Journal Description
Future Internet
- Open Access— free for readers, with article processing charges (APC) paid by authors or their institutions.
- High Visibility: indexed within Scopus, ESCI (Web of Science), Ei Compendex, dblp, Inspec, and other databases.
- Journal Rank: JCR - Q2 (Computer Science, Information Systems) / CiteScore - Q1 (Computer Networks and Communications)
- Rapid Publication: manuscripts are peer-reviewed and a first decision is provided to authors approximately 13.1 days after submission; acceptance to publication is undertaken in 2.8 days (median values for papers published in this journal in the first half of 2024).
- Recognition of Reviewers: reviewers who provide timely, thorough peer-review reports receive vouchers entitling them to a discount on the APC of their next publication in any MDPI journal, in appreciation of the work done.
Latest Articles
E-Mail Alert
News
Future Internet | Invitation to Read Editor's Choice Articles from the First Half of 2023
Meet Us at the 18th European Conference on Computer Vision, 29 September–4 October 2024, Milano, Italy
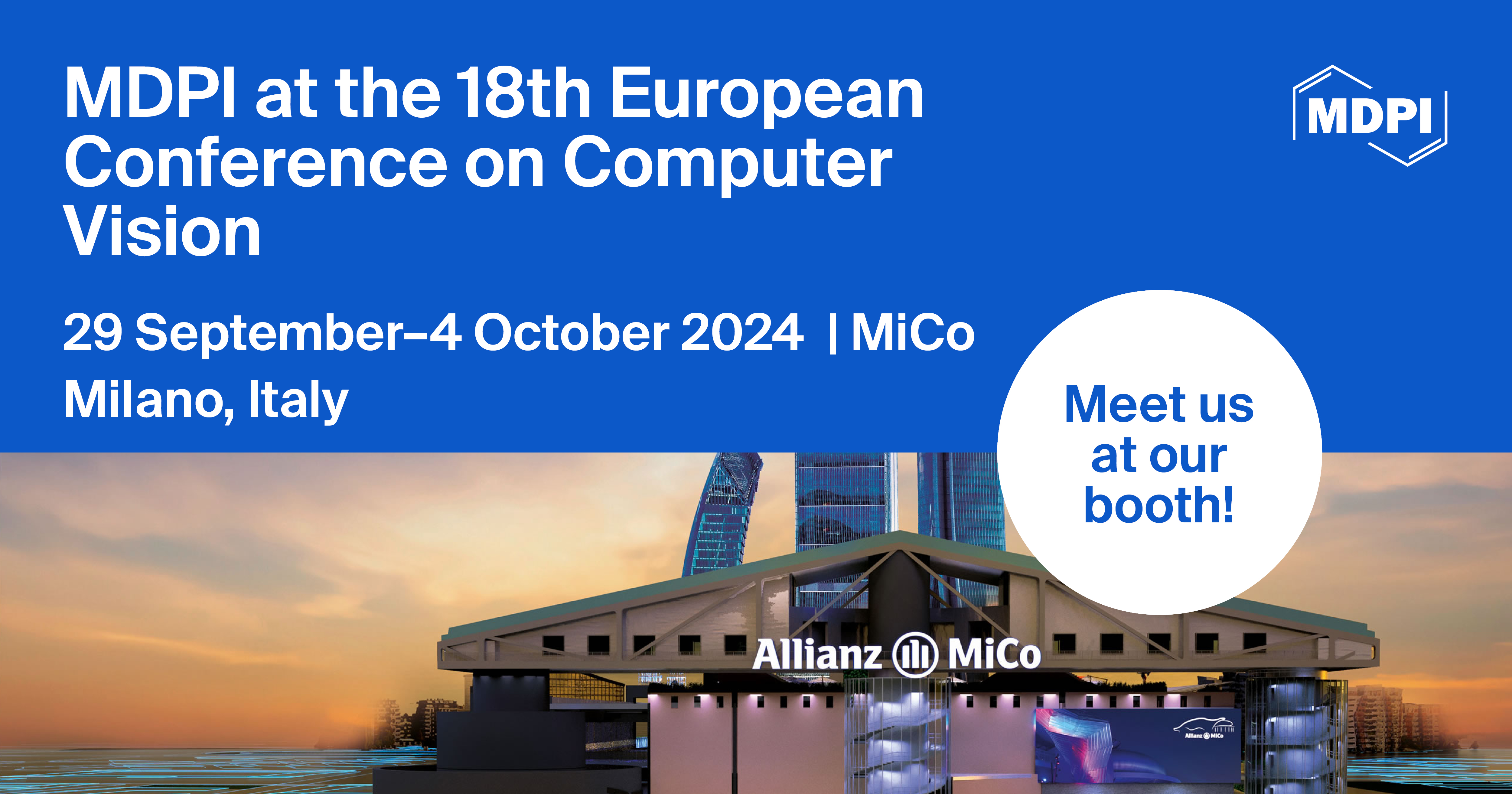
Topics
Deadline: 31 October 2024
Deadline: 20 November 2024
Deadline: 31 January 2025
Deadline: 28 February 2025
Conferences
Special Issues
Deadline: 30 September 2024
Deadline: 30 September 2024
Deadline: 30 September 2024
Deadline: 30 September 2024