Symmetry Applied in Computer Vision, Automation, and Robotics
A special issue of Symmetry (ISSN 2073-8994). This special issue belongs to the section "Computer".
Deadline for manuscript submissions: 31 May 2025 | Viewed by 8195
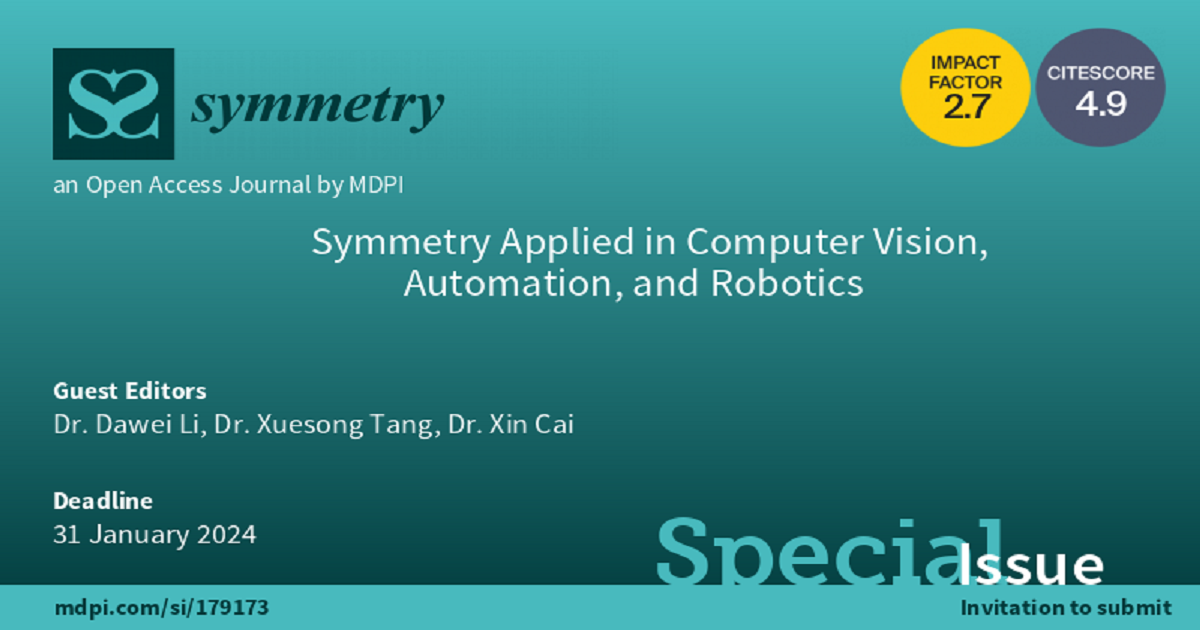
Special Issue Editors
Interests: image processing; point cloud processing; Artificial Intelligence; intelligent visual surveillance and plant phenotyping
Interests: computer vision; deep learning; brain science; natural language processing; evolutionary computation
Special Issue Information
Dear Colleagues,
Computer vision has become an indispensable part of many fields such as manufacturing, health care, transportation, and has profoundly influenced our daily life (e.g., through algorithms in smart phones and cameras, search engines, and social networks). Automation and robotic systems, such as self-driving vehicles, autonomous robots and unmanned workshop plants, have also had a significant impact on society and human life. Therefore, it is necessary to keep carrying out advanced research on computer vision, automation and robotics.
Symmetry plays a crucial role in various aspects of computer vision, automation and robotics. This Special Issue, entitled “Symmetry in Applied Computer Vision, Automation, and Robotics”, mainly covers the topics of the theory, phenomenon, and research regarding symmetry in applied computer vision, automation, and robotics. This Special Issue will also attempt to cover the whole field of symmetry (and asymmetry) in its widest sense. We cordially and earnestly invite researchers to contribute their original and high-quality research papers that will inspire advances in computer vision, image processing, 3D sensing, automation, control system and control engineering, robotics, robotic control, optimization, and their symmetry-related applications. We encourage submissions that cover a wide range of topics, including, but not limited to, the following:
- Learning-based modeling of automation systems with symmetry;
- Advanced controller design for automation systems with symmetry;
- Model predictive control based on symmetry;
- Motion planning and control strategies with symmetry;
- Symmetry in robot design and morphology;
- Human–robot interaction with symmetry;
- Multi-robot systems and swarm robotics with symmetry;
- Learning and adaptation in robotics with symmetry;
- Robot manipulation and grasping with symmetry;
- Robot mapping and localization with symmetry;
- Symmetry in computer vision;
- Image processing with symmetry;
- Point cloud processing with symmetry;
- Pattern analysis and machine learning in computer vision with symmetry applications;
- Deep learning on images or other regular data forms with symmetry.
Dr. Dawei Li
Dr. Xuesong Tang
Dr. Xin Cai
Guest Editors
Manuscript Submission Information
Manuscripts should be submitted online at www.mdpi.com by registering and logging in to this website. Once you are registered, click here to go to the submission form. Manuscripts can be submitted until the deadline. All submissions that pass pre-check are peer-reviewed. Accepted papers will be published continuously in the journal (as soon as accepted) and will be listed together on the special issue website. Research articles, review articles as well as short communications are invited. For planned papers, a title and short abstract (about 100 words) can be sent to the Editorial Office for announcement on this website.
Submitted manuscripts should not have been published previously, nor be under consideration for publication elsewhere (except conference proceedings papers). All manuscripts are thoroughly refereed through a single-blind peer-review process. A guide for authors and other relevant information for submission of manuscripts is available on the Instructions for Authors page. Symmetry is an international peer-reviewed open access monthly journal published by MDPI.
Please visit the Instructions for Authors page before submitting a manuscript. The Article Processing Charge (APC) for publication in this open access journal is 2400 CHF (Swiss Francs). Submitted papers should be well formatted and use good English. Authors may use MDPI's English editing service prior to publication or during author revisions.
Keywords
- symmetry phenomenon and theory
- symmetry-based applications
- dynamical systems
- optimization
- optimal control
- model predictive control
- learning-based control
- learning-based modelling
- robot design and morphology
- multi-robot systems
- robot manipulation and grasping
- motion planning
- robot mapping and localization
- computer vision
- image processing
- pattern analysis and machine learning
- deep learning
Benefits of Publishing in a Special Issue
- Ease of navigation: Grouping papers by topic helps scholars navigate broad scope journals more efficiently.
- Greater discoverability: Special Issues support the reach and impact of scientific research. Articles in Special Issues are more discoverable and cited more frequently.
- Expansion of research network: Special Issues facilitate connections among authors, fostering scientific collaborations.
- External promotion: Articles in Special Issues are often promoted through the journal's social media, increasing their visibility.
- e-Book format: Special Issues with more than 10 articles can be published as dedicated e-books, ensuring wide and rapid dissemination.
Further information on MDPI's Special Issue policies can be found here.