Topic Menu
► Topic MenuTopic Editors

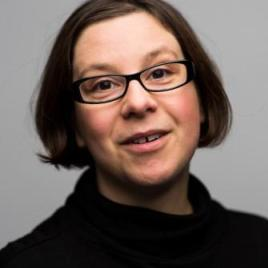
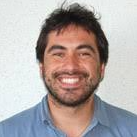
Estimating Carbon Stocks in Forest Ecosystems: From Allometric Equations to Remote Sensing-Based Methods
Topic Information
Dear Colleagues,
The determination of carbon stocks in forest ecosystems is a relevant input of global relevance. Many countries have signed the United Nations Framework Convention on Climate Change (UNFCCC) agreement, where initiatives related to reducing emissions from deforestation and forest degradation (REDD+) have been implemented. Considering such factors, rigorous methodologies regarding the quantification of carbon stocks are of paramount importance. Traditional approaches using allometric equations have been used for a long time in forest sciences. However, the accurate definition of allometric equations requires a destructive approach, which is also expensive, time-consuming, and in some cases inappropriate, considering that some species are protected by international organizations (e.g., CITES, IUCN).
Novel technologies that have become established and new ones that are being tested and validated can validly support, complement and in some cases substitute traditional methods. Remote sensing techniques and geomatics techniques in general i.e., measuring Earth data from satellite image analysis, photogrammetry, laser scanning etc.…, can be integrated by local and general allometric equations. However, even if the technologies are quite advanced, field data collection is always required, for training and/or validation.
The focus of this Special Issue is on documenting developing methodologies using existing site- and species-specific allometric equations to estimate carbon stocks and explore advanced and novel remote sensing technologies that allow measuring these data. Since allometric equations are specific to a species and to an environmental site’s condition, it will be relevant to include local equations as ground truth values. On the other hand, developing local and general allometric equations through photogrammetry, laser scanning and mobile applications can be a valid solution to the lack of allometric equations in the case of protected species which cannot undergo destructive analyses.
Suitable research papers for this Special Issue can be related to the following topics:
- Active and passive earth-observation sensors;
- Error propagation;
- Photogrammetry;
- Close-range sensing;
- Airborne and terrestrial laser scanning;
- SLAM-based hand-carried mobile laser scanning;
- Forest inventory;
- Forest biometry and modeling;
- 3D modeling and augmented reality from remote sensing data;
- Uncertainty metrics.
Dr. Francesco Pirotti
Prof. Dr. L. Monika Moskal
Dr. H. Jaime Hernández Palma
Dr. Gaia Vaglio Laurin
Mr. Erico Kutchartt
Topic Editors
Keywords
- active/passive earth observation
- photogrammetry
- close-range sensing
- error propagation
- 3D modelling
- augmented and virtual reality AR/VR
- laser scanning / lidar
Participating Journals
Journal Name | Impact Factor | CiteScore | Launched Year | First Decision (median) | APC |
---|---|---|---|---|---|
![]()
Remote Sensing
|
4.2 | 8.6 | 2009 | 23.9 Days | CHF 2700 |
![]()
Forests
|
2.4 | 4.6 | 2010 | 16.2 Days | CHF 2600 |
![]()
Sensors
|
3.4 | 8.2 | 2001 | 18.6 Days | CHF 2600 |
![]()
Geomatics
|
- | 5.1 | 2021 | 22.1 Days | CHF 1000 |
Preprints.org is a multidisciplinary platform offering a preprint service designed to facilitate the early sharing of your research. It supports and empowers your research journey from the very beginning.
MDPI Topics is collaborating with Preprints.org and has established a direct connection between MDPI journals and the platform. Authors are encouraged to take advantage of this opportunity by posting their preprints at Preprints.org prior to publication:
- Share your research immediately: disseminate your ideas prior to publication and establish priority for your work.
- Safeguard your intellectual contribution: Protect your ideas with a time-stamped preprint that serves as proof of your research timeline.
- Boost visibility and impact: Increase the reach and influence of your research by making it accessible to a global audience.
- Gain early feedback: Receive valuable input and insights from peers before submitting to a journal.
- Ensure broad indexing: Web of Science (Preprint Citation Index), Google Scholar, Crossref, SHARE, PrePubMed, Scilit and Europe PMC.