Journal Description
Forecasting
Forecasting
is an international, peer-reviewed, open access journal on all aspects of forecasting published quarterly online by MDPI.
- Open Access— free for readers, with article processing charges (APC) paid by authors or their institutions.
- High Visibility: indexed within Scopus, ESCI (Web of Science), RePEc, and other databases.
- Journal Rank: JCR - Q1 (Multidisciplinary Sciences) / CiteScore - Q1 (Economics, Econometrics and Finance (miscellaneous))
- Rapid Publication: manuscripts are peer-reviewed and a first decision is provided to authors approximately 22.9 days after submission; acceptance to publication is undertaken in 2.7 days (median values for papers published in this journal in the first half of 2025).
- Recognition of Reviewers: reviewers who provide timely, thorough peer-review reports receive vouchers entitling them to a discount on the APC of their next publication in any MDPI journal, in appreciation of the work done.
Impact Factor:
3.2 (2024);
5-Year Impact Factor:
2.9 (2024)
Latest Articles
Optimizing Credit Risk Prediction for Peer-to-Peer Lending Using Machine Learning
Forecasting 2025, 7(3), 35; https://doi.org/10.3390/forecast7030035 - 29 Jun 2025
Abstract
Hyperparameter optimization (HPO) is critical for enhancing the predictive performance of machine learning models in credit risk assessment for peer-to-peer (P2P) lending. This study evaluates four HPO methods, Grid Search, Random Search, Hyperopt, and Optuna, across four models, Logistic Regression, Random Forest, XGBoost,
[...] Read more.
Hyperparameter optimization (HPO) is critical for enhancing the predictive performance of machine learning models in credit risk assessment for peer-to-peer (P2P) lending. This study evaluates four HPO methods, Grid Search, Random Search, Hyperopt, and Optuna, across four models, Logistic Regression, Random Forest, XGBoost, and LightGBM, using three real-world datasets (Lending Club, Australia, Taiwan). We assess predictive accuracy (AUC, Sensitivity, Specificity, G-Mean), computational efficiency, robustness, and interpretability. LightGBM achieves the highest AUC (e.g., on Lending Club, on Australia, on Taiwan), with XGBoost performing comparably. Bayesian methods (Hyperopt, Optuna) match or approach Grid Search’s accuracy while reducing runtime by up to -fold (e.g., vs. min for LightGBM on Lending Club). A sensitivity analysis confirms robust hyperparameter configurations, with AUC variations typically below under perturbations. A feature importance analysis, using gain and SHAP metrics, identifies debt-to-income ratio and employment title as key default predictors, with stable rankings (Spearman correlation ) across tuning methods, enhancing model interpretability. Operational impact depends on data quality, scalable infrastructure, fairness audits for features like employment title, and stakeholder collaboration to ensure compliance with regulations like the EU AI Act and U.S. Equal Credit Opportunity Act. These findings advocate Bayesian HPO and ensemble models in P2P lending, offering scalable, transparent, and fair solutions for default prediction, with future research suggested to explore advanced resampling, cost-sensitive metrics, and feature interactions.
Full article
(This article belongs to the Special Issue Feature Papers of Forecasting 2025)
►
Show Figures
Open AccessArticle
Forecasting Outcomes Using Multi-Option, Advantage-Sensitive Thurstone-Motivated Models
by
László Gyarmati, Csaba Mihálykó and Éva Orbán-Mihálykó
Forecasting 2025, 7(3), 34; https://doi.org/10.3390/forecast7030034 - 26 Jun 2025
Abstract
In this paper, multi-option probabilistic paired comparison models are presented and applied for prediction. As these models operate on the basis of probabilities, they can estimate the likelihood of future outcomes and thus predict future events. The aim of the paper is to
[...] Read more.
In this paper, multi-option probabilistic paired comparison models are presented and applied for prediction. As these models operate on the basis of probabilities, they can estimate the likelihood of future outcomes and thus predict future events. The aim of the paper is to demonstrate that these models have strong predictive capabilities when the information embedded into the data is properly utilized. To this end, we incorporate the degree (e.g., large or small) of the differences between the compared objects. By refining the usual three-option model, we define a five-option model capable of leveraging information derived from the goal differences. To incorporate additional information, the model is further extended to account for potential advantages in the comparisons. As a further refinement, temporal weighting is also introduced. These models are applied to forecasting football match outcomes in the top five European leagues (Premier League, La Liga, Serie A, Bundesliga, and Ligue 1), and their predictive performance is evaluated using various metrics. Based on the most recent football seasons, this model consistently delivers better predictive metrics, on average, than those of the already strong benchmark model. The effect of a home-field advantage is statistically supported across all five leagues. The model fits are illustrated using confidence intervals, and, as an interesting insight, we also present the evolution of the team strengths for the top four English clubs during the 2023/24 season.
Full article
(This article belongs to the Section Forecasting in Economics and Management)
►▼
Show Figures

Figure 1
Open AccessArticle
Machine Learning-Based Prediction of External Pressure in High-Speed Rail Tunnels: Model Optimization and Comparison
by
Xiazhou She, Yongxing Jia, Rui Li, Jianlin Xu, Yonggang Yang, Weiqiang Cao, Lei Xiao and Wenhao Zhao
Forecasting 2025, 7(3), 33; https://doi.org/10.3390/forecast7030033 - 24 Jun 2025
Abstract
►▼
Show Figures
The pressure fluctuations generated during high-speed train passage through tunnels can compromise both the train’s structural integrity and passenger comfort, highlighting the need for the accurate prediction of external pressure wave amplitudes. To address the high computational cost of multi-condition Computational Fluid Dynamics
[...] Read more.
The pressure fluctuations generated during high-speed train passage through tunnels can compromise both the train’s structural integrity and passenger comfort, highlighting the need for the accurate prediction of external pressure wave amplitudes. To address the high computational cost of multi-condition Computational Fluid Dynamics simulations, this study proposes a hybrid method combining numerical simulation and machine learning. A dataset was generated using simulations with five input features: tunnel length, train length, train speed, blockage ratio, and measurement point location. Four machine learning models—random forest, support vector regression, Extreme Gradient Boosting, and Multilayer Perceptron (MLP)—were evaluated, with the MLP model showing the highest baseline accuracy. To further improve performance, six metaheuristic algorithms were applied to optimize the MLP model, among which, the sparrow search algorithm (SSA) achieved the highest accuracy, with R2 = 0.993, MAPE = 0.052, and RMSE = 0.112. A SHapley Additive exPlanations (SHAP) analysis indicated that the train speed and the blockage ratio were the most influential features. This study provides an effective and interpretable method for pressure wave prediction in tunnel environments and demonstrates the first integration of SSA optimization into aerodynamic pressure modeling.
Full article
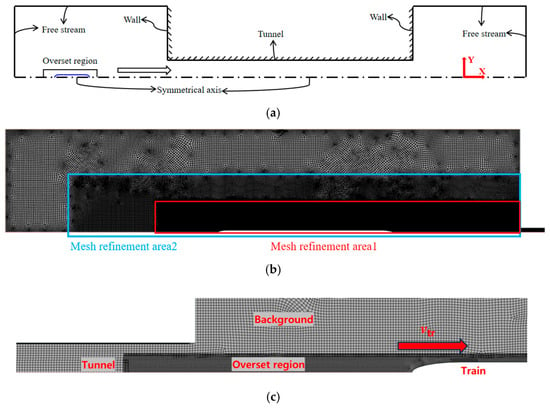
Figure 1
Open AccessArticle
Sensitivity Analysis of Priors in the Bayesian Dirichlet Auto-Regressive Moving Average Model
by
Harrison Katz, Liz Medina and Robert E. Weiss
Forecasting 2025, 7(3), 32; https://doi.org/10.3390/forecast7030032 - 20 Jun 2025
Abstract
We examine how prior specification affects the Bayesian Dirichlet Auto-Regressive Moving Average (B-DARMA) model for compositional time series. Through three simulation scenarios—correct specification, overfitting, and underfitting—we compare five priors: informative, horseshoe, Laplace, mixture of normals, and hierarchical. Under correct model specification, all priors
[...] Read more.
We examine how prior specification affects the Bayesian Dirichlet Auto-Regressive Moving Average (B-DARMA) model for compositional time series. Through three simulation scenarios—correct specification, overfitting, and underfitting—we compare five priors: informative, horseshoe, Laplace, mixture of normals, and hierarchical. Under correct model specification, all priors perform similarly, although the horseshoe and hierarchical priors produce slightly lower bias. When the model overfits, strong shrinkage—particularly from the horseshoe prior—proves advantageous. However, none of the priors can compensate for model misspecification if key VAR/VMA terms are omitted. We apply B-DARMA to daily S&P 500 sector trading data, using a large-lag model to demonstrate overparameterization risks. Shrinkage priors effectively mitigate spurious complexity, whereas weakly informative priors inflate errors in volatile sectors. These findings highlight the critical role of carefully selecting priors and managing model complexity in compositional time-series analysis, particularly in high-dimensional settings.
Full article
(This article belongs to the Section Forecasting in Economics and Management)
►▼
Show Figures
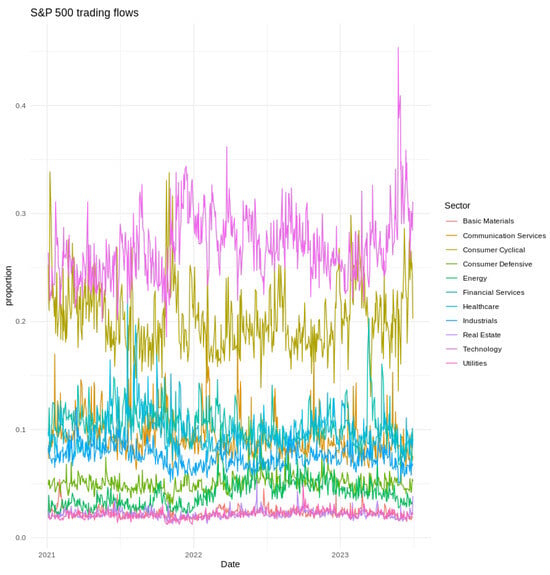
Figure 1
Open AccessArticle
A Unified Transformer–BDI Architecture for Financial Fraud Detection: Distributed Knowledge Transfer Across Diverse Datasets
by
Parul Dubey, Pushkar Dubey and Pitshou N. Bokoro
Forecasting 2025, 7(2), 31; https://doi.org/10.3390/forecast7020031 - 19 Jun 2025
Abstract
►▼
Show Figures
Financial fraud detection is a critical application area within the broader domains of cybersecurity and intelligent financial analytics. With the growing volume and complexity of digital transactions, the traditional rule-based and shallow learning models often fall short in detecting sophisticated fraud patterns. This
[...] Read more.
Financial fraud detection is a critical application area within the broader domains of cybersecurity and intelligent financial analytics. With the growing volume and complexity of digital transactions, the traditional rule-based and shallow learning models often fall short in detecting sophisticated fraud patterns. This study addresses the challenge of accurately identifying fraudulent financial activities, especially in highly imbalanced datasets where fraud instances are rare and often masked by legitimate behavior. The existing models also lack interpretability, limiting their utility in regulated financial environments. Experiments were conducted on three benchmark datasets: IEEE-CIS Fraud Detection, European Credit Card Transactions, and PaySim Mobile Money Simulation, each representing diverse transaction behaviors and data distributions. The proposed methodology integrates a transformer-based encoder, multi-teacher knowledge distillation, and a symbolic belief–desire–intention (BDI) reasoning layer to combine deep feature extraction with interpretable decision making. The novelty of this work lies in the incorporation of cognitive symbolic reasoning into a high-performance learning architecture for fraud detection. The performance was assessed using key metrics, including the F1-score, AUC, precision, recall, inference time, and model size. Results show that the proposed transformer–BDI model outperformed traditional and state-of-the-art baselines across all datasets, achieving improved fraud detection accuracy and interpretability while remaining computationally efficient for real-time deployment.
Full article
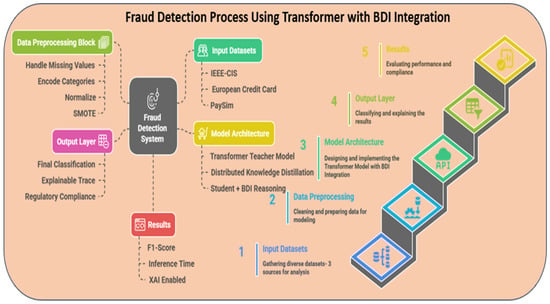
Figure 1
Open AccessArticle
A Set of New Tools to Measure the Effective Value of Probabilistic Forecasts of Continuous Variables
by
Josselin Le Gal La Salle, Mathieu David and Philippe Lauret
Forecasting 2025, 7(2), 30; https://doi.org/10.3390/forecast7020030 - 19 Jun 2025
Abstract
►▼
Show Figures
In recent years, the prominence of probabilistic forecasting has risen among numerous research fields (finance, meteorology, banking, etc.). Best practices on using such forecasts are, however, neither well explained nor well understood. The question of the benefits derived from these forecasts is of
[...] Read more.
In recent years, the prominence of probabilistic forecasting has risen among numerous research fields (finance, meteorology, banking, etc.). Best practices on using such forecasts are, however, neither well explained nor well understood. The question of the benefits derived from these forecasts is of primary interest, especially for the industrial sector. A sound methodology already exists to evaluate the value of probabilistic forecasts of binary events. In this paper, we introduce a comprehensive methodology for assessing the value of probabilistic forecasts of continuous variables, which is valid for a specific class of problems where the cost functions are piecewise linear. The proposed methodology is based on a set of visual diagnostic tools. In particular, we propose a new diagram called EVC (“Effective economic Value of a forecast of Continuous variable”) which provides the effective value of a forecast. Using simple case studies, we show that the value of probabilistic forecasts of continuous variables is strongly dependent on a key variable that we call the risk ratio. It leads to a quantitative metric of a value called the OEV (“Overall Effective Value”). The preliminary results suggest that typical OEVs demonstrate the benefits of probabilistic forecasting over a deterministic approach.
Full article
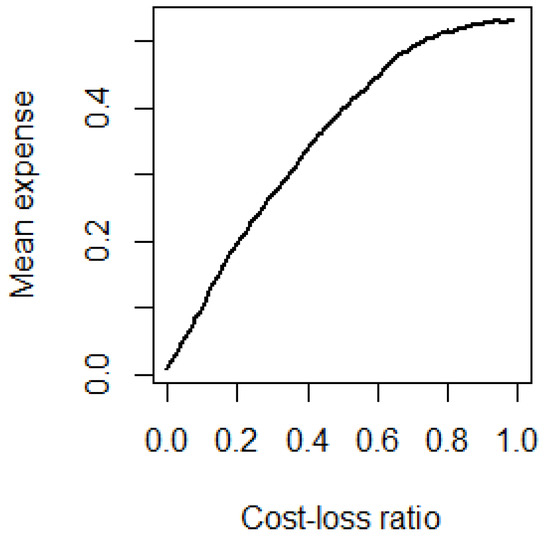
Figure 1
Open AccessArticle
A Fusion of Deep Learning and Time Series Regression for Flood Forecasting: An Application to the Ratnapura Area Based on the Kalu River Basin in Sri Lanka
by
Shanthi Saubhagya, Chandima Tilakaratne, Pemantha Lakraj and Musa Mammadov
Forecasting 2025, 7(2), 29; https://doi.org/10.3390/forecast7020029 - 18 Jun 2025
Abstract
Flooding is the most frequent natural hazard that accompanies hardships for millions of civilians and substantial economic losses. In Sri Lanka, fluvial floods cause the highest damage to lives and properties. Ratnapura, which is in the Kalu River Basin, is the area most
[...] Read more.
Flooding is the most frequent natural hazard that accompanies hardships for millions of civilians and substantial economic losses. In Sri Lanka, fluvial floods cause the highest damage to lives and properties. Ratnapura, which is in the Kalu River Basin, is the area most vulnerable to frequent flood events in Sri Lanka due to inherent weather patterns and its geographical location. However, flood-related studies conducted based on the Kalu River Basin and its most vulnerable cities are given minimal attention by researchers. Therefore, it is crucial to develop a robust and reliable dynamic flood forecasting system to issue accurate and timely early flood warnings to vulnerable victims. Modeling the water level at the initial stage and then classifying the results of this into pre-defined flood risk levels facilitates more accurate forecasts for upcoming susceptibilities, since direct flood classification often produces less accurate predictions due to the heavily imbalanced nature of the data. Thus, this study introduces a novel hybrid model that combines a deep leaning technique with a traditional Linear Regression model to first forecast water levels and then detect rare but destructive flood events (i.e., major and critical floods) with high accuracy, from 1 to 3 days ahead. Initially, the water level of the Kalu River at Ratnapura was forecasted 1 to 3 days ahead by employing a Vanilla Bi-LSTM model. Similarly to water level modeling, rainfall at the same location was forecasted 1 to 3 days ahead by applying another Bi-LSTM model. To further improve the forecasting accuracy of the water level, the forecasted water level at day t was combined with the forecasted rainfall for the same day by applying a Time Series Regression model, thereby resulting in a hybrid model. This improvement is imperative mainly because the water level forecasts obtained for a longer lead time may change with the real-time appearance of heavy rainfall. Nevertheless, this important phenomenon has often been neglected in past studies related to modeling water levels. The performances of the models were compared by examining their ability to accurately forecast flood risks, especially at critical levels. The combined model with Bi-LSTM and Time Series Regression outperformed the single Vanilla Bi-LSTM model by forecasting actionable flood events (minor and critical) occurring in the testing period with accuracies of 80%, 80%, and 100% for 1- to 3-day-ahead forecasting, respectively. Moreover, overall, the results evidenced lower RMSE and MAE values (<0.4 m MSL) for three-days-ahead water level forecasts. Therefore, this enhanced approach enables more trustworthy, impact-based flood forecasting for the Rathnapura area in the Kalu River Basin. The same modeling approach could be applied to obtain flood risk levels caused by rivers across the globe.
Full article
(This article belongs to the Section Environmental Forecasting)
►▼
Show Figures
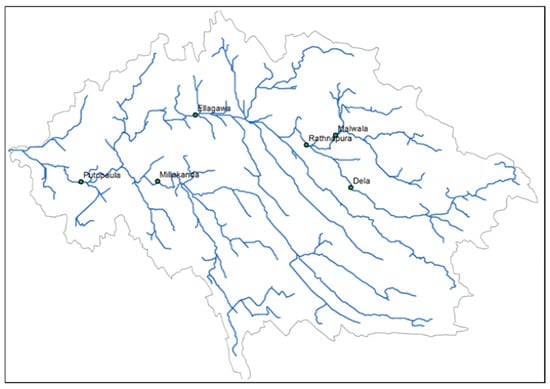
Figure 1
Open AccessArticle
Prediction of Airtightness Performance of Stratospheric Ships Based on Multivariate Environmental Time-Series Data
by
Yitong Bi, Wenkuan Xu, Lin Song, Molan Yang and Xiangqiang Zhang
Forecasting 2025, 7(2), 28; https://doi.org/10.3390/forecast7020028 - 12 Jun 2025
Abstract
►▼
Show Figures
This study addresses the challenge of predicting the airtightness of stratospheric airship envelopes, a critical factor influencing flight performance. Traditional ground-based airtightness tests often rely on limited resources and empirical formulas. To overcome these limitations, this paper explores the use of predictive models
[...] Read more.
This study addresses the challenge of predicting the airtightness of stratospheric airship envelopes, a critical factor influencing flight performance. Traditional ground-based airtightness tests often rely on limited resources and empirical formulas. To overcome these limitations, this paper explores the use of predictive models to integrate multi-source test data, enhancing the accuracy of airtightness assessments. A performance comparison of various prediction models was conducted using ground-based test data from a specific stratospheric airship. Among the models evaluated, the NeuralProphet model demonstrated superior accuracy in long-term airtightness predictions, effectively capturing time-series dependencies and spatial interactions with environmental conditions. This work introduces an innovative approach to modeling airtightness, providing both experimental and theoretical contributions to the field of stratospheric airship performance prediction.
Full article
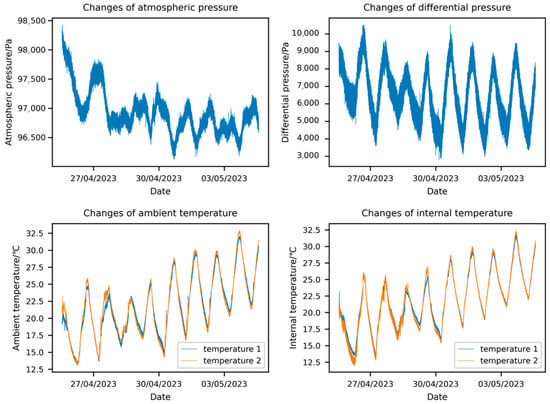
Figure 1
Open AccessArticle
Wind Speed Forecasting with Differentially Evolved Minimum-Bandwidth Filters and Gated Recurrent Units
by
Khathutshelo Steven Sivhugwana and Edmore Ranganai
Forecasting 2025, 7(2), 27; https://doi.org/10.3390/forecast7020027 - 10 Jun 2025
Abstract
Wind data are often cyclostationary due to cyclic variations, non-constant variance resulting from fluctuating weather conditions, and structural breaks due to transient behaviour (due to wind gusts and turbulence), resulting in unreliable wind power supply. In wavelet hybrid forecasting, wind prediction accuracy depends
[...] Read more.
Wind data are often cyclostationary due to cyclic variations, non-constant variance resulting from fluctuating weather conditions, and structural breaks due to transient behaviour (due to wind gusts and turbulence), resulting in unreliable wind power supply. In wavelet hybrid forecasting, wind prediction accuracy depends heavily on the decomposition level ( ) and the wavelet filter technique selected. Hence, we examined the efficacy of wind predictions as a function of and wavelet filters. In the proposed hybrid approach, differential evolution (DE) optimises the decomposition level of various wavelet filters (i.e., least asymmetric (LA), Daubechies (DB), and Morris minimum-bandwidth (MB)) using the maximal overlap discrete wavelet transform (MODWT), allowing for the decomposition of wind data into more statistically sound sub-signals. These sub-signals are used as inputs into the gated recurrent unit (GRU) to accurately capture wind speed. The final predicted values are obtained by reconciling the sub-signal predictions using multiresolution analysis (MRA) to form wavelet-MODWT-GRUs. Using wind data from three Wind Atlas South Africa (WASA) locations, Alexander Bay, Humansdorp, and Jozini, the root mean square error, mean absolute error, coefficient of determination, probability integral transform, pinball loss, and Dawid-Sebastiani showed that the MB-MODWT-GRU at was best across the three locations.
Full article
(This article belongs to the Special Issue Feature Papers of Forecasting 2025)
►▼
Show Figures
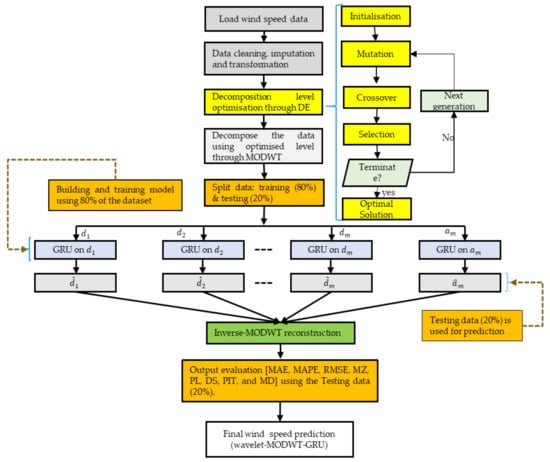
Figure 1
Open AccessArticle
Identifying and Forecasting Recurrently Emerging Stock Trend Structures via Rising Visibility Graphs
by
Zhen Zeng and Yu Chen
Forecasting 2025, 7(2), 26; https://doi.org/10.3390/forecast7020026 - 9 Jun 2025
Abstract
This study introduces a novel forecasting framework that identifies and predicts recurrently emerging structural patterns in stock trends using rising visibility graphs (RVGs) and the Weisfeiler–Lehman (WL) subtree kernel. The proposed method, RVGWL, addresses a key limitation of traditional visibility graphs, namely the
[...] Read more.
This study introduces a novel forecasting framework that identifies and predicts recurrently emerging structural patterns in stock trends using rising visibility graphs (RVGs) and the Weisfeiler–Lehman (WL) subtree kernel. The proposed method, RVGWL, addresses a key limitation of traditional visibility graphs, namely the structural indistinguishability between rising and falling trends, by selectively constructing edges only along upward price movements. This approach produces graph representations that capture direction-sensitive market dynamics and facilitate the extraction of meaningful topological features from price data. By applying the WL kernel, RVGWL quantifies structural similarities between graph-transformed time series, enabling the identification of structurally similar preceding patterns and the probabilistic forecasting of their subsequent trajectories based on nine canonical trend templates. Experiments on time series data from four major stock indices and their constituent stocks during the year 2023—characterized by diverse market regimes across the U.S., Japan, the U.K., and China—demonstrate that RVGWL consistently outperforms classical rule-based strategies. These results support the predictive value of recurring topological structures in financial time series and higight the potential of structure-aware forecasting methods in quantitative analysis.
Full article
(This article belongs to the Section Forecasting in Economics and Management)
►▼
Show Figures
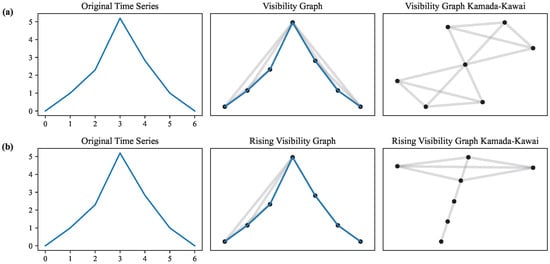
Figure 1
Open AccessArticle
Parallel Multi-Model Energy Demand Forecasting with Cloud Redundancy: Leveraging Trend Correction, Feature Selection, and Machine Learning
by
Kamran Hassanpouri Baesmat, Zeinab Farrokhi, Grzegorz Chmaj and Emma E. Regentova
Forecasting 2025, 7(2), 25; https://doi.org/10.3390/forecast7020025 - 31 May 2025
Abstract
In this work, we present a novel approach for predicting short-term electrical energy consumption. Most energy consumption methods work well for their case study datasets. The proposed method utilizes a cloud computing platform that allows for integrating information from different sources, such as
[...] Read more.
In this work, we present a novel approach for predicting short-term electrical energy consumption. Most energy consumption methods work well for their case study datasets. The proposed method utilizes a cloud computing platform that allows for integrating information from different sources, such as weather data and historical energy consumption, while employing machine learning techniques to achieve higher accuracy in forecasting. We collected detailed weather data from the “Weather Underground Company” website, known for its accurate records. Then, we studied past energy consumption data provided by PJM (focusing on DEO&K, which serves Cincinnati and northern Kentucky) and identified features that significantly impact energy consumption. We also introduced a processing step to ensure accurate predictions for holidays. Our goal is to predict the next 24 h of load consumption. We developed a hybrid, generalizable forecasting methodology with deviation correction. The methodology is characterized by fault tolerance due to distributed cloud deployment and an introduced voting mechanism. The proposed approach improved the accuracy of LSTM, SARIMAX, and SARIMAX + SVM, with MAPE values of 5.17%, 4.21%, and 2.21% reduced to 1.65%, 1.00%, and 0.88%, respectively, using our CM-LSTM-DC, CM-SARIMAX-DC, and CM-SARIMAX + SVM-DC models.
Full article
(This article belongs to the Section Power and Energy Forecasting)
►▼
Show Figures
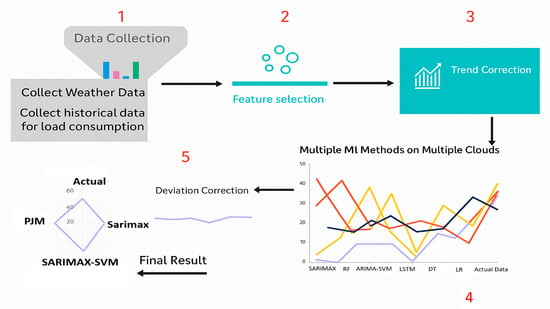
Figure 1
Open AccessArticle
Three Environments, One Problem: Forecasting Water Temperature in Central Europe in Response to Climate Change
by
Mariusz Ptak, Mariusz Sojka, Katarzyna Szyga-Pluta and Teerachai Amnuaylojaroen
Forecasting 2025, 7(2), 24; https://doi.org/10.3390/forecast7020024 - 29 May 2025
Abstract
Water temperature is a fundamental parameter influencing a range of biotic and abiotic processes occurring within various components of the hydrosphere. This study presents a multi-step, data-driven predictive modeling framework to estimate water temperatures for the period 2021–2100 in three aquatic environments in
[...] Read more.
Water temperature is a fundamental parameter influencing a range of biotic and abiotic processes occurring within various components of the hydrosphere. This study presents a multi-step, data-driven predictive modeling framework to estimate water temperatures for the period 2021–2100 in three aquatic environments in Central Europe: the Odra River, the Szczecin Lagoon, and the Baltic Sea. The framework integrates Bayesian Model Averaging (BMA), Random Sample Consensus (RANSAC) regression, Gradient Boosting Regressor (GBR), and Random Forest (RF) machine learning models. To assess the performance of the models, the coefficient of determination (R2), mean absolute error (MAE), and root mean square error (RMSE) were used. The results showed that the application of statistical downscaling methods improved the prediction of air temperatures with respect to the BMA. Moreover, the RF method was used to predict water temperature. The best model performance was obtained for the Baltic Sea and the lowest for the Odra River. Under the SSP2-4.5 and SSP5-8.5 scenario-based simulations, projected air temperature increases in the period 2021–2100 could range from 1.5 °C to 1.7 °C and 4.7 to 5.1 °C. In contrast, the increase in water temperatures by 2100 will be between 1.2 °C and 1.6 °C (SSP2-4.5 scenario) and between 3.5 °C and 4.9 °C (SSP5-8.5).
Full article
(This article belongs to the Section Weather and Forecasting)
►▼
Show Figures
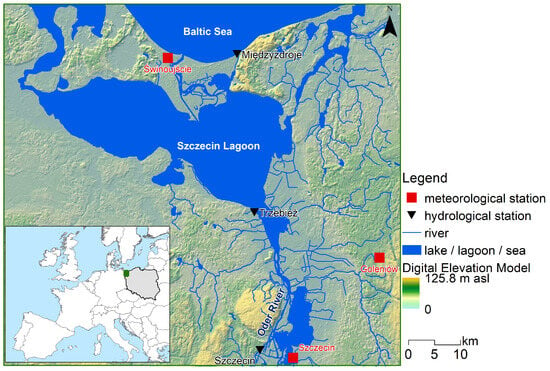
Figure 1
Open AccessArticle
Dynamic Forecasting of Gas Consumption in Selected European Countries
by
Mariangela Guidolin and Stefano Rizzelli
Forecasting 2025, 7(2), 23; https://doi.org/10.3390/forecast7020023 - 26 May 2025
Abstract
Natural gas consumption in Europe has undergone substantial changes in recent years, driven by geopolitical tensions, economic dynamics, and the continent’s ongoing transition towards cleaner energy sources. Furthermore, as noted in the International Energy Agency’s Gas Market Report 2025, natural gas demand is
[...] Read more.
Natural gas consumption in Europe has undergone substantial changes in recent years, driven by geopolitical tensions, economic dynamics, and the continent’s ongoing transition towards cleaner energy sources. Furthermore, as noted in the International Energy Agency’s Gas Market Report 2025, natural gas demand is becoming increasingly sensitive to fluctuations in weather patterns, including cold snaps and heatwaves. These factors make the task of forecasting future annual consumption particularly challenging from a statistical perspective and underscore the importance of accurately quantifying the uncertainty surrounding predictions. In this paper, we propose a simple yet flexible approach to issuing dynamic probabilistic forecasts based on an additive time series model. To capture long-term trends, the model incorporates a deterministic component based on the Guseo–Guidolin innovation diffusion framework. In addition, a stochastic innovation term governed by an ARIMAX process is used to describe year-over-year fluctuations, helping to account for the potential presence of variance nonstationarity over time. The proposed methodology is applied to forecast future annual consumption in six key European countries: Austria, France, Germany, Italy, the Netherlands, and the United Kingdom.
Full article
(This article belongs to the Section Power and Energy Forecasting)
►▼
Show Figures
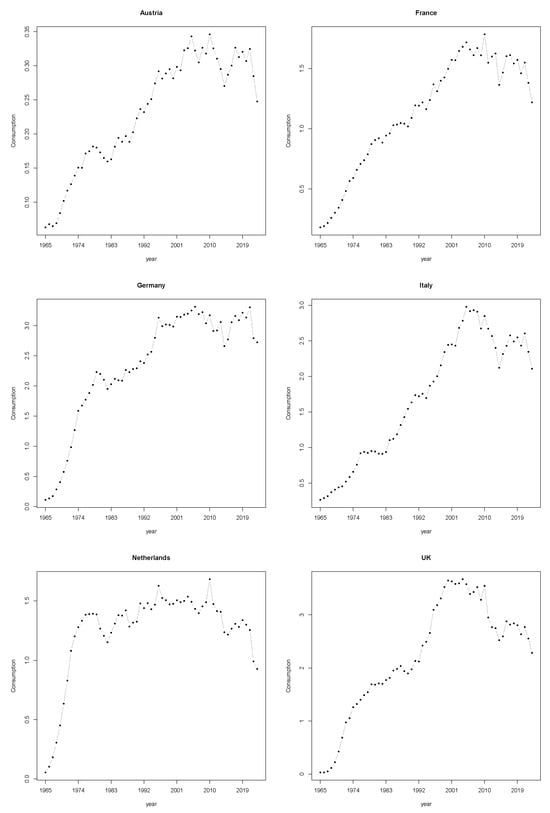
Figure 1
Open AccessArticle
Forecasting Robust Gaussian Process State Space Models for Assessing Intervention Impact in Internet of Things Time Series
by
Patrick Toman, Nalini Ravishanker, Nathan Lally and Sanguthevar Rajasekaran
Forecasting 2025, 7(2), 22; https://doi.org/10.3390/forecast7020022 - 26 May 2025
Abstract
This article describes a robust Gaussian Prior process state space modeling (GPSSM) approach to assess the impact of an intervention in a time series. Numerous applications can benefit from this approach. Examples include: (1) time series could be the stock price of a
[...] Read more.
This article describes a robust Gaussian Prior process state space modeling (GPSSM) approach to assess the impact of an intervention in a time series. Numerous applications can benefit from this approach. Examples include: (1) time series could be the stock price of a company and the intervention could be the acquisition of another company; (2) the time series under concern could be the noise coming out of an engine, and the intervention could be a corrective step taken to reduce the noise; (3) the time series could be the number of visits to a web service, and the intervention is changes done to the user interface; and so on. The approach we describe in this article applies to any times series and intervention combination. It is well known that Gaussian process (GP) prior models provide flexibility by placing a non-parametric prior on the functional form of the model. While GPSSMs enable us to model a time series in a state space framework by placing a Gaussian Process (GP) prior over the state transition function, probabilistic recurrent state space models (PRSSM) employ variational approximations for handling complicated posterior distributions in GPSSMs. The robust PRSSMs (R-PRSSMs) discussed in this article assume a scale mixture of normal distributions instead of the usually proposed normal distribution. This assumption will accommodate heavy-tailed behavior or anomalous observations in the time series. On any exogenous intervention, we use R-PRSSM for Bayesian fitting and forecasting of the IoT time series. By comparing forecasts with the future internal temperature observations, we can assess with a high level of confidence the impact of an intervention. The techniques presented in this paper are very generic and apply to any time series and intervention combination. To illustrate our techniques clearly, we employ a concrete example. The time series of interest will be an Internet of Things (IoT) stream of internal temperatures measured by an insurance firm to address the risk of pipe-freeze hazard in a building. We treat the pipe-freeze hazard alert as an exogenous intervention. A comparison of forecasts and the future observed temperatures will be utilized to assess whether an alerted customer took preventive action to prevent pipe-freeze loss.
Full article
(This article belongs to the Section Forecasting in Computer Science)
►▼
Show Figures
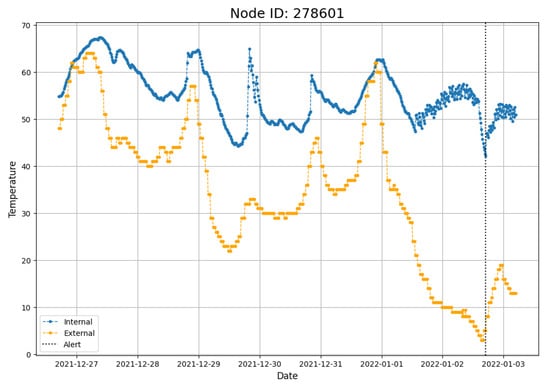
Figure 1
Open AccessArticle
Cognitive and Spatial Forecasting Model for Maritime Migratory Incidents: SIFM
by
Donatien Agbissoh Otote and Antonio Vázquez Hoehne
Forecasting 2025, 7(2), 21; https://doi.org/10.3390/forecast7020021 - 20 May 2025
Abstract
►▼
Show Figures
The security challenges associated with maritime migratory incidents in the Mediterranean Sea since the onset of the 21st century are considerable. Reports of such incidents are generated almost daily, leading to significant scientific interest, including that of this manuscript. This article introduces a
[...] Read more.
The security challenges associated with maritime migratory incidents in the Mediterranean Sea since the onset of the 21st century are considerable. Reports of such incidents are generated almost daily, leading to significant scientific interest, including that of this manuscript. This article introduces a forecasting model specifically designed to equip maritime security stakeholders around the Mediterranean Sea with a technical instrument for estimating the frequency of maritime migratory incidents. The proposed model, the SIFM, encompasses five methodological steps: Tessellation: The initial step involves partitioning the maritime area affected by these incidents into distinct cells. Subsidiary process: In this phase, the cells are classified according to the year in which incidents were recorded. Containment index: This index quantifies the magnitude of incidents within the designated cells. Incidence growth index: This metric further refines the forecasting methodology. Maritime migration incident forecasting: The concluding step establishes a forecast interval for the anticipated quantity of maritime migratory incidents. This systematic approach aims to enhance the understanding and prediction of maritime migratory incidents within the Mediterranean region.
Full article
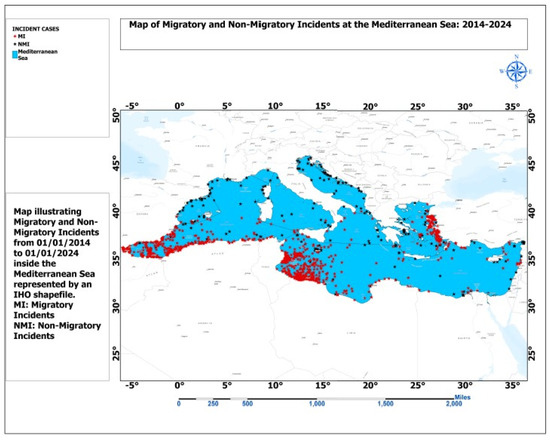
Figure 1
Open AccessArticle
Stochastic Model and Rhythm-Adaptive Technologies of Statistical Analysis and Forecasting of Economic Processes with Cyclic Components
by
Serhii Lupenko and Andrii Horkunenko
Forecasting 2025, 7(2), 20; https://doi.org/10.3390/forecast7020020 - 19 May 2025
Abstract
This article presents a mathematical model of cyclical economic processes, formulated as the sum of a deterministic polynomial function and a cyclic random process that simultaneously captures trend, stochasticity, cyclicity, and rhythm variability. Building on this stochastic framework, we propose rhythm-adaptive statistical techniques
[...] Read more.
This article presents a mathematical model of cyclical economic processes, formulated as the sum of a deterministic polynomial function and a cyclic random process that simultaneously captures trend, stochasticity, cyclicity, and rhythm variability. Building on this stochastic framework, we propose rhythm-adaptive statistical techniques for estimating the probabilistic characteristics of the cyclic component; by adjusting to rhythm changes, these techniques improve estimation accuracy. We also introduce a forecasting procedure that constructs a system of rhythm-adaptive confidence intervals for future cycles. The effectiveness of the model and associated methods is demonstrated through a series of computational experiments using Federal Reserve Economic Data. Results show that the rhythm-adaptive forecasting approach achieves mean absolute errors less than half of those produced by a comparable non-adaptive method, underscoring its practical advantage for the analysis and prediction of cyclic economic phenomena.
Full article
(This article belongs to the Section Forecasting in Economics and Management)
►▼
Show Figures
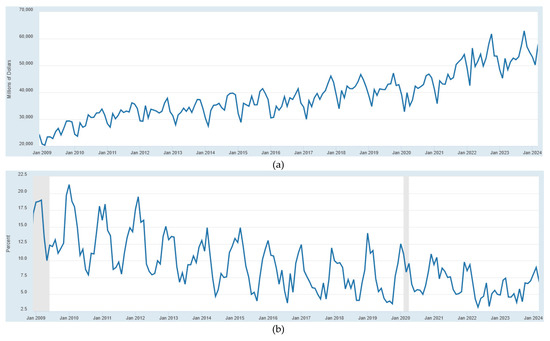
Figure 1
Open AccessArticle
A Deep Learning-Based Prediction and Forecasting of Tomato Prices for the Cape Town Fresh Produce Market: A Model Comparative Analysis
by
Emmanuel Ekene Okere and Vipin Balyan
Forecasting 2025, 7(2), 19; https://doi.org/10.3390/forecast7020019 - 13 May 2025
Abstract
The fresh produce supply chain sector is a vital pillar of any society and an indispensable part of the national economic structure. As a significant segment of the agricultural market, accurately forecasting vegetable prices holds significant importance. Vegetable market pricing is subject to
[...] Read more.
The fresh produce supply chain sector is a vital pillar of any society and an indispensable part of the national economic structure. As a significant segment of the agricultural market, accurately forecasting vegetable prices holds significant importance. Vegetable market pricing is subject to a myriad of complex influences, resulting in nonlinear patterns that conventional time series methodologies often struggle to decode. Future planning for commodity pricing is achievable by forecasting the future price anticipated by the current circumstances. This paper presents a price forecasting methodology for tomatoes which uses price and production data taken from 2008 to 2021 and analyzed by means of advanced deep learning-based Long Short-Term Memory (LSTM) networks. A comparative analysis of three models based on Root Mean Square Error (RMSE) identifies LSTM as the most accurate model, achieving the lowest RMSE (0.2818), while SARIMA performs relatively well. The proposed deep learning-based method significantly improved the results versus other conventional machine learning and statistical time series analysis methods.
Full article
(This article belongs to the Section Forecasting in Economics and Management)
►▼
Show Figures
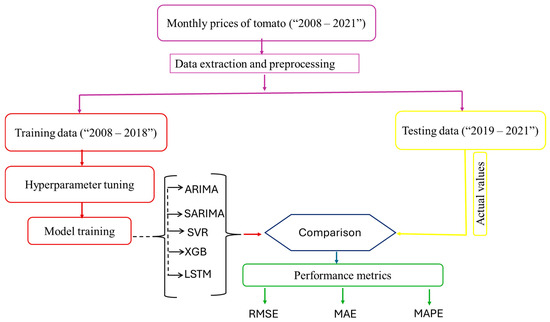
Figure 1
Open AccessArticle
Day-Ahead Energy Price Forecasting with Machine Learning: Role of Endogenous Predictors
by
Chibuike Chiedozie Ibebuchi
Forecasting 2025, 7(2), 18; https://doi.org/10.3390/forecast7020018 - 9 Apr 2025
Cited by 1
Abstract
Accurate Day-Ahead Energy Price (DAEP) forecasting is essential for optimizing energy market operations. This study introduces a machine learning framework to predict the DAEP with a 24 h lead time, leveraging historical data and forecasts available at the prediction time. Hourly DAEP data
[...] Read more.
Accurate Day-Ahead Energy Price (DAEP) forecasting is essential for optimizing energy market operations. This study introduces a machine learning framework to predict the DAEP with a 24 h lead time, leveraging historical data and forecasts available at the prediction time. Hourly DAEP data from the California Independent System Operator (January 2017 to July 2023) were integrated with exogenous and engineered endogenous features. A custom rolling window cross-validation, with 24 h validation blocks sliding daily across 2372 folds, evaluates an Extreme Gradient Boosting (XGBoost) model’s performance under diverse market conditions, achieving a median mean absolute error of 6.26 USD/MWh and root mean squared error of 8.27 USD/MWh, with variability reflecting market volatility. The feature importance analysis using Shapley additive explanations highlighted the dominance of engineered endogenous features in driving the 24 h lead time forecasts under relatively stable market conditions. Forecasting the DAEP at a runtime of 10 AM on the prior day was used to assess model uncertainty. This involved training random forest, support vector regression, XGBoost, and feed forward neural network models, followed by stacking and voting ensembles. The results indicate the need for ensemble forecasting and evaluation beyond a static train–test split to ensure the practical utility of machine learning for DAEP forecasting across varied market dynamics. Finally, operationalizing the forecast model for bidding decisions by forecasting the DAEP and real-time prices at runtime is presented and discussed.
Full article
(This article belongs to the Special Issue New Challenges in Energy and Finance Forecasting in the Era of Big Data)
►▼
Show Figures
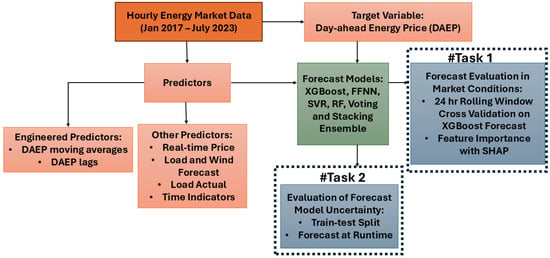
Figure 1
Open AccessCorrection
Correction: Ferreira Lima dos Santos et al. Riding into Danger: Predictive Modeling for ATV-Related Injuries and Seasonal Patterns. Forecasting 2024, 6, 266–278
by
Fernando Ferreira Lima dos Santos, Farzaneh Khorsandi and Guilherme De Moura Araujo
Forecasting 2025, 7(2), 17; https://doi.org/10.3390/forecast7020017 - 7 Apr 2025
Abstract
Addition of an Author [...]
Full article
Open AccessArticle
Volatility Modelling of the Johannesburg Stock Exchange All Share Index Using the Family GARCH Model
by
Israel Maingo, Thakhani Ravele and Caston Sigauke
Forecasting 2025, 7(2), 16; https://doi.org/10.3390/forecast7020016 - 3 Apr 2025
Abstract
In numerous domains of finance and economics, modelling and predicting stock market volatility is essential. Predicting stock market volatility is widely used in the management of portfolios, analysis of risk, and determination of option prices. This study is about volatility modelling of the
[...] Read more.
In numerous domains of finance and economics, modelling and predicting stock market volatility is essential. Predicting stock market volatility is widely used in the management of portfolios, analysis of risk, and determination of option prices. This study is about volatility modelling of the daily Johannesburg Stock Exchange All Share Index (JSE ALSI) stock price data between 1 January 2014 and 29 December 2023. The modelling process incorporated daily log returns derived from the JSE ALSI. The following volatility models were presented for the period: sGARCH(1, 1) and fGARCH(1, 1). The models for volatility were fitted using five unique error distribution assumptions, including Student’s t, its skewed version, the generalized error and skewed generalized error distributions, and the generalized hyperbolic distribution. Based on information criteria such as Akaike, Bayesian, and Hannan–Quinn, the ARMA(0, 0)-fGARCH(1, 1) model with a skewed generalized error distribution emerged as the best fit. The chosen model revealed that the JSE ALSI prices are highly persistent with the leverage effect. JSE ALSI price volatility was notably influenced during the COVID-19 pandemic. The forecast over the next 10 days shows a rise in volatility. A comparative study was then carried out with the JSE Top 40 and the S&P500 indices. Comparison of the FTSE/JSE Top 40, S&P 500, and JSE ALLSI return indices over the COVID-19 pandemic indicated higher initial volatility in the FTSE/JSE Top 40 and S&P 500, with the JSE ALLSI following a similar trend later. The S&P 500 showed long-term reliability and high rolling returns in spite of short-run volatility, the FTSE/JSE Top 40 showed more pre-pandemic risk and volatility but reduced levels of rolling volatility after the pandemic, similar in magnitude for each index with low correlations among them. These results provide important insights for risk managers and investors navigating the South African equity market.
Full article
(This article belongs to the Section Forecasting in Economics and Management)
►▼
Show Figures
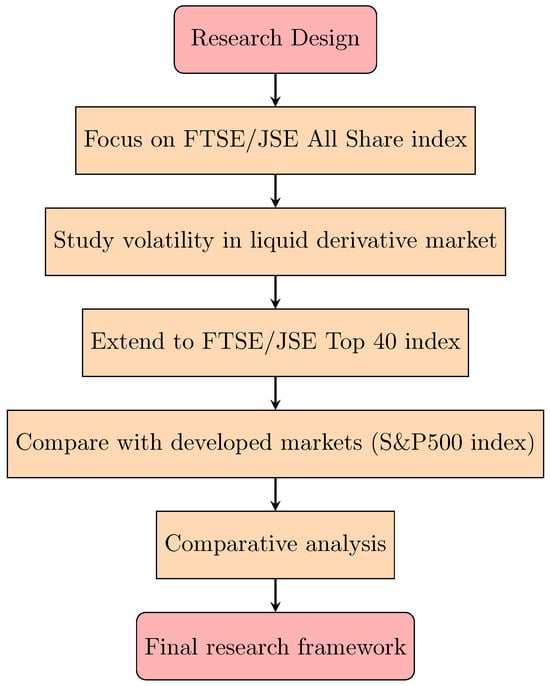
Figure 1
Highly Accessed Articles
Latest Books
E-Mail Alert
News
Topics
Topic in
Electricity, Energies, Forecasting, Processes, Smart Cities, Sustainability
Intelligent, Flexible, and Effective Operation of Smart Grids with Novel Energy Technologies and Equipment
Topic Editors: Pengfei Zhao, Sheng Chen, Yunqi Wang, Liwei Ju, Zhengmao Li, Minglei BaoDeadline: 31 July 2025
Topic in
Economies, Energies, Environments, Forecasting, Laws
Energy Policy, Regulation and Sustainable Development—2nd Edition
Topic Editors: Grzegorz Mentel, Mirela Panait, Xin ZhaoDeadline: 31 December 2025
Topic in
Applied Sciences, Energies, Forecasting, Solar, Wind, Batteries
Solar and Wind Power and Energy Forecasting, 2nd Edition
Topic Editors: Emanuele Ogliari, Alessandro Niccolai, Sonia LevaDeadline: 31 July 2026

Conferences
Special Issues
Special Issue in
Forecasting
Renewable Energy Forecasting: Innovations and Breakthroughs
Guest Editors: Grzegorz Mentel, Xin ZhaoDeadline: 30 December 2025
Special Issue in
Forecasting
Feature Papers of Forecasting 2025
Guest Editor: Sonia LevaDeadline: 31 December 2025
Topical Collections
Topical Collection in
Forecasting
Supply Chain Management Forecasting
Collection Editors: Gokhan Egilmez, Juan Ramón Trapero Arenas
Topical Collection in
Forecasting
Near-Term Ecological Forecasting
Collection Editor: Michael Dietze