Journal Description
AI
AI
is an international, peer-reviewed, open access journal on artificial intelligence (AI), including broad aspects of cognition and reasoning, perception and planning, machine learning, intelligent robotics, and applications of AI, published monthly online by MDPI.
- Open Access— free for readers, with article processing charges (APC) paid by authors or their institutions.
- High Visibility: indexed within ESCI (Web of Science), Scopus, EBSCO, and other databases.
- Journal Rank: JCR - Q1 (Computer Science, Interdisciplinary Applications) / CiteScore - Q2 (Artificial Intelligence)
- Rapid Publication: manuscripts are peer-reviewed and a first decision is provided to authors approximately 20.7 days after submission; acceptance to publication is undertaken in 3.9 days (median values for papers published in this journal in the first half of 2025).
- Recognition of Reviewers: APC discount vouchers, optional signed peer review, and reviewer names published annually in the journal.
Impact Factor:
5.0 (2024);
5-Year Impact Factor:
4.6 (2024)
Latest Articles
Geo-Statistics and Deep Learning-Based Algorithm Design for Real-Time Bus Geo-Location and Arrival Time Estimation Features with Load Resiliency Capacity
AI 2025, 6(7), 142; https://doi.org/10.3390/ai6070142 - 1 Jul 2025
Abstract
►
Show Figures
This paper introduces a groundbreaking decentralized approach for real-time bus monitoring and geo-location, leveraging advanced geo-statistical and multivariate statistical methods. The proposed long short-term memory (LSTM) model predicts bus arrival times with confidence intervals and reconstructs missing positioning data, offering cities an accurate,
[...] Read more.
This paper introduces a groundbreaking decentralized approach for real-time bus monitoring and geo-location, leveraging advanced geo-statistical and multivariate statistical methods. The proposed long short-term memory (LSTM) model predicts bus arrival times with confidence intervals and reconstructs missing positioning data, offering cities an accurate, resource-efficient tracking solution within typical infrastructure limits. By employing decentralized data processing, our system significantly reduces network traffic and computational load, enabling data sharing and sophisticated analysis. Utilizing the Haversine formula, the system estimates pessimistic and optimistic arrival times, providing real-time updates and enhancing the accuracy of bus tracking. Our innovative approach optimizes real-time bus tracking and arrival time estimation, ensuring robust performance under varying traffic conditions. This research demonstrates the potential of integrating advanced statistical techniques with decentralized computing to revolutionize public transit systems.
Full article
Open AccessArticle
ODEI: Object Detector Efficiency Index
by
Wenan Yuan
AI 2025, 6(7), 141; https://doi.org/10.3390/ai6070141 - 1 Jul 2025
Abstract
Object detectors often rely on multiple metrics to reflect their accuracy and speed performances independently. This article introduces object detector efficiency index (ODEI), a hardware-agnostic metric designed to assess object detector efficiency based on speed-normalized accuracy, utilizing established concepts including mean average precision
[...] Read more.
Object detectors often rely on multiple metrics to reflect their accuracy and speed performances independently. This article introduces object detector efficiency index (ODEI), a hardware-agnostic metric designed to assess object detector efficiency based on speed-normalized accuracy, utilizing established concepts including mean average precision (mAP) and floating-point operations (FLOPs). By defining seven mandatory parameters that must be specified when ODEI is invoked, the article aims to clarify long-standing confusions within literature regarding evaluation metrics and promote fair and transparent benchmarking research in the object detection space. Usage demonstration of ODEI using state-of-the-art (SOTA) YOLOv12 and RT-DETRv3 studies is also included.
Full article
(This article belongs to the Topic State-of-the-Art Object Detection, Tracking, and Recognition Techniques)
►▼
Show Figures
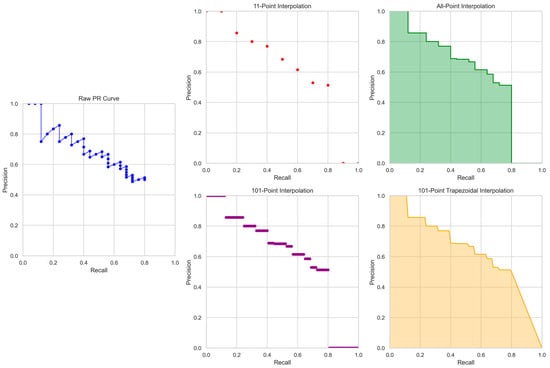
Figure 1
Open AccessArticle
A Practice-Oriented Computational Thinking Framework for Teaching Neural Networks to Working Professionals
by
Jing Tian
AI 2025, 6(7), 140; https://doi.org/10.3390/ai6070140 - 29 Jun 2025
Abstract
Background: Conventional machine learning courses are usually designed for academic learners, instead of working professionals. This study addresses this gap by proposing a new instructional framework that builds practical computational thinking skills for developing neural network models on business data. Methods: This study
[...] Read more.
Background: Conventional machine learning courses are usually designed for academic learners, instead of working professionals. This study addresses this gap by proposing a new instructional framework that builds practical computational thinking skills for developing neural network models on business data. Methods: This study proposes a five-component computational thinking framework tailed for working professionals, aligned with the standard data science pipeline and an artificial intelligence instructional taxonomy. The proposed course instructional framework consists of mixed lectures, visualization-driven and coding-driven workshops, case studies, group discussions, and gamified model tuning tasks. Results: Across 28 face-to-face course iterations conducted between 2019 and 2024, participants consistently demonstrated satisfactions in gaining computational-thinking skills. Conclusions: The tailored framework has been implemented to strengthen working professionals’ computational thinking skills for neural-network work on industrial applications.
Full article
(This article belongs to the Special Issue Development of Artificial Intelligence and Computational Thinking: Future Directions, Opportunities, and Challenges)
►▼
Show Figures
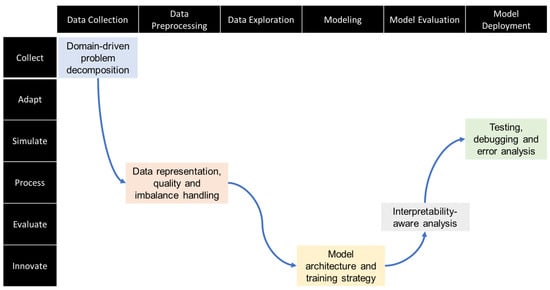
Figure 1
Open AccessArticle
Leveraging ChatGPT in K-12 School Discipline: Potential Applications and Ethical Considerations
by
Joseph C. Kush
AI 2025, 6(7), 139; https://doi.org/10.3390/ai6070139 - 27 Jun 2025
Abstract
This paper investigates the utility of an Artificial Intelligence (AI) system, as it examines AI-generated output when prompted with a series of vignettes reflecting typical disciplinary challenges encountered by K-12 students. Specifically, the study focuses on possible racial biases embedded within ChatGPT, a
[...] Read more.
This paper investigates the utility of an Artificial Intelligence (AI) system, as it examines AI-generated output when prompted with a series of vignettes reflecting typical disciplinary challenges encountered by K-12 students. Specifically, the study focuses on possible racial biases embedded within ChatGPT, a prominent language-based AI system. An analysis of AI-generated responses to disciplinary vignettes involving students of diverse racial backgrounds uncovered subtle yet prevalent racial biases present in the output. The findings indicate that while ChatGPT generally offered recommendations that were consistent and appropriate across racial lines, instances of pronounced and prejudicial disparities were observed. This study highlights the critical necessity of acknowledging and rectifying racial biases inherent in AI systems, especially in contexts where such technologies are utilized for school discipline. It provides guidance for educators and practitioners on the cautious use of AI-driven tools in disciplinary contexts, and emphasizes the ongoing imperative to mitigate biases in AI systems to ensure fair and equitable outcomes for all students, irrespective of race or ethnicity.
Full article
(This article belongs to the Special Issue AI Bias in the Media and Beyond)
Open AccessArticle
Architectural Gaps in Generative AI: Quantifying Cognitive Risks for Safety Applications
by
He Wen and Pingfan Hu
AI 2025, 6(7), 138; https://doi.org/10.3390/ai6070138 - 25 Jun 2025
Abstract
Background: The rapid integration of generative AIs, such as ChatGPT, into industrial, process, and construction management introduces both operational advantages and emerging cognitive risks. While these models support task automation and safety analysis, their internal architecture differs fundamentally from human cognition, posing
[...] Read more.
Background: The rapid integration of generative AIs, such as ChatGPT, into industrial, process, and construction management introduces both operational advantages and emerging cognitive risks. While these models support task automation and safety analysis, their internal architecture differs fundamentally from human cognition, posing interpretability and trust challenges in high-risk contexts. Methods: This study investigates whether architectural design elements in Transformer-based generative models contribute to a measurable divergence from human reasoning. A methodological framework is developed to examine core AI mechanisms—vectorization, positional encoding, attention scoring, and optimization functions—focusing on how these introduce quantifiable “distances” from human semantic understanding. Results: Through theoretical analysis and a case study involving fall prevention advice in construction, six types of architectural distances are identified and evaluated using cosine similarity and attention mapping. The results reveal misalignments in focus, semantics, and response stability, which may hinder effective human–AI collaboration in safety-critical decisions. Conclusions: These findings suggest that such distances represent not only algorithmic abstraction but also potential safety risks when generative AI is deployed in practice. The study advocates for the development of AI architectures that better reflect human cognitive structures to reduce these risks and improve reliability in safety applications.
Full article
(This article belongs to the Special Issue Leveraging Simulation and Deep Learning for Enhanced Health and Safety)
►▼
Show Figures
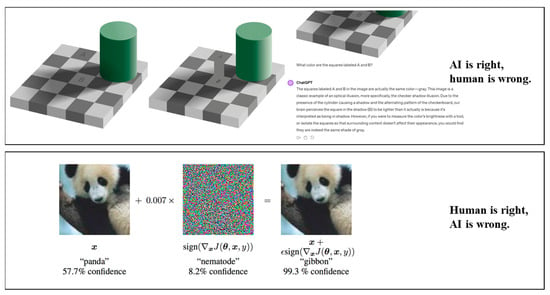
Figure 1
Open AccessArticle
AI-HOPE-TGFbeta: A Conversational AI Agent for Integrative Clinical and Genomic Analysis of TGF-β Pathway Alterations in Colorectal Cancer to Advance Precision Medicine
by
Ei-Wen Yang, Brigette Waldrup and Enrique Velazquez-Villarreal
AI 2025, 6(7), 137; https://doi.org/10.3390/ai6070137 - 24 Jun 2025
Abstract
Introduction: Early-onset colorectal cancer (EOCRC) is rising rapidly, particularly among the Hispanic/Latino (H/L) populations, who face disproportionately poor outcomes. The transforming growth factor-beta (TGF-β) signaling pathway plays a critical role in colorectal cancer (CRC) progression by mediating epithelial-to-mesenchymal transition (EMT), immune evasion, and
[...] Read more.
Introduction: Early-onset colorectal cancer (EOCRC) is rising rapidly, particularly among the Hispanic/Latino (H/L) populations, who face disproportionately poor outcomes. The transforming growth factor-beta (TGF-β) signaling pathway plays a critical role in colorectal cancer (CRC) progression by mediating epithelial-to-mesenchymal transition (EMT), immune evasion, and metastasis. However, integrative analyses linking TGF-β alterations to clinical features remain limited—particularly for diverse populations—hindering translational research and the development of precision therapies. To address this gap, we developed AI-HOPE-TGFbeta (Artificial Intelligence agent for High-Optimization and Precision Medicine focused on TGF-β), the first conversational artificial intelligence (AI) agent designed to explore TGF-β dysregulation in CRC by integrating harmonized clinical and genomic data via natural language queries. Methods: AI-HOPE-TGFbeta utilizes a large language model (LLM), Large Language Model Meta AI 3 (LLaMA 3), a natural language-to-code interpreter, and a bioinformatics backend to automate statistical workflows. Tailored for TGF-β pathway analysis, the platform enables real-time cohort stratification and hypothesis testing using harmonized datasets from the cBio Cancer Genomics Portal (cBioPortal). It supports mutation frequency comparisons, odds ratio testing, Kaplan–Meier survival analysis, and subgroup evaluations across race/ethnicity, microsatellite instability (MSI) status, tumor stage, treatment exposure, and age. The platform was validated by replicating findings on the SMAD4, TGFBR2, and BMPR1A mutations in EOCRC. Exploratory queries were conducted to examine novel associations with clinical outcomes in H/L populations. Results: AI-HOPE-TGFbeta successfully recapitulated established associations, including worse survival in SMAD4-mutant EOCRC patients treated with FOLFOX (fluorouracil, leucovorin and oxaliplatin) (p = 0.0001) and better outcomes in early-stage TGFBR2-mutated CRC patients (p = 0.00001). It revealed potential population-specific enrichment of BMPR1A mutations in H/L patients (OR = 2.63; p = 0.052) and uncovered MSI-specific survival benefits among SMAD4-mutated patients (p = 0.00001). Exploratory analysis showed better outcomes in SMAD2-mutant primary tumors vs. metastatic cases (p = 0.0010) and confirmed the feasibility of disaggregated ethnicity-based queries for TGFBR1 mutations, despite small sample sizes. These findings underscore the platform’s capacity to detect both known and emerging clinical–genomic patterns in CRC. Conclusions: AI-HOPE-TGFbeta introduces a new paradigm in cancer bioinformatics by enabling natural language-driven, real-time integration of genomic and clinical data specific to TGF-β pathway alterations in CRC. The platform democratizes complex analyses, supports disparity-focused investigation, and reveals clinically actionable insights in underserved populations, such as H/L EOCRC patients. As a first-of-its-kind system studying TGF-β, AI-HOPE-TGFbeta holds strong promise for advancing equitable precision oncology and accelerating translational discovery in the CRC TGF-β pathway.
Full article
(This article belongs to the Section Medical & Healthcare AI)
►▼
Show Figures
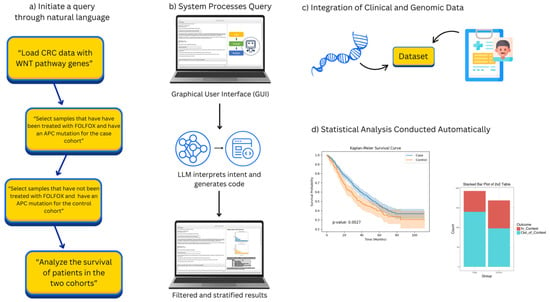
Figure 1
Open AccessArticle
Enhancing Acoustic Leak Detection with Data Augmentation: Overcoming Background Noise Challenges
by
Deniz Quick, Jens Denecke and Jürgen Schmidt
AI 2025, 6(7), 136; https://doi.org/10.3390/ai6070136 - 24 Jun 2025
Abstract
A leak detection method is developed for leaks typically encountered in industrial production. Leaks of 1 mm diameter and less are considered at operating pressures up to 10 bar. The system uses two separate datasets—one for the leak noises and the other for
[...] Read more.
A leak detection method is developed for leaks typically encountered in industrial production. Leaks of 1 mm diameter and less are considered at operating pressures up to 10 bar. The system uses two separate datasets—one for the leak noises and the other for the background noises—both are linked using a developed mixup technique and thus simulate leaks trained in background noises. A specific frequency window between 11 and 20 kHz is utilized to generate a quadratic input for image recognition. With this method, detection accuracies of over 95% with a false alarm rate under 2% can be achieved on a test dataset under the background noises of hydraulic machines in laboratory conditions.
Full article
(This article belongs to the Section AI Systems: Theory and Applications)
►▼
Show Figures

Figure 1
Open AccessArticle
High-Performance and Lightweight AI Model with Integrated Self-Attention Layers for Soybean Pod Number Estimation
by
Qian Huang
AI 2025, 6(7), 135; https://doi.org/10.3390/ai6070135 - 24 Jun 2025
Abstract
►▼
Show Figures
Background: Soybean is an important global crop in food security and agricultural economics. Accurate estimation of soybean pod counts is critical for yield prediction, breeding programs, precision farming, etc. Traditional methods, such as manual counting, are slow, labor-intensive, and prone to errors. With
[...] Read more.
Background: Soybean is an important global crop in food security and agricultural economics. Accurate estimation of soybean pod counts is critical for yield prediction, breeding programs, precision farming, etc. Traditional methods, such as manual counting, are slow, labor-intensive, and prone to errors. With rapid advancements in artificial intelligence (AI), deep learning has enabled automatic pod number estimation in collaboration with unmanned aerial vehicles (UAVs). However, existing AI models are computationally demanding and require significant processing resources (e.g., memory). These resources are often not available in rural regions and small farms. Methods: To address these challenges, this study presents a set of lightweight, efficient AI models designed to overcome these limitations. By integrating model simplification, weight quantization, and squeeze-and-excitation (SE) self-attention blocks, we develop compact AI models capable of fast and accurate soybean pod count estimation. Results and Conclusions: Experimental results show a comparable estimation accuracy of 84–87%, while the AI model size is significantly reduced by a factor of 9–65, thus making them suitable for deployment in edge devices, such as Raspberry Pi. Compared to existing models such as YOLO POD and SoybeanNet, which rely on over 20 million parameters to achieve approximately 84% accuracy, our proposed lightweight models deliver a comparable or even higher accuracy (84.0–86.76%) while using fewer than 2 million parameters. In future work, we plan to expand the dataset by incorporating diverse soybean images to enhance model generalizability. Additionally, we aim to explore more advanced attention mechanisms—such as CBAM or ECA—to further improve feature extraction and model performance. Finally, we aim to implement the complete system in edge devices and conduct real-world testing in soybean fields.
Full article
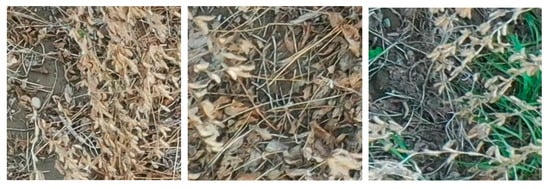
Figure 1
Open AccessArticle
Identification of Perceptual Phonetic Training Gains in a Second Language Through Deep Learning
by
Georgios P. Georgiou
AI 2025, 6(7), 134; https://doi.org/10.3390/ai6070134 - 23 Jun 2025
Abstract
►▼
Show Figures
Background/Objectives: While machine learning has made substantial strides in pronunciation detection in recent years, there remains a notable gap in the literature regarding research on improvements in the acquisition of speech sounds following a training intervention, especially in the domain of perception. This
[...] Read more.
Background/Objectives: While machine learning has made substantial strides in pronunciation detection in recent years, there remains a notable gap in the literature regarding research on improvements in the acquisition of speech sounds following a training intervention, especially in the domain of perception. This study addresses this gap by developing a deep learning algorithm designed to identify perceptual gains resulting from second language (L2) phonetic training. Methods: The participants underwent multiple sessions of high-variability phonetic training, focusing on discriminating challenging L2 vowel contrasts. The deep learning model was trained on perceptual data collected before and after the intervention. Results: The results demonstrated good model performance across a range of metrics, confirming that learners’ gains in phonetic training could be effectively detected by the algorithm. Conclusions: This research underscores the potential of deep learning techniques to track improvements in phonetic training, offering a promising and practical approach for evaluating language learning outcomes and paving the way for more personalized, adaptive language learning solutions. Deep learning enables the automatic extraction of complex patterns in learner behavior that might be missed by traditional methods. This makes it especially valuable in educational contexts where subtle improvements need to be captured and assessed objectively.
Full article
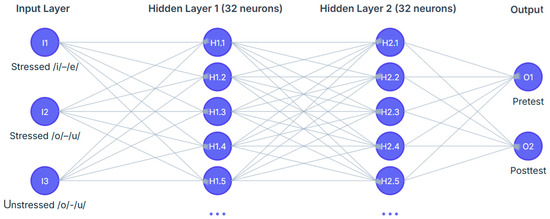
Figure 1
Open AccessArticle
Machine Learning-Based Predictive Maintenance for Photovoltaic Systems
by
Ali Al-Humairi, Enmar Khalis, Zuhair A. Al-Hemyari and Peter Jung
AI 2025, 6(7), 133; https://doi.org/10.3390/ai6070133 - 20 Jun 2025
Abstract
►▼
Show Figures
The performance of photovoltaic systems is highly dependent on environmental conditions, with soiling due to dust accumulation often being referred to as a predominant energy degradation factor, especially in dry and semi-arid environments. This paper introduces an AI-based robotic cleaning system that can
[...] Read more.
The performance of photovoltaic systems is highly dependent on environmental conditions, with soiling due to dust accumulation often being referred to as a predominant energy degradation factor, especially in dry and semi-arid environments. This paper introduces an AI-based robotic cleaning system that can independently forecast and schedule cleaning sessions from real-time sensor and environmental data. Methods: The system integrates sources of data like embedded sensors, weather stations, and DustIQ data to create an integrated dataset for predictive modeling. Machine learning models were employed to forecast soiling loss based on significant atmospheric parameters such as relative humidity, air pressure, ambient temperature, and wind speed. Dimensionality reduction through the principal component analysis and correlation-based feature selection enhanced the model performance as well as the interpretability. A comparative study of four conventional machine learning models, including logistic regression, k-nearest neighbors, decision tree, and support vector machine, was conducted to determine the most appropriate approach to classifying cleaning needs. Results: Performance, based on accuracy, precision, recall, and F1-score, demonstrated that logistic regression and SVM provided optimal classification performance with accuracy levels over 92%, and F1-scores over 0.90, demonstrating outstanding balance between recall and precision. The KNN and decision tree models, while slightly poorer in terms of accuracy (around 85–88%), had computational efficiency benefits, making them suitable for utilization in resource-constrained applications. Conclusions: The proposed system employs a dry-cleaning mechanism that requires no water, making it highly suitable for arid regions. It reduces unnecessary cleaning operations by approximately 30%, leading to decreased mechanical wear and lower maintenance costs. Additionally, by minimizing delays in necessary cleaning, the system can improve annual energy yield by 3–5% under high-soiling conditions. Overall, the intelligent cleaning schedule minimizes manual intervention, enhances sustainability, reduces operating costs, and improves system performance in challenging environments.
Full article
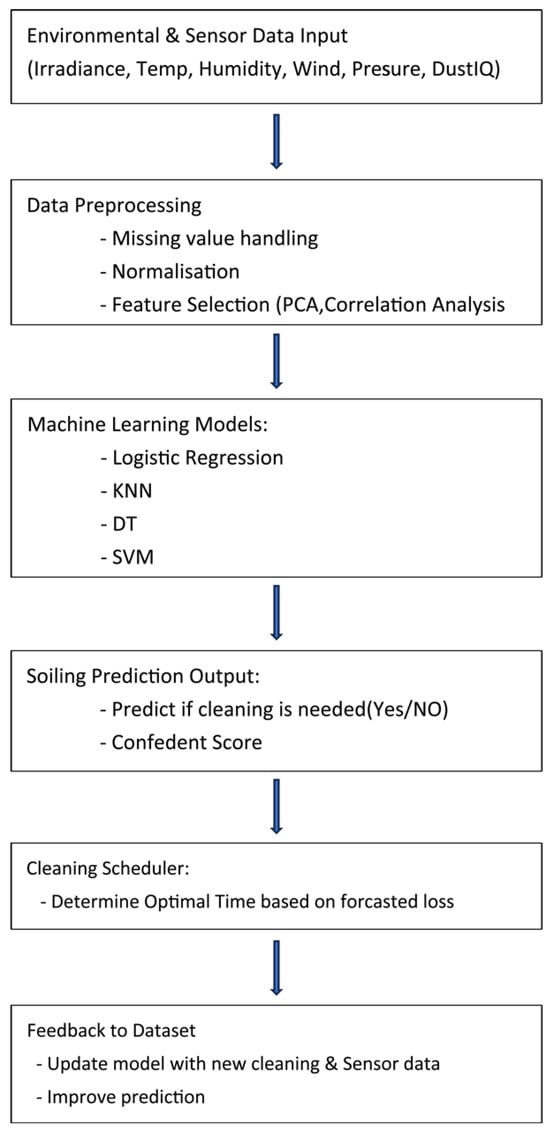
Figure 1
Open AccessArticle
AEA-YOLO: Adaptive Enhancement Algorithm for Challenging Environment Object Detection
by
Abdulrahman Kariri and Khaled Elleithy
AI 2025, 6(7), 132; https://doi.org/10.3390/ai6070132 - 20 Jun 2025
Abstract
Despite deep learning-based object detection techniques showing promising results, identifying items from low-quality images under unfavorable weather settings remains challenging because of balancing demands and overlooking useful latent information. On the other hand, YOLO is being developed for real-time object detection, addressing limitations
[...] Read more.
Despite deep learning-based object detection techniques showing promising results, identifying items from low-quality images under unfavorable weather settings remains challenging because of balancing demands and overlooking useful latent information. On the other hand, YOLO is being developed for real-time object detection, addressing limitations of current models, which struggle with low accuracy and high resource requirements. To address these issues, we provide an Adaptive Enhancement Algorithm YOLO (AEA-YOLO) framework that allows for an enhancement in each image for improved detection capabilities. A lightweight Parameter Prediction Network (PPN) containing only six thousand parameters predicts scene-adaptive coefficients for a differentiable Image Enhancement Module (IEM), and the enhanced image is then processed by a standard YOLO detector, called the Detection Network (DN). Adaptively processing images in both favorable and unfavorable weather conditions is possible with our suggested method. Extremely encouraging experimental results compared with existing models show that our suggested approach achieves 7% and more than 12% in mean average precision (mAP) on the PASCAL VOC Foggy artificially degraded and the Real-world Task-driven Testing Set (RTTS) datasets. Moreover, our approach achieves good results compared with other state-of-the-art and adaptive domain models of object detection in normal and challenging environments.
Full article
(This article belongs to the Section AI Systems: Theory and Applications)
►▼
Show Figures
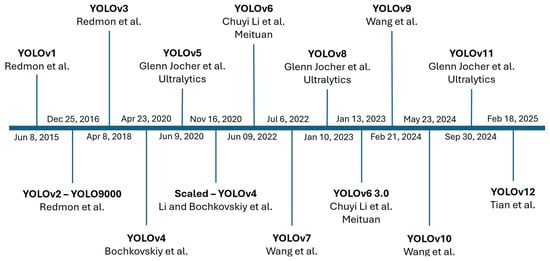
Figure 1
Open AccessArticle
Resilient Anomaly Detection in Fiber-Optic Networks: A Machine Learning Framework for Multi-Threat Identification Using State-of-Polarization Monitoring
by
Gulmina Malik, Imran Chowdhury Dipto, Muhammad Umar Masood, Mashboob Cheruvakkadu Mohamed, Stefano Straullu, Sai Kishore Bhyri, Gabriele Maria Galimberti, Antonio Napoli, João Pedro, Walid Wakim and Vittorio Curri
AI 2025, 6(7), 131; https://doi.org/10.3390/ai6070131 - 20 Jun 2025
Abstract
We present a thorough machine-learning framework based on real-time state-of-polarization (SOP) monitoring for robust anomaly identification in optical fiber networks. We exploit SOP data under three different threat scenarios: (i) malicious or critical vibration events, (ii) overlapping mechanical disturbances, and (iii) malicious fiber
[...] Read more.
We present a thorough machine-learning framework based on real-time state-of-polarization (SOP) monitoring for robust anomaly identification in optical fiber networks. We exploit SOP data under three different threat scenarios: (i) malicious or critical vibration events, (ii) overlapping mechanical disturbances, and (iii) malicious fiber tapping (eavesdropping). We used various supervised machine learning techniques like k-Nearest Neighbor (k-NN), random forest, extreme gradient boosting (XGBoost), and decision trees to classify different vibration events. We also assessed the framework’s resilience to background interference by superimposing sinusoidal noise at different frequencies and examining its effects on the polarization signatures. This analysis provides insight into how subsurface installations, subject to ambient vibrations, affect detection fidelity. This highlights the sensitivity to which external interference affects polarization fingerprints. Crucially, it demonstrates the system’s capacity to discern and alert on malicious vibration events even in the presence of environmental noise. However, we focus on the necessity of noise-mitigation techniques in real-world implementations while providing a potent, real-time mechanism for multi-threat recognition in the fiber networks.
Full article
(This article belongs to the Special Issue Artificial Intelligence in Optical Communication Networks)
►▼
Show Figures
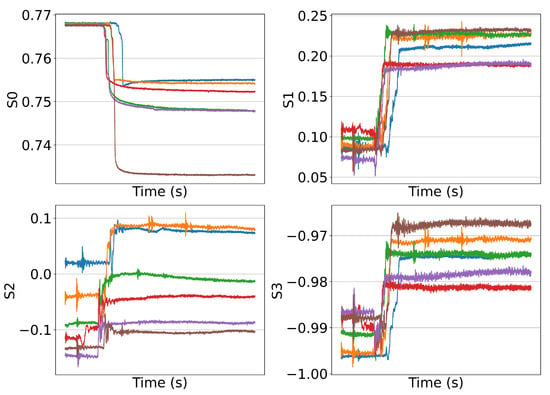
Figure 1
Open AccessFeature PaperArticle
Early Detection of the Marathon Wall to Improve Pacing Strategies in Recreational Marathoners
by
Mohamad-Medhi El Dandachi, Veronique Billat, Florent Palacin and Vincent Vigneron
AI 2025, 6(6), 130; https://doi.org/10.3390/ai6060130 - 19 Jun 2025
Abstract
►▼
Show Figures
The individual marathon optimal pacing sparring the runner to hit the “wall” after 2 h of running remain unclear. In the current study we examined to what extent Deep neural Network contributes to identify the individual optimal pacing training a Variational Auto Encoder
[...] Read more.
The individual marathon optimal pacing sparring the runner to hit the “wall” after 2 h of running remain unclear. In the current study we examined to what extent Deep neural Network contributes to identify the individual optimal pacing training a Variational Auto Encoder (VAE) with a small dataset of nine runners. This last one has been constructed from an original one that contains the values of multiple physiological variables for 10 different runners during a marathon. We plot the Lyapunov exponent/Time graph on these variables for each runner showing that the marathon wall could be anticipated. The pacing strategy that this innovative technique sheds light on is to predict and delay the moment when the runner empties his reserves and ’hits the wall’ while considering the individual physical capabilities of each athlete. Our data suggest that given that a further increase of marathon runner using a cardio-GPS could benefit of their pacing run for optimizing their performance if AI would be used for learning how to self-pace his marathon race for avoiding hitting the wall.
Full article
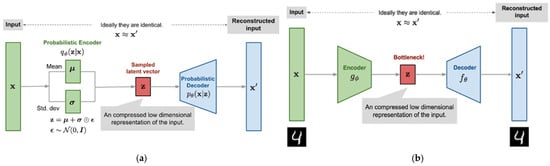
Figure 1
Open AccessArticle
Designing Ship Hull Forms Using Generative Adversarial Networks
by
Kazuo Yonekura, Kotaro Omori, Xinran Qi and Katsuyuki Suzuki
AI 2025, 6(6), 129; https://doi.org/10.3390/ai6060129 - 18 Jun 2025
Abstract
►▼
Show Figures
We proposed a GAN-based method to generate a ship hull form. Unlike mathematical hull forms that require geometrical parameters to generate ship hull forms, the proposed method requires desirable ship performance parameters, i.e., the drag coefficient and tonnage. The objective of this study
[...] Read more.
We proposed a GAN-based method to generate a ship hull form. Unlike mathematical hull forms that require geometrical parameters to generate ship hull forms, the proposed method requires desirable ship performance parameters, i.e., the drag coefficient and tonnage. The objective of this study is to demonstrate the feasibility of generating hull geometries directly from performance specifications, without relying on explicit geometrical inputs. To achieve this, we implemented a conditional Wasserstein GAN with gradient penalty (cWGAN-GP) framework. The generator learns to synthesize hull geometries conditioned on target performance values, while the discriminator is trained to distinguish real hull forms from generated ones. The GAN model was trained using a ship hull form dataset generated using the generalized Wigley hull form. The proposed method was evaluated through numerical experiments and successfully generated ship data with small errors: less than 0.08 in mean average percentage error.
Full article
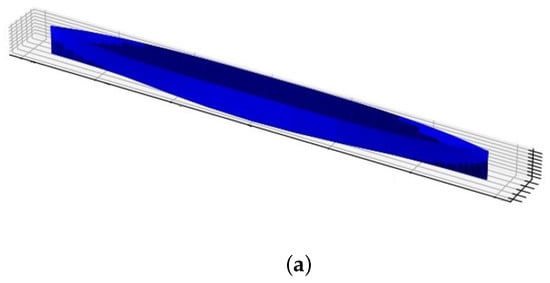
Figure 1
Open AccessArticle
The Proof Is in the Eating: Lessons Learnt from One Year of Generative AI Adoption in a Science-for-Policy Organisation
by
Bertrand De Longueville, Ignacio Sanchez, Snezha Kazakova, Stefano Luoni, Fabrizio Zaro, Kalliopi Daskalaki and Marco Inchingolo
AI 2025, 6(6), 128; https://doi.org/10.3390/ai6060128 - 17 Jun 2025
Abstract
►▼
Show Figures
This paper presents the key results of a large-scale empirical study on the adoption of Generative AI (GenAI) by the Joint Research Centre (JRC), the European Commission’s science-for-policy department. Since spring 2023, the JRC has developed and deployed GPT@JRC, a platform providing safe
[...] Read more.
This paper presents the key results of a large-scale empirical study on the adoption of Generative AI (GenAI) by the Joint Research Centre (JRC), the European Commission’s science-for-policy department. Since spring 2023, the JRC has developed and deployed GPT@JRC, a platform providing safe and compliant access to state-of-the-art Large Language Models for over 10,000 knowledge workers. While the literature highlighting the potential of GenAI to enhance productivity for knowledge-intensive tasks is abundant, there is a scarcity of empirical evidence on impactful use case types and success factors. This study addresses this gap and proposes the JRC GenAI Compass conceptual framework based on the lessons learnt from the JRC’s GenAI adoption journey. It includes the concept of AI-IQ, which reflects the complexity of a given GenAI system. This paper thus draws on a case study of enterprise-scale AI implementation in European public institutions to provide approaches to harness GenAI’s potential while mitigating the risks.
Full article
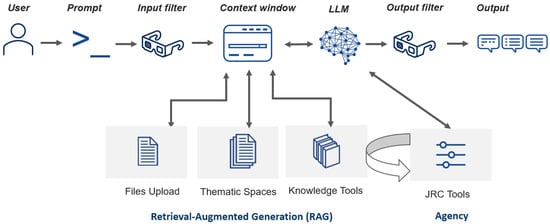
Figure 1
Open AccessArticle
NSA-CHG: An Intelligent Prediction Framework for Real-Time TBM Parameter Optimization in Complex Geological Conditions
by
Youliang Chen, Wencan Guan, Rafig Azzam and Siyu Chen
AI 2025, 6(6), 127; https://doi.org/10.3390/ai6060127 - 16 Jun 2025
Abstract
►▼
Show Figures
This study proposes an intelligent prediction framework integrating native sparse attention (NSA) with the Chen-Guan (CHG) algorithm to optimize tunnel boring machine (TBM) operations in heterogeneous geological environments. The framework resolves critical limitations of conventional experience-driven approaches that inadequately address the nonlinear coupling
[...] Read more.
This study proposes an intelligent prediction framework integrating native sparse attention (NSA) with the Chen-Guan (CHG) algorithm to optimize tunnel boring machine (TBM) operations in heterogeneous geological environments. The framework resolves critical limitations of conventional experience-driven approaches that inadequately address the nonlinear coupling between the spatial heterogeneity of rock mass parameters and mechanical system responses. Three principal innovations are introduced: (1) a hardware-compatible sparse attention architecture achieving O(n) computational complexity while preserving high-fidelity geological feature extraction capabilities; (2) an adaptive kernel function optimization mechanism that reduces confidence interval width by 41.3% through synergistic integration of boundary likelihood-driven kernel selection with Chebyshev inequality-based posterior estimation; and (3) a physics-enhanced modelling methodology combining non-Hertzian contact mechanics with eddy field evolution equations. Validation experiments employing field data from the Pujiang Town Plot 125-2 Tunnel Project demonstrated superior performance metrics, including 92.4% ± 1.8% warning accuracy for fractured zones, ≤28 ms optimization response time, and ≤4.7% relative error in energy dissipation analysis. Comparative analysis revealed a 32.7% reduction in root mean square error (p < 0.01) and 4.8-fold inference speed acceleration relative to conventional methods, establishing a novel data–physics fusion paradigm for TBM control with substantial implications for intelligent tunnelling in complex geological formations.
Full article
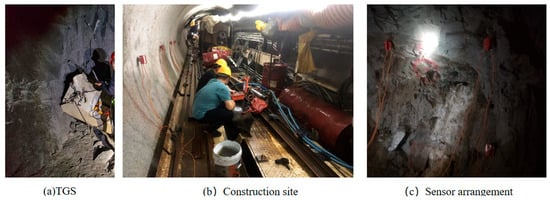
Figure 1
Open AccessReview
Artificial Intelligence Empowering Dynamic Spectrum Access in Advanced Wireless Communications: A Comprehensive Overview
by
Abiodun Gbenga-Ilori, Agbotiname Lucky Imoize, Kinzah Noor and Paul Oluwadara Adebolu-Ololade
AI 2025, 6(6), 126; https://doi.org/10.3390/ai6060126 - 13 Jun 2025
Abstract
This review paper examines the integration of artificial intelligence (AI) in wireless communication, focusing on cognitive radio (CR), spectrum sensing, and dynamic spectrum access (DSA). As the demand for spectrum continues to rise with the expansion of mobile users and connected devices, cognitive
[...] Read more.
This review paper examines the integration of artificial intelligence (AI) in wireless communication, focusing on cognitive radio (CR), spectrum sensing, and dynamic spectrum access (DSA). As the demand for spectrum continues to rise with the expansion of mobile users and connected devices, cognitive radio networks (CRNs), leveraging AI-driven spectrum sensing and dynamic access, provide a promising solution to improve spectrum utilization. The paper reviews various deep learning (DL)-based spectrum-sensing methods, highlighting their advantages and challenges. It also explores the use of multi-agent reinforcement learning (MARL) for distributed DSA networks, where agents autonomously optimize power allocation (PA) to minimize interference and enhance quality of service. Additionally, the paper discusses the role of machine learning (ML) in predicting spectrum requirements, which is crucial for efficient frequency management in the fifth generation (5G) networks and beyond. Case studies show how ML can help self-optimize networks, reducing energy consumption while improving performance. The review also introduces the potential of generative AI (GenAI) for demand-planning and network optimization, enhancing spectrum efficiency and energy conservation in wireless networks (WNs). Finally, the paper highlights future research directions, including improving AI-driven network resilience, refining predictive models, and addressing ethical considerations. Overall, AI is poised to transform wireless communication, offering innovative solutions for spectrum management (SM), security, and network performance.
Full article
(This article belongs to the Special Issue Artificial Intelligence for Network Management)
►▼
Show Figures
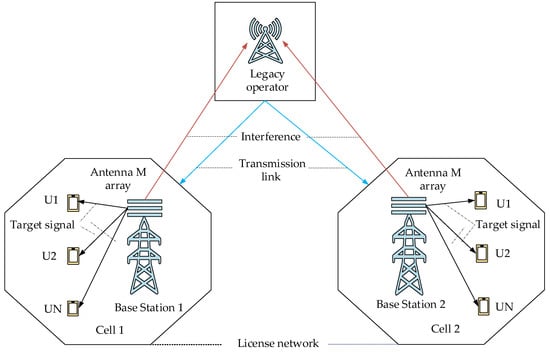
Figure 1
Open AccessArticle
Advanced Interpretation of Bullet-Affected Chest X-Rays Using Deep Transfer Learning
by
Shaheer Khan, Nirban Bhowmick, Azib Farooq, Muhammad Zahid, Sultan Shoaib, Saqlain Razzaq, Abdul Razzaq and Yasar Amin
AI 2025, 6(6), 125; https://doi.org/10.3390/ai6060125 - 13 Jun 2025
Abstract
Deep learning has brought substantial progress to medical imaging, which has resulted in continuous improvements in diagnostic procedures. Through deep learning architecture implementations, radiology professionals achieve automated pathological condition detection, segmentation, and classification with improved accuracy. The research tackles a rarely studied clinical
[...] Read more.
Deep learning has brought substantial progress to medical imaging, which has resulted in continuous improvements in diagnostic procedures. Through deep learning architecture implementations, radiology professionals achieve automated pathological condition detection, segmentation, and classification with improved accuracy. The research tackles a rarely studied clinical medical imaging issue that involves bullet identification and positioning within X-ray images. The purpose is to construct a sturdy deep learning system that will identify and classify ballistic trauma in images. Our research examined various deep learning models that functioned either as classifiers or as object detectors to develop effective solutions for ballistic trauma detection in X-ray images. Research data was developed by replicating controlled bullet damage in chest X-rays while expanding to a wider range of anatomical areas that include the legs, abdomen, and head. Special deep learning algorithms went through a process of optimization before researchers improved their ability to detect and place objects. Multiple computational systems were used to verify the results, which showcased the effectiveness of the proposed solution. This research provides new perspectives on understanding forensic radiology trauma assessment by developing the first deep learning system that detects and classifies gun-related radiographic injuries automatically. The first system for forensic radiology designed with automated deep learning to classify gunshot wounds in radiographs is introduced by this research. This approach offers new ways to look at trauma which is helpful for work in clinics as well as in law enforcement.
Full article
(This article belongs to the Special Issue Multimodal Artificial Intelligence in Healthcare)
►▼
Show Figures
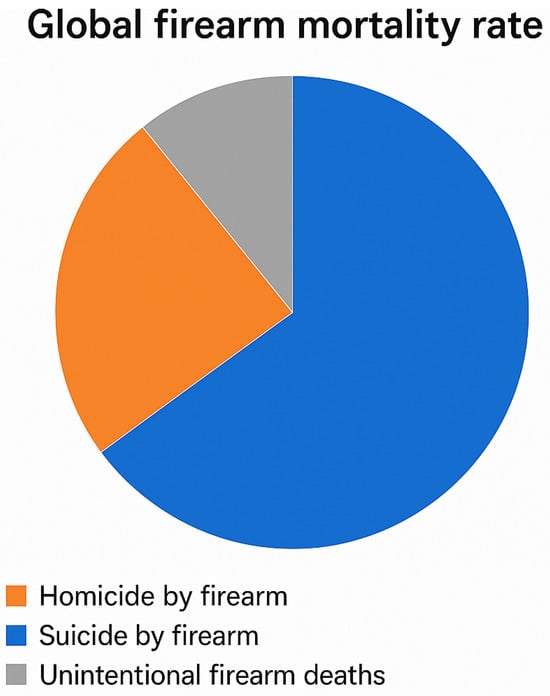
Figure 1
Open AccessReview
Advances of Machine Learning in Phased Array Ultrasonic Non-Destructive Testing: A Review
by
Yiming Na, Yunze He, Baoyuan Deng, Xiaoxia Lu, Hongjin Wang, Liwen Wang and Yi Cao
AI 2025, 6(6), 124; https://doi.org/10.3390/ai6060124 - 12 Jun 2025
Abstract
Recent advancements in machine learning (ML) have led to state-of-the-art performance in various domain-specific tasks, driving increasing interest in its application to non-destructive testing (NDT). Among NDT techniques, phased array ultrasonic testing (PAUT) is an advanced extension of conventional ultrasonic testing (UT). This
[...] Read more.
Recent advancements in machine learning (ML) have led to state-of-the-art performance in various domain-specific tasks, driving increasing interest in its application to non-destructive testing (NDT). Among NDT techniques, phased array ultrasonic testing (PAUT) is an advanced extension of conventional ultrasonic testing (UT). This article provides an overview of recent research advances in ML applied to PAUT, covering key applications such as phased array ultrasonic imaging, defect detection and characterization, and data generation, with a focus on multimodal data processing and multidimensional modeling. The challenges and pathways for integrating the two techniques are examined. Finally, the article discusses the limitations of current methodologies and outlines future research directions toward more accurate, interpretable, and efficient ML-powered PAUT solutions.
Full article
(This article belongs to the Section AI Systems: Theory and Applications)
►▼
Show Figures
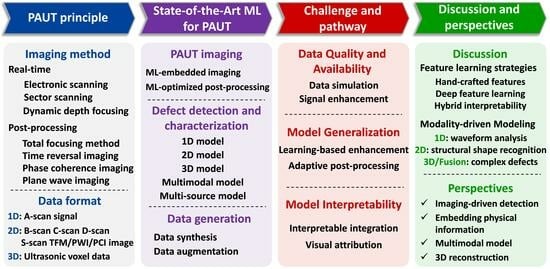
Graphical abstract
Open AccessSystematic Review
Agentic AI Frameworks in SMMEs: A Systematic Literature Review of Ecosystemic Interconnected Agents
by
Peter Adebowale Olujimi, Pius Adewale Owolawi, Refilwe Constance Mogase and Etienne Van Wyk
AI 2025, 6(6), 123; https://doi.org/10.3390/ai6060123 - 11 Jun 2025
Abstract
This study examines the application of agentic artificial intelligence (AI) frameworks within small, medium, and micro-enterprises (SMMEs), highlighting how interconnected autonomous agents improve operational efficiency and adaptability. Using the PRISMA 2020 framework, this study systematically identified, screened, and analyzed 66 studies, including peer-reviewed
[...] Read more.
This study examines the application of agentic artificial intelligence (AI) frameworks within small, medium, and micro-enterprises (SMMEs), highlighting how interconnected autonomous agents improve operational efficiency and adaptability. Using the PRISMA 2020 framework, this study systematically identified, screened, and analyzed 66 studies, including peer-reviewed and credible gray literature, published between 2019 and 2024, to assess agentic AI frameworks in SMMEs. Recognizing the constraints faced by SMMEs, such as limited scalability, high operational demands, and restricted access to advanced technologies, the review synthesizes existing research to highlight the characteristics, implementations, and impacts of agentic AI in task automation, decision-making, and ecosystem-wide collaboration. The results demonstrate the potential of agentic AI to address technological, ethical, and infrastructure barriers while promoting innovation, scalability, and competitiveness. This review contributes to the understanding of agentic AI frameworks by offering practical insights and setting the groundwork for further research into their applications in SMMEs’ dynamic and resource-constrained economic environments.
Full article
(This article belongs to the Section AI in Autonomous Systems)
►▼
Show Figures
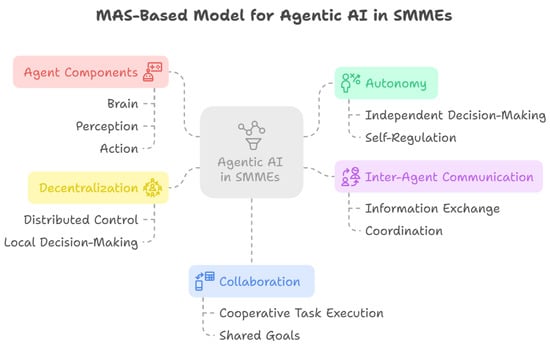
Figure 1
Highly Accessed Articles
Latest Books
E-Mail Alert
News
Topics
Topic in
Applied Sciences, Energies, Buildings, Smart Cities, AI
Smart Electric Energy in Buildings
Topic Editors: Daniel Villanueva Torres, Ali Hainoun, Sergio Gómez MelgarDeadline: 15 July 2025
Topic in
AI, BDCC, Fire, GeoHazards, Remote Sensing
AI for Natural Disasters Detection, Prediction and Modeling
Topic Editors: Moulay A. Akhloufi, Mozhdeh ShahbaziDeadline: 25 July 2025
Topic in
Algorithms, Applied Sciences, Electronics, MAKE, AI, Software
Applications of NLP, AI, and ML in Software Engineering
Topic Editors: Affan Yasin, Javed Ali Khan, Lijie WenDeadline: 31 August 2025
Topic in
AI, Data, Economies, Mathematics, Risks
Advanced Techniques and Modeling in Business and Economics
Topic Editors: José Manuel Santos-Jaén, Ana León-Gomez, María del Carmen Valls MartínezDeadline: 30 September 2025

Conferences
Special Issues
Special Issue in
AI
Artificial Intelligence Challenges to the Industrial Internet of Things and Industrial Control Systems Applications
Guest Editor: Fiammetta MarulliDeadline: 10 July 2025
Special Issue in
AI
AI Bias in the Media and Beyond
Guest Editors: Venetia Papa, Theodoros Kouros, Savvas A. ChatzichristofisDeadline: 31 July 2025
Special Issue in
AI
AI Applications in Emergency Response and Fire Safety
Guest Editors: Yoon J. Ko, Ankit AgrawalDeadline: 31 July 2025
Special Issue in
AI
AI in Finance: Leveraging AI to Transform Financial Services
Guest Editor: Xianrong (Shawn) ZhengDeadline: 31 August 2025