Topic Menu
► Topic MenuTopic Editors
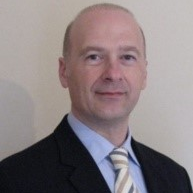
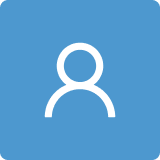

Artificial Intelligence (AI) Applied in Civil Engineering, 2nd Volume
Topic Information
Dear Colleagues,
In recent years, artificial intelligence (AI) has drawn significant attention with respect to its applications in several scientific fields, varying from big data handling to medical diagnosis. The use of AI is already present in our everyday lives with several applications, such as personalized ads, virtual assistants, autonomous driving, etc. Nowadays, AI techniques are widely used in several forms of engineering applications. It is our great pleasure to invite you to contribute to this topic by presenting your results on applications and advances of AI to civil engineering problems. The papers can focus on applications related to structural engineering, transportation engineering, geotechnical engineering, hydraulic engineering, environmental engineering, coastal and ocean engineering, and structural health monitoring, as well as construction management. Articles submitted to this Topic could also be concerned with the most significant recent developments on the topics of AI and their application in civil engineering, the papers can present modeling, optimization, control, measurements, analysis, and applications.
Prof. Dr. Nikos D. Lagaros
Dr. Stelios K. Georgantzinos
Dr. Denis Istrati
Topic Editors
Keywords
- deep learning
- IoT and real-time monitoring
- optimization
- learning systems
- mathematical and computational analysis
Participating Journals
Journal Name | Impact Factor | CiteScore | Launched Year | First Decision (median) | APC |
---|---|---|---|---|---|
![]()
AI
|
5.0 | 6.9 | 2020 | 20.7 Days | CHF 1600 |
![]()
Applied Sciences
|
2.5 | 5.5 | 2011 | 19.8 Days | CHF 2400 |
![]()
Buildings
|
3.1 | 4.4 | 2011 | 14.9 Days | CHF 2600 |
![]()
CivilEng
|
2.0 | 4.0 | 2020 | 27 Days | CHF 1400 |
![]()
Mathematics
|
2.2 | 4.6 | 2013 | 18.4 Days | CHF 2600 |
![]()
Symmetry
|
2.2 | 5.3 | 2009 | 17.1 Days | CHF 2400 |
![]()
Water
|
3.0 | 6.0 | 2009 | 19.1 Days | CHF 2600 |
Preprints.org is a multidisciplinary platform offering a preprint service designed to facilitate the early sharing of your research. It supports and empowers your research journey from the very beginning.
MDPI Topics is collaborating with Preprints.org and has established a direct connection between MDPI journals and the platform. Authors are encouraged to take advantage of this opportunity by posting their preprints at Preprints.org prior to publication:
- Share your research immediately: disseminate your ideas prior to publication and establish priority for your work.
- Safeguard your intellectual contribution: Protect your ideas with a time-stamped preprint that serves as proof of your research timeline.
- Boost visibility and impact: Increase the reach and influence of your research by making it accessible to a global audience.
- Gain early feedback: Receive valuable input and insights from peers before submitting to a journal.
- Ensure broad indexing: Web of Science (Preprint Citation Index), Google Scholar, Crossref, SHARE, PrePubMed, Scilit and Europe PMC.