Journal Description
Econometrics
Econometrics
is an international, peer-reviewed, open access journal on econometric modeling and forecasting, as well as new advances in econometrics theory, and is published quarterly online by MDPI.
- Open Access— free for readers, with article processing charges (APC) paid by authors or their institutions.
- High Visibility: indexed within Scopus, ESCI (Web of Science), EconLit, EconBiz, RePEc, and other databases.
- Rapid Publication: manuscripts are peer-reviewed and a first decision is provided to authors approximately 34.6 days after submission; acceptance to publication is undertaken in 8.6 days (median values for papers published in this journal in the first half of 2025).
- Recognition of Reviewers: reviewers who provide timely, thorough peer-review reports receive vouchers entitling them to a discount on the APC of their next publication in any MDPI journal, in appreciation of the work done.
Impact Factor:
1.4 (2024);
5-Year Impact Factor:
1.2 (2024)
Latest Articles
Analyzing the Impact of Carbon Mitigation on the Eurozone’s Trade Dynamics with the US and China
Econometrics 2025, 13(3), 28; https://doi.org/10.3390/econometrics13030028 - 29 Jul 2025
Abstract
►
Show Figures
This study focusses on the transmission of carbon pricing mechanisms in shaping trade dynamics between the Eurozone and key partners: the USA and China. Using Bayesian variable selection methods and a Time-Varying Structural Vector Autoregressions (TV-SVAR) model, the research identifies the key variables
[...] Read more.
This study focusses on the transmission of carbon pricing mechanisms in shaping trade dynamics between the Eurozone and key partners: the USA and China. Using Bayesian variable selection methods and a Time-Varying Structural Vector Autoregressions (TV-SVAR) model, the research identifies the key variables impacting EU carbon emissions over time. The results reveal that manufactured products from the US have a diminishing positive impact on EU carbon emissions, suggesting potential exemption from future regulations. In contrast, manufactured goods from the US and petroleum products from China are expected to increase emissions, indicating a need for stricter trade policies. These findings provide strategic insights for policymakers aiming to balance trade and environmental objectives.
Full article
Open AccessArticle
Pseudo-Panel Decomposition of the Blinder–Oaxaca Gender Wage Gap
by
Jhon James Mora and Diana Yaneth Herrera
Econometrics 2025, 13(3), 27; https://doi.org/10.3390/econometrics13030027 (registering DOI) - 19 Jul 2025
Abstract
This article introduces a novel approach to decomposing the Blinder–Oaxaca gender wage gap using pseudo-panel data. In many developing countries, panel data are not available; however, understanding the evolution of the gender wage gap over time requires tracking individuals longitudinally. When individuals change
[...] Read more.
This article introduces a novel approach to decomposing the Blinder–Oaxaca gender wage gap using pseudo-panel data. In many developing countries, panel data are not available; however, understanding the evolution of the gender wage gap over time requires tracking individuals longitudinally. When individuals change across time periods, estimators tend to be inconsistent and inefficient. To address this issue, and building upon the traditional Blinder–Oaxaca methodology, we propose an alternative procedure that follows cohorts over time rather than individuals. This approach enables the estimation of both the explained and unexplained components—“endowment effect” and “remuneration effect”—of the wage gap, along with their respective standard errors, even in the absence of true panel data. We apply this methodology to the case of Colombia, finding a gender wage gap of approximately 15% in favor of male cohorts. This gap comprises a −5.6% explained component and a 20% unexplained component without controls. When we control by informality, size of the firm and sector the gap comprises a −3.5% explained component and a 18.7% unexplained component.
Full article
Open AccessArticle
Daily Emissions of CO2 in the World: A Fractional Integration Approach
by
Luis Alberiko Gil-Alana and Carlos Poza
Econometrics 2025, 13(3), 26; https://doi.org/10.3390/econometrics13030026 - 17 Jul 2025
Abstract
►▼
Show Figures
In this article, daily CO2 emissions for the years 2019–2022 are examined using fractional integration for Brazil, China, EU-27 (and the UK), India, and the USA. According to the findings, all series exhibit long memory mean-reversion tendencies, with orders of integration ranging
[...] Read more.
In this article, daily CO2 emissions for the years 2019–2022 are examined using fractional integration for Brazil, China, EU-27 (and the UK), India, and the USA. According to the findings, all series exhibit long memory mean-reversion tendencies, with orders of integration ranging between 0.22 in the case of India (with white noise errors) and 0.70 for Brazil (under autocorrelated disturbances). Nevertheless, the differencing parameter estimates are all considerably below 1, which supports the theory of mean reversion and transient shocks. These results suggest the need for a greater intensification of green policies complemented with economic structural reforms to achieve the zero-emissions target by 2050.
Full article
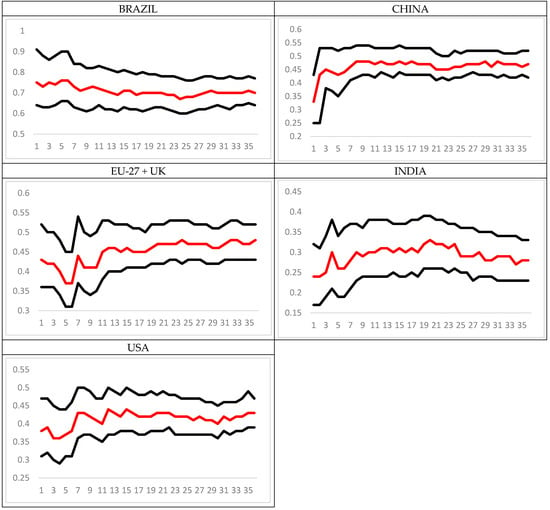
Figure 1
Open AccessArticle
The Long-Run Impact of Changes in Prescription Drug Sales on Mortality and Hospital Utilization in Belgium, 1998–2019
by
Frank R. Lichtenberg
Econometrics 2025, 13(3), 25; https://doi.org/10.3390/econometrics13030025 - 23 Jun 2025
Abstract
►▼
Show Figures
Objectives: We investigate the long-run impact of changes in prescription drug sales on mortality and hospital utilization in Belgium during the first two decades of the 21st century. Methods: We analyze the correlation across diseases between changes in the drugs used to treat
[...] Read more.
Objectives: We investigate the long-run impact of changes in prescription drug sales on mortality and hospital utilization in Belgium during the first two decades of the 21st century. Methods: We analyze the correlation across diseases between changes in the drugs used to treat the disease and changes in mortality or hospital utilization from that disease. The measure of the change in prescription drug sales we use is the long-run (1998–2018 or 2000–2019) change in the fraction of post-1999 drugs sold. A post-1999 drug is a drug that was not sold during 1989–1999. Results: The 1998–2018 increase in the fraction of post-1999 drugs sold is estimated to have reduced the number of years of life lost before ages 85, 75, and 65 in 2018 by about 438 thousand (31%), 225 thousand (31%), and 114 thousand (32%), respectively. The 1995–2014 increase in in the fraction of post-1999 drugs sold is estimated to have reduced the number of hospital days in 2019 by 2.66 million (20%). Conclusions: Even if we ignore the reduction in hospital utilization attributable to changes in pharmaceutical consumption, a conservative estimate of the 2018 cost per life-year before age 85 gained is EUR 6824. We estimate that previous changes in pharmaceutical consumption reduced 2019 expenditure on inpatient curative and rehabilitative care by EUR 3.55 billion, which is higher than the 2018 expenditure on drugs that were authorized during the period 1998–2018: EUR 2.99 billion.
Full article
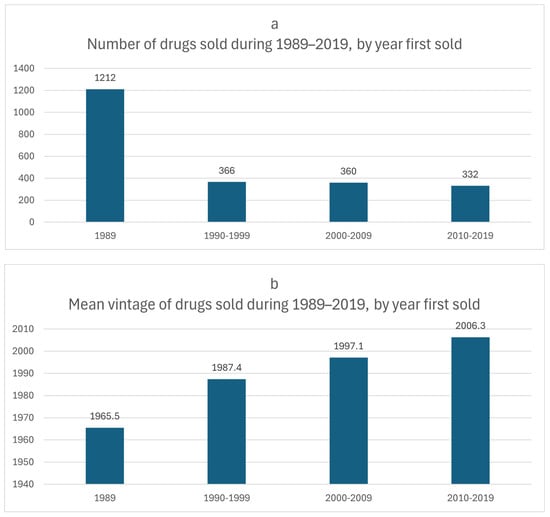
Figure 1
Open AccessArticle
The Effect of Macroeconomic Announcements on U.S. Treasury Markets: An Autometric General-to-Specific Analysis of the Greenspan Era
by
James J. Forest
Econometrics 2025, 13(3), 24; https://doi.org/10.3390/econometrics13030024 - 21 Jun 2025
Abstract
This research studies the impact of macroeconomic announcement surprises on daily U.S. Treasury excess returns during the heart of Alan Greenspan’s tenure as Federal Reserve Chair, addressing the possible limitations of standard static regression (SSR) models, which may suffer from omitted variable bias,
[...] Read more.
This research studies the impact of macroeconomic announcement surprises on daily U.S. Treasury excess returns during the heart of Alan Greenspan’s tenure as Federal Reserve Chair, addressing the possible limitations of standard static regression (SSR) models, which may suffer from omitted variable bias, parameter instability, and poor mis-specification diagnostics. To complement the SSR framework, an automated general-to-specific (Gets) modeling approach, enhanced with modern indicator saturation methods for robustness, is applied to improve empirical model discovery and mitigate potential biases. By progressively reducing an initially broad set of candidate variables, the Gets methodology steers the model toward congruence, dispenses unstable parameters, and seeks to limit information loss while seeking model congruence and precision. The findings, herein, suggest that U.S. Treasury market responses to macroeconomic news shocks exhibited stability for a core set of announcements that reliably influenced excess returns. In contrast to computationally costless standard static models, the automated Gets-based approach enhances parameter precision and provides a more adaptive structure for identifying relevant predictors. These results demonstrate the potential value of incorporating interpretable automated model selection techniques alongside traditional SSR and Markov switching approaches to improve empirical insights into macroeconomic announcement effects on financial markets.
Full article
(This article belongs to the Special Issue Advancements in Macroeconometric Modeling and Time Series Analysis)
►▼
Show Figures
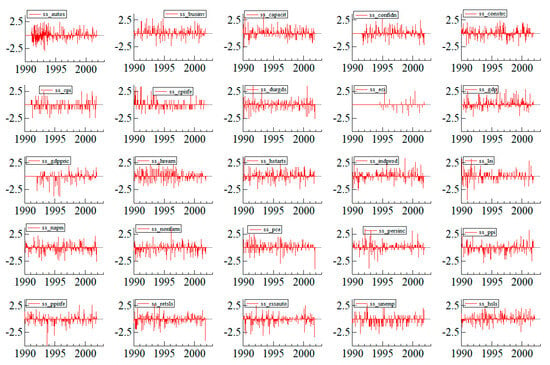
Figure 1
Open AccessArticle
Leveraging Success: The Hidden Peak in Debt and Firm Performance
by
Suzan Dsouza, Krishnamoorthy Kathavarayan, Franklin Mathias, Dharmesh Bhatia and Abdallah AlKhawaja
Econometrics 2025, 13(2), 23; https://doi.org/10.3390/econometrics13020023 - 10 Jun 2025
Abstract
►▼
Show Figures
This study investigates the relationship between capital structure and financial performance in South African firms, focusing on the potential non-linear, inverse U-shaped effect of leverage on profitability. Drawing on data from 1548 firm-year observations covering 183 publicly listed South African companies between 2013
[...] Read more.
This study investigates the relationship between capital structure and financial performance in South African firms, focusing on the potential non-linear, inverse U-shaped effect of leverage on profitability. Drawing on data from 1548 firm-year observations covering 183 publicly listed South African companies between 2013 and 2022, the analysis employs both Fixed Effects (FE) and System Generalized Method of Moments (System-GMM) models to address endogeneity and capture dynamic adjustments. The findings indicate that moderate levels of debt enhance profitability, but excessive leverage leads to diminishing returns, confirming an inverse U-shaped relationship. System-GMM results further reveal the persistence of past profitability and validate the dynamic nature of capital structure decisions. Larger firms appear more capable of sustaining higher leverage without adverse effects, while smaller firms benefit from maintaining lower debt levels. The study concludes that strategic debt management, tailored to firm size and economic context, is critical for optimizing financial performance in emerging markets like South Africa. The study identifies the optimal leverage ratio for South African firms and shows how firm size moderates the relationship between debt and profitability, offering tailored insights for firms of different sizes. These insights offer valuable guidance for managers, investors, and policymakers aiming to strengthen financial stability and efficiency through informed capital structure choices.
Full article
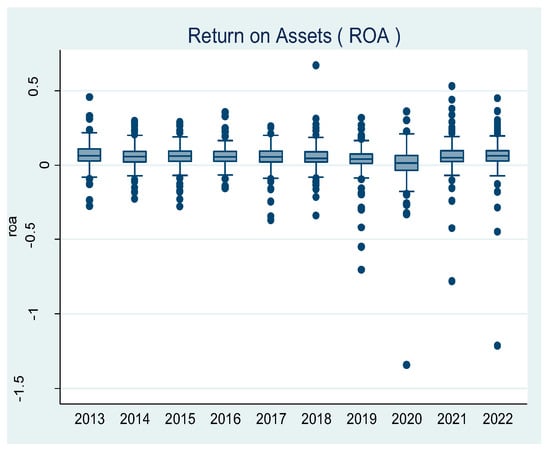
Figure 1
Open AccessArticle
Dependent and Independent Time Series Errors Under Elliptically Countered Models
by
Fredy O. Pérez-Ramirez, Francisco J. Caro-Lopera, José A. Díaz-García and Graciela González Farías
Econometrics 2025, 13(2), 22; https://doi.org/10.3390/econometrics13020022 - 21 May 2025
Abstract
►▼
Show Figures
We explore the impact of time series behavior on model errors when working under an elliptically contoured distribution. By adopting a time series approach aligned with the realistic dependence between errors under such distributions, this perspective shifts the focus from increasingly complex and
[...] Read more.
We explore the impact of time series behavior on model errors when working under an elliptically contoured distribution. By adopting a time series approach aligned with the realistic dependence between errors under such distributions, this perspective shifts the focus from increasingly complex and challenging correlation analyses to volatility modeling that utilizes a novel likelihood framework based on dependent probabilistic samples. With the introduction of a modified Bayesian Information Criterion, which incorporates a ranking of degrees of evidence of significant differences between the compared models, the critical issue of model selection is reinforced, clarifying the relationships among the most common information criteria and revealing limited relevance among the models based on independent probabilistic samples, when tested on a well-established database. Our approach challenges the traditional hierarchical models commonly used in time series analysis, which assume independent errors. The application of rigorous differentiation criteria under this novel perspective on likelihood, based on dependent probabilistic samples, provides a new viewpoint on likelihood that arises naturally in the context of finance, adding a novel result. We provide new results for criterion selection, evidence invariance, and transitions between volatility models and heuristic methods to calibrate nested or non-nested models via convergence properties in a distribution.
Full article
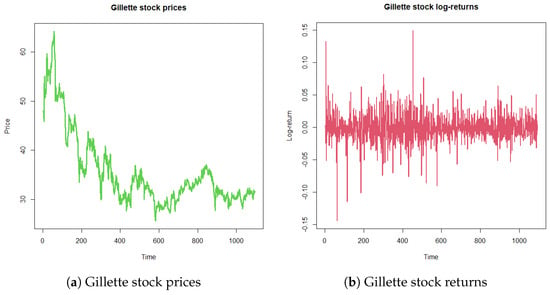
Figure 1
Open AccessArticle
Decomposing the Household Herding Behavior in Stock Investment: The Case of China
by
Yung-Ching Tseng, I.-Fan Hsiao and Guo-Chen Wang
Econometrics 2025, 13(2), 21; https://doi.org/10.3390/econometrics13020021 - 12 May 2025
Abstract
►▼
Show Figures
Financial studies on the herding effect have been very popular for decades, as detecting herding behavior helps to explain price deviations and market inefficiencies. However, studying the herding effect as a single influencing factor is believed to be insufficient to explain the changes
[...] Read more.
Financial studies on the herding effect have been very popular for decades, as detecting herding behavior helps to explain price deviations and market inefficiencies. However, studying the herding effect as a single influencing factor is believed to be insufficient to explain the changes in investment behavior, as the herding effect itself may be caused by other influencing factors. In other words, the issue must be studied alongside other factors. In this study, we adopt the quantile regression model to comprehensively understand the herding effect’s influence on household investment in China, and the empirical results indicate that herding behavior leads to different investment outcomes for households in different scenarios. In this analysis, we consider a variety of household characteristics, such as income level and risk tolerance, to provide a nuanced understanding of investment behavior. Additionally, in this study, we explore the interaction between herding behavior and macroeconomic variables. Nevertheless, the results suggest that, if herding behavior can be reduced by the head of the household, profitability can be increased, or at the very least, losses can be reduced.
Full article
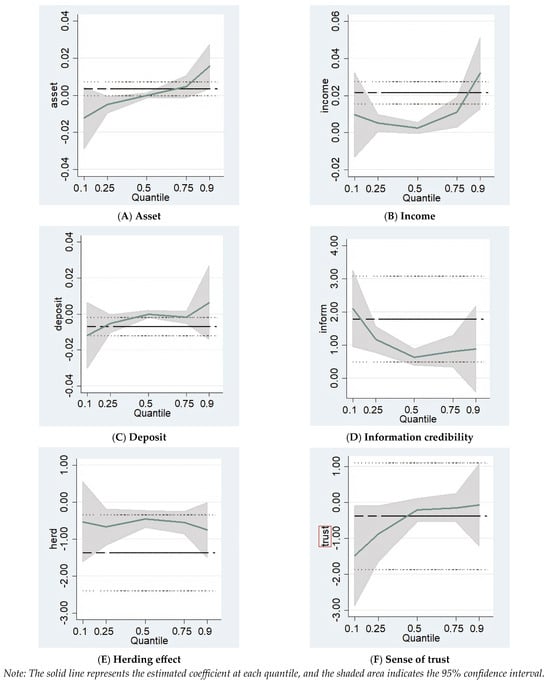
Figure 1
Open AccessArticle
Government Subsidies and Industrial Productivity in South Africa: A Focus on the Channels
by
Brian Tavonga Mazorodze
Econometrics 2025, 13(2), 20; https://doi.org/10.3390/econometrics13020020 - 1 May 2025
Cited by 1
Abstract
►▼
Show Figures
This article estimates the impact of government subsidies on productivity growth in South Africa, joining the ongoing debate among economists regarding the effectiveness of subsidies as a driver of industrial productivity. While some argue that subsidies address market failures, facilitate R&D, and improve
[...] Read more.
This article estimates the impact of government subsidies on productivity growth in South Africa, joining the ongoing debate among economists regarding the effectiveness of subsidies as a driver of industrial productivity. While some argue that subsidies address market failures, facilitate R&D, and improve efficiency, others criticise the attendant dependence, which reduces the incentive for industries to operate efficiently. This article contributes by examining the specific channels—efficiency and technical changes—through which subsidies affect productivity in South Africa. The analysis is based on a panel dataset comprising 64 three-digit industries observed between 1993 and 2023. Estimation is performed through an endogeneity robust panel stochastic frontier model, which treats subsidies as both an inefficiency driver and a technology variable. An additional estimation approach is proposed integrating the true fixed effects with a control function in a bid to account for both unobserved heterogeneity and idiosyncratic endogeneity. The results show that subsidies are detrimental to productivity, particularly through stifling technological progress. This result supports the view that subsidies reduce the incentive for beneficiaries to innovate. This evidence calls for a reevaluation and a possible restructuring of subsidy programmes in South Africa in a bid to mitigate their adverse effects on industrial productivity.
Full article
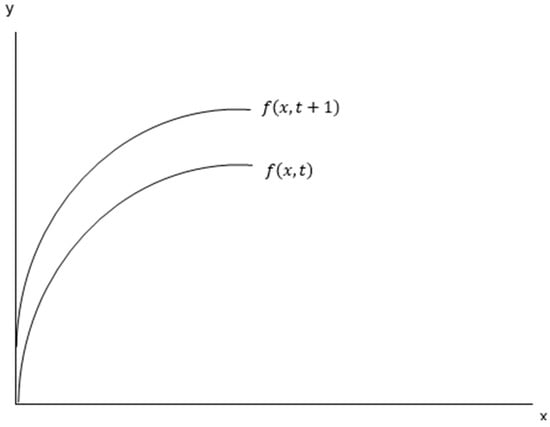
Figure 1
Open AccessArticle
Generalized Recentered Influence Function Regressions
by
Javier Alejo, Antonio Galvao, Julián Martínez-Iriarte and Gabriel Montes-Rojas
Econometrics 2025, 13(2), 19; https://doi.org/10.3390/econometrics13020019 - 18 Apr 2025
Abstract
►▼
Show Figures
This paper suggests a generalization of covariate shifts to study distributional impacts on inequality and distributional measures. It builds on the recentered influence function (RIF) regression method, originally designed for location shifts in covariates, and extends it to general policy interventions, such as
[...] Read more.
This paper suggests a generalization of covariate shifts to study distributional impacts on inequality and distributional measures. It builds on the recentered influence function (RIF) regression method, originally designed for location shifts in covariates, and extends it to general policy interventions, such as location–scale or asymmetric interventions. Numerical simulations for the Gini, Theil, and Atkinson indexes demonstrate strong performance across a myriad of cases and distributional measures. An empirical application examining changes in Mincerian equations is presented to illustrate the method.
Full article
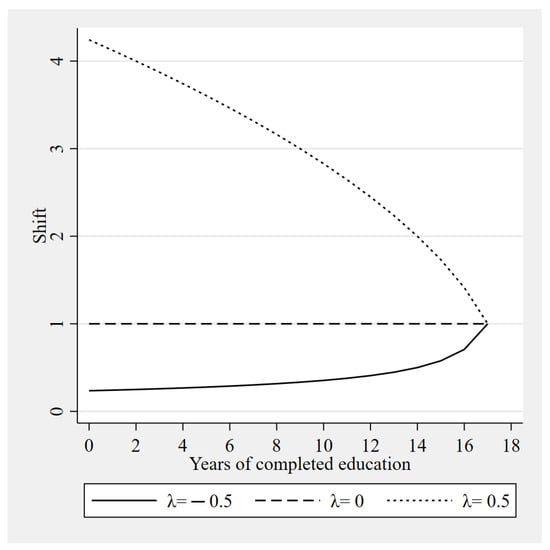
Figure 1
Open AccessArticle
Is VIX a Contrarian Indicator? On the Positivity of the Conditional Sharpe Ratio †
by
Ehud I. Ronn and Liying Xu
Econometrics 2025, 13(2), 18; https://doi.org/10.3390/econometrics13020018 - 14 Apr 2025
Abstract
The notion of compensation for systematic risk is well ingrained in finance and constitutes the basis for numerous empirical tests. The concept an increase in systematic risk is accompanied by an increase in the required risk premium has strong intuitive content: The more
[...] Read more.
The notion of compensation for systematic risk is well ingrained in finance and constitutes the basis for numerous empirical tests. The concept an increase in systematic risk is accompanied by an increase in the required risk premium has strong intuitive content: The more risk there is to be borne, the greater the compensation therefor. In recognizing previous research on the ex ante and ex post reward to risk, the thrust of this paper is to augment those previous tests of expected and realized returns by providing several distinct empirical tests of the proposition the market rewards the undertaking of systematic equity risk, the latter as measured by the VIX volatility index. Thus, in this paper’s empirical section, we use several empirical approaches to answer the question, Using realized returns, is an increase in systematic risk VIX accompanied by an increase in the equity risk premium? While the empirical results are not always statistically significant, our answer is in the affirmative.
Full article
Open AccessArticle
Forecasting Asset Returns Using Nelson–Siegel Factors Estimated from the US Yield Curve
by
Massimo Guidolin and Serena Ionta
Econometrics 2025, 13(2), 17; https://doi.org/10.3390/econometrics13020017 - 11 Apr 2025
Abstract
This paper explores the hypothesis that the returns of asset classes can be predicted using common, systematic risk factors represented by the level, slope, and curvature of the US interest rate term structure. These are extracted using the Nelson–Siegel model, which effectively captures
[...] Read more.
This paper explores the hypothesis that the returns of asset classes can be predicted using common, systematic risk factors represented by the level, slope, and curvature of the US interest rate term structure. These are extracted using the Nelson–Siegel model, which effectively captures the three dimensions of the yield curve. To forecast the factors, we applied autoregressive (AR) and vector autoregressive (VAR) models. Using their forecasts, we predict the returns of government and corporate bonds, equities, REITs, and commodity futures. Our predictions were compared against two benchmarks: the historical mean, and an AR(1) model based on past returns. We employed the Diebold–Mariano test and the Model Confidence Set procedure to assess the comparative forecast accuracy. We found that Nelson–Siegel factors had significant predictive power for one-month-ahead returns of bonds, equities, and REITs, but not for commodity futures. However, for 6-month and 12-month-ahead forecasts, neither the AR(1) nor VAR(1) models based on Nelson–Siegel factors outperformed the benchmarks. These results suggest that the Nelson–Siegel factors affect the aggregate stochastic discount factor for pricing all assets traded in the US economy.
Full article
(This article belongs to the Special Issue Advancements in Macroeconometric Modeling and Time Series Analysis)
►▼
Show Figures
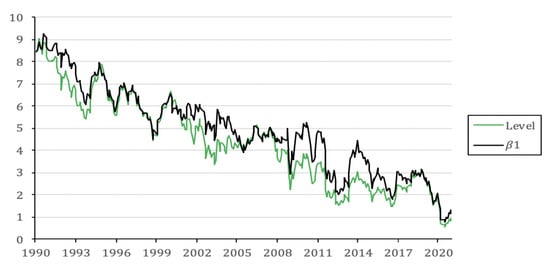
Figure 1
Open AccessArticle
A Meta-Analysis of Determinants of Success and Failure of Economic Sanctions
by
Binyam Afewerk Demena and Peter A. G. van Bergeijk
Econometrics 2025, 13(2), 16; https://doi.org/10.3390/econometrics13020016 - 9 Apr 2025
Abstract
►▼
Show Figures
Political scientists and economists often assert that they understand how economic sanctions function as a foreign policy tool and claim to have backed their theories with compelling statistical evidence. The research puzzle that this article addresses is the observation that despite almost four
[...] Read more.
Political scientists and economists often assert that they understand how economic sanctions function as a foreign policy tool and claim to have backed their theories with compelling statistical evidence. The research puzzle that this article addresses is the observation that despite almost four decades of empirical research on economic sanctions, there is still no consensus on the direction and magnitude of the key variables that theoretically determine the success of economic sanctions. To address part of this research puzzle, we conducted a meta-analysis of 37 studies published between 1985 and 2018, focusing on three key determinants of sanction success: trade linkage, prior relations, and duration. Our analysis examines the factors contributing to the variation in findings reported by these primary studies. By constructing up to 27 moderator variables that capture the contexts in which researchers derive their estimates, we found that the differences across studies are primarily influenced by the data used, the variables controlled for in estimation methods, publication quality, and author characteristics. Our results reveal highly significant effects, indicating that sanctions are more likely to succeed when there is strong pre-sanction trade, when sanctions are implemented swiftly, and when they involve countries with better pre-sanction relationships. In our robustness checks, we consistently confirmed these core findings across different estimation techniques.
Full article
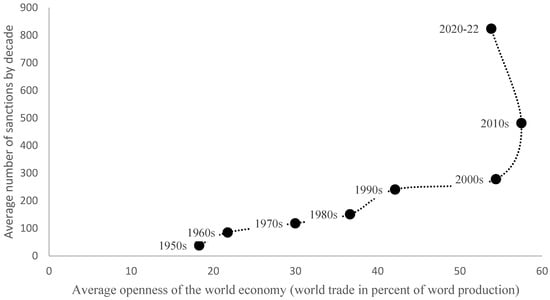
Figure 1
Open AccessArticle
Inference of Impulse Responses via Bayesian Graphical Structural VAR Models
by
Daniel Felix Ahelegbey
Econometrics 2025, 13(2), 15; https://doi.org/10.3390/econometrics13020015 - 2 Apr 2025
Abstract
Impulse response functions (IRFs) are crucial for analyzing the dynamic interactions of macroeconomic variables in vector autoregressive (VAR) models. However, traditional IRF estimation methods often have limitations with assumptions on variable ordering and restrictive identification constraints. This paper applies the Bayesian graphical structural
[...] Read more.
Impulse response functions (IRFs) are crucial for analyzing the dynamic interactions of macroeconomic variables in vector autoregressive (VAR) models. However, traditional IRF estimation methods often have limitations with assumptions on variable ordering and restrictive identification constraints. This paper applies the Bayesian graphical structural vector autoregressive (BGSVAR) model, which integrates structural learning to capture both temporal and contemporaneous dependencies for more accurate impulse response estimation. The BGSVAR framework provides a more efficient and interpretable method for estimating IRFs, which can enhance both forecasting performance and structural inferences in economic modelling. Through extensive simulations across various data-generating processes, we evaluate BGSVAR’s effectiveness in modelling dynamic interactions among US macroeconomic variables. Our results demonstrate that BGSVAR outperforms traditional methods, such as LASSO and Bayesian VAR (BVAR), by delivering more precise impulse response estimates and better capturing the structural dynamics of VAR-based models.
Full article
(This article belongs to the Special Issue Innovations in Bayesian Econometrics: Theory, Techniques, and Economic Analysis)
►▼
Show Figures
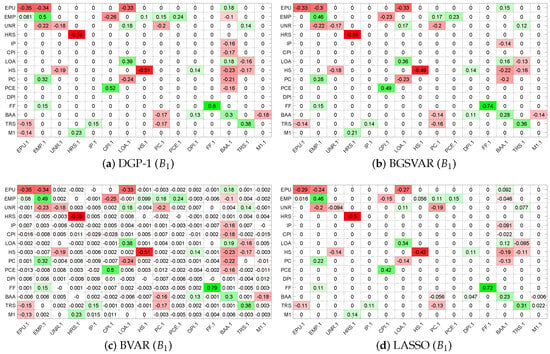
Figure 1
Open AccessArticle
Modeling and Forecasting Time-Series Data with Multiple Seasonal Periods Using Periodograms
by
Solomon Buke Chudo and Gyorgy Terdik
Econometrics 2025, 13(2), 14; https://doi.org/10.3390/econometrics13020014 - 28 Mar 2025
Cited by 2
Abstract
►▼
Show Figures
Applications of high-frequency data, including energy management, economics, and finance, frequently require time-series forecasting characterized by complex seasonality. Recognizing prevailing seasonal trends continues to be difficult, given that the majority of solutions depend on basic decomposition techniques. This study introduces a new approach
[...] Read more.
Applications of high-frequency data, including energy management, economics, and finance, frequently require time-series forecasting characterized by complex seasonality. Recognizing prevailing seasonal trends continues to be difficult, given that the majority of solutions depend on basic decomposition techniques. This study introduces a new approach employing periodograms from spectral density analysis to identify predominant seasonal periods. When analyzing hourly electricity consumption data from Brazil, we identified three significant seasonal patterns: sub-daily (6 h), half-daily (12 h), and daily (24 h). We assessed the predictive efficacy of the BATS, TBATS, and STL + ETS models using these seasonal periods. We performed data analysis and model fitting in R 4.4.1 and used accuracy metrics like MAE, MAPE, and others to compare the models. The STL + ETS model exhibited an enhanced performance, surpassing both BATS and TBATS in energy forecasting. These findings improve our understanding of multiple seasonal patterns, assist us in selecting dominating periods, provide new practical forecasting approaches for time-series analysis, and inform professionals seeking superior forecasting solutions in various fields.
Full article
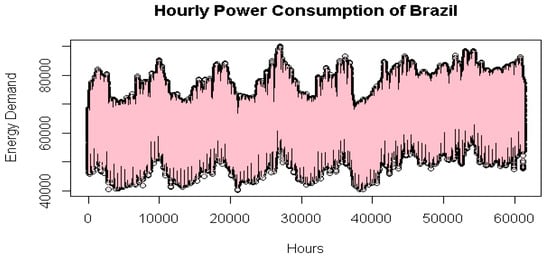
Figure 1
Open AccessArticle
Explosive Episodes and Time-Varying Volatility: A New MARMA–GARCH Model Applied to Cryptocurrencies
by
Alain Hecq and Daniel Velasquez-Gaviria
Econometrics 2025, 13(2), 13; https://doi.org/10.3390/econometrics13020013 - 24 Mar 2025
Cited by 1
Abstract
►▼
Show Figures
Financial assets often exhibit explosive price surges followed by abrupt collapses, alongside persistent volatility clustering. Motivated by these features, we introduce a mixed causal–noncausal invertible–noninvertible autoregressive moving average generalized autoregressive conditional heteroskedasticity (MARMA–GARCH) model. Unlike standard ARMA processes, our model admits roots inside
[...] Read more.
Financial assets often exhibit explosive price surges followed by abrupt collapses, alongside persistent volatility clustering. Motivated by these features, we introduce a mixed causal–noncausal invertible–noninvertible autoregressive moving average generalized autoregressive conditional heteroskedasticity (MARMA–GARCH) model. Unlike standard ARMA processes, our model admits roots inside the unit disk, capturing bubble-like episodes and speculative feedback, while the GARCH component explains time-varying volatility. We propose two estimation approaches: (i) Whittle-based frequency-domain methods, which are asymptotically equivalent to Gaussian likelihood under stationarity and finite variance, and (ii) time-domain maximum likelihood, which proves to be more robust to heavy tails and skewness—common in financial returns. To identify causal vs. noncausal structures, we develop a higher-order diagnostics procedure using spectral densities and residual-based tests. Simulation results reveal that overlooking noncausality biases GARCH parameters, downplaying short-run volatility reactions to news ( ) while overstating volatility persistence ( ). Our empirical application to Bitcoin and Ethereum enhances these insights: we find significant noncausal dynamics in the mean, paired with pronounced GARCH effects in the variance. Imposing a purely causal ARMA specification leads to systematically misspecified volatility estimates, potentially underestimating market risks. Our results emphasize the importance of relaxing the usual causality and invertibility assumption for assets prone to extreme price movements, ultimately improving risk metrics and expanding our understanding of financial market dynamics.
Full article
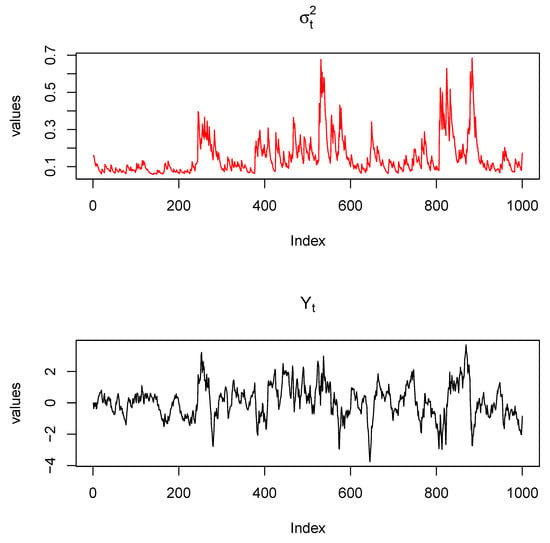
Figure 1
Open AccessArticle
Dynamic Interaction Between Microfinance and Household Well-Being: Evidence from the Microcredit Progressive Model for Sustainable Development
by
Ahmad Alqatan, Najoua Talbi, Hasan Behbehani, Samira Ben Belgacem, Muhammad Arslan and Wafaa Sbeiti
Econometrics 2025, 13(1), 12; https://doi.org/10.3390/econometrics13010012 - 6 Mar 2025
Abstract
Microfinance aims to promote financial inclusion among underprivileged individuals, particularly through progressive microcredit, which enables borrowers to access increasing loan amounts over time. This study examines the conditions under which progressive microcredit positively impacts both small business performance and household well-being, considering borrower
[...] Read more.
Microfinance aims to promote financial inclusion among underprivileged individuals, particularly through progressive microcredit, which enables borrowers to access increasing loan amounts over time. This study examines the conditions under which progressive microcredit positively impacts both small business performance and household well-being, considering borrower characteristics and business activity conditions. Using a dataset of 278 households across 110 administrative sectors in Tunisia from 2012 to 2020, this study employs two-stage least squares (2SLS) and three-stage least squares (3SLS) econometric techniques to estimate simultaneous equation models. The findings reveal that the cumulative amount of progressive microcredit received is mainly determined by project capital, suggesting that businesses with higher capital requirements tend to secure larger loans over successive cycles. Household well-being is significantly influenced by progressive microcredit, household income, net business benefit, rate of development index, and homeownership. Meanwhile, business profitability is driven by project capital and total fixed assets, highlighting the long-term impact of microcredit. The results highlight the critical role of microfinance in enabling small-scale entrepreneurs to expand their businesses while simultaneously improving household financial security. By promoting sustainable income generation, progressive microcredit serves as a key instrument in poverty alleviation and economic stability. This study underscores the necessity for microfinance institutions (MFIs) to tailor their lending strategies, ensuring optimal loan progression that balances business expansion with financial sustainability. Additionally, policymakers should refine microcredit frameworks to enhance accessibility and long-term economic benefits for low-income borrowers. Overall, these insights contribute to the broader discourse on financial inclusion and sustainable development, emphasizing that progressive microcredit not only facilitates entrepreneurship, but also serves as a driver of socioeconomic mobility.
Full article
Open AccessArticle
Real Option Valuation of an Emerging Renewable Technology Design in Wave Energy Conversion
by
James A. DiLellio, John C. Butler, Igor Rizaev, Wanan Sheng and George Aggidis
Econometrics 2025, 13(1), 11; https://doi.org/10.3390/econometrics13010011 - 4 Mar 2025
Abstract
►▼
Show Figures
The untapped potential of wave energy offers another alternative to diversifying renewable energy sources and addressing climate change by reducing CO2 emissions. However, development costs to mature the technology remain significant hurdles to adoption at scale and the technology often must compete
[...] Read more.
The untapped potential of wave energy offers another alternative to diversifying renewable energy sources and addressing climate change by reducing CO2 emissions. However, development costs to mature the technology remain significant hurdles to adoption at scale and the technology often must compete against other marine energy renewables such as offshore wind. Here, we conduct a real option valuation that includes the uncertain market price of wholesale electricity and managerial flexibility expressed in determining future optimal decisions. We demonstrate the probability that the project’s embedded compound real option value can turn a negative net present value wave energy project to a positive expected value. This change in investment decision uses decision tree analysis, where real options are developed as decision nodes, and models the uncertainty as a risk-neutral stochastic process using chance nodes. We also show how our results are analogous to a financial out-of-the-money call option. Our results highlight the distribution of outcomes and the benefit of a staged long-term investment in wave energy systems to better understand and manage project risk, recognizing that these probabilistic results are subject to the ongoing evolution of wholesale electricity prices and the stochastic process models used here to capture their future dynamics. Lastly, we show that the near-term optimal decision is to continue to fund ongoing development of a reference architecture to a higher technology readiness level to maintain the long-term option to deploy such a renewable energy system through private investment or private–public partnerships.
Full article
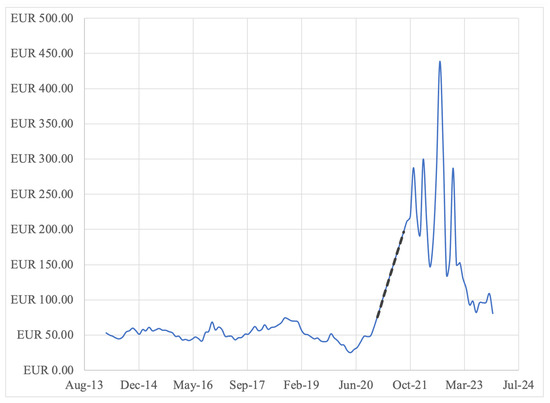
Figure 1
Open AccessArticle
A Study of Economic and Social Preferences in Energy-Saving Behavior Using a Structural Equation Modeling Approach: The Case of Romania
by
Cristian Busu, Mihail Busu, Stelian Grasu, Ilona Skačkauskienė and Luis Miguel Fonseca
Econometrics 2025, 13(1), 10; https://doi.org/10.3390/econometrics13010010 - 24 Feb 2025
Abstract
►▼
Show Figures
Examining the energy consumer behavioral model is critical for national governments and academia. This endeavor seeks to uncover effective solutions amid the energy crisis and climate change challenges. This article delves into legislative developments within the energy sector, European Commission recommendations for reducing
[...] Read more.
Examining the energy consumer behavioral model is critical for national governments and academia. This endeavor seeks to uncover effective solutions amid the energy crisis and climate change challenges. This article delves into legislative developments within the energy sector, European Commission recommendations for reducing energy consumption, and existing constraints impacting individual consumers. By scrutinizing the relevant literature, we aimed to identify and analyze factors that can enhance individual benefits derived from energy savings. Then, a comprehensive set of variables was formulated to model the final consumers’ behavior. Data collection involved administering questionnaires to individual consumers, consumer associations, and energy micro-enterprises in Romania. The gathered data were meticulously analyzed using the Smart-Pls 4 statistical software. Building upon insights from specialized literature, this paper pinpoints the behavioral determinants influencing the reduction in energy consumption. These determinants serve as independent variables shaping the voluntary adoption of measures in lifestyle and behavior among various types of energy users. This study’s findings validate the assumptions presented in this article, highlighting that a reduction in energy consumption is a direct and intrinsic outcome achieved by cumulatively addressing several factors. These factors encompass investments in the energy sector, budget allocation for energy consumption expenditure, adherence to social behavior norms, access to pertinent information about the consequences of the energy crisis, and individual responsibility. Notably, the perception of energy-saving opportunities emerges as a mediator between the independent variables and energy savings with a significant effect. This aspect, developed for the first time in this article, draws inspiration from the prospect theory introduced by Kahneman and Tversky.
Full article
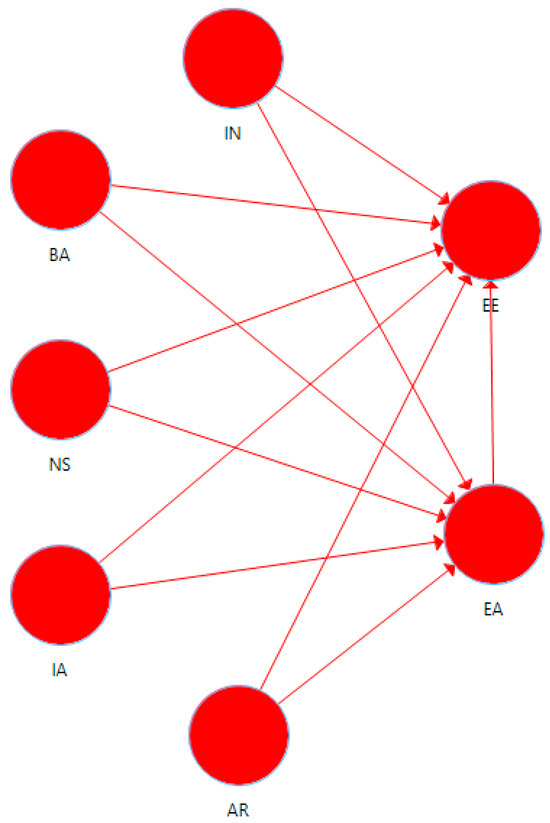
Figure 1
Open AccessArticle
Investigating Some Issues Relating to Regime Matching
by
Anthony D. Hall and Adrian R. Pagan
Econometrics 2025, 13(1), 9; https://doi.org/10.3390/econometrics13010009 - 21 Feb 2025
Abstract
Markov switching models are a common tool used in many disciplines as well as in Economics, and estimation methods are available in many software packages. Estimated models are commonly used for allocating observations to regimes. This allocation is usually done using a rule
[...] Read more.
Markov switching models are a common tool used in many disciplines as well as in Economics, and estimation methods are available in many software packages. Estimated models are commonly used for allocating observations to regimes. This allocation is usually done using a rule based on the estimated smoothed probabilities, such as, in the two regime case, when it exceeds the threshold of 0.5. The accuracy of the regime matching is often measured by the concordance index. Can regime matching be improved by using other rules? By replicating a number of published two-and three- regime studies and the use of simulation methods, it demonstrates that other rules can improve on the performance of the rule based on the threshold of 0.5. Using simulated models we extend the analysis of a single series to investigate, and demonstrate the efficacy of Markov switching models identifying a common factor in multiple time series.
Full article
(This article belongs to the Special Issue Advancements in Macroeconometric Modeling and Time Series Analysis)
►▼
Show Figures
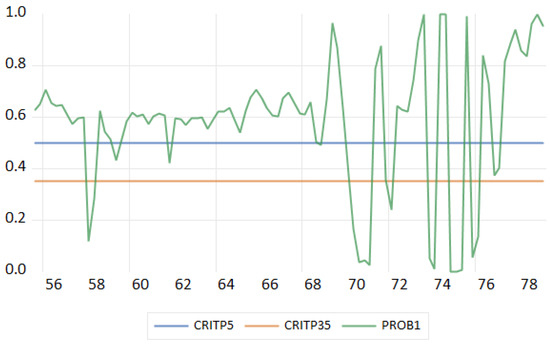
Figure 1
Highly Accessed Articles
Latest Books
E-Mail Alert
News
Topics

Conferences
Special Issues
Special Issue in
Econometrics
Advancements in Macroeconometric Modeling and Time Series Analysis
Guest Editor: Julien ChevallierDeadline: 31 December 2025
Special Issue in
Econometrics
Innovations in Bayesian Econometrics: Theory, Techniques, and Economic Analysis
Guest Editor: Deborah GefangDeadline: 31 May 2026
Special Issue in
Econometrics
Labor Market Dynamics and Wage Inequality: Econometric Models of Income Distribution
Guest Editor: Marc K. ChanDeadline: 25 June 2026