Smart Energy Systems: Learning Methods for Control and Optimization
A special issue of Energies (ISSN 1996-1073). This special issue belongs to the section "F5: Artificial Intelligence and Smart Energy".
Deadline for manuscript submissions: closed (13 November 2024) | Viewed by 17118
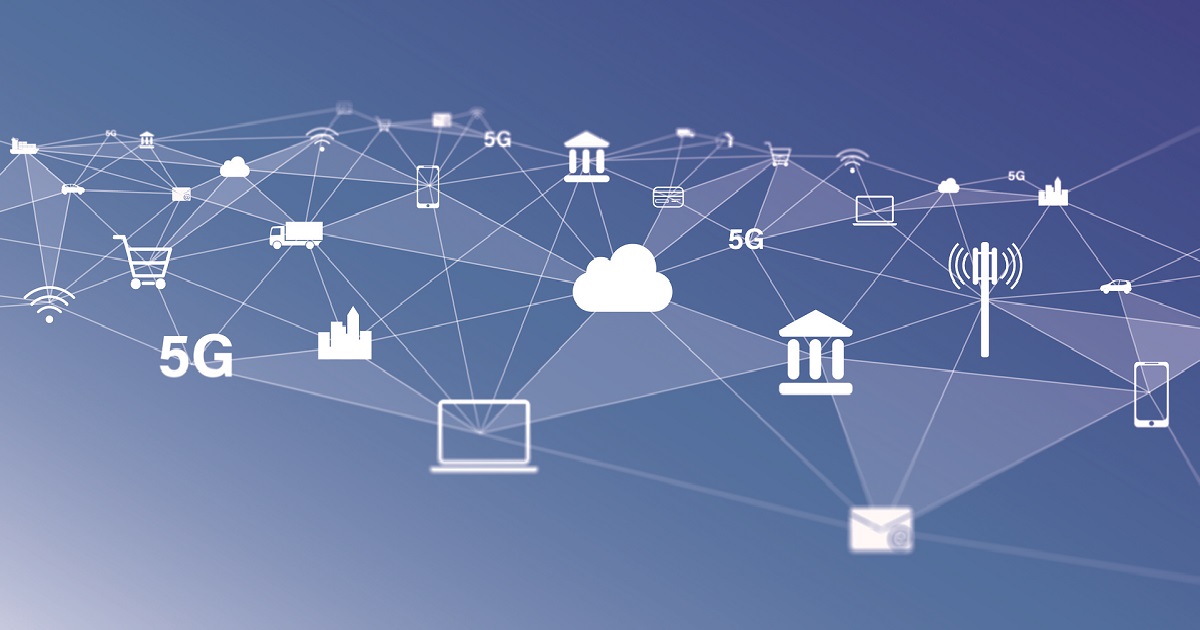
Image courtesy of Roman from Pixabay
Special Issue Editors
Interests: control; simulation; optimization; fractional calculus; evolutionary algorithms; artificial intelligence
Special Issues, Collections and Topics in MDPI journals
Interests: demand response; electricity markets; energy communities; renewable energy integration; real-time simulation; smart grids
Special Issues, Collections and Topics in MDPI journals
Special Issue Information
Dear Colleagues,
This Special Issue focuses on the application of learning methods for the control and optimization of smart energy systems, which incorporate a wide range of technologies such as renewable energy sources, distributed energy resources, smart grids, smart energy infrastructures, energy storage systems, electric vehicles, and demand response. Through the integration of these technologies, the system can balance energy supply and demand, reduce energy waste, and increase energy efficiency, moving to future renewable and sustainable energy solutions. The potential authors are encouraged to contribute to all aspects related to smart energy and sustainable energy systems. The covering of relevant up-to-date learning methods of machine learning, deep learning, reinforcement learning, and evolutionary algorithms will bring new outcomes in the development of smart energy systems control and optimization.
Prof. Dr. Ramiro Barbosa
Dr. Pedro Faria
Guest Editors
Manuscript Submission Information
Manuscripts should be submitted online at www.mdpi.com by registering and logging in to this website. Once you are registered, click here to go to the submission form. Manuscripts can be submitted until the deadline. All submissions that pass pre-check are peer-reviewed. Accepted papers will be published continuously in the journal (as soon as accepted) and will be listed together on the special issue website. Research articles, review articles as well as short communications are invited. For planned papers, a title and short abstract (about 100 words) can be sent to the Editorial Office for announcement on this website.
Submitted manuscripts should not have been published previously, nor be under consideration for publication elsewhere (except conference proceedings papers). All manuscripts are thoroughly refereed through a single-blind peer-review process. A guide for authors and other relevant information for submission of manuscripts is available on the Instructions for Authors page. Energies is an international peer-reviewed open access semimonthly journal published by MDPI.
Please visit the Instructions for Authors page before submitting a manuscript. The Article Processing Charge (APC) for publication in this open access journal is 2600 CHF (Swiss Francs). Submitted papers should be well formatted and use good English. Authors may use MDPI's English editing service prior to publication or during author revisions.
Keywords
- smart energy systems
- smart grids
- energy systems
- building energy management systems
- renewable energy and smart applications
- demand response
- artificial intelligence
- energy forecasting
- smart management of complex energy systems
- multi-agent control
- metaheuristics
- evolutionary algorithms
- neural networks
- machine learning
- deep learning
- reinforcement learning
Benefits of Publishing in a Special Issue
- Ease of navigation: Grouping papers by topic helps scholars navigate broad scope journals more efficiently.
- Greater discoverability: Special Issues support the reach and impact of scientific research. Articles in Special Issues are more discoverable and cited more frequently.
- Expansion of research network: Special Issues facilitate connections among authors, fostering scientific collaborations.
- External promotion: Articles in Special Issues are often promoted through the journal's social media, increasing their visibility.
- e-Book format: Special Issues with more than 10 articles can be published as dedicated e-books, ensuring wide and rapid dissemination.
Further information on MDPI's Special Issue policies can be found here.