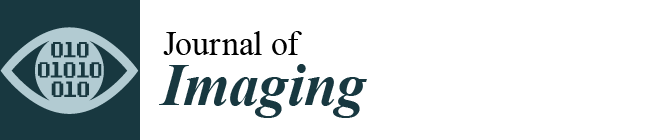
Journal Menu
► ▼ Journal Menu-
- J. Imaging Home
- Aims & Scope
- Editorial Board
- Reviewer Board
- Topical Advisory Panel
- Instructions for Authors
- Special Issues
- Topics
- Sections
- Article Processing Charge
- Indexing & Archiving
- Most Cited & Viewed
- Journal Statistics
- Journal History
- Journal Awards
- Conferences
- Editorial Office
- 10th Anniversary
Journal Browser
► ▼ Journal BrowserHighly Accessed Articles
Latest Books
E-Mail Alert
News
Topics
Topic in
Applied Sciences, Computation, Entropy, J. Imaging, Optics
Color Image Processing: Models and Methods (CIP: MM)
Topic Editors: Giuliana Ramella, Isabella TorcicolloDeadline: 30 July 2025
Topic in
Applied Sciences, Bioengineering, Diagnostics, J. Imaging, Signals
Signal Analysis and Biomedical Imaging for Precision Medicine
Topic Editors: Surbhi Bhatia Khan, Mo SaraeeDeadline: 31 August 2025
Topic in
Animals, Computers, Information, J. Imaging, Veterinary Sciences
AI, Deep Learning, and Machine Learning in Veterinary Science Imaging
Topic Editors: Vitor Filipe, Lio Gonçalves, Mário GinjaDeadline: 31 October 2025
Topic in
Applied Sciences, Electronics, MAKE, J. Imaging, Sensors
Applied Computer Vision and Pattern Recognition: 2nd Edition
Topic Editors: Antonio Fernández-Caballero, Byung-Gyu KimDeadline: 31 December 2025

Conferences
Special Issues
Special Issue in
J. Imaging
Advances and Challenges in Bone Imaging
Guest Editor: Shai FactorDeadline: 30 May 2025
Special Issue in
J. Imaging
Recent Developments in Optical Imaging
Guest Editor: Ralf PalmisanoDeadline: 30 May 2025
Special Issue in
J. Imaging
Color in Image Processing and Computer Vision
Guest Editors: Alain Tremeau, Marco Buzzelli, Shoji TominagaDeadline: 31 May 2025
Special Issue in
J. Imaging
Image and Video Processing for Blind and Visually Impaired
Guest Editors: Zhigang Zhu, John Ross Rizzo, Hao TangDeadline: 31 May 2025