Topic Menu
► Topic MenuTopic Editors


Electric Vehicles Energy Management, 2nd Volume
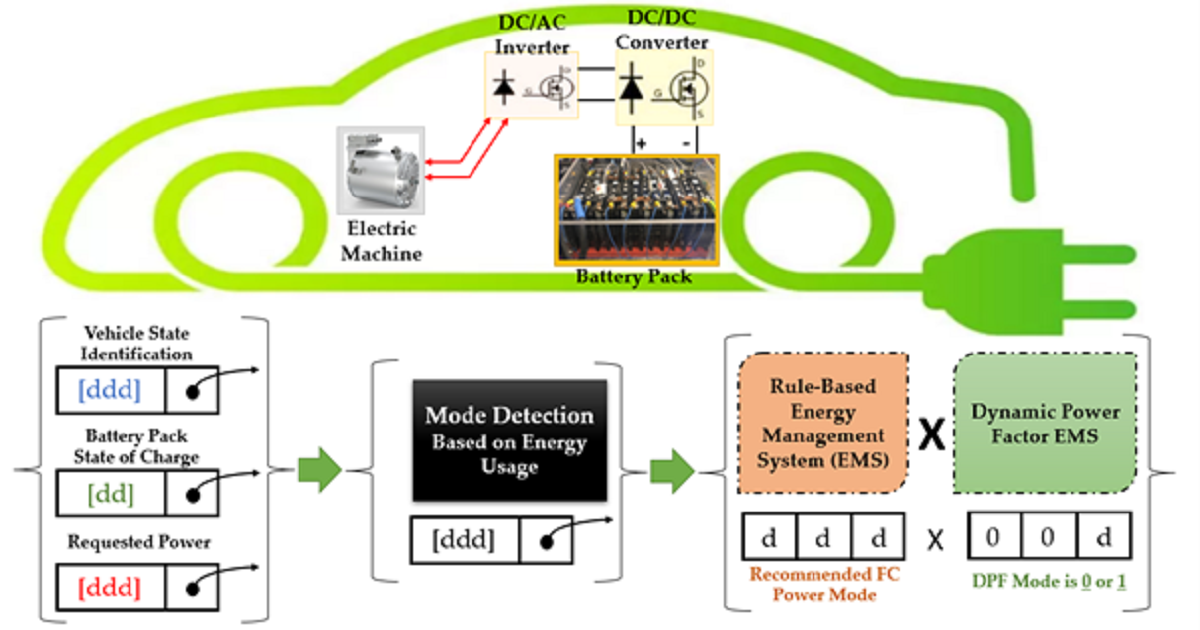
Topic Information
Dear Colleagues,
The current Topic "Electric Vehicles Energy Management, 2nd Volume" aims to build upon the achievements of its predecessor Topic, “Electric Vehicles Energy Management”, maintaining the continuity of advancement and delving deeper into the intricacies of managing energy in the realm of hybrid and electric vehicles. Continuing in this trajectory, the present Topic endeavors to foster interdisciplinary dialogue and collaboration among researchers, engineers, policymakers, and industry practitioners to bring together cutting-edge research, innovative technologies, and real-world insights, and aspires to catalyze advancements that drive the widespread integration of hybrid and electric vehicles into our transportation ecosystem.
In this context, we invite contributions that explore methodologies, tests, algorithms, models, and technologies aimed at optimizing energy utilization, enhancing vehicle performance, extending battery life, and improving overall system efficiency in electric and hybrid vehicles. Topics of interest include but are not limited to:
- Energy-efficient powertrain design, sizing, and control.
- Predictive energy management strategies.
- Advanced battery management systems.
- Life cycle assessments.
- Minimized drivetrain cost/energy consumption/environmental impact.
- Extended-range analyses and trajectory planning.
- Vehicle-to-grid and grid-to-vehicle integration and protocols.
- Connected and non-connected self-driving vehicle energy management.
- Smart charging infrastructures.
- Sustainable mobility solutions and policy frameworks.
We encourage researchers, practitioners, and stakeholders from academia, industry, and government agencies to contribute original research articles, review papers, and case studies that shed light on the latest advancements and best practices within the discipline.
Dr. Danial Karimi
Dr. Majid Vafaeipour
Topic Editors
Keywords
- energy management
- electric vehicles
- extended range
- energy storage
- batteries
- fuel economy
- powertrain
- power electronics
- electrical machines and drives
- modeling and design
Â
Participating Journals
Journal Name | Impact Factor | CiteScore | Launched Year | First Decision (median) | APC | |
---|---|---|---|---|---|---|
![]()
Batteries
|
4.6 | 4.0 | 2015 | 19.7 Days | CHF 2700 | Submit |
![]()
Electronics
|
2.6 | 5.3 | 2012 | 16.4 Days | CHF 2400 | Submit |
![]()
Energies
|
3.0 | 6.2 | 2008 | 16.8 Days | CHF 2600 | Submit |
![]()
Sustainability
|
3.3 | 6.8 | 2009 | 19.7 Days | CHF 2400 | Submit |
![]()
Vehicles
|
2.4 | 4.1 | 2019 | 19.9 Days | CHF 1600 | Submit |
![]()
World Electric Vehicle Journal
|
2.6 | 4.5 | 2007 | 16.2 Days | CHF 1400 | Submit |
Preprints.org is a multidisciplinary platform offering a preprint service designed to facilitate the early sharing of your research. It supports and empowers your research journey from the very beginning.
MDPI Topics is collaborating with Preprints.org and has established a direct connection between MDPI journals and the platform. Authors are encouraged to take advantage of this opportunity by posting their preprints at Preprints.org prior to publication:
- Share your research immediately: disseminate your ideas prior to publication and establish priority for your work.
- Safeguard your intellectual contribution: Protect your ideas with a time-stamped preprint that serves as proof of your research timeline.
- Boost visibility and impact: Increase the reach and influence of your research by making it accessible to a global audience.
- Gain early feedback: Receive valuable input and insights from peers before submitting to a journal.
- Ensure broad indexing: Web of Science (Preprint Citation Index), Google Scholar, Crossref, SHARE, PrePubMed, Scilit and Europe PMC.
Related Topic
- Electric Vehicles Energy Management (30 articles)