Journal Description
Computation
Computation
is a peer-reviewed journal of computational science and engineering published monthly online by MDPI.
- Open Access— free for readers, with article processing charges (APC) paid by authors or their institutions.
- High Visibility: indexed within Scopus, ESCI (Web of Science), CAPlus / SciFinder, Inspec, dblp, and other databases.
- Journal Rank: JCR - Q2 (Mathematics, Interdisciplinary Applications) / CiteScore - Q2 (Applied Mathematics)
- Rapid Publication: manuscripts are peer-reviewed and a first decision is provided to authors approximately 18.6 days after submission; acceptance to publication is undertaken in 4.2 days (median values for papers published in this journal in the second half of 2024).
- Recognition of Reviewers: reviewers who provide timely, thorough peer-review reports receive vouchers entitling them to a discount on the APC of their next publication in any MDPI journal, in appreciation of the work done.
Impact Factor:
1.9 (2023);
5-Year Impact Factor:
2.0 (2023)
Latest Articles
Assessing the Validity of k-Fold Cross-Validation for Model Selection: Evidence from Bankruptcy Prediction Using Random Forest and XGBoost
Computation 2025, 13(5), 127; https://doi.org/10.3390/computation13050127 - 21 May 2025
Abstract
Predicting corporate bankruptcy is a key task in financial risk management, and selecting a machine learning model with superior generalization performance is crucial for prediction accuracy. This study evaluates the effectiveness of k-fold cross-validation as a model selection strategy for random forest and
[...] Read more.
Predicting corporate bankruptcy is a key task in financial risk management, and selecting a machine learning model with superior generalization performance is crucial for prediction accuracy. This study evaluates the effectiveness of k-fold cross-validation as a model selection strategy for random forest and XGBoost classifiers using a publicly available dataset of Taiwanese listed companies. We employ a nested cross-validation framework to assess the relationship between cross-validation (CV) and out-of-sample (OOS) performance on 40 different train/test data partitions. On average, we find k-fold cross-validation to be a valid selection technique when applied within a model class; however, k-fold cross-validation may fail for specific train/test splits. We find that 67% of model selection regret variability is explained by the particular train/test split, highlighting an irreducible uncertainty real world practitioners must contend with. Our study extensively explores hyperparameter tuning for both classifiers and highlights key insights. Additionally, we investigate practical implementation choices in k-fold cross-validation—such as the value of k or prediction strategies. We conclude that k-fold cross-validation is effective for model selection within a model class and on average, but it can be unreliable in specific cases or when comparing models from different classes—this latter issue warranting further investigation.
Full article
(This article belongs to the Special Issue Computational Approaches in Corporate Finance, Risk Management and Financial Markets)
►
Show Figures
Open AccessArticle
Simulation and Fitting of a PID Fuzzy Control in a Virtual Prototype of a Knee Orthosis
by
Agustín Barrera Sánchez, Rafael Campos Amezcua, Héctor R. Azcaray Rivera, Arturo Martínez Mata, Andrés Blanco Ortega, Cuauhtémoc Mazón Valadez and César Humberto Guzmán Valdivia
Computation 2025, 13(5), 126; https://doi.org/10.3390/computation13050126 - 21 May 2025
Abstract
Nowadays, the use of biomechanical devices in medical processes and industrial applications allows us to perform tasks in a simpler and faster way. In the medical field, these devices are becoming more and more common, especially in therapeutic applications. In the design and
[...] Read more.
Nowadays, the use of biomechanical devices in medical processes and industrial applications allows us to perform tasks in a simpler and faster way. In the medical field, these devices are becoming more and more common, especially in therapeutic applications. In the design and development of orthopedic devices, it is essential to consider the limbs’ kinematic, kinetic, and anthropometric conditions, as well as the implementation of control strategies (robust, PID, fuzzy, and impedance, among others). This work presents a virtual prototype of a knee orthosis and the implementation of a control system to follow a desired trajectory. Results are presented with the virtual prototype through a co-simulation between MSC Adams and MATLAB Simulink with fuzzy control, virtually replicating the gait cycle.
Full article
(This article belongs to the Special Issue Kinematics, Dynamics and Control for Rehabilitation Robotics and Prostheses)
►▼
Show Figures
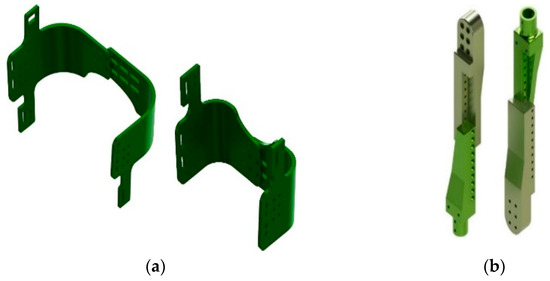
Figure 1
Open AccessArticle
Hybrid Machine Learning-Driven Automated Quality Prediction and Classification of Silicon Solar Modules in Production Lines
by
Yuxiang Liu, Xinzhong Xia, Jingyang Zhang, Kun Wang, Bo Yu, Mengmeng Wu, Jinchao Shi, Chao Ma, Ying Liu, Boyang Hu, Xinying Wang, Bo Wang, Ruzhi Wang and Bing Wang
Computation 2025, 13(5), 125; https://doi.org/10.3390/computation13050125 - 20 May 2025
Abstract
This research introduces a novel hybrid machine learning framework for automated quality prediction and classification of silicon solar modules in production lines. Unlike conventional approaches that rely solely on encapsulation loss rate (ELR) for performance evaluation—a method limited to assessing encapsulation-related
[...] Read more.
This research introduces a novel hybrid machine learning framework for automated quality prediction and classification of silicon solar modules in production lines. Unlike conventional approaches that rely solely on encapsulation loss rate (ELR) for performance evaluation—a method limited to assessing encapsulation-related power loss—our framework integrates unsupervised clustering and supervised classification to achieve a comprehensive analysis. By leveraging six critical performance parameters (open circuit voltage (VOC), short circuit current (ISC), maximum output power (Pmax), voltage at maximum power point (VPM), current at maximum power point (IPM), and fill factor (FF)), we first employ k-means clustering to dynamically categorize modules into three performance classes: excellent performance (ELR: 0–0.77%), good performance (0.77–8.39%), and poor performance (>8.39%). This multidimensional clustering approach overcomes the narrow focus of traditional ELR-based methods by incorporating photoelectric conversion efficiency and electrical characteristics. Subsequently, five machine learning classifiers—decision trees (DT), random forest (RF), k-nearest neighbors (KNN), naive Bayes classifier (NBC), and support vector machines (SVMs)—are trained to classify modules, achieving 98.90% accuracy with RF demonstrating superior robustness. Pearson correlation analysis further identifies VOC, Pmax, and VPM as the most influential quality determinants, exhibiting strong negative correlations with ELR (−0.953, −0.993, −0.959). The proposed framework not only automates module quality assessment but also enhances production line efficiency by enabling real-time anomaly detection and yield optimization. This work represents a significant advancement in solar module evaluation, bridging the gap between data-driven automation and holistic performance analysis in photovoltaic manufacturing.
Full article
(This article belongs to the Topic Advances in Computational Materials Sciences)
►▼
Show Figures
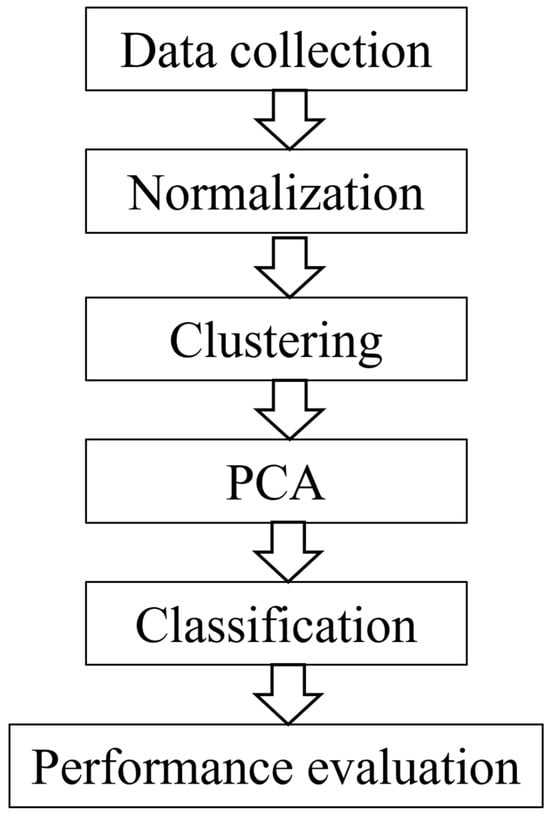
Figure 1
Open AccessArticle
MultiGNN: A Graph Neural Network Framework for Inferring Gene Regulatory Networks from Single-Cell Multi-Omics Data
by
Dongbo Liu, Hao Chen, Jianxin Wang and Yeru Wang
Computation 2025, 13(5), 124; https://doi.org/10.3390/computation13050124 - 19 May 2025
Abstract
Gene regulatory networks (GRNs) describe the interactions between transcription factors (TFs) and their target genes, playing a crucial role in understanding gene functions and how cells regulate gene expression under different conditions. Recent advancements in multi-omics technologies have provided new opportunities for more
[...] Read more.
Gene regulatory networks (GRNs) describe the interactions between transcription factors (TFs) and their target genes, playing a crucial role in understanding gene functions and how cells regulate gene expression under different conditions. Recent advancements in multi-omics technologies have provided new opportunities for more comprehensive GRN inference. Among these data types, gene expression and chromatin accessibility are particularly important, as they are key to distinguishing between direct and indirect regulatory relationships. However, existing methods primarily rely on gene expression data while neglecting biological information such as chromatin accessibility, leading to an increased occurrence of false positives in the inference results. To address the limitations of existing approaches, we propose MultiGNN, a supervised framework based on graph neural networks (GNNs). Unlike conventional GRN inference methods, MultiGNN leverages features extracted from both gene expression and chromatin accessibility data to predict regulatory interactions between genes. Experimental results demonstrate that MultiGNN consistently outperforms other methods across seven datasets. Additionally, ablation studies validate the effectiveness of our multi-omics feature integration strategy, offering a new direction for more accurate GRN inference.
Full article
(This article belongs to the Topic Computational Intelligence and Bioinformatics (CIB))
►▼
Show Figures
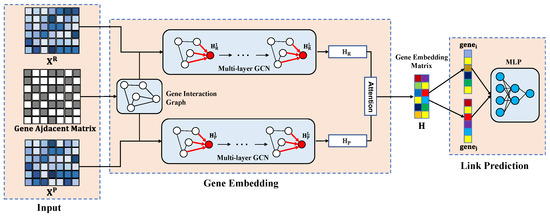
Figure 1
Open AccessArticle
Modeling Networks of Four Elements
by
Olga Kozlovska and Felix Sadyrbaev
Computation 2025, 13(5), 123; https://doi.org/10.3390/computation13050123 - 19 May 2025
Abstract
In this article, fourth-order systems of ordinary differential equations are studied. These systems are of a special form, which is used in modeling gene regulatory networks. The nonlinear part depends on the regulatory matrix W, which describes the interrelation between network elements.
[...] Read more.
In this article, fourth-order systems of ordinary differential equations are studied. These systems are of a special form, which is used in modeling gene regulatory networks. The nonlinear part depends on the regulatory matrix W, which describes the interrelation between network elements. The behavior of solutions heavily depends on this matrix and other parameters. We research the evolution of trajectories. Two approaches are employed for this. The first approach combines a fourth-order system of two two-dimensional systems and then introduces specific perturbations. This results in a system with periodic attractors that may exhibit sensitive dependence on initial conditions. The second approach involves extending a previously identified system with chaotic solution behavior to a fourth-order system. By skillfully scanning multiple parameters, this method can produce four-dimensional chaotic systems.
Full article
(This article belongs to the Section Computational Biology)
►▼
Show Figures
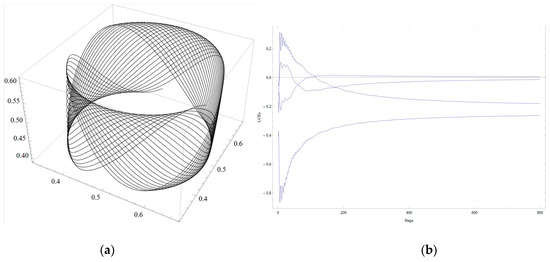
Figure 1
Open AccessArticle
Fractional Order Mathematical Model for Predicting and Controlling Dengue Fever Spread Based on Awareness Dynamics
by
Ahmed S. Rashed, Mahy M. Mahdy, Samah M. Mabrouk and Rasha Saleh
Computation 2025, 13(5), 122; https://doi.org/10.3390/computation13050122 - 17 May 2025
Abstract
Dengue fever (DF) is considered one of the most rapidly spreading infectious diseases, which is primarily transmitted to humans by bites from infected Aedes mosquitoes. The current investigation considers the spread patterns of dengue disease with and without host population awareness. It is
[...] Read more.
Dengue fever (DF) is considered one of the most rapidly spreading infectious diseases, which is primarily transmitted to humans by bites from infected Aedes mosquitoes. The current investigation considers the spread patterns of dengue disease with and without host population awareness. It is assumed that some individuals decrease their contact with infected mosquitoes by adopting precautionary behaviors due to their awareness of the disease. Certain susceptible groups actively prevent mosquito bites, and a few infected are isolated to reduce further infections. The basic reproduction number and population dynamics are modeled by a system of fractional-order differential equations. The system of equations is solved using the Adomian Decomposition Method (ADM) since it converges rapidly to the exact solution and can give explicit analytical solutions. Solutions derived are analyzed and plotted for different fractional orders, providing useful insights into population dynamics and contributing to a better understanding of the initiation and control of disease.
Full article
(This article belongs to the Topic Fractional Calculus: Theory and Applications, 2nd Edition)
►▼
Show Figures
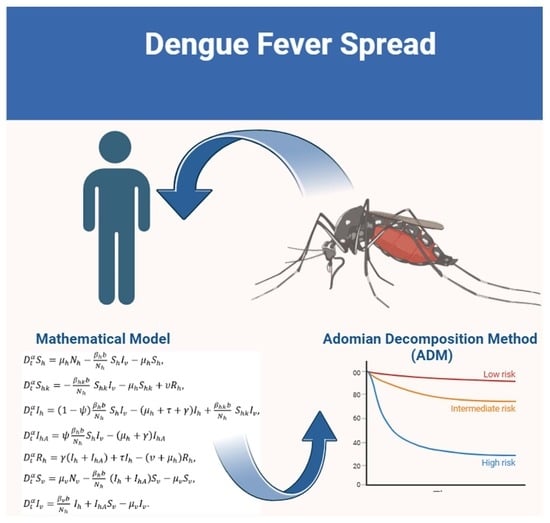
Graphical abstract
Open AccessArticle
Numerical Solution of Locally Loaded Volterra Integral Equations
by
Vladislav Byankin, Aleksandr Tynda, Denis Sidorov and Aliona Dreglea
Computation 2025, 13(5), 121; https://doi.org/10.3390/computation13050121 - 15 May 2025
Abstract
►▼
Show Figures
Loaded Volterra integral equations represent a novel class of integral equations that have attracted considerable attention in recent years due to their numerous applications in various fields of science and engineering. This class of Volterra integral equations is characterized by the presence of
[...] Read more.
Loaded Volterra integral equations represent a novel class of integral equations that have attracted considerable attention in recent years due to their numerous applications in various fields of science and engineering. This class of Volterra integral equations is characterized by the presence of a loading function, which complicates their theoretical and numerical analysis. In this paper, we study Volterra equations with locally loaded integral operators. The existence and uniqueness of their solutions are examined. A collocation-type method for the approximate solution of such equations is proposed, based on piecewise linear approximation of the exact solution. To confirm the convergence of the method, several numerical results for solving model problems are provided.
Full article
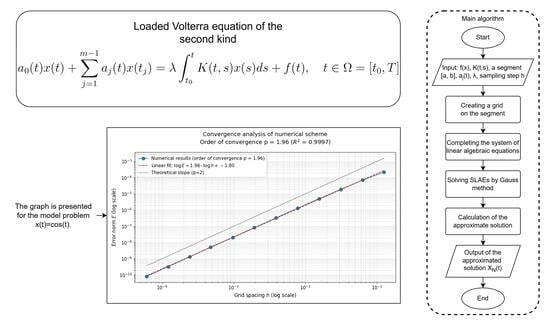
Graphical abstract
Open AccessArticle
High-Fidelity Synthetic Data Generation Framework for Unique Objects Detection
by
Nataliya Shakhovska, Bohdan Sydor, Solomiia Liaskovska, Olga Duran, Yevgen Martyn and Volodymyr Vira
Computation 2025, 13(5), 120; https://doi.org/10.3390/computation13050120 - 14 May 2025
Abstract
►▼
Show Figures
One of the key barriers to neural network adoption is the lack of computational resources and high-quality training data—particularly for unique objects without existing datasets. This research explores methods for generating realistic synthetic images that preserve the visual properties of target objects, ensuring
[...] Read more.
One of the key barriers to neural network adoption is the lack of computational resources and high-quality training data—particularly for unique objects without existing datasets. This research explores methods for generating realistic synthetic images that preserve the visual properties of target objects, ensuring their similarity to real-world appearance. We propose a flexible approach for synthetic data generation, focusing on improved accuracy and adaptability. Unlike many existing methods that rely heavily on specific generative models and require retraining with each new version, our method remains compatible with state-of-the-art models without high computational overhead. It is especially suited for user-defined objects, leveraging a 3D representation to preserve fine details and support integration into diverse environments. The approach also addresses resolution limitations by ensuring consistent object placement within high-quality scenes.
Full article
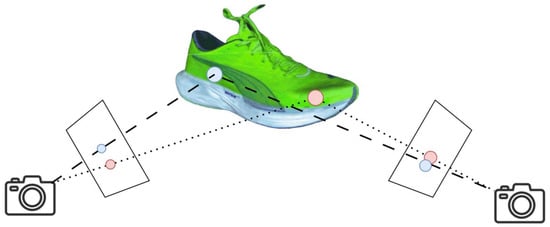
Figure 1
Open AccessArticle
Multiscale Modeling of Mechanical Response of Carbon Nanotube Yarn with Orthotropic Properties Across Hierarchies
by
Aref Mehditabar, Hossein Esfandian and Seyed Sadegh Motallebi Hasankola
Computation 2025, 13(5), 119; https://doi.org/10.3390/computation13050119 - 14 May 2025
Abstract
This study aims to comprehensively evaluate the mechanical performance of dry-spun twisted carbon nanotube (CNT) yarns (CNTYs) subjected to uniaxial tensile load. To this end, in contrast to earlier approaches, the current research lies in an innovative approach to incorporating the orthotropic properties
[...] Read more.
This study aims to comprehensively evaluate the mechanical performance of dry-spun twisted carbon nanotube (CNT) yarns (CNTYs) subjected to uniaxial tensile load. To this end, in contrast to earlier approaches, the current research lies in an innovative approach to incorporating the orthotropic properties of all hierarchical structures of a CNTY structure. The proposed bottom-up model ranges from nanoscale bundles to mesoscale fibrillar and, finally, microscale CNTYs. The proposed methodology distinguishes itself by addressing the interplay of constituents across multiple scale levels to compute the transverse properties (orthotropic nature). By doing so, rigidity and mass equivalent principles are adopted to introduce a replacement of the model by converting the truss structure containing two-node beam elements representing (vdW) van der Waals forces in a nanoscale bundle and inclined narrower bundles in mesoscale fibrillar used in previous works to the equivalent shell model. Followed by the evaluation of mechanical properties of nanoscale bundles, they are translated to the mesoscale level to quantify its orthotropic properties and then are fed into the microscale CNTY model. The results indicate that the resultant CNT bundle and fibrillar exhibit much lower transverse elastic modulus compared to those in the axial direction reported in the prior literature. For the sake of validation of the proposed method, the reproduced overall stress–strain curve of CNTYs is compared to that attained experimentally, showing excellent correlation. The presented theoretical approach provides a valuable tool for enhancing the understanding and predictive capabilities related to the mechanical performances of CNTY structures.
Full article
(This article belongs to the Topic Advances in Computational Materials Sciences)
►▼
Show Figures
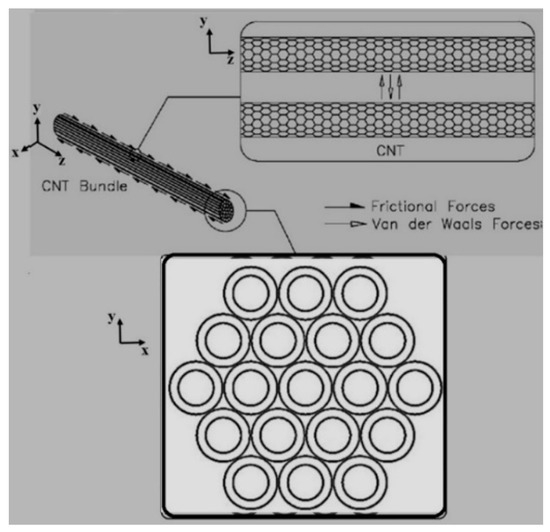
Figure 1
Open AccessArticle
Quantifying Durability and Failure Risk for Concrete Dam–Reservoir System by Using Digital Twin Technology
by
Emina Hadzalic and Adnan Ibrahimbegovic
Computation 2025, 13(5), 118; https://doi.org/10.3390/computation13050118 - 13 May 2025
Abstract
►▼
Show Figures
This study presents a digital twin approach to quantifying the durability and failure risk of concrete gravity dams by integrating advanced numerical modelling with field monitoring data. Building on a previously developed finite element model for dam–reservoir interaction analysis, this research extends its
[...] Read more.
This study presents a digital twin approach to quantifying the durability and failure risk of concrete gravity dams by integrating advanced numerical modelling with field monitoring data. Building on a previously developed finite element model for dam–reservoir interaction analysis, this research extends its application to the assessment of existing, fully operational dams by using digital twin technology. One such case study of a digital twin is given for the concrete gravity dam, Salakovac. The numerical model combines finite element formulations representing the dam as a nonisothermal saturated porous medium and the reservoir water as an acoustic fluid, ensuring realistic simulation results of their interactions. The selected finite element discrete approximations enable the detailed analysis of the dam failure mechanisms under varying extreme conditions, while simultaneously ensuring the consistent transfer of all fields (displacement, temperature, and pressure) at the dam–reservoir interface. A key aspect of this research is the calibration of the numerical model through the systematic definition of boundary conditions, external loads, and material parameters to ensure that the simulation results closely align with observed behaviour, thereby reflecting the current state of the ageing concrete dam. For the given case study of the Salakovac Dam, we illustrate the use of the digital twin to predict the failure mechanism of an ageing concrete dam for the chosen scenario of extreme loads.
Full article
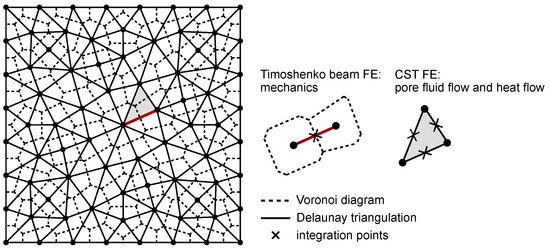
Figure 1
Open AccessArticle
Mathematical Model for Quantitative Estimation of Thermophysical Properties of Flat Samples of Potatoes by Active Thermography at Varying Boundary Layer Conditions
by
Pavel Balabanov, Andrey Egorov, Alexander Divin and Alexander N. Pchelintsev
Computation 2025, 13(5), 117; https://doi.org/10.3390/computation13050117 - 12 May 2025
Abstract
This article proposes a mathematical model for experimental estimation of the volumetric heat capacity and thermal conductivity of flat samples, in particular samples cut from potato tubers. The method involved using two pairs of samples, each of which includes the test sample and
[...] Read more.
This article proposes a mathematical model for experimental estimation of the volumetric heat capacity and thermal conductivity of flat samples, in particular samples cut from potato tubers. The method involved using two pairs of samples, each of which includes the test sample and a reference sample. The pairs of samples were pre-cooled in a refrigerator to a temperature that was 10 to 15 °C below room temperature. Then, the samples were removed from the refrigerator and placed in an air thermostat at ambient temperature, with one pair of samples additionally blown with a weak air flow. Using a thermal imager, the surface temperatures of the samples were recorded. The temperature measurement results were processed using the proposed mathematical models. The temperature measurement results of the reference samples were used to determine the Bi numbers characterizing the heat exchange conditions on the surfaces of the test samples. Taking into account the found Bi values, the volumetric heat capacity and thermal conductivity were calculated using the formulas described in the article. The article also presents a diagram of the measuring device and a method for processing experimental data using the results of experiments as an example, where potato samples were used as the test samples, and polymethyl methacrylate samples were used as the reference samples. The studies were conducted at an ambient air temperature of 20 to 24 °C and at a Bi < 0.3. The specific heat capacity of the potato samples was in the range of 2120–3795 J/(kg·K), and the thermal conductivity was in the range of 0.17–0.5 W/(m·K) with a moisture content of 10–60%.
Full article
(This article belongs to the Special Issue Mathematical Modeling and Study of Nonlinear Dynamic Processes)
►▼
Show Figures
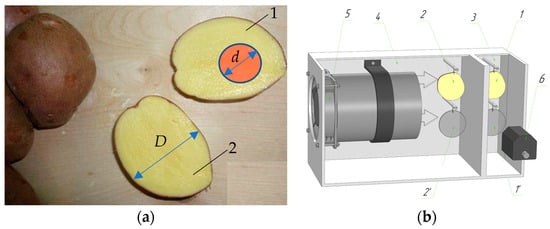
Figure 1
Open AccessArticle
An Explainable Framework Integrating Local Biplots and Gaussian Processes for Unemployment Rate Prediction in Colombia
by
Diego Armando Pérez-Rosero, Diego Alejandro Manrique-Cabezas, Jennifer Carolina Triana-Martinez, Andrés Marino Álvarez-Meza and German Castellanos-Dominguez
Computation 2025, 13(5), 116; https://doi.org/10.3390/computation13050116 - 10 May 2025
Abstract
Addressing unemployment is essential for formulating effective public policies. In particular, socioeconomic and monetary variables serve as essential indicators for anticipating labor market trends, given their strong influence on employment dynamics and economic stability. However, effective unemployment rate prediction requires addressing the non-stationary
[...] Read more.
Addressing unemployment is essential for formulating effective public policies. In particular, socioeconomic and monetary variables serve as essential indicators for anticipating labor market trends, given their strong influence on employment dynamics and economic stability. However, effective unemployment rate prediction requires addressing the non-stationary and non-linear characteristics of labor data. Equally important is the preservation of interpretability in both samples and features to ensure that forecasts can meaningfully inform public decision-making. Here, we provide an explainable framework integrating unsupervised and supervised machine learning to enhance unemployment rate prediction and interpretability. Our approach is threefold: (i) we gather a dataset for Colombian unemployment rate prediction including monetary and socioeconomic variables. (ii) Then, we used a Local Biplot technique from the widely recognized Uniform Manifold Approximation and Projection (UMAP) method along with local affine transformations as an unsupervised representation of non-stationary and non-linear data patterns in a simplified and comprehensible manner. (iii) A Gaussian Processes regressor with kernel-based feature relevance analysis is coupled as a supervised counterpart for both unemployment rate prediction and input feature importance analysis. We demonstrated the effectiveness of our proposed approach through a series of experiments conducted on our customized database focused on unemployment indicators in Colombia. Furthermore, we carried out a comparative analysis between traditional statistical techniques and modern machine learning methods. The results revealed that our framework significantly enhances both clustering and predictive performance, while also emphasizing the importance of input samples and feature selection in driving accurate outcomes.
Full article
(This article belongs to the Special Issue Applications of Machine Learning and Data Science Methods in Social Sciences)
►▼
Show Figures
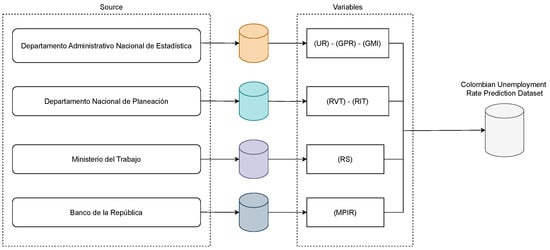
Figure 1
Open AccessArticle
Three-Dimensional and Multiple Image Encryption Algorithm Using a Fractional-Order Chaotic System
by
Ghader Ghasemi, Reza Parvaz and Yavar Khedmati Yengejeh
Computation 2025, 13(5), 115; https://doi.org/10.3390/computation13050115 - 10 May 2025
Abstract
►▼
Show Figures
The rapid development of communication in the last decade has heightened the necessity to create a secure platform for transferring data, including images, more than in previous years. One of the methods of secure image transmission is the encryption method. In this work,
[...] Read more.
The rapid development of communication in the last decade has heightened the necessity to create a secure platform for transferring data, including images, more than in previous years. One of the methods of secure image transmission is the encryption method. In this work, an encryption algorithm for multiple images is introduced. In the first step of the proposed algorithm, a key generation algorithm based on a chaotic system and wavelet transform is introduced, and in the next step, the encryption algorithm is developed by introducing rearrange and shift functions based on a chaotic system. One of the most important tools used in the proposed algorithm is the hybrid chaotic system, which is obtained by fractional derivatives and the Cat map. Different types of tests used to study the behavior of this system demonstrate the efficiency of the proposed hybrid system. In the last step of the proposed method, various statistical and security tests, including histogram analysis, correlation coefficient analysis, data loss and noise attack simulations, have been performed on the proposed algorithm. The results show that the proposed algorithm performs well in secure transmission.
Full article
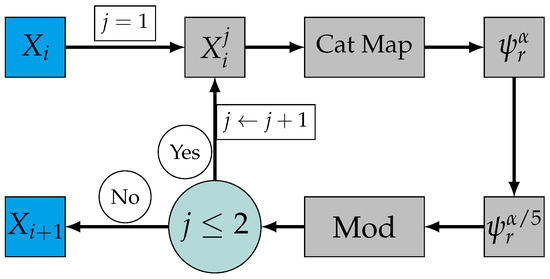
Figure 1
Open AccessArticle
Battery Sizing Method for Microgrids—A Colombian Application Case
by
Andres Felipe Zamora-Muñoz, Martha Lucia Orozco-Gutierrez, Dany Mauricio Lopez-Santiago, Jhoan Alejandro Montenegro-Oviedo and Carlos Andres Ramos-Paja
Computation 2025, 13(5), 114; https://doi.org/10.3390/computation13050114 - 10 May 2025
Abstract
The introduction of renewable energy sources in microgrids increases energy reliability, especially in small communities that operate disconnected from the main power grid. A battery energy storage system (BESS) plays an important role in microgrids because it helps mitigate the problems caused by
[...] Read more.
The introduction of renewable energy sources in microgrids increases energy reliability, especially in small communities that operate disconnected from the main power grid. A battery energy storage system (BESS) plays an important role in microgrids because it helps mitigate the problems caused by the variability of renewable energy sources, such as unattended demand and voltage instability. However, a BESS increases the cost of a microgrid due to the initial investment and maintenance, requiring a cost–benefit analysis to determine its size for each application. This paper addresses this problem by formulating a method that combines economic and technical approaches to provide favorable relations between costs and performances. Mixed integer linear programming (MILP) is used as optimization algorithm to size BESS, which is applied to an isolated community in Colombia located at Isla Múcura. The results indicate that the optimal BESS requires a maximum power of 17.6 kW and a capacity of 76.61 kWh, which is significantly smaller than the existing 480 kWh system. Thus, a reduction of 83.33% in the number of batteries is obtained. This optimized size reduces operational costs while maintaining technical reliability. The proposed method aims to solve an important problem concerning state policy and the universalization of electrical services, providing more opportunities to decision makers in minimizing the costs and efforts in the implementation of energy storage systems for isolated microgrids.
Full article
(This article belongs to the Section Computational Engineering)
►▼
Show Figures
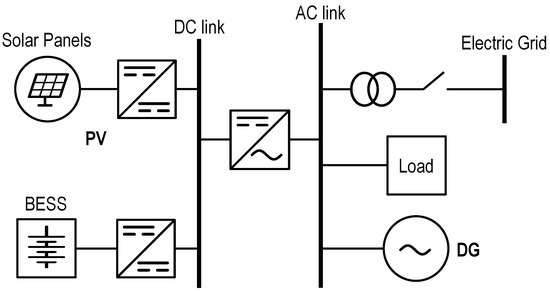
Figure 1
Open AccessArticle
How Re-Infections and Newborns Can Impact Visible and Hidden Epidemic Dynamics?
by
Igor Nesteruk
Computation 2025, 13(5), 113; https://doi.org/10.3390/computation13050113 - 9 May 2025
Abstract
Mathematical modeling allows taking into account registered and hidden infections to make correct predictions of epidemic dynamics and develop recommendations that can reduce the negative impact on public health and the economy. A model for visible and hidden epidemic dynamics (published by the
[...] Read more.
Mathematical modeling allows taking into account registered and hidden infections to make correct predictions of epidemic dynamics and develop recommendations that can reduce the negative impact on public health and the economy. A model for visible and hidden epidemic dynamics (published by the author in February 2025) has been generalized to account for the effects of re-infection and newborns. An analysis of the equilibrium points, examples of numerical solutions, and comparisons with the dynamics of real epidemics are provided. A stable quasi-equilibrium for the particular case of almost completely hidden epidemics was also revealed. Numerical results and comparisons with the COVID-19 epidemic dynamics in Austria and South Korea showed that re-infections, newborns, and hidden cases make epidemics endless. Newborns can cause repeated epidemic waves even without re-infections. In particular, the next epidemic peak of pertussis in England is expected to occur in 2031. With the use of effective algorithms for parameter identification, the proposed approach can ensure effective predictions of visible and hidden numbers of cases and infectious and removed patients.
Full article
(This article belongs to the Special Issue Artificial Intelligence Applications in Public Health: 2nd Edition)
►▼
Show Figures
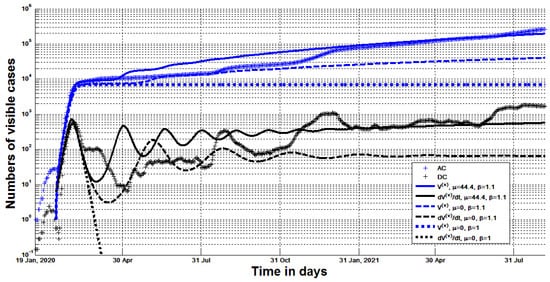
Figure 1
Open AccessArticle
A Novel Algorithm for the Decomposition of Non-Stationary Multidimensional and Multivariate Signals
by
Roberto Cavassi, Antonio Cicone, Enza Pellegrino and Haomin Zhou
Computation 2025, 13(5), 112; https://doi.org/10.3390/computation13050112 - 8 May 2025
Abstract
►▼
Show Figures
The decomposition of a signal is a fundamental tool in many fields of research, including signal processing, geophysics, astrophysics, engineering, medicine, and many more. By breaking down complex signals into simpler oscillatory components, we can enhance the understanding and processing of the data,
[...] Read more.
The decomposition of a signal is a fundamental tool in many fields of research, including signal processing, geophysics, astrophysics, engineering, medicine, and many more. By breaking down complex signals into simpler oscillatory components, we can enhance the understanding and processing of the data, unveiling hidden information contained in them. Traditional methods, such as Fourier analysis and wavelet transforms, which are effective in handling mono-dimensional stationary signals, struggle with non-stationary datasets and they require the selection of predefined basis functions. In contrast, the empirical mode decomposition (EMD) method and its variants, such as Iterative Filtering (IF), have emerged as effective non-linear approaches, adapting to signals without any need for a priori assumptions. To accelerate these methods, the Fast Iterative Filtering (FIF) algorithm was developed, and further extensions, such as Multivariate FIF (MvFIF) and Multidimensional FIF (FIF2), have been proposed to handle higher-dimensional data. In this work, we introduce the Multidimensional and Multivariate Fast Iterative Filtering (MdMvFIF) technique, an innovative method that extends FIF to handle data that varies simultaneously in space and time, like the ones sampled using sensor arrays. This new algorithm is capable of extracting Intrinsic Mode Functions (IMFs) from complex signals that vary in both space and time, overcoming limitations found in prior methods. The potentiality of the proposed method is demonstrated through applications to artificial and real-life signals, highlighting its versatility and effectiveness in decomposing multidimensional and multivariate non-stationary signals. The MdMvFIF method offers a powerful tool for advanced signal analysis across many scientific and engineering disciplines.
Full article
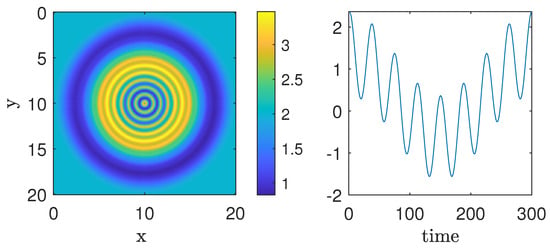
Figure 1
Open AccessArticle
Exploring the Chemical and Pharmaceutical Potential of Kapakahines A–G Using Conceptual Density Functional Theory-Based Computational Peptidology
by
Norma Flores-Holguín, Juan Frau and Daniel Glossman-Mitnik
Computation 2025, 13(5), 111; https://doi.org/10.3390/computation13050111 - 7 May 2025
Abstract
Kapakahines A–G are natural products isolated from the marine sponge Carteriospongia sp., characterized by complex molecular architectures composed of fused rings and diverse functional groups. Preliminary studies have indicated that some of these peptides may exhibit cytotoxic and antitumor activities, which has prompted
[...] Read more.
Kapakahines A–G are natural products isolated from the marine sponge Carteriospongia sp., characterized by complex molecular architectures composed of fused rings and diverse functional groups. Preliminary studies have indicated that some of these peptides may exhibit cytotoxic and antitumor activities, which has prompted interest in further exploring their chemical and pharmacokinetic properties. Computational chemistry—particularly Conceptual Density Functional Theory (CDFT)-based Computational Peptidology (CP)—offers a valuable framework for investigating such compounds. In this study, the CDFT-CP approach is applied to analyze the structural and electronic properties of Kapakahines A–G. Alongside the calculation of global and local reactivity descriptors, predicted ADMET (Absorption, Distribution, Metabolism, Excretion, and Toxicity) profiles and pharmacokinetic parameters, including pKa and LogP, are evaluated. The integrated computational analysis provides insights into the stability, reactivity, and potential drug-like behavior of these marine-derived cyclopeptides and contributes to the theoretical groundwork for future studies aimed at optimizing their bioactivity and safety profiles.
Full article
(This article belongs to the Section Computational Chemistry)
►▼
Show Figures
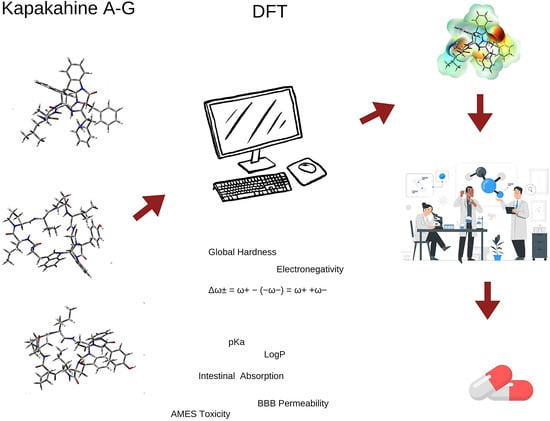
Graphical abstract
Open AccessArticle
An Algorithm Based on Connectivity Properties for Finding Cycles and Paths on Kidney Exchange Compatibility Graphs
by
Roger Z. Ríos-Mercado, L. Carolina Riascos-Álvarez and Jonathan F. Bard
Computation 2025, 13(5), 110; https://doi.org/10.3390/computation13050110 - 6 May 2025
Abstract
Kidney-paired donation programs assist patients in need of a kidney to swap their incompatible donor with another incompatible patient–donor pair for a suitable kidney in return. The kidney exchange problem (KEP) is a mathematical optimization problem that consists of finding the maximum set
[...] Read more.
Kidney-paired donation programs assist patients in need of a kidney to swap their incompatible donor with another incompatible patient–donor pair for a suitable kidney in return. The kidney exchange problem (KEP) is a mathematical optimization problem that consists of finding the maximum set of matches in a directed graph representing the pool of incompatible pairs. Depending on the specific framework, these matches can come in the form of (bounded) directed cycles or directed paths. This gives rise to a family of KEP models that have been studied over the past few years. Several of these models require an exponential number of constraints to eliminate cycles and chains that exceed a given length. In this paper, we present enhancements to a subset of existing models that exploit the connectivity properties of the underlying graphs, thereby rendering more compact and tractable models in both cycle-only and cycle-and-chain versions. In addition, an efficient algorithm is developed for detecting violated constraints and solving the problem. To assess the value of our enhanced models and algorithm, an extensive computational study was carried out comparing with existing formulations. The results demonstrated the effectiveness of the proposed approach. For example, among the main findings for edge-based cycle-only models, the proposed (*PRE(i)) model uses a new set of constraints and a small subset of the full set of length-k paths that are included in the edge formulation. The proposed model was observed to achieve a more than 98% reduction in the number of such paths among all tested instances. With respect to cycle-and-chain formulations, the proposed (*ReSPLIT) model outperformed Anderson’s arc-based (AA) formulation and the path constrained-TSP formulation on all instances that we tested. In particular, when tested on a difficult sets of instances from the literature, the proposed (*ReSPLIT) model provided the best results compared to the AA and PC-based models.
Full article
(This article belongs to the Section Computational Social Science)
►▼
Show Figures
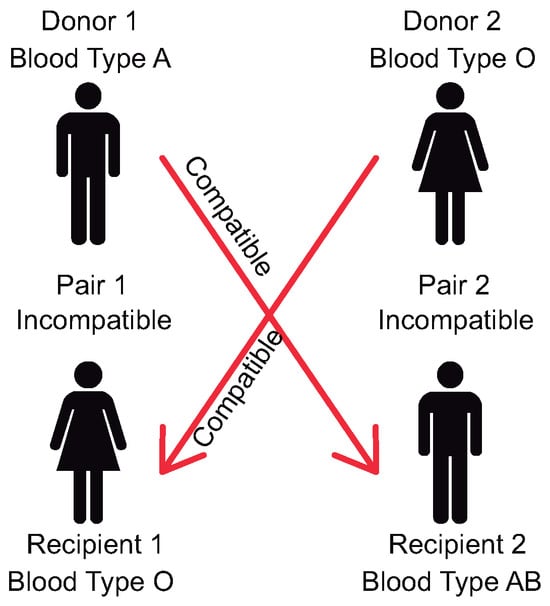
Figure 1
Open AccessArticle
Research on a Method for Identifying Key Fault Information in Substations
by
Pan Zhang, Lei Guo, Zhicheng Huang, Zhoupeng Rao, Ying Zhang, Zhi Sun, Rui Xu and Deng Li
Computation 2025, 13(5), 109; https://doi.org/10.3390/computation13050109 - 6 May 2025
Abstract
►▼
Show Figures
The identification of critical fault information plays a crucial role in ensuring the reliability and stability of power systems. However, existing fault-identification technologies heavily rely on high-dimensional sensor data, which often contain redundant and noisy information. Moreover, conventional data preprocessing approaches typically employ
[...] Read more.
The identification of critical fault information plays a crucial role in ensuring the reliability and stability of power systems. However, existing fault-identification technologies heavily rely on high-dimensional sensor data, which often contain redundant and noisy information. Moreover, conventional data preprocessing approaches typically employ fixed time windows, neglecting variations in fault characteristics under different system states. This limitation may lead to incomplete feature selection and ineffective dimensionality reduction, ultimately affecting the accuracy of fault classification. To address these challenges, this study proposes a method of critical fault information identification that integrates a scalable time window with Principal Component Analysis (PCA). The proposed method dynamically adjusts the time window size based on real-time system conditions, ensuring more flexible data capture under diverse fault scenarios. Simultaneously, PCA is employed to reduce dimensionality, extract representative features, and remove redundant noise, thereby enhancing the quality of the extracted fault information. Furthermore, this approach lays a solid foundation for the subsequent application of deep learning-based fault-diagnosis techniques. By improving feature extraction and reducing computational complexity, the proposed method effectively alleviates the workload of operation and maintenance personnel while enhancing fault classification accuracy. Our experimental results demonstrate that the proposed method significantly improves the precision and robustness of fault identification in power systems.
Full article
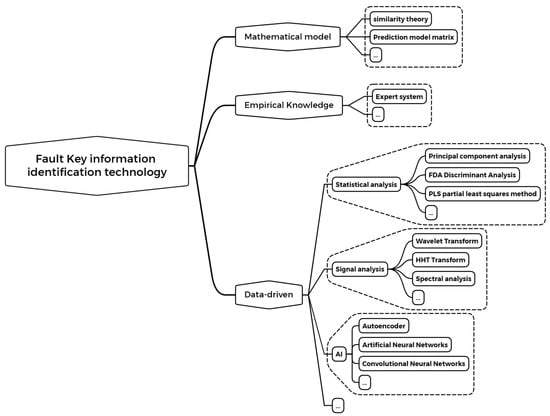
Figure 1
Open AccessArticle
AraEyebility: Eye-Tracking Data for Arabic Text Readability
by
Ibtehal Baazeem, Hend Al-Khalifa and Abdulmalik Al-Salman
Computation 2025, 13(5), 108; https://doi.org/10.3390/computation13050108 - 5 May 2025
Abstract
Assessing text readability is important for helping language learners and readers select texts that match their proficiency levels. Research in cognitive psychology, which uses behavioral data such as eye-tracking and electroencephalogram signals, has shown its effectiveness in detecting cognitive activities that correlate with
[...] Read more.
Assessing text readability is important for helping language learners and readers select texts that match their proficiency levels. Research in cognitive psychology, which uses behavioral data such as eye-tracking and electroencephalogram signals, has shown its effectiveness in detecting cognitive activities that correlate with text difficulty during reading. However, Arabic, with its distinctive linguistic characteristics, presents unique challenges in readability assessment using cognitive data. While behavioral data have been employed in readability assessments, their full potential, particularly in Arabic contexts, remains underexplored. This paper presents the development of the first Arabic eye-tracking corpus, comprising eye movement data collected from Arabic-speaking participants, with a total of 57,617 words. Subsequently, this corpus can be utilized to evaluate a broad spectrum of text-based and gaze-based features, employing machine learning and deep learning methods to improve Arabic readability assessments by integrating cognitive data into the readability assessment process.
Full article
(This article belongs to the Special Issue Recent Advances on Computational Linguistics and Natural Language Processing)
►▼
Show Figures
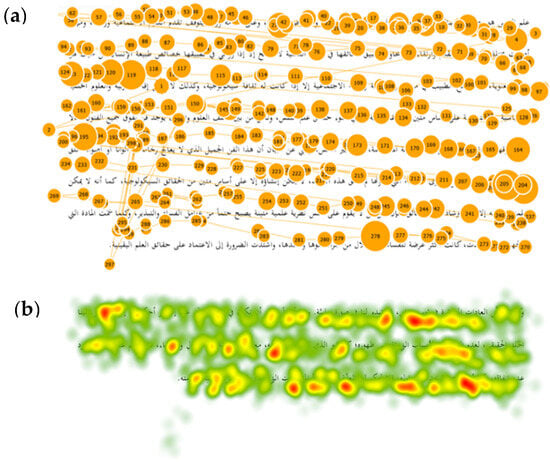
Figure 1
Highly Accessed Articles
Latest Books
E-Mail Alert
News
Topics
Topic in
Axioms, Computation, Entropy, MCA, Mathematics, Symmetry
Numerical Methods for Partial Differential Equations
Topic Editors: Pengzhan Huang, Yinnian HeDeadline: 30 June 2025
Topic in
Applied Sciences, Computation, Entropy, J. Imaging, Optics
Color Image Processing: Models and Methods (CIP: MM)
Topic Editors: Giuliana Ramella, Isabella TorcicolloDeadline: 30 July 2025
Topic in
Algorithms, Computation, Mathematics, Molecules, Symmetry, Nanomaterials, Materials
Advances in Computational Materials Sciences
Topic Editors: Cuiying Jian, Aleksander CzekanskiDeadline: 30 September 2025
Topic in
AppliedMath, Axioms, Computation, Mathematics, Symmetry
A Real-World Application of Chaos Theory
Topic Editors: Adil Jhangeer, Mudassar ImranDeadline: 28 February 2026

Conferences
Special Issues
Special Issue in
Computation
Computational Methods in Structural Engineering
Guest Editors: Manolis Georgioudakis, Vagelis Plevris, Mahdi KioumarsiDeadline: 31 May 2025
Special Issue in
Computation
Computational Medical Image Analysis—2nd Edition
Guest Editor: Anando SenDeadline: 31 May 2025
Special Issue in
Computation
Generative AI in Action: Trends, Applications, and Implications
Guest Editors: Xin Gu, Fariza SabrinaDeadline: 15 June 2025
Special Issue in
Computation
Kinematics, Dynamics and Control for Rehabilitation Robotics and Prostheses
Guest Editor: Manuel Arias-MontielDeadline: 30 June 2025