Topic Editors


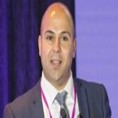
Recent Advances in Security, Privacy, and Trust
Topic Information
Dear Colleagues,
The proliferation of information, communication, and computer technologies has brought us into the realm of the cyber–physical–social system (CPSS). The CPSS comprises the cyber space, physical space and social space, and their integration into such systems as the cyber–physical system (CPS), Internet of Things (IoT), social computing system, and even the system integrating all three spaces. Recently, the CPSS has brought enormous opportunities that have significantly influenced applications. However, there are increasing security, privacy, and trust concerns, such as the exposure of user privacy and business information in the CPSS. Although theories and technologies regarding security, privacy, and trust have been widely studied and applied in recent years, existing methods are still insecure, impractical or inefficient. To address these challenges, this topic solicits articles reflecting the latest research outcomes and developments in security, privacy, and trust.
The topics of interest include, but not limited to, the following:
- Privacy-enhancing technologies
- Privacy-preserving/secure/trust data analysis and processing
- Network security, privacy, and trust
- Differentially private data analysis
- Sustainable security, privacy, and trust
- Economics of security, privacy, and trust
- Blockchain and its applications
- IoT/CPS/CPSS security, privacy, and trust
- Security, privacy, and trust in edge/fog/cloud computing
- AI/Machine learning security
- Federated learning
- System security
- Hardware security
- Web security, privacy, and trust
- Big data, artificial intelligence for security, privacy, and trust
- Digital twin security, privacy, and trust
- Cryptographic techniques, cryptographic protocols
Dr. Jun Feng
Dr. Changqing Luo
Prof. Dr. Mamoun Alazab
Topic Editors
Keywords
- security
- privacy
- trust
- cyberspace security
- cryptography
Participating Journals
Journal Name | Impact Factor | CiteScore | Launched Year | First Decision (median) | APC | |
---|---|---|---|---|---|---|
![]()
Electronics
|
2.6 | 5.3 | 2012 | 16.8 Days | CHF 2400 | Submit |
![]()
Journal of Cybersecurity and Privacy
|
- | 5.3 | 2021 | 32.4 Days | CHF 1000 | Submit |
![]()
Mathematics
|
2.3 | 4.0 | 2013 | 17.1 Days | CHF 2600 | Submit |
![]()
Sustainability
|
3.3 | 6.8 | 2009 | 20 Days | CHF 2400 | Submit |
![]()
Remote Sensing
|
4.2 | 8.3 | 2009 | 24.7 Days | CHF 2700 | Submit |
![]()
ISPRS International Journal of Geo-Information
|
2.8 | 6.9 | 2012 | 36.2 Days | CHF 1700 | Submit |
![]()
Cryptography
|
1.8 | 3.8 | 2017 | 23.9 Days | CHF 1600 | Submit |
![]()
Blockchains
|
- | - | 2023 | 15.0 days * | CHF 1000 | Submit |
* Median value for all MDPI journals in the first half of 2024.
MDPI Topics is cooperating with Preprints.org and has built a direct connection between MDPI journals and Preprints.org. Authors are encouraged to enjoy the benefits by posting a preprint at Preprints.org prior to publication:
- Immediately share your ideas ahead of publication and establish your research priority;
- Protect your idea from being stolen with this time-stamped preprint article;
- Enhance the exposure and impact of your research;
- Receive feedback from your peers in advance;
- Have it indexed in Web of Science (Preprint Citation Index), Google Scholar, Crossref, SHARE, PrePubMed, Scilit and Europe PMC.