Journal Description
Mathematical and Computational Applications
Mathematical and Computational Applications
is an international, peer-reviewed, open access journal on applications of mathematical and/or computational techniques, published bimonthly online by MDPI.
- Open Access— free for readers, with article processing charges (APC) paid by authors or their institutions.
- High Visibility: indexed within Scopus, ESCI (Web of Science), Inspec, and other databases.
- Rapid Publication: manuscripts are peer-reviewed and a first decision is provided to authors approximately 22.5 days after submission; acceptance to publication is undertaken in 3.2 days (median values for papers published in this journal in the second half of 2023).
- Recognition of Reviewers: reviewers who provide timely, thorough peer-review reports receive vouchers entitling them to a discount on the APC of their next publication in any MDPI journal, in appreciation of the work done.
- Testimonials: See what our editors and authors say about MCA.
Impact Factor:
1.9 (2022);
5-Year Impact Factor:
1.9 (2022)
Latest Articles
Recognizable Languages of k-Forcing Automata
Math. Comput. Appl. 2024, 29(3), 32; https://doi.org/10.3390/mca29030032 - 25 Apr 2024
Abstract
►
Show Figures
In this study, we show that automata theory is also a suitable tool for analyzing a more complex type of the k-forcing process. First, the definition of k-forcing automata is presented according to the definition of k-forcing for graphs. Moreover, we study and
[...] Read more.
In this study, we show that automata theory is also a suitable tool for analyzing a more complex type of the k-forcing process. First, the definition of k-forcing automata is presented according to the definition of k-forcing for graphs. Moreover, we study and discuss the language of k-forcing automata for particular graphs. Also, for some graphs with different k-forcing sets, we study the languages of their k-forcing automata. In addition, for some given recognizable languages, we study the structure of graphs. After that, we show that k-forcing automata arising from isomorph graphs are also isomorph. Also, we present the style of words that can be recognized with k-forcing automata. Moreover, we introduce the structure of graphs the k-forcing automata arising from which recognize some particular languages. To clarify the notions and the results obtained in this study, some examples are submitted as well.
Full article
Open AccessArticle
A New Generalized Definition of Fractal–Fractional Derivative with Some Applications
by
Francisco Martínez and Mohammed K. A. Kaabar
Math. Comput. Appl. 2024, 29(3), 31; https://doi.org/10.3390/mca29030031 - 25 Apr 2024
Abstract
In this study, a new generalized fractal–fractional (FF) derivative is proposed. By applying this definition to some elementary functions, we show its compatibility with the results of the FF derivative in the Caputo sense with the power law. The main elements of classical
[...] Read more.
In this study, a new generalized fractal–fractional (FF) derivative is proposed. By applying this definition to some elementary functions, we show its compatibility with the results of the FF derivative in the Caputo sense with the power law. The main elements of classical differential calculus are introduced in terms of this new derivative. Thus, we establish and demonstrate the basic operations with derivatives, chain rule, mean value theorems with their immediate applications and inverse function’s derivative. We complete the theory of generalized FF calculus by proposing a notion of integration and presenting two important results of integral calculus: the fundamental theorem and Barrow’s rule. Finally, we analytically solve interesting FF ordinary differential equations by applying our proposed definition.
Full article
Open AccessArticle
Simulation of Temperature Field in Steel Billets during Reheating in Pusher-Type Furnace by Meshless Method
by
Qingguo Liu, Umut Hanoglu, Zlatko Rek and Božidar Šarler
Math. Comput. Appl. 2024, 29(3), 30; https://doi.org/10.3390/mca29030030 - 24 Apr 2024
Abstract
Using a meshless method, a simulation of steel billets in a pusher-type reheating furnace is carried out for the first time. The simulation represents an affordable way to replace the measurements. The heat transfer from the billets with convection and radiation is considered.
[...] Read more.
Using a meshless method, a simulation of steel billets in a pusher-type reheating furnace is carried out for the first time. The simulation represents an affordable way to replace the measurements. The heat transfer from the billets with convection and radiation is considered. Inside each of the billets, the heat diffusion equation is solved on a two-dimensional central slice of the billet. The diffusion equation is solved in a strong form by the Local Radial Basis Function Collocation Method (LRBFCM) with explicit time-stepping. The ray tracing procedure solves the radiation, where the view factors are computed with the Monte Carlo method. The changing number of billets in the furnace at the start and the end of the loading and unloading of the furnace is considered. A sensitivity study on billets' temperature evolution is performed as a function of a different number of rays used in the Monte Carlo method, different stopping times of the billets in the furnace, and different spacing between the billets. The temperature field simulation is also essential for automatically optimizing the furnace’s productivity, energy consumption, and the billet’s quality. For the first time, the LRBFCM is successfully demonstrated for solving such a complex industrial problem.
Full article
(This article belongs to the Special Issue Radial Basis Functions)
Open AccessArticle
A Robust FOPD Controller That Allows Faster Detection of Defects for Touch Panels
by
Yuan-Jay Wang
Math. Comput. Appl. 2024, 29(2), 29; https://doi.org/10.3390/mca29020029 - 16 Apr 2024
Abstract
This study aims to synthesize and implement a robust fractional order PD (RFOPD) controller to increase the speed at which defects in automated touch panel inspection systems (ATPISs) are detected. A three-dimensional orthogonal stage (TDOS) driven by BLDC servo motors moves the inspection
[...] Read more.
This study aims to synthesize and implement a robust fractional order PD (RFOPD) controller to increase the speed at which defects in automated touch panel inspection systems (ATPISs) are detected. A three-dimensional orthogonal stage (TDOS) driven by BLDC servo motors moves the inspection pen (IP) vertically and horizontally. The dynamic equation relating the BLDC servo motor input to the tip motion is established. A touch position identification (TPI) system is used to locate the touch point rapidly. An RFOPD controller is used to actuate the BLDC servo motors and move the TDOS rapidly and accurately in three dimensions. This method displaces the IP to any specified position and shows user-defined inspection trajectories on the touch screens. The gain-phase margin tester (GPMT) and stability equation methods are exploited to schedule the RFOPD controller gain settings and to maintain the specific safety margins for the controlled system. The simulation studies show that the proposed RFOPD controller exhibits better tracking and disturbance rejection responses than a conventional PID controller. The robustness of the RFOPD-controlled ATPIS, considering unmodeled uncertainties and friction-induced disturbances, is verified through simulation and experimental studies. Several user-defined inspection patterns are used to verify performance, and the experimental results show that the proposed RFOPD controller is effective.
Full article
(This article belongs to the Topic Mathematical Modeling)
►▼
Show Figures
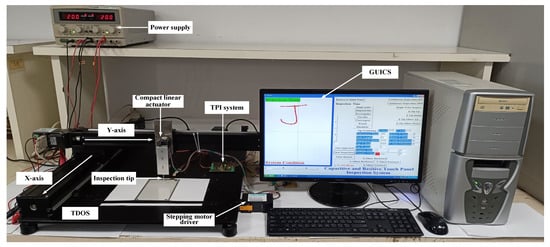
Figure 1
Open AccessArticle
Global Behavior of Solutions to a Higher-Dimensional System of Difference Equations with Lucas Numbers Coefficients
by
Messaoud Berkal, Juan Francisco Navarro and Raafat Abo-Zeid
Math. Comput. Appl. 2024, 29(2), 28; https://doi.org/10.3390/mca29020028 - 31 Mar 2024
Abstract
►▼
Show Figures
In this paper, we derive the well-defined solutions to a -dimensional system of difference equations. We show that, the well-defined solutions to that system are represented in terms of Fibonacci and Lucas sequences. Moreover, we study the global stability of the solutions
[...] Read more.
In this paper, we derive the well-defined solutions to a -dimensional system of difference equations. We show that, the well-defined solutions to that system are represented in terms of Fibonacci and Lucas sequences. Moreover, we study the global stability of the solutions to that system. Finally, we give some numerical examples which confirm our theoretical results.
Full article
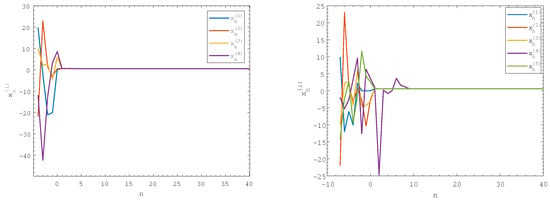
Figure 1
Open AccessArticle
Modeling of Chemical Vapor Infiltration for Fiber-Reinforced Silicon Carbide Composites Using Meshless Method of Fundamental Solutions
by
Patrick Mahoney and Alex Povitsky
Math. Comput. Appl. 2024, 29(2), 27; https://doi.org/10.3390/mca29020027 - 22 Mar 2024
Abstract
In this study, the Method of Fundamental Solutions (MFSs) is adopted to model Chemical Vapor Infiltration (CVI) in a fibrous preform. The preparation of dense fiber-reinforced silicon carbide composites is considered. The reaction flux at the solid surface is equal to the diffusion
[...] Read more.
In this study, the Method of Fundamental Solutions (MFSs) is adopted to model Chemical Vapor Infiltration (CVI) in a fibrous preform. The preparation of dense fiber-reinforced silicon carbide composites is considered. The reaction flux at the solid surface is equal to the diffusion flux towards the surface. The Robin or third-type boundary condition is implemented into the MFS. From the fibers’ surface concentrations obtained by MFS, deposition rates are calculated, and the geometry is updated at each time step, modeling the pore filling over time. The MFS solution is verified by comparing the results to a known analytical solution for a simplified geometry of concentric cylinders with a concentration set at the outer cylinder and a reaction at the inner cylinder. MFS solutions are compared to published experimental data. Porosity transients are obtained by a combination of MFSs with surface deposition to show the relation between the initial and final porosities.
Full article
(This article belongs to the Special Issue Recent Advances and New Challenges in Coupled Systems and Networks: Theory, Modelling, and Applications)
►▼
Show Figures
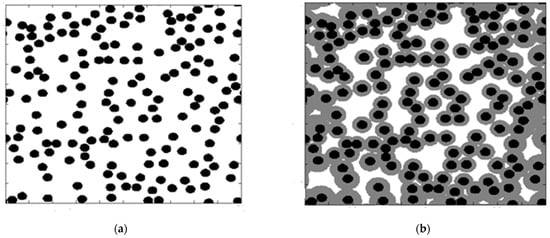
Figure 1
Open AccessFeature PaperArticle
Testing Homogeneity of Proportion Ratios for Stratified Bilateral Correlated Data
by
Wanqing Tian and Changxing Ma
Math. Comput. Appl. 2024, 29(2), 26; https://doi.org/10.3390/mca29020026 - 22 Mar 2024
Abstract
►▼
Show Figures
Intraclass correlation in bilateral data has been investigated in recent decades with various statistical methods. In practice, stratifying bilateral data by some control variables will provide more sophisticated statistical results to satisfy different research proposed in randomized clinical trials. In this article, we
[...] Read more.
Intraclass correlation in bilateral data has been investigated in recent decades with various statistical methods. In practice, stratifying bilateral data by some control variables will provide more sophisticated statistical results to satisfy different research proposed in randomized clinical trials. In this article, we propose three test statistics (the likelihood ratio test, score test, and Wald-type test statistics) to evaluate the homogeneity of proportion ratios for stratified bilateral correlated data under an equal correlation assumption. Monte Carlo simulations of Type I error and power are performed, and the score test yields a robust outcome based on empirical Type I error and power. Lastly, two real data examples are conducted to illustrate the proposed three tests.
Full article
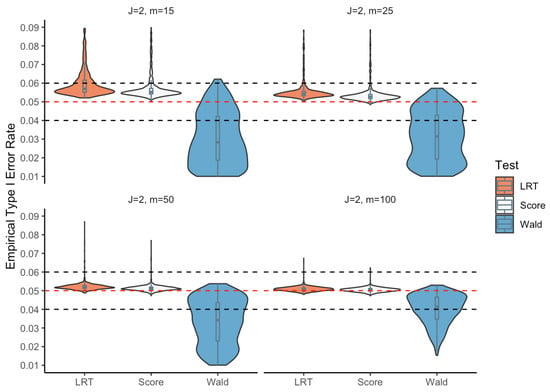
Figure 1
Open AccessArticle
M5GP: Parallel Multidimensional Genetic Programming with Multidimensional Populations for Symbolic Regression
by
Luis Cárdenas Florido, Leonardo Trujillo, Daniel E. Hernandez and Jose Manuel Muñoz Contreras
Math. Comput. Appl. 2024, 29(2), 25; https://doi.org/10.3390/mca29020025 - 18 Mar 2024
Abstract
Machine learning and artificial intelligence are growing in popularity thanks to their ability to produce models that exhibit unprecedented performance in domains that include computer vision, natural language processing and code generation. However, such models tend to be very large and complex and
[...] Read more.
Machine learning and artificial intelligence are growing in popularity thanks to their ability to produce models that exhibit unprecedented performance in domains that include computer vision, natural language processing and code generation. However, such models tend to be very large and complex and impossible to understand using traditional analysis or human scrutiny. Conversely, Symbolic Regression methods attempt to produce models that are relatively small and (potentially) human-readable. In this domain, Genetic Programming (GP) has proven to be a powerful search strategy that achieves state-of-the-art performance. This paper presents a new GP-based feature transformation method called M5GP, which is hybridized with multiple linear regression to produce linear models, implemented to exploit parallel processing on graphical processing units for efficient computation. M5GP is the most recent variant from a family of feature transformation methods (M2GP, M3GP and M4GP) that have proven to be powerful tools for both classification and regression tasks applied to tabular data. The proposed method was evaluated on SRBench v2.0, the current standard benchmarking suite for Symbolic Regression. Results show that M5GP achieves performance that is competitive with the state-of-the-art, achieving a top-three rank on the most difficult subset of black-box problems. Moreover, it achieves the lowest computation time when compared to other GP-based methods that have similar accuracy scores.
Full article
(This article belongs to the Special Issue New Trends in Computational Intelligence and Applications 2023)
►▼
Show Figures
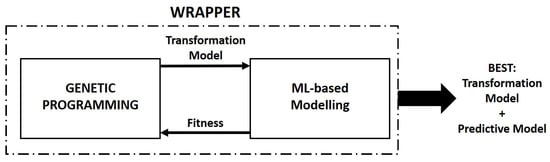
Figure 1
Open AccessArticle
A Coupled Finite-Boundary Element Method for Efficient Dynamic Structure-Soil-Structure Interaction Modeling
by
Parham Azhir, Jafar Asgari Marnani, Mehdi Panji and Mohammad Sadegh Rohanimanesh
Math. Comput. Appl. 2024, 29(2), 24; https://doi.org/10.3390/mca29020024 - 18 Mar 2024
Abstract
►▼
Show Figures
This paper introduces an innovative approach to numerically model Structure–Soil-Structure Interaction (SSSI) by integrating the Boundary Element Method (BEM) and the Finite Element Method (FEM) in a coupled manner. To assess the accuracy of the proposed method, a comparative study is undertaken, comparing
[...] Read more.
This paper introduces an innovative approach to numerically model Structure–Soil-Structure Interaction (SSSI) by integrating the Boundary Element Method (BEM) and the Finite Element Method (FEM) in a coupled manner. To assess the accuracy of the proposed method, a comparative study is undertaken, comparing its outcomes with those generated by the conventional FEM technique. Alongside accuracy, the computational efficiency aspect is crucial for the analysis of large-scale SSSI problems. Hence, the computational performance of the coupled BEM–FEM method undergoes a thorough examination and is compared with that of the standalone FEM method. The results from these comparisons illustrate the superior capabilities of the proposed method in comparison to the FEM method. The novel approach provides more reliable results compared to traditional FEM methods, serving as a valuable tool for engineers and researchers involved in structural analysis and design.
Full article
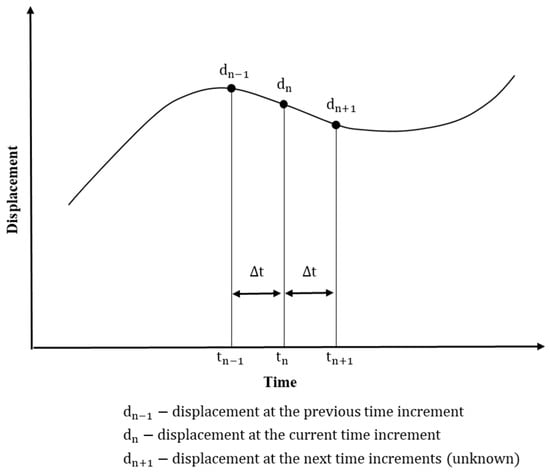
Figure 1
Open AccessArticle
Assessment of Local Radial Basis Function Collocation Method for Diffusion Problems Structured with Multiquadrics and Polyharmonic Splines
by
Izaz Ali, Umut Hanoglu, Robert Vertnik and Božidar Šarler
Math. Comput. Appl. 2024, 29(2), 23; https://doi.org/10.3390/mca29020023 - 17 Mar 2024
Abstract
This paper aims to systematically assess the local radial basis function collocation method, structured with multiquadrics (MQs) and polyharmonic splines (PHSs), for solving steady and transient diffusion problems. The boundary value test involves a rectangle with Dirichlet, Neuman, and Robin boundary conditions, and
[...] Read more.
This paper aims to systematically assess the local radial basis function collocation method, structured with multiquadrics (MQs) and polyharmonic splines (PHSs), for solving steady and transient diffusion problems. The boundary value test involves a rectangle with Dirichlet, Neuman, and Robin boundary conditions, and the initial value test is associated with the Dirichlet jump problem on a square. The spectra of the free parameters of the method, i.e., node density, timestep, shape parameter, etc., are analyzed in terms of the average error. It is found that the use of MQs is less stable compared to PHSs for irregular node arrangements. For MQs, the most suitable shape parameter is determined for multiple cases. The relationship of the shape parameter with the total number of nodes, average error, node scattering factor, and the number of nodes in the local subdomain is also provided. For regular node arrangements, MQs produce slightly more accurate results, while for irregular node arrangements, PHSs provide higher accuracy than MQs. PHSs are recommended for use in diffusion problems that require irregular node spacing.
Full article
(This article belongs to the Special Issue Radial Basis Functions)
►▼
Show Figures
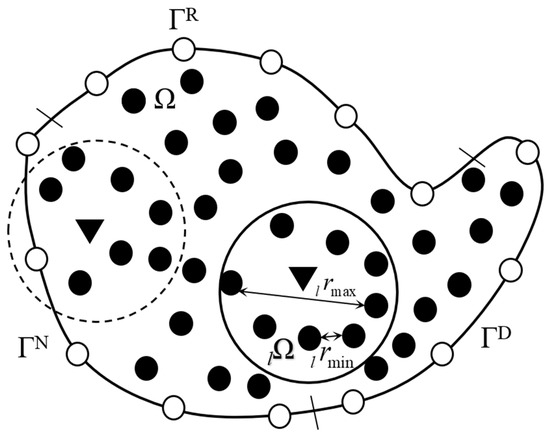
Figure 1
Open AccessArticle
Variability on Functionally Graded Plates’ Deflection Due to Uncertainty on Carbon Nanotubes’ Properties
by
Alda Carvalho, Ana Martins, Ana F. Mota and Maria A. R. Loja
Math. Comput. Appl. 2024, 29(2), 22; https://doi.org/10.3390/mca29020022 - 16 Mar 2024
Abstract
Carbon nanotubes are widely used as material reinforcement in diverse fields of engineering. Being that their contribution is significant to improving the mean properties of the resulting materials, it is important to assess the influence of the variability on carbon nanotubes’ material and
[...] Read more.
Carbon nanotubes are widely used as material reinforcement in diverse fields of engineering. Being that their contribution is significant to improving the mean properties of the resulting materials, it is important to assess the influence of the variability on carbon nanotubes’ material and geometrical properties to structures’ responses. This work considers functionally graded plates constituted by an aluminum continuous phase reinforced with single-walled or multi-walled carbon. The nanotubes' weight fraction evolution through the thickness is responsible for the plates’ functional gradient. The plates’ samples are simulated considering that only the nanotubes’ material and geometrical characteristics are affected by uncertainty. The results obtained from the multiple regression models developed allow us to conclude that the length of the nanotubes has no impact on the maximum transverse displacement of the plates in opposition to the carbon nanotubes’ weight fraction evolution, their internal and external diameters, and the Young’s modulus. The multiple regression models developed can be used as alternative prediction tools within the domain of the study.
Full article
(This article belongs to the Special Issue Mathematical and Computational Approaches in Applied Mechanics: A Themed Issue Dedicated to Professor J.N. Reddy)
►▼
Show Figures
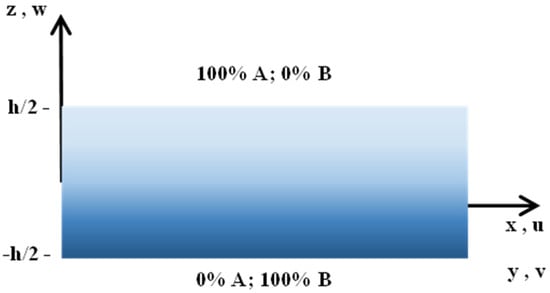
Figure 1
Open AccessArticle
Semi-Supervised Machine Learning Method for Predicting Observed Individual Risk Preference Using Gallup Data
by
Faroque Ahmed, Mrittika Shamsuddin, Tanzila Sultana and Rittika Shamsuddin
Math. Comput. Appl. 2024, 29(2), 21; https://doi.org/10.3390/mca29020021 - 15 Mar 2024
Abstract
Risk and uncertainty play a vital role in almost every significant economic decision, and an individual’s propensity to make riskier decisions also depends on various circumstances. This article aims to investigate the effects of social and economic covariates on an individual’s willingness to
[...] Read more.
Risk and uncertainty play a vital role in almost every significant economic decision, and an individual’s propensity to make riskier decisions also depends on various circumstances. This article aims to investigate the effects of social and economic covariates on an individual’s willingness to take general risks and extends the scope of existing works by using quantitative measures of risk-taking from the GPS and Gallup datasets (in addition to the qualitative measures used in the literature). Based on the available observed risk-taking data for one year, this article proposes a semi-supervised machine learning-based approach that can efficiently predict the observed risk index for those countries/individuals for years when the observed risk-taking index was not collected. We find that linear models are insufficient to capture certain patterns among risk-taking factors, and non-linear models, such as random forest regression, can obtain better root mean squared values than those reported in past literature. In addition to finding factors that agree with past studies, we also find that subjective well-being influences risk-taking behavior.
Full article
(This article belongs to the Section Social Sciences)
►▼
Show Figures
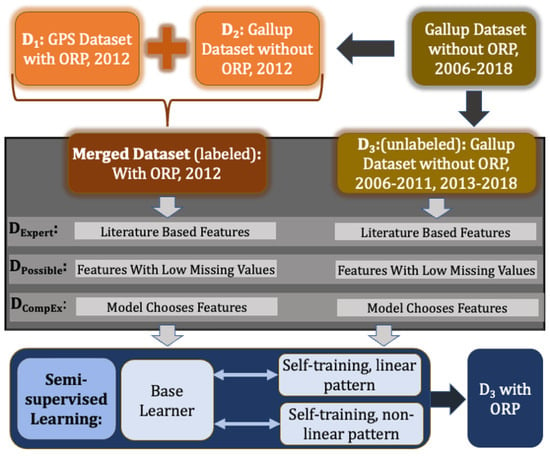
Figure 1
Open AccessArticle
A Four-Variable Shear Deformation Theory for the Static Analysis of FG Sandwich Plates with Different Porosity Models
by
Rabab A. Alghanmi and Rawan H. Aljaghthami
Math. Comput. Appl. 2024, 29(2), 20; https://doi.org/10.3390/mca29020020 - 08 Mar 2024
Cited by 1
Abstract
►▼
Show Figures
This study is centered on examining the static bending behavior of sandwich plates featuring functionally graded materials, specifically addressing distinct representations of porosity distribution across their thickness. The composition of the sandwich plate involves a ceramic core and two face sheets with functionally
[...] Read more.
This study is centered on examining the static bending behavior of sandwich plates featuring functionally graded materials, specifically addressing distinct representations of porosity distribution across their thickness. The composition of the sandwich plate involves a ceramic core and two face sheets with functionally graded properties. Mechanical loads with a sinusoidal distribution are applied to the sandwich plate, and a four-variable shear deformation theory is employed to establish the displacement field. Notably, this theory involves only four unknowns, distinguishing it from alternative shear deformation theories. Equilibrium equations are derived using the virtual work concept, and Navier’s method is applied to obtain the solution. The study addresses the impact of varying porosities, inhomogeneity parameters, aspect ratios, and side-to-thickness ratios on the static bending behavior of the sandwich plates. The influence of various porosities, inhomogeneity parameter, aspect ratio, and side-to-thickness ratio of the sandwich plates are explored and compared in the context of static bending behavior. The three porosity distributions are compared in terms of their influence on the bending behavior of the sandwich plate. The findings indicate that a higher porosity causes larger deflections and Model A has the highest central deflection. Adopting the four-variable shear deformation theory demonstrated its validity since the results were similar to those obtained in the literature. Several important findings have been found, which could be useful in the construction and application of FG sandwich structures. Examples of comparison will be discussed to support the existing theory’s accuracy. Further findings are presented to serve as benchmarks for comparison.
Full article
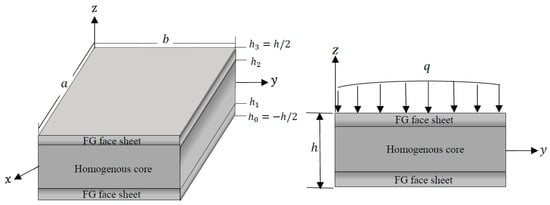
Figure 1
Open AccessArticle
SSA-Deep Learning Forecasting Methodology with SMA and KF Filters and Residual Analysis
by
Juan Frausto-Solís, José Christian de Jesús Galicia-González, Juan Javier González-Barbosa, Guadalupe Castilla-Valdez and Juan Paulo Sánchez-Hernández
Math. Comput. Appl. 2024, 29(2), 19; https://doi.org/10.3390/mca29020019 - 05 Mar 2024
Abstract
Accurate forecasting remains a challenge, even with advanced techniques like deep learning (DL), ARIMA, and Holt–Winters (H&W), particularly for chaotic phenomena such as those observed in several areas, such as COVID-19, energy, and financial time series. Addressing this, we introduce a Forecasting Method
[...] Read more.
Accurate forecasting remains a challenge, even with advanced techniques like deep learning (DL), ARIMA, and Holt–Winters (H&W), particularly for chaotic phenomena such as those observed in several areas, such as COVID-19, energy, and financial time series. Addressing this, we introduce a Forecasting Method with Filters and Residual Analysis (FMFRA), a hybrid methodology specifically applied to datasets of COVID-19 time series, which we selected for their complexity and exemplification of current forecasting challenges. FMFFRA consists of the following two approaches: FMFRA-DL, employing deep learning, and FMFRA-SSA, using singular spectrum analysis. This proposed method applies the following three phases: filtering, forecasting, and residual analysis. Initially, each time series is split into filtered and residual components. The second phase involves a simple fine-tuning for the filtered time series, while the third phase refines the forecasts and mitigates noise. FMFRA-DL is adept at forecasting complex series by distinguishing primary trends from insufficient relevant information. FMFRA-SSA is effective in data-scarce scenarios, enhancing forecasts through automated parameter search and residual analysis. Chosen for their geographical and substantial populations and chaotic dynamics, time series for Mexico, the United States, Colombia, and Brazil permitted a comparative perspective. FMFRA demonstrates its efficacy by improving the common forecasting performance measures of MAPE by 22.91%, DA by 13.19%, and RMSE by 25.24% compared to the second-best method, showcasing its potential for providing essential insights into various rapidly evolving domains.
Full article
(This article belongs to the Topic Mathematical Modeling)
►▼
Show Figures
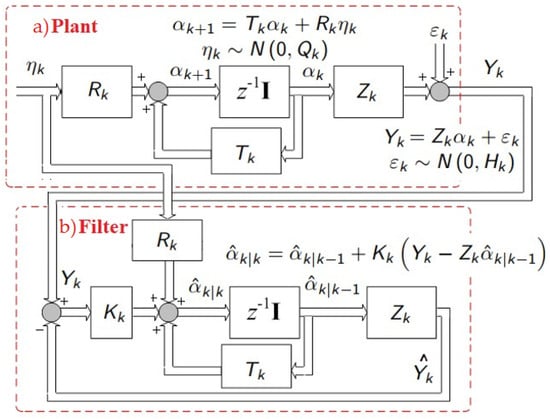
Figure 1
Open AccessArticle
Energy-and-Blocking-Aware Routing and Device Assignment in Software-Defined Networking—A MILP and Genetic Algorithm Approach
by
Gerardo J. Riveros-Rojas, Pedro P. Cespedes-Sanchez, Diego P. Pinto-Roa and Horacio Legal-Ayala
Math. Comput. Appl. 2024, 29(2), 18; https://doi.org/10.3390/mca29020018 - 04 Mar 2024
Abstract
Internet energy consumption has increased rapidly, and energy conservation has become a significant issue that requires focused research efforts. The most promising solution is to identify the minimum power subsets within the network and shut down unnecessary network devices and links to satisfy
[...] Read more.
Internet energy consumption has increased rapidly, and energy conservation has become a significant issue that requires focused research efforts. The most promising solution is to identify the minimum power subsets within the network and shut down unnecessary network devices and links to satisfy traffic loads. Due to their distributed network control, implementing a centralized and coordinated strategy in traditional networks is challenging. Software-Defined Networking (SDN) is an emerging technology with dynamic, manageable, cost-effective, and adaptable solutions. SDN decouples network control and forwarding functions, allowing network control to be directly programmable, centralizing control with a global network view to manage power states. Nevertheless, it is crucial to develop efficient algorithms that leverage the centralized control of SDN to achieve maximum energy savings and consider peak traffic times. Traffic demand usually cannot be satisfied, even when all network devices are active. This work jointly addresses the routing of traffic flows and the assignment of SDN devices to these flows, called the Routing and Device Assignment (RDA) problem. It simultaneously seeks to minimize the network’s energy consumption and blocked traffic flows. For this approach, we develop an exact solution based on Mixed-Integer Linear Programming (MILP) as well as a metaheuristic based on a Genetic Algorithm (GA) that seeks to optimize both criteria by routing flows efficiently and suspending devices not used by the flows. Conducted simulations on traffic environment scenarios show up to 34% savings in overall energy consumption for the MILP and 33% savings achieved by the GA. These values are better than those obtained using competitive state-of-the-art strategies.
Full article
(This article belongs to the Section Engineering)
►▼
Show Figures
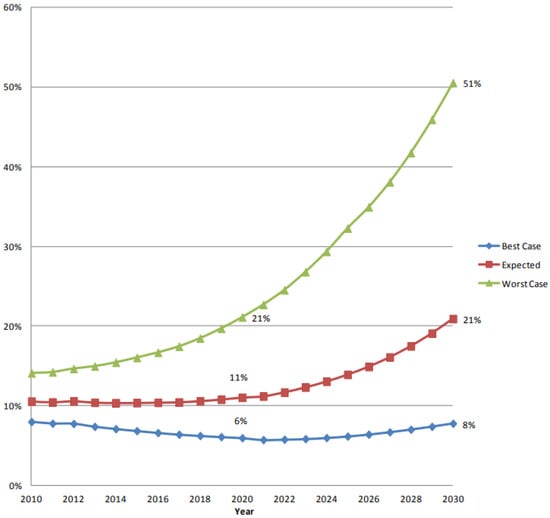
Figure 1
Open AccessArticle
An Iterative Method for Computing π by Argument Reduction of the Tangent Function
by
Sanjar M. Abrarov, Rehan Siddiqui, Rajinder Kumar Jagpal and Brendan M. Quine
Math. Comput. Appl. 2024, 29(2), 17; https://doi.org/10.3390/mca29020017 - 25 Feb 2024
Abstract
In this work, we develop a new iterative method for computing the digits of by argument reduction of the tangent function. This method combines a modified version of the iterative formula for with squared convergence that we proposed in a previous
[...] Read more.
In this work, we develop a new iterative method for computing the digits of by argument reduction of the tangent function. This method combines a modified version of the iterative formula for with squared convergence that we proposed in a previous work and a leading arctangent term from the Machin-like formula. The computational test we performed shows that algorithmic implementation can provide more than 17 digits of per increment. Mathematica codes, showing the convergence rate for computing the digits of , are presented.
Full article
Open AccessArticle
Three-Dimensional Model for Bioventing: Mathematical Solution, Calibration and Validation
by
Mohammad Khodabakhshi Soureshjani, Hermann J. Eberl and Richard G. Zytner
Math. Comput. Appl. 2024, 29(1), 16; https://doi.org/10.3390/mca29010016 - 19 Feb 2024
Abstract
Bioventing is an established technique extensively employed in the remediation of soil contaminated with petroleum hydrocarbons. In this study, the objective was to develop an improved foundational bioventing model that characterizes gas flow in vadose zones where aqueous and non-aqueous phase liquid (NAPL)
[...] Read more.
Bioventing is an established technique extensively employed in the remediation of soil contaminated with petroleum hydrocarbons. In this study, the objective was to develop an improved foundational bioventing model that characterizes gas flow in vadose zones where aqueous and non-aqueous phase liquid (NAPL) are present and immobile, accounting for interphase mass transfer and first order biodegradation kinetics. By incorporating a correlation for the biodegradation rate constant, which is a function of soil properties including initial population of petroleum degrader microorganisms in soil, sand content, clay content, water content, and soil organic matter content, this model offers the ability to integrate a specific biodegradation rate constant tailored to the soil properties for each site. The governing equations were solved using the finite volume method in OpenFOAM employing the “porousMultiphaseFoam v2107” (PMF) toolbox. The equation describing gas flow in unsaturated soil was solved using a mixed pressure-saturation method, where calculated values were employed to solve the component transport equations. Calibration was done against a set of experimental data for a meso-scale reactor considering contaminant volatilization rate as the pre-calibration parameter and the mass transfer coefficient between aqueous and NAPL phase as the main calibration parameter. The calibrated model then was validated by simulating a large-scale reactor. The modelling results showed an error of 2.9% for calibrated case and 4.7% error for validation case which present the fitness to the experimental data, proving that the enhanced bioventing model holds the potential to improve predictions of bioventing and facilitate the development of efficient strategies to remediate soil contaminated with petroleum hydrocarbons.
Full article
(This article belongs to the Collection Feature Papers in Mathematical and Computational Applications 2024)
►▼
Show Figures
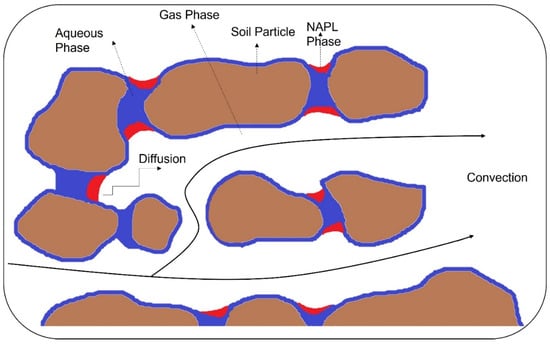
Figure 1
Open AccessArticle
Complex Connections between Symmetry and Singularity Analysis
by
Asghar Qadir
Math. Comput. Appl. 2024, 29(1), 15; https://doi.org/10.3390/mca29010015 - 19 Feb 2024
Abstract
In this paper, it is noted that three apparently disparate areas of mathematics—singularity analysis, complex symmetry analysis and the distributional representation of special functions—have a basic commonality in the underlying methods used. The insights obtained from the first of these provides a much-needed
[...] Read more.
In this paper, it is noted that three apparently disparate areas of mathematics—singularity analysis, complex symmetry analysis and the distributional representation of special functions—have a basic commonality in the underlying methods used. The insights obtained from the first of these provides a much-needed explanation for the effectiveness of the latter two. The consequent explanations are provided in the form of two theorems and their corollaries.
Full article
(This article belongs to the Special Issue Symmetry Methods for Solving Differential Equations)
Open AccessArticle
On the Parallelization of Square-Root Vélu’s Formulas
by
Jorge Chávez-Saab, Odalis Ortega and Amalia Pizarro-Madariaga
Math. Comput. Appl. 2024, 29(1), 14; https://doi.org/10.3390/mca29010014 - 16 Feb 2024
Abstract
A primary challenge in isogeny-based cryptography lies in the substantial computational cost associated to computing and evaluating prime-degree isogenies. This computation traditionally relied on Vélu’s formulas, an approach with time complexity linear in the degree but which was further enhanced by Bernstein, De
[...] Read more.
A primary challenge in isogeny-based cryptography lies in the substantial computational cost associated to computing and evaluating prime-degree isogenies. This computation traditionally relied on Vélu’s formulas, an approach with time complexity linear in the degree but which was further enhanced by Bernstein, De Feo, Leroux, and Smith to a square-root complexity. The improved square-root Vélu’s formulas exhibit a degree of parallelizability that has not been exploited in major implementations. In this study, we introduce a theoretical framework for parallelizing isogeny computations and provide a proof-of-concept implementation in C with OpenMP. While the parallelization effectiveness exhibits diminishing returns with the number of cores, we still obtain strong results when using a small number of cores. Concretely, our implementation shows that for large degrees it is easy to achieve speedup factors of up to , , and for two, four, and eight cores, respectively.
Full article
(This article belongs to the Topic Mathematical Modeling)
►▼
Show Figures
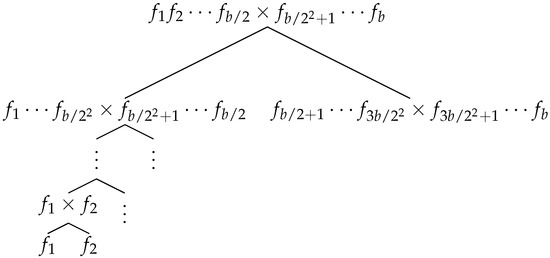
Figure 1
Open AccessArticle
Magnesium and Calcium Transport along the Male Rat Kidney: Effect of Diuretics
by
Pritha Dutta and Anita T. Layton
Math. Comput. Appl. 2024, 29(1), 13; https://doi.org/10.3390/mca29010013 - 07 Feb 2024
Abstract
Calcium (Ca2+) and magnesium (Mg2+) are essential for cellular function. The kidneys play an important role in maintaining the homeostasis of these cations. Their reabsorption along the nephron is dependent on distinct trans- and paracellular pathways and is coupled
[...] Read more.
Calcium (Ca2+) and magnesium (Mg2+) are essential for cellular function. The kidneys play an important role in maintaining the homeostasis of these cations. Their reabsorption along the nephron is dependent on distinct trans- and paracellular pathways and is coupled to the transport of other electrolytes. Notably, sodium (Na+) transport establishes an electrochemical gradient to drive Ca2+ and Mg2+ reabsorption. Consequently, alterations in renal Na+ handling, under pathophysiological conditions or pharmacological manipulations, can have major effects on Ca2+ and Mg2+ transport. One such condition is the administration of diuretics, which are used to treat a large range of clinical conditions, but most commonly for the management of blood pressure and fluid balance. While the pharmacological targets of diuretics typically directly mediate Na+ transport, they also indirectly affect renal Ca2+ and Mg2+ handling through alterations in the electrochemical gradient. To investigate renal Ca2+ and Mg2 handling and how those processes are affected by diuretic treatment, we have developed computational models of electrolyte transport along the nephrons. Model simulations indicate that along the proximal tubule and thick ascending limb, the transport of Ca2+ and Mg2+ occurs in parallel with Na+, but those processes are dissociated along the distal convoluted tubule. We also simulated the effects of acute administration of loop, thiazide, and K-sparing diuretics. The model predicted significantly increased Ca2+ and Mg2+ excretions and significantly decreased Ca2+ and Mg2+ excretions on treatment with loop and K-sparing diuretics, respectively. Treatment with thiazide diuretics significantly decreased Ca2+ excretion, but there was no significant alteration in Mg2+ excretion. The present models can be used to conduct in silico studies on how the kidney adapts to alterations in Ca2+ and Mg2+ homeostasis during various physiological and pathophysiological conditions, such as pregnancy, diabetes, and chronic kidney disease.
Full article
(This article belongs to the Special Issue Recent Advances and New Challenges in Coupled Systems and Networks: Theory, Modelling, and Applications)
►▼
Show Figures
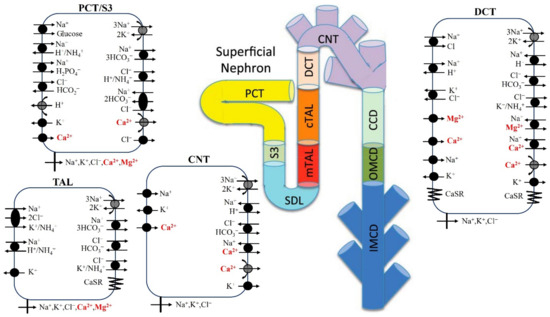
Figure 1
Highly Accessed Articles
Latest Books
E-Mail Alert
News
Topics
Topic in
Algorithms, Computation, Entropy, Fractal Fract, MCA
Analytical and Numerical Methods for Stochastic Biological Systems
Topic Editors: Mehmet Yavuz, Necati Ozdemir, Mouhcine Tilioua, Yassine SabbarDeadline: 10 May 2024
Topic in
Axioms, Computation, MCA, Mathematics, Symmetry
Mathematical Modeling
Topic Editors: Babak Shiri, Zahra AlijaniDeadline: 31 May 2024
Topic in
Axioms, Computation, Entropy, MCA, Mathematics, Symmetry
Numerical Methods for Partial Differential Equations
Topic Editors: Pengzhan Huang, Yinnian HeDeadline: 30 June 2024
Topic in
BDCC, Entropy, Information, MCA, Mathematics
New Advances in Granular Computing and Data Mining
Topic Editors: Xibei Yang, Bin Xie, Pingxin Wang, Hengrong JuDeadline: 30 October 2024

Conferences
Special Issues
Special Issue in
MCA
Mathematical and Computational Approaches in Applied Mechanics: A Themed Issue Dedicated to Professor J.N. Reddy
Guest Editors: Michele Bacciocchi, Eugenio Ruocco, Maria Amélia Ramos Loja, Nicholas Fantuzzi, Jose LoyaDeadline: 31 May 2024
Special Issue in
MCA
Blockchain-Based Solutions for Information Governance
Guest Editors: Kiran Khatter, Arpit Bhardwaj, Ziya Uddin, Rohit VermaDeadline: 3 August 2024
Special Issue in
MCA
Advancements in Mathematical Models, Probability Distributions, and Digital Twins: Bridging the Gap between Theory and Practice
Guest Editor: Sandra FerreiraDeadline: 15 September 2024
Special Issue in
MCA
Recent Advances and New Challenges in Coupled Systems and Networks: Theory, Modelling, and Applications
Guest Editors: Weizhong Dai, Sundeep SinghDeadline: 15 October 2024
Topical Collections
Topical Collection in
MCA
Feature Papers in Mathematical and Computational Applications
Collection Editors: Gianluigi Rozza, Oliver Schütze, Nicholas Fantuzzi
Topical Collection in
MCA
Feature Papers in Mathematical and Computational Applications 2023
Collection Editors: Gianluigi Rozza, Oliver Schütze, Nicholas Fantuzzi
Topical Collection in
MCA
Feature Papers in Mathematical and Computational Applications 2024
Collection Editors: Gianluigi Rozza, Oliver Schütze, Nicholas Fantuzzi