Journal Description
Big Data and Cognitive Computing
Big Data and Cognitive Computing
is an international, peer-reviewed, open access journal on big data and cognitive computing published monthly online by MDPI.
- Open Access— free for readers, with article processing charges (APC) paid by authors or their institutions.
- High Visibility: indexed within Scopus, ESCI (Web of Science), dblp, Inspec, Ei Compendex, and other databases.
- Journal Rank: JCR - Q1 (Computer Science, Theory and Methods) / CiteScore - Q1 (Management Information Systems)
- Rapid Publication: manuscripts are peer-reviewed and a first decision is provided to authors approximately 25.3 days after submission; acceptance to publication is undertaken in 5.6 days (median values for papers published in this journal in the second half of 2024).
- Recognition of Reviewers: reviewers who provide timely, thorough peer-review reports receive vouchers entitling them to a discount on the APC of their next publication in any MDPI journal, in appreciation of the work done.
Impact Factor:
3.7 (2023)
Latest Articles
Tri-Collab: A Machine Learning Project to Leverage Innovation Ecosystems in Portugal
Big Data Cogn. Comput. 2025, 9(5), 139; https://doi.org/10.3390/bdcc9050139 - 20 May 2025
Abstract
►
Show Figures
This project consists of a digital platform named Tri-Collab, where investors, entrepreneurs, and other agents (mainly talents) can cooperate on their ideas and eventually co-create. It is a digital means for this triad of actors (among other potential ones) to better adjust their
[...] Read more.
This project consists of a digital platform named Tri-Collab, where investors, entrepreneurs, and other agents (mainly talents) can cooperate on their ideas and eventually co-create. It is a digital means for this triad of actors (among other potential ones) to better adjust their requirements. It includes an app that easily communicates with a database of projects, innovation agents and their profiles, and the originality lies in the matching algorithm. Thus, co-creation can have better support through this assertive interconnection of players and their resources. This work also highlights the usefulness of the Canvas Business Model in structuring the idea and its dashboard, allowing a comprehensive view of channels, challenges and gains. Also, the potential of machine learning in improving matchmaking platforms is discussed, especially when technological advancements allow for forecasts and match people at scale.
Full article
Open AccessArticle
A Comparative Study of Ensemble Machine Learning and Explainable AI for Predicting Harmful Algal Blooms
by
Omer Mermer, Eddie Zhang and Ibrahim Demir
Big Data Cogn. Comput. 2025, 9(5), 138; https://doi.org/10.3390/bdcc9050138 - 20 May 2025
Abstract
Harmful algal blooms (HABs), driven by environmental pollution, pose significant threats to water quality, public health, and aquatic ecosystems. This study enhances the prediction of HABs in Lake Erie, part of the Great Lakes system, by utilizing ensemble machine learning (ML) models coupled
[...] Read more.
Harmful algal blooms (HABs), driven by environmental pollution, pose significant threats to water quality, public health, and aquatic ecosystems. This study enhances the prediction of HABs in Lake Erie, part of the Great Lakes system, by utilizing ensemble machine learning (ML) models coupled with explainable artificial intelligence (XAI) for interpretability. Using water quality data from 2013 to 2020, various physical, chemical, and biological parameters were analyzed to predict chlorophyll-a (Chl-a) concentrations, which are a commonly used indicator of phytoplankton biomass and a proxy for algal blooms. This study employed multiple ensemble ML models, including random forest (RF), deep forest (DF), gradient boosting (GB), and XGBoost, and compared their performance against individual models, such as support vector machine (SVM), decision tree (DT), and multi-layer perceptron (MLP). The findings revealed that the ensemble models, particularly XGBoost and deep forest (DF), achieved superior predictive accuracy, with R2 values of 0.8517 and 0.8544, respectively. The application of SHapley Additive exPlanations (SHAPs) provided insights into the relative importance of the input features, identifying the particulate organic nitrogen (PON), particulate organic carbon (POC), and total phosphorus (TP) as the critical factors influencing the Chl-a concentrations. This research demonstrates the effectiveness of ensemble ML models for achieving high predictive accuracy, while the integration of XAI enhances model interpretability. The results support the development of proactive water quality management strategies and highlight the potential of advanced ML techniques for environmental monitoring.
Full article
(This article belongs to the Special Issue Machine Learning Applications and Big Data Challenges)
Open AccessArticle
The Development of Small-Scale Language Models for Low-Resource Languages, with a Focus on Kazakh and Direct Preference Optimization
by
Nurgali Kadyrbek, Zhanseit Tuimebayev, Madina Mansurova and Vítor Viegas
Big Data Cogn. Comput. 2025, 9(5), 137; https://doi.org/10.3390/bdcc9050137 - 20 May 2025
Abstract
►▼
Show Figures
Low-resource languages remain underserved by contemporary large language models (LLMs) because they lack sizable corpora, bespoke preprocessing tools, and the computing budgets assumed by mainstream alignment pipelines. Focusing on Kazakh, we present a 1.94B parameter LLaMA-based model that demonstrates how strong, culturally aligned
[...] Read more.
Low-resource languages remain underserved by contemporary large language models (LLMs) because they lack sizable corpora, bespoke preprocessing tools, and the computing budgets assumed by mainstream alignment pipelines. Focusing on Kazakh, we present a 1.94B parameter LLaMA-based model that demonstrates how strong, culturally aligned performance can be achieved without massive infrastructure. The contribution is threefold. (i) Data and tokenization—we compile a rigorously cleaned, mixed-domain Kazakh corpus and design a tokenizer that respects the language’s agglutinative morphology, mixed-script usage, and diacritics. (ii) Training recipe—the model is built in two stages: causal language modeling from scratch followed by instruction tuning. Alignment is further refined with Direct Preference Optimization (DPO), extended by contrastive and entropy-based regularization to stabilize training under sparse, noisy preference signals. Two complementary resources support this step: ChatTune-DPO, a crowd-sourced set of human preference pairs, and Pseudo-DPO, an automatically generated alternative that repurposes instruction data to reduce annotation cost. (iii) Evaluation and impact—qualitative and task-specific assessments show that targeted monolingual training and the proposed DPO variant markedly improve factuality, coherence, and cultural fidelity over baseline instruction-only and multilingual counterparts. The model and datasets are released under open licenses, offering a reproducible blueprint for extending state-of-the-art language modeling to other under-represented languages and domains.
Full article
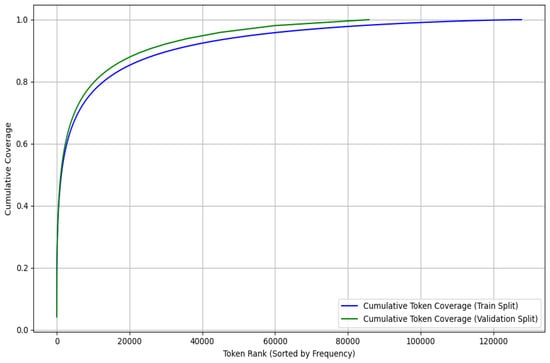
Figure 1
Open AccessArticle
Helium Speech Recognition Method Based on Spectrogram with Deep Learning
by
Yonghong Chen, Shibing Zhang and Dongmei Li
Big Data Cogn. Comput. 2025, 9(5), 136; https://doi.org/10.3390/bdcc9050136 - 20 May 2025
Abstract
►▼
Show Figures
With the development of the marine economy and the increase in marine activities, deep saturation diving has gained significant attention. Helium speech communication is indispensable for saturation diving operations and is a critical technology for deep saturation diving, serving as the sole communication
[...] Read more.
With the development of the marine economy and the increase in marine activities, deep saturation diving has gained significant attention. Helium speech communication is indispensable for saturation diving operations and is a critical technology for deep saturation diving, serving as the sole communication method to ensure the smooth execution of such operations. This study introduces deep learning into helium speech recognition and proposes a spectrogram-based dual-model helium speech recognition method. First, we extract the spectrogram features from the helium speech. Then, we combine a deep fully convolutional neural network with connectionist temporal classification (CTC) to form an acoustic model, in which the spectrogram features of helium speech are used as an input to convert speech signals into phonetic sequences. Finally, a maximum entropy hidden Markov model (MEMM) is employed as the language model to convert the phonetic sequences to word outputs, which is regarded as a dynamic programming problem. We use a Viterbi algorithm to find the optimal path to decode the phonetic sequences to word sequences. The simulation results show that the method can effectively recognize helium speech with a recognition rate of 97.89% for isolated words and 95.99% for continuous helium speech.
Full article
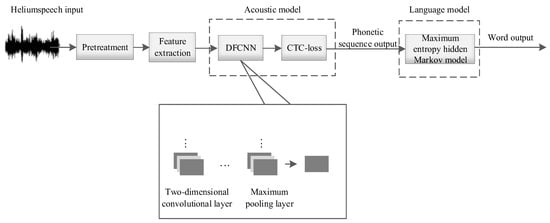
Figure 1
Open AccessArticle
Applying Big Data for Maritime Accident Risk Assessment: Insights, Predictive Insights and Challenges
by
Vicky Zampeta, Gregory Chondrokoukis and Dimosthenis Kyriazis
Big Data Cogn. Comput. 2025, 9(5), 135; https://doi.org/10.3390/bdcc9050135 - 19 May 2025
Abstract
►▼
Show Figures
Maritime safety is a critical concern for the transport sector and remains a key challenge for the international shipping industry. Recognizing that maritime accidents pose significant risks to both safety and operational efficiency, this study explores the application of big data analysis techniques
[...] Read more.
Maritime safety is a critical concern for the transport sector and remains a key challenge for the international shipping industry. Recognizing that maritime accidents pose significant risks to both safety and operational efficiency, this study explores the application of big data analysis techniques to understand the factors influencing maritime transport accidents (MTA). Specifically, using extensive datasets derived from vessel performance measurements, environmental conditions, and accident reports, it seeks to identify the key intrinsic and extrinsic factors contributing to maritime accidents. The research examines more than 90 thousand incidents for the period 2014–2022. Leveraging big data analytics and advanced statistical techniques, the findings reveal significant correlations between vessel size, speed, and specific environmental factors. Furthermore, the study highlights the potential of big data analytics in enhancing predictive modeling, real-time risk assessment, and decision-making processes for maritime traffic management. The integration of big data with intelligent transportation systems (ITSs) can optimize safety strategies, improve accident prevention mechanisms, and enhance the resilience of ocean-going transportation systems. By bridging the gap between big data applications and maritime safety research, this work contributes to the literature by emphasizing the importance of examining both intrinsic and extrinsic factors in predicting maritime accident risks. Additionally, it underscores the transformative role of big data in shaping safer and more efficient waterway transportation systems.
Full article
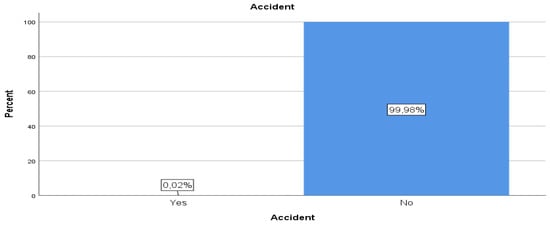
Figure 1
Open AccessArticle
Predicting Early Employability of Vietnamese Graduates: Insights from Data-Driven Analysis Through Machine Learning Methods
by
Long-Sheng Chen, Thao-Trang Huynh-Cam, Van-Canh Nguyen, Tzu-Chuen Lu and Dang-Khoa Le-Huynh
Big Data Cogn. Comput. 2025, 9(5), 134; https://doi.org/10.3390/bdcc9050134 - 19 May 2025
Abstract
►▼
Show Figures
Graduate employability remains a crucial challenge for higher education institutions, especially in developing economies. This study investigates the key academic and vocational factors influencing early employment outcomes among recent graduates at a public university in Vietnam’s Mekong Delta region. By leveraging predictive analytics,
[...] Read more.
Graduate employability remains a crucial challenge for higher education institutions, especially in developing economies. This study investigates the key academic and vocational factors influencing early employment outcomes among recent graduates at a public university in Vietnam’s Mekong Delta region. By leveraging predictive analytics, the research explores how data-driven approaches can enhance career readiness strategies. The analysis employed AI-driven models, particularly classification and regression trees (CARTs), using a dataset of 610 recent graduates from a public university in the Mekong Delta to predict early employability. The input factors included gender, field of study, university entrance scores, and grade point average (GPA) scores for four university years. The output factor was recent graduates’ (un)employment within six months after graduation. Among all input factors, third-year GPA, university entrance scores, and final-year academic performance are the most significant predictors of early employment. Among the tested models, CARTs achieved the highest accuracy (93.6%), offering interpretable decision rules that can inform curriculum design and career support services. This study contributes to the intersection of artificial intelligence and vocational education by providing actionable insights for universities, policymakers, and employers, supporting the alignment of education with labor market demands and improving graduate employability outcomes.
Full article
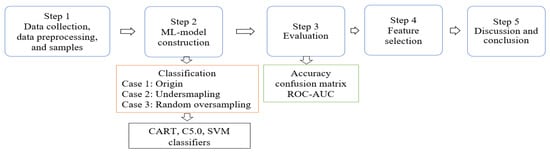
Figure 1
Open AccessArticle
Adaptive Augmented Reality Architecture for Optimising Assistance and Safety in Industry 4.0
by
Ginés Morales Méndez and Francisco del Cerro Velázquez
Big Data Cogn. Comput. 2025, 9(5), 133; https://doi.org/10.3390/bdcc9050133 - 19 May 2025
Abstract
►▼
Show Figures
The present study proposes adaptive augmented reality (AR) architecture, specifically designed to enhance real-time operator assistance and occupational safety in industrial environments, which is representative of Industry 4.0. The proposed system addresses key challenges in AR adoption, such as the need for dynamic
[...] Read more.
The present study proposes adaptive augmented reality (AR) architecture, specifically designed to enhance real-time operator assistance and occupational safety in industrial environments, which is representative of Industry 4.0. The proposed system addresses key challenges in AR adoption, such as the need for dynamic personalisation of instructions based on operator profiles and the mitigation of technical and cognitive barriers. Architecture integrates theoretical modelling, modular design, and real-time adaptability to match instruction complexity with user expertise and environmental conditions. A working prototype was implemented using Microsoft HoloLens 2, Unity 3D, and Vuforia and validated in a controlled industrial scenario involving predictive maintenance and assembly tasks. The experimental results demonstrated statistically significant enhancements in task completion time, error rates, perceived cognitive load, operational efficiency, and safety indicators in comparison with conventional methods. The findings underscore the system’s capacity to enhance both performance and consistency while concomitantly bolstering risk mitigation in intricate operational settings. This study proposes a scalable and modular AR framework with built-in safety and adaptability mechanisms, demonstrating practical benefits for human–machine interaction in Industry 4.0. The present study is subject to certain limitations, including validation in a simulated environment, which limits the direct extrapolation of the results to real industrial scenarios; further evaluation in various operational contexts is required to verify the overall scalability and applicability of the proposed system. It is recommended that future research studies explore the long-term ergonomics, scalability, and integration of emerging technologies in decision support within adaptive AR systems.
Full article
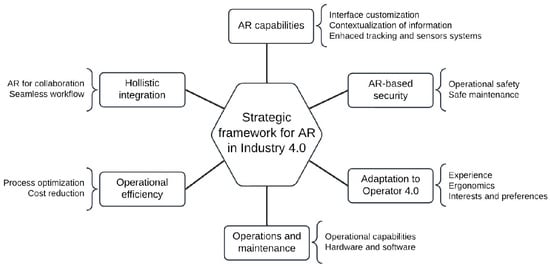
Figure 1
Open AccessArticle
Comparative Evaluation of Multimodal Large Language Models for No-Reference Image Quality Assessment with Authentic Distortions: A Study of OpenAI and Claude.AI Models
by
Domonkos Varga
Big Data Cogn. Comput. 2025, 9(5), 132; https://doi.org/10.3390/bdcc9050132 - 16 May 2025
Abstract
This study presents a comparative analysis of several multimodal large language models (LLMs) for no-reference image quality assessment, with a particular focus on images containing authentic distortions. We evaluate three models developed by OpenAI and three models from Claude.AI, comparing their performance in
[...] Read more.
This study presents a comparative analysis of several multimodal large language models (LLMs) for no-reference image quality assessment, with a particular focus on images containing authentic distortions. We evaluate three models developed by OpenAI and three models from Claude.AI, comparing their performance in estimating image quality without reference images. Our results demonstrate that these LLMs outperform traditional methods based on hand-crafted features. However, more advanced deep learning models, especially those based on deep convolutional networks, surpass LLMs in performance. Notably, we make a unique contribution by publishing the processed outputs of the LLMs, providing a transparent and direct comparison of their quality assessments based solely on the predicted quality scores. This work underscores the potential of multimodal LLMs in image quality evaluation, while also highlighting the continuing advantages of specialized deep learning approaches.
Full article
(This article belongs to the Special Issue Advances in Natural Language Processing and Text Mining)
►▼
Show Figures
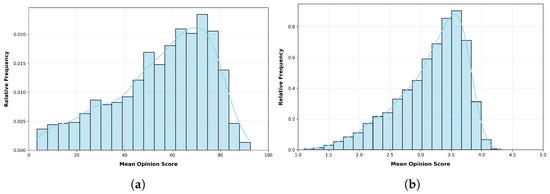
Figure 1
Open AccessArticle
Evaluating the Impact of Artificial Intelligence Tools on Enhancing Student Academic Performance: Efficacy Amidst Security and Privacy Concerns
by
Jwern Tick Kiet Phua, Han-Foon Neo and Chuan-Chin Teo
Big Data Cogn. Comput. 2025, 9(5), 131; https://doi.org/10.3390/bdcc9050131 - 15 May 2025
Abstract
The rapid advancements in artificial intelligence (AI) have significantly transformed various domains, including education, by introducing innovative tools that reshape teaching and learning processes. This research investigates the perceptions and attitudes of students towards the use of AI tools in their academic activities,
[...] Read more.
The rapid advancements in artificial intelligence (AI) have significantly transformed various domains, including education, by introducing innovative tools that reshape teaching and learning processes. This research investigates the perceptions and attitudes of students towards the use of AI tools in their academic activities, focusing on constructs such as perceived usefulness, the perceived ease of use, security and privacy concerns, and both positive and negative attitudes towards AI. On the basis of Technology Acceptance Model (TAM) and the General Attitudes towards Artificial Intelligence Scale (GAAIS), this research seeks to identify the factors influencing students’ behavioral intentions and actual adoption of AI tools in educational settings. A structured survey was administered to students at Multimedia University, Malaysia, capturing their experiences and opinions on widely used AI tools such as ChatGPT, Quillbot, Grammarly, and Perplexity. Hypothesis testing was used to evaluate the statistical significance of relationships between the constructs and behavioral intention and actual use of the AI tools. The findings reveal a high level of engagement with AI tools among University students, primarily driven by their perceived benefits in enhancing academic performance, improving efficiency, and facilitating personalized learning experiences. The findings also uncover significant concerns related to data security, privacy, and the potential over-reliance on AI tools, which may hinder the development of critical thinking and problem-solving skills.
Full article
(This article belongs to the Special Issue Security, Privacy, and Trust in Artificial Intelligence Applications)
►▼
Show Figures
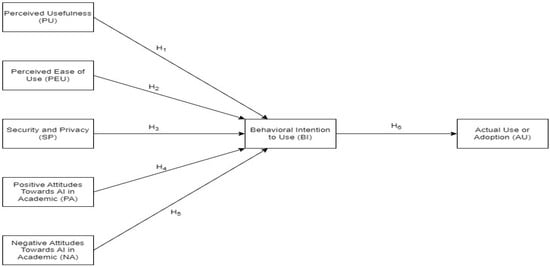
Figure 1
Open AccessArticle
Machine Learning-Based Classification of Sulfide Mineral Spectral Emission in High Temperature Processes
by
Carlos Toro, Walter Díaz, Gonzalo Reyes, Miguel Peña, Nicolás Caselli, Carla Taramasco, Pablo Ormeño-Arriagada and Eduardo Balladares
Big Data Cogn. Comput. 2025, 9(5), 130; https://doi.org/10.3390/bdcc9050130 - 14 May 2025
Abstract
Accurate classification of sulfide minerals during combustion is essential for optimizing pyrometallurgical processes such as flash smelting, where efficient combustion impacts resource utilization, energy efficiency, and emission control. This study presents a deep learning-based approach for classifying visible and near-infrared (VIS-NIR) emission spectra
[...] Read more.
Accurate classification of sulfide minerals during combustion is essential for optimizing pyrometallurgical processes such as flash smelting, where efficient combustion impacts resource utilization, energy efficiency, and emission control. This study presents a deep learning-based approach for classifying visible and near-infrared (VIS-NIR) emission spectra from the combustion of high-grade sulfide minerals. A one-dimensional convolutional neural network (1D-CNN) was developed and trained on experimentally acquired spectral data, achieving a balanced accuracy score of 99.0% in a test set. The optimized deep learning model outperformed conventional machine learning methods, highlighting the effectiveness of deep learning for spectral analysis in high-temperature environments. In addition, Gradient-weighted Class Activation Mapping (Grad-CAM) was applied to enhance model interpretability and identify key spectral regions contributing to classification decisions. The results demonstrated that the model successfully distinguished spectral features associated with different mineral species, offering insights into combustion dynamics. These findings support the potential integration of deep learning for real-time spectral monitoring in industrial flash smelting operations, thereby enabling more precise process control and decision-making.
Full article
(This article belongs to the Special Issue Machine Learning and AI Technology for Sustainable Development)
►▼
Show Figures
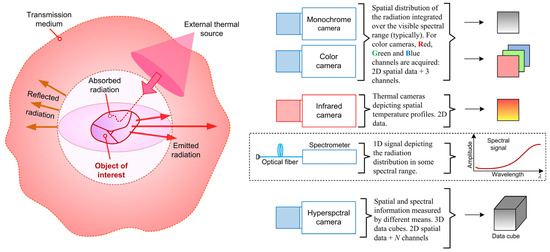
Figure 1
Open AccessArticle
Identifying Influential Nodes in Complex Networks via Transformer with Multi-Scale Feature Fusion
by
Tingshuai Jiang, Yirun Ruan, Tianyuan Yu, Liang Bai and Yifei Yuan
Big Data Cogn. Comput. 2025, 9(5), 129; https://doi.org/10.3390/bdcc9050129 - 14 May 2025
Abstract
In complex networks, the identification of critical nodes is vital for optimizing information dissemination. Given the significant role of these nodes in network structures, researchers have proposed various identification methods. In recent years, deep learning has emerged as a promising approach for identifying
[...] Read more.
In complex networks, the identification of critical nodes is vital for optimizing information dissemination. Given the significant role of these nodes in network structures, researchers have proposed various identification methods. In recent years, deep learning has emerged as a promising approach for identifying key nodes in networks. However, existing algorithms fail to effectively integrate local and global structural information, leading to incomplete and limited network understanding. To overcome this limitation, we introduce a transformer framework with multi-scale feature fusion (MSF-Former). In this framework, we construct local and global feature maps for nodes and use them as input. Through the transformer module, node information is effectively aggregated, thereby improving the model’s ability to recognize key nodes. We perform evaluations using six real-world and three synthetic network datasets, comparing our method against multiple baselines using the SIR model to validate its effectiveness. Experimental analysis confirms that MSF-Former achieves consistently high accuracy in the identification of influential nodes across real-world and synthetic networks.
Full article
(This article belongs to the Special Issue Advances in Complex Networks)
►▼
Show Figures
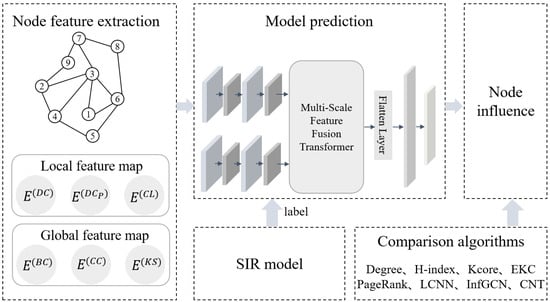
Figure 1
Open AccessReview
Benchmarking of Anomaly Detection Methods for Industry 4.0: Evaluation, Ranking, and Practical Recommendations
by
Aurélie Cools, Mohammed Amin Belarbi and Sidi Ahmed Mahmoudi
Big Data Cogn. Comput. 2025, 9(5), 128; https://doi.org/10.3390/bdcc9050128 - 13 May 2025
Abstract
Quality control and predictive maintenance are two essential pillars of Industry 4.0, aiming to optimize production, reduce operational costs, and enhance system reliability. Real-time visual inspection ensures early detection of manufacturing defects, assembly errors, or texture inconsistencies, preventing defective products from reaching customers.
[...] Read more.
Quality control and predictive maintenance are two essential pillars of Industry 4.0, aiming to optimize production, reduce operational costs, and enhance system reliability. Real-time visual inspection ensures early detection of manufacturing defects, assembly errors, or texture inconsistencies, preventing defective products from reaching customers. Predictive maintenance leverages sensor data by analyzing vibrations, temperature, and pressure signals to anticipate failures and avoid production downtime. Image-based quality control has become critical in industries such as automotive, electronics, aerospace, and food processing, where visual appearance is a key quality indicator. Although advances in deep learning and computer vision have significantly improved anomaly detection, industrial deployments remain challenged by the scarcity of labeled anomalies and the variability of defects. These issues increasingly lead to the adoption of unsupervised methods and generative approaches, which, despite their effectiveness, introduce substantial computational complexity. We conduct a unified comparison of ten anomaly detection methods, categorizing them according to their reliance on synthetic anomaly generation and their detection strategy, either reconstruction-based or feature-based. All models are trained exclusively on normal data to mirror realistic industrial conditions. Our evaluation framework combines performance metrics such as recall, precision, and their harmonic mean, emphasizing the need to minimize false negatives that could lead to critical production failures. In addition, we assess environmental impact and hardware complexity to better guide method selection. Practical recommendations are provided to balance robustness, operational feasibility, and sustainability in industrial applications.
Full article
(This article belongs to the Special Issue Fault Diagnosis and Detection Based on Deep Learning)
►▼
Show Figures
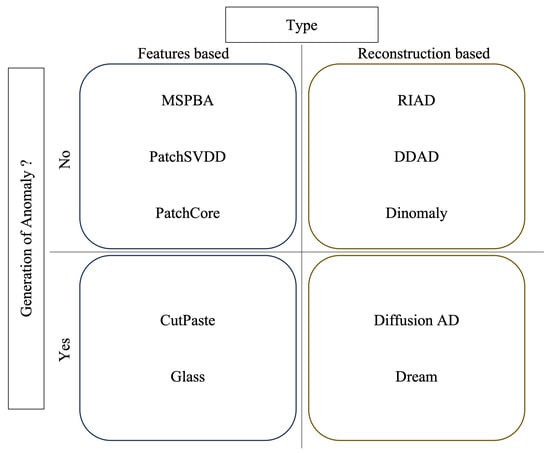
Figure 1
Open AccessArticle
Rail Surface Defect Diagnosis Based on Image–Vibration Multimodal Data Fusion
by
Zhongmei Wang, Shenao Peng, Wenxiu Ao, Jianhua Liu and Changfan Zhang
Big Data Cogn. Comput. 2025, 9(5), 127; https://doi.org/10.3390/bdcc9050127 - 12 May 2025
Abstract
►▼
Show Figures
To address the challenges in existing multi-sensor data fusion methods for rail surface defect diagnosis, particularly their limitations in fully exploiting potential synergistic information among multimodal data and effectively bridging the semantic gap between heterogeneous multi-source data, this paper proposes a diagnostic approach
[...] Read more.
To address the challenges in existing multi-sensor data fusion methods for rail surface defect diagnosis, particularly their limitations in fully exploiting potential synergistic information among multimodal data and effectively bridging the semantic gap between heterogeneous multi-source data, this paper proposes a diagnostic approach based on a Progressive Joint Representation Graph Attention Fusion Network (PJR-GAFN). The methodology comprises five principal phases: Firstly, shared and specific autoencoders are used to extract joint representations of multimodal features through shared and modality-specific representations. Secondly, a squeeze-and-excitation module is implemented to amplify defect-related features while suppressing non-essential characteristics. Thirdly, a progressive fusion module is introduced to comprehensively utilize cross-modal synergistic information during feature extraction. Fourthly, a source domain classifier and domain discriminator are employed to capture modality-invariant features across different modalities. Finally, the spatial attention aggregation properties of graph attention networks are leveraged to fuse multimodal features, thereby fully exploiting contextual semantic information. Experimental results on real-world rail surface defect datasets from domestic railway lines demonstrate that the proposed method achieves 95% diagnostic accuracy, confirming its effectiveness in rail surface defect detection.
Full article
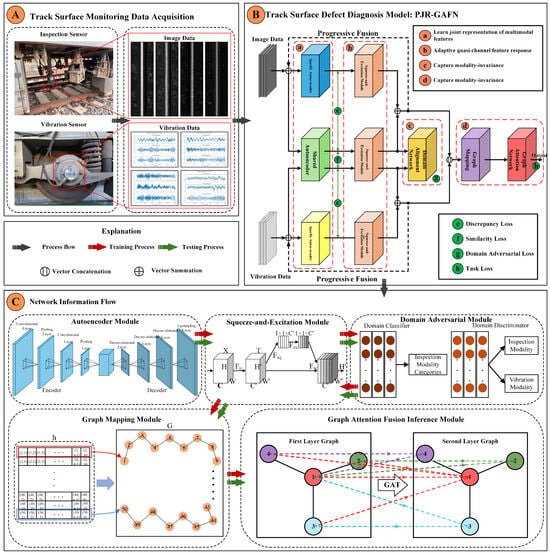
Figure 1
Open AccessArticle
Introducing a Novel Fast Neighbourhood Component Analysis–Deep Neural Network Model for Enhanced Driver Drowsiness Detection
by
Sama Hussein Al-Gburi, Kanar Alaa Al-Sammak, Ion Marghescu, Claudia Cristina Oprea, Ana-Maria Claudia Drăgulinescu, George Suciu, Khattab M. Ali Alheeti, Nayef A. M. Alduais and Nawar Alaa Hussein Al-Sammak
Big Data Cogn. Comput. 2025, 9(5), 126; https://doi.org/10.3390/bdcc9050126 - 8 May 2025
Abstract
►▼
Show Figures
Driver fatigue is a key factor in road accidents worldwide, requiring effective real-time detection mechanisms. Traditional deep neural network (DNN)-based solutions have shown promising results in detecting drowsiness; however, they are often less suitable for real-time applications due to their high computational complexity,
[...] Read more.
Driver fatigue is a key factor in road accidents worldwide, requiring effective real-time detection mechanisms. Traditional deep neural network (DNN)-based solutions have shown promising results in detecting drowsiness; however, they are often less suitable for real-time applications due to their high computational complexity, risk of overfitting, and reliance on large datasets. Hence, this paper introduces an innovative approach that integrates fast neighbourhood component analysis (FNCA) with a deep neural network (DNN) to enhance the detection of driver drowsiness using electroencephalogram (EEG) data. FNCA is employed to optimize feature representation, effectively highlighting critical features for drowsiness detection, which are then analysed using a DNN to achieve high accuracy in recognizing signs of driver fatigue. Our model has been evaluated on the SEED-VIG dataset and achieves state-of-the-art accuracy: 94.29% when trained on 12 subjects and 90.386% with 21 subjects, surpassing existing methods such as TSception, ConvNeXt LMDA-Net, and CNN + LSTM.
Full article
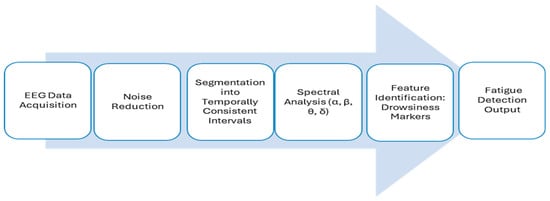
Figure 1
Open AccessArticle
Quantifying Post-Purchase Service Satisfaction: A Topic–Emotion Fusion Approach with Smartphone Data
by
Peijun Guo, Huan Li and Xinyue Mo
Big Data Cogn. Comput. 2025, 9(5), 125; https://doi.org/10.3390/bdcc9050125 - 8 May 2025
Abstract
►▼
Show Figures
Effectively identifying factors related to user satisfaction is crucial for evaluating customer experience. This study proposes a two-phase analytical framework that combines natural language processing techniques with hierarchical decision-making methods. In Phase 1, an ERNIE-LSTM-based emotion model (ELEM) is used to detect fake
[...] Read more.
Effectively identifying factors related to user satisfaction is crucial for evaluating customer experience. This study proposes a two-phase analytical framework that combines natural language processing techniques with hierarchical decision-making methods. In Phase 1, an ERNIE-LSTM-based emotion model (ELEM) is used to detect fake reviews from 4016 smartphone evaluations collected from JD.com (accuracy: 84.77%, recall: 84.86%, F1 score: 84.81%). The filtered genuine reviews are then analyzed using Biterm Topic Modeling (BTM) to extract key satisfaction-related topics, which are weighted based on sentiment scores and organized into a multi-criteria evaluation matrix through the Analytic Hierarchy Process (AHP). These topics are further clustered into five major factors: user-centered design (70.8%), core performance (10.0%), imaging features (8.6%), promotional incentives (7.8%), and industrial design (2.8%). This framework is applied to a comparative analysis of two smartphone stores, revealing that Huawei Mate 60 Pro emphasizes performance, while Redmi Note 11 5G focuses on imaging capabilities. Further clustering of user reviews identifies six distinct user groups, all prioritizing user-centered design and core performance, but showing differences in other preferences. In Phase 2, a comparison of word frequencies between product reviews and community Q and A content highlights hidden user concerns often missed by traditional single-source sentiment analysis, such as screen calibration and pixel density. These findings provide insights into how product design influences satisfaction and offer practical guidance for improving product development and marketing strategies.
Full article
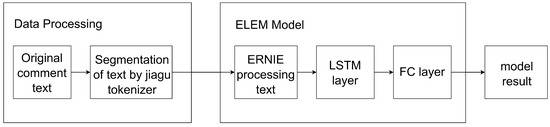
Figure 1
Open AccessArticle
Enhancing Recommendation Systems with Real-Time Adaptive Learning and Multi-Domain Knowledge Graphs
by
Zeinab Shahbazi, Rezvan Jalali and Zahra Shahbazi
Big Data Cogn. Comput. 2025, 9(5), 124; https://doi.org/10.3390/bdcc9050124 - 8 May 2025
Abstract
In the era of information explosion, recommendation systems play a crucial role in filtering vast amounts of content for users. Traditional recommendation models leverage knowledge graphs, sentiment analysis, social capital, and generative AI to enhance personalization. However, existing models still struggle to adapt
[...] Read more.
In the era of information explosion, recommendation systems play a crucial role in filtering vast amounts of content for users. Traditional recommendation models leverage knowledge graphs, sentiment analysis, social capital, and generative AI to enhance personalization. However, existing models still struggle to adapt dynamically to users’ evolving interests across multiple content domains in real-time. To address this gap, the cross-domain adaptive recommendation system (CDARS) is proposed, which integrates real-time behavioral tracking with multi-domain knowledge graphs to refine user preference modeling continuously. Unlike conventional methods that rely on static or historical data, CDARS dynamically adjusts its recommendation strategies based on contextual factors such as real-time engagement, sentiment fluctuations, and implicit preference drifts. Furthermore, a novel explainable adaptive learning (EAL) module was introduced, providing transparent insights into recommendations’ evolving nature, thereby improving user trust and system interpretability. To enable such real-time adaptability, CDARS incorporates multimodal sentiment analysis of user-generated content, behavioral pattern mining (e.g., click timing, revisit frequency), and learning trajectory modeling through time-aware embeddings and incremental updates of user representations. These dynamic signals are mapped into evolving knowledge graphs, forming continuously updated learning charts that drive more context-aware and emotionally intelligent recommendations. Our experimental results on datasets spanning social media, e-commerce, and entertainment domains demonstrate that CDARS significantly enhances recommendation relevance, achieving an average improvement of 7.8% in click-through rate (CTR) and 8.3% in user engagement compared to state-of-the-art models. This research presents a paradigm shift toward truly dynamic and explainable recommendation systems, creating a way for more personalized and user-centric experiences in the digital landscape.
Full article
(This article belongs to the Special Issue Knowledge Graphs in the Big Data Era: Navigating the Confluence of Distribution, Visualization, and Advanced Computational Models)
►▼
Show Figures
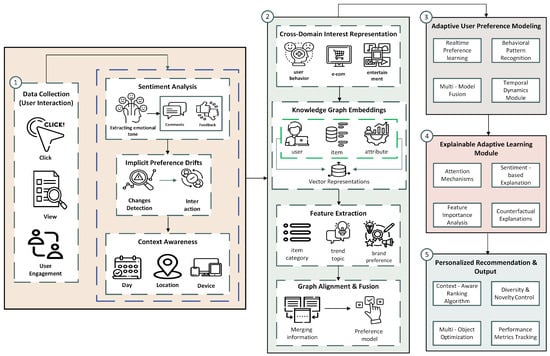
Figure 1
Open AccessArticle
Assessing the Transformation of Armed Conflict Types: A Dynamic Approach
by
Dong Jiang, Jun Zhuo, Peiwei Fan, Fangyu Ding, Mengmeng Hao, Shuai Chen, Jiping Dong and Jiajie Wu
Big Data Cogn. Comput. 2025, 9(5), 123; https://doi.org/10.3390/bdcc9050123 - 8 May 2025
Abstract
►▼
Show Figures
Armed conflict is a dynamic social phenomenon, yet existing research often overlooks its evolving nature. We propose a method to simulate the dynamic transformations of armed conflicts. First, we enhanced the Spatial Conflict Dynamic Indicator (SCDi) by integrating conflict intensity and clustering, which
[...] Read more.
Armed conflict is a dynamic social phenomenon, yet existing research often overlooks its evolving nature. We propose a method to simulate the dynamic transformations of armed conflicts. First, we enhanced the Spatial Conflict Dynamic Indicator (SCDi) by integrating conflict intensity and clustering, which allowed for the distinction of various conflict types. Second, we established transformation rules for the SCDi, quantifying five types of transformations: outbreak, stabilization, escalation, de-escalation, and maintaining peace. Using the random forest algorithm with multiple covariates, we simulated these transformations and analyzed the driving factors. The results reveal a global trend of polarization in armed conflicts over the past 20 years, with an increase in clustered/high-intensity (CH) and dispersed/low-intensity (DL) conflicts. Stable regions of ongoing conflict have emerged, notably in areas like Syria, the border of Afghanistan, and Nepal’s border region. New conflicts are more likely to arise near these zones. Various driving forces shape conflict transformations, with neighboring conflict scenarios acting as key catalysts. The capacity of a region to maintain peace largely depends on neighboring conflict dynamics, while local factors are more influential in other types of transformations. This study quantifies the dynamic process of conflict transformations and reveals detailed changes.
Full article
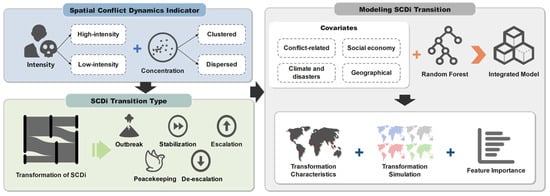
Figure 1
Open AccessArticle
Robust Anomaly Detection of Multivariate Time Series Data via Adversarial Graph Attention BiGRU
by
Yajing Xing, Jinbiao Tan, Rui Zhang and Jiafu Wan
Big Data Cogn. Comput. 2025, 9(5), 122; https://doi.org/10.3390/bdcc9050122 - 8 May 2025
Abstract
►▼
Show Figures
Multivariate time series data (MTSD) anomaly detection due to complex spatio-temporal dependencies among sensors and pervasive environmental noise. The existing methods struggle to balance anomaly detection accuracy with robustness against data contamination. Hence, this paper proposes a robust multivariate temporal data anomaly detection
[...] Read more.
Multivariate time series data (MTSD) anomaly detection due to complex spatio-temporal dependencies among sensors and pervasive environmental noise. The existing methods struggle to balance anomaly detection accuracy with robustness against data contamination. Hence, this paper proposes a robust multivariate temporal data anomaly detection method based on graph attention for training convolutional neural networks (PGAT-BiGRU-NRA). Firstly, the parallel graph attention (PGAT) mechanism extracts the time-dependent and spatially related features of MTSD to realize the MTSD fusion. Then, a bidirectional gate recurrent unit (BiGRU) is utilized to extract the contextual information of the data to avoid information loss. In addition, reconstructing the noise for adversarial training aims to achieve a more robust anomaly detection of MTSD. The experiments conducted on real industrial equipment datasets evaluate the effectiveness of the method in the task of MTSD, and the comparative experiments verify that the proposed method outperforms the mainstream baseline model. The proposed method achieves anomaly detection and robust performance in noise interference, which provides feasible technical support for the stable operation of industrial equipment in complex environments.
Full article
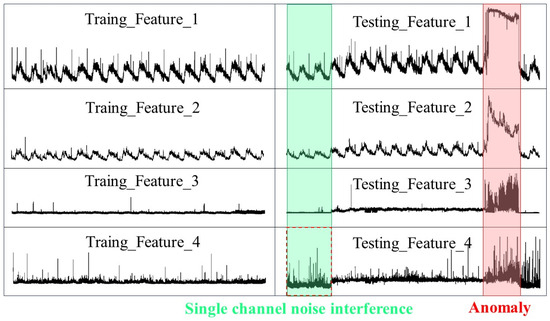
Figure 1
Open AccessArticle
Edge vs. Cloud: Empirical Insights into Data-Driven Condition Monitoring
by
Chikumbutso Christopher Walani and Wesley Doorsamy
Big Data Cogn. Comput. 2025, 9(5), 121; https://doi.org/10.3390/bdcc9050121 - 8 May 2025
Abstract
This study evaluates edge and cloud computing paradigms in the context of data-driven condition monitoring of rotating electrical machines. Two well-known platforms, the Raspberry Pi and Amazon Web Services Elastic Compute Cloud, are used to compare and contrast these two computing paradigms in
[...] Read more.
This study evaluates edge and cloud computing paradigms in the context of data-driven condition monitoring of rotating electrical machines. Two well-known platforms, the Raspberry Pi and Amazon Web Services Elastic Compute Cloud, are used to compare and contrast these two computing paradigms in terms of different metrics associated with their application suitability. The tested induction machine fault diagnosis models are developed using popular algorithms, namely support vector machines, k-nearest neighbours, and decision trees. The findings reveal that while the cloud platform offers superior computational and memory resources, making it more suitable for complex machine learning tasks, it also incurs higher costs and latency. On the other hand, the edge platform excels in real-time processing and reduces network data burden, but its computational and memory resources are found to be a limitation with certain tasks. The study provides both quantitative and qualitative insights into the trade-offs involved in selecting the most suitable computing approach for condition monitoring applications. Although the scope of the empirical study is primarily limited to factors such as computational efficiency, scalability, and resource utilisation, particularly in the context of specific machine learning models, this paper offers broader discussion and future research directions of other key issues, including latency, network variability, and energy consumption.
Full article
(This article belongs to the Special Issue Application of Cloud Computing in Industrial Internet of Things)
►▼
Show Figures
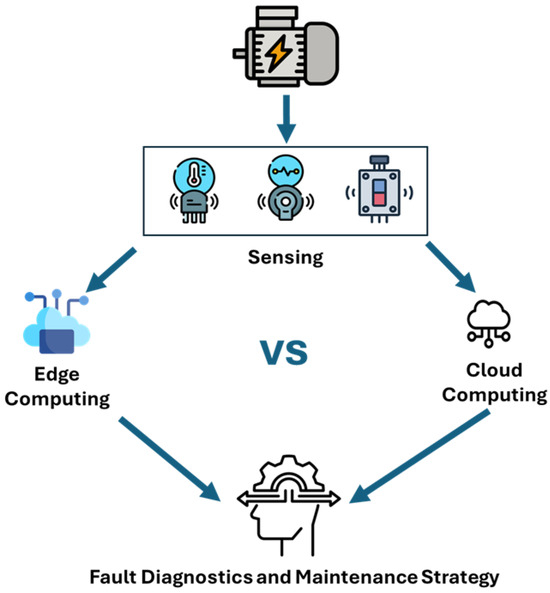
Figure 1
Open AccessArticle
A Computational–Cognitive Model of Audio-Visual Attention in Dynamic Environments
by
Hamideh Yazdani, Alireza Bosaghzadeh, Reza Ebrahimpour and Fadi Dornaika
Big Data Cogn. Comput. 2025, 9(5), 120; https://doi.org/10.3390/bdcc9050120 - 6 May 2025
Abstract
►▼
Show Figures
Human visual attention is influenced by multiple factors, including visual, auditory, and facial cues. While integrating auditory and visual information enhances prediction accuracy, many existing models rely solely on visual-temporal data. Inspired by cognitive studies, we propose a computational model that combines spatial,
[...] Read more.
Human visual attention is influenced by multiple factors, including visual, auditory, and facial cues. While integrating auditory and visual information enhances prediction accuracy, many existing models rely solely on visual-temporal data. Inspired by cognitive studies, we propose a computational model that combines spatial, temporal, face (low-level and high-level visual cues), and auditory saliency to predict visual attention more effectively. Our approach processes video frames to generate spatial, temporal, and face saliency maps, while an audio branch localizes sound-producing objects. These maps are then integrated to form the final audio-visual saliency map. Experimental results on the audio-visual dataset demonstrate that our model outperforms state-of-the-art image and video saliency models and the basic model and aligns more closely with behavioral and eye-tracking data. Additionally, ablation studies highlight the contribution of each information source to the final prediction.
Full article
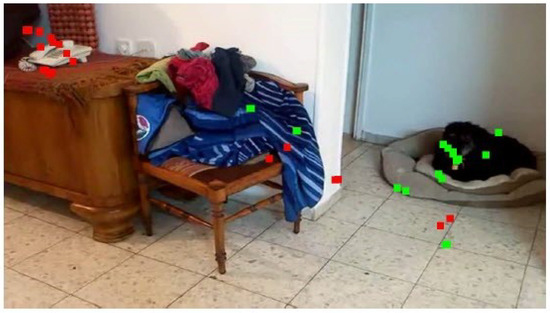
Figure 1
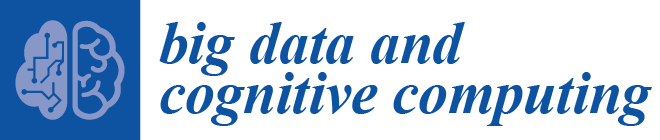
Journal Menu
► ▼ Journal Menu-
- BDCC Home
- Aims & Scope
- Editorial Board
- Reviewer Board
- Topical Advisory Panel
- Instructions for Authors
- Special Issues
- Topics
- Topical Collections
- Article Processing Charge
- Indexing & Archiving
- Editor’s Choice Articles
- Most Cited & Viewed
- Journal Statistics
- Journal History
- Journal Awards
- Editorial Office
Journal Browser
► ▼ Journal BrowserHighly Accessed Articles
Latest Books
E-Mail Alert
News
Topics
Topic in
Applied Sciences, BDCC, Future Internet, Information, Sci
Social Computing and Social Network Analysis
Topic Editors: Carson K. Leung, Fei Hao, Giancarlo Fortino, Xiaokang ZhouDeadline: 30 June 2025
Topic in
AI, BDCC, Fire, GeoHazards, Remote Sensing
AI for Natural Disasters Detection, Prediction and Modeling
Topic Editors: Moulay A. Akhloufi, Mozhdeh ShahbaziDeadline: 25 July 2025
Topic in
Algorithms, BDCC, BioMedInformatics, Information, Mathematics
Machine Learning Empowered Drug Screen
Topic Editors: Teng Zhou, Jiaqi Wang, Youyi SongDeadline: 31 August 2025
Topic in
IJERPH, JPM, Healthcare, BDCC, Applied Sciences, Sensors
eHealth and mHealth: Challenges and Prospects, 2nd EditionTopic Editors: Antonis Billis, Manuel Dominguez-Morales, Anton CivitDeadline: 31 October 2025

Conferences
Special Issues
Special Issue in
BDCC
Advances in Intelligent Defense Systems for the Internet of Things
Guest Editors: Qasem Abu Al-Haija, Ammar Odeh, Abdulaziz Alsulami, Nik BessisDeadline: 31 May 2025
Special Issue in
BDCC
Application of Semantic Technologies in Intelligent Environment
Guest Editors: Maria Nisheva-Pavlova, Galia Angelova, Moulay A. AkhloufiDeadline: 31 May 2025
Special Issue in
BDCC
Artificial Intelligence in Sustainable Reconfigurable Manufacturing Systems and Operations Management
Guest Editors: Hamed Gholami, Jose Arturo Garza-ReyesDeadline: 31 May 2025
Special Issue in
BDCC
Transforming Cyber Security Provision through Utilizing Artificial Intelligence
Guest Editors: Peter R. J. Trim, Yang-Im LeeDeadline: 25 June 2025