Journal Description
Stats
Stats
is an international, peer-reviewed, open access journal on statistical science published quarterly online by MDPI. The journal focuses on methodological and theoretical papers in statistics, probability, stochastic processes and innovative applications of statistics in all scientific disciplines including biological and biomedical sciences, medicine, business, economics and social sciences, physics, data science and engineering.
- Open Access— free for readers, with article processing charges (APC) paid by authors or their institutions.
- High Visibility: indexed within ESCI (Web of Science), Scopus, RePEc, and other databases.
- Rapid Publication: manuscripts are peer-reviewed and a first decision is provided to authors approximately 19.7 days after submission; acceptance to publication is undertaken in 3.9 days (median values for papers published in this journal in the second half of 2024).
- Recognition of Reviewers: reviewers who provide timely, thorough peer-review reports receive vouchers entitling them to a discount on the APC of their next publication in any MDPI journal, in appreciation of the work done.
Impact Factor:
0.9 (2023);
5-Year Impact Factor:
1.0 (2023)
Latest Articles
Revisiting the Replication Crisis and the Untrustworthiness of Empirical Evidence
Stats 2025, 8(2), 41; https://doi.org/10.3390/stats8020041 - 20 May 2025
Abstract
The current replication crisis relating to the non-replicability and the untrustworthiness of published empirical evidence is often viewed through the lens of the Positive Predictive Value (PPV) in the context of the Medical Diagnostic Screening (MDS) model. The PPV is misconstrued as a
[...] Read more.
The current replication crisis relating to the non-replicability and the untrustworthiness of published empirical evidence is often viewed through the lens of the Positive Predictive Value (PPV) in the context of the Medical Diagnostic Screening (MDS) model. The PPV is misconstrued as a measure that evaluates `the probability of rejecting when false’, after being metamorphosed by replacing its false positive/negative probabilities with the type I/II error probabilities. This perspective gave rise to a widely accepted diagnosis that the untrustworthiness of published empirical evidence stems primarily from abuses of frequentist testing, including p-hacking, data-dredging, and cherry-picking. It is argued that the metamorphosed PPV misrepresents frequentist testing and misdiagnoses the replication crisis, promoting ill-chosen reforms. The primary source of untrustworthiness is statistical misspecification: invalid probabilistic assumptions imposed on one’s data. This is symptomatic of the much broader problem of the uninformed and recipe-like implementation of frequentist statistics without proper understanding of (a) the invoked probabilistic assumptions and their validity for the data used, (b) the reasoned implementation and interpretation of the inference procedures and their error probabilities, and (c) warranted evidential interpretations of inference results. A case is made that Fisher’s model-based statistics offers a more pertinent and incisive diagnosis of the replication crisis, and provides a well-grounded framework for addressing the issues (a)–(c), which would unriddle the non-replicability/untrustworthiness problems.
Full article
Open AccessArticle
An Analysis of Vectorised Automatic Differentiation for Statistical Applications
by
Chun Fung Kwok, Dan Zhu and Liana Jacobi
Stats 2025, 8(2), 40; https://doi.org/10.3390/stats8020040 - 19 May 2025
Abstract
Automatic differentiation (AD) is a general method for computing exact derivatives in complex sensitivity analyses and optimisation tasks, particularly when closed-form solutions are unavailable and traditional analytical or numerical methods fall short. This paper introduces a vectorised formulation of AD grounded in matrix
[...] Read more.
Automatic differentiation (AD) is a general method for computing exact derivatives in complex sensitivity analyses and optimisation tasks, particularly when closed-form solutions are unavailable and traditional analytical or numerical methods fall short. This paper introduces a vectorised formulation of AD grounded in matrix calculus. It aligns naturally with the matrix-oriented style prevalent in statistics, supports convenient implementations, and takes advantage of sparse matrix representation and other high-level optimisation techniques that are not available in the scalar counterpart. Our formulation is well-suited to high-dimensional statistical applications, where finite differences (FD) scale poorly due to the need to repeat computations for each input dimension, resulting in significant overhead, and is advantageous in simulation-intensive settings—such as Markov Chain Monte Carlo (MCMC)-based inference—where FD requires repeated sampling and multiple function evaluations, while AD can compute exact derivatives in a single pass, substantially reducing computational cost. Numerical studies are presented to demonstrate the efficacy and speed of the proposed AD method compared with FD schemes.
Full article
(This article belongs to the Section Computational Statistics)
Open AccessArticle
Theoretical Advancements in Small Area Modeling: A Case Study with the CHILD Cohort
by
Charanpal Singh and Mahmoud Torabi
Stats 2025, 8(2), 39; https://doi.org/10.3390/stats8020039 - 16 May 2025
Abstract
►▼
Show Figures
Developing accurate predictive models in statistical analysis presents significant challenges, especially in domains with limited routine assessments. This study aims to advance the theoretical underpinnings of longitudinal logistic and zero-inflated Poisson (ZIP) models in the context of small area estimation (SAE). Utilizing data
[...] Read more.
Developing accurate predictive models in statistical analysis presents significant challenges, especially in domains with limited routine assessments. This study aims to advance the theoretical underpinnings of longitudinal logistic and zero-inflated Poisson (ZIP) models in the context of small area estimation (SAE). Utilizing data from the Canadian Healthy Infant Longitudinal Development (CHILD) study as a case study, we explore the use of individual- and area-level random effects to enhance model precision and reliability. The study evaluates various covariates’ impact (such as mother’s asthma, mother wheezed, mother smoked) on model performance to predict child’s wheezing, emphasizing the role of location within Manitoba. Our main findings contribute to the literature by providing insights into the development and refinement of small area models, emphasizing the significance of advancing theoretical frameworks in statistical modeling.
Full article
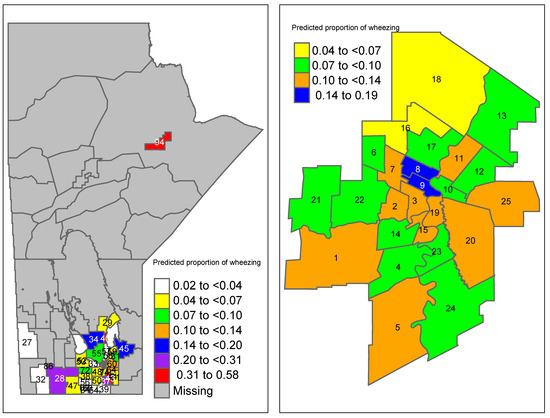
Figure 1
Open AccessArticle
Determinants of Blank and Null Votes in the Brazilian Presidential Elections
by
Renata Rojas Guerra, Kerolene De Souza Moraes, Fernando De Jesus Moreira Junior, Fernando A. Peña-Ramírez and Ryan Novaes Pereira
Stats 2025, 8(2), 38; https://doi.org/10.3390/stats8020038 - 13 May 2025
Abstract
This study analyzes the factors influencing the proportions of blank and null votes in Brazilian municipalities during the 2018 presidential elections. The behavior of the variable of interest is examined using unit regression models within the Generalized Additive Models for Location, Scale, and
[...] Read more.
This study analyzes the factors influencing the proportions of blank and null votes in Brazilian municipalities during the 2018 presidential elections. The behavior of the variable of interest is examined using unit regression models within the Generalized Additive Models for Location, Scale, and Shape (GAMLSS) framework. Specifically, five different unit regression models are explored, beta, simplex, Kumaraswamy, unit Weibull, and reflected unit Burr XII regressions, each incorporating submodels for both indexed distribution parameters. The beta regression model emerges as the best fit through rigorous model selection and diagnostic procedures. The findings reveal that the disaggregated municipal human development index (MHDI), particularly its income, longevity, and education dimensions, along with the municipality’s geographic region, significantly affect voting behavior. Notably, higher income and longevity values are linked to greater proportions of blank and null votes, whereas the educational level exhibits a negative relationship with the variable of interest. Additionally, municipalities in the Southeast region tend to have higher average proportions of blank and null votes. In terms of variability, the ability of a municipality’s population to acquire goods and services is shown to negatively influence the dispersion of vote proportions, while municipalities in the Northeast, North, and Southeast regions exhibit distinct patterns of variation compared to other regions. These results provide valuable insights into electoral participation’s socioeconomic and regional determinants, contributing to broader discussions on political engagement and democratic representation in Brazil.
Full article
(This article belongs to the Section Regression Models)
►▼
Show Figures

Figure 1
Open AccessArticle
A Cox Proportional Hazards Model with Latent Covariates Reflecting Students’ Preparation, Motives, and Expectations for the Analysis of Time to Degree
by
Dimitrios Kalamaras, Laura Maska and Fani Nasika
Stats 2025, 8(2), 37; https://doi.org/10.3390/stats8020037 - 13 May 2025
Abstract
Issues related to the duration of university studies have attracted the interest of many researchers from different scientific fields, as far back as the middle of the 20th century. In this study, a Survival Analysis methodology and, more specifically, a Cox Proportional hazards
[...] Read more.
Issues related to the duration of university studies have attracted the interest of many researchers from different scientific fields, as far back as the middle of the 20th century. In this study, a Survival Analysis methodology and, more specifically, a Cox Proportional hazards model, has been proposed to evaluate a theoretical framework/model that relates the risk a student might face either graduating on time or having a late graduation, with a number of observed and latent factors that have been proposed in the literature as the main determinants of time to degree completion. The major findings of the analysis suggest that the factors contributing to reducing the duration of studies include high academic achievements at early stages, positive motivation, expectations, attitudes, and beliefs regarding studies. On the contrary, external situations, negative academic experiences, and some individual characteristics of the students contribute to an extended duration of studies.
Full article
(This article belongs to the Topic Interfacing Statistics, Machine Learning and Data Science from a Probabilistic Modelling Viewpoint)
►▼
Show Figures
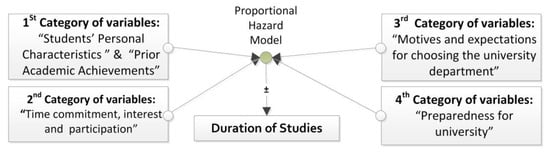
Figure 1
Open AccessCommunication
Unraveling Meteorological Dynamics: A Two-Level Clustering Algorithm for Time Series Pattern Recognition with Missing Data Handling
by
Ekaterini Skamnia, Eleni S. Bekri and Polychronis Economou
Stats 2025, 8(2), 36; https://doi.org/10.3390/stats8020036 - 9 May 2025
Abstract
►▼
Show Figures
Identifying regions with similar meteorological features is of both socioeconomic and ecological importance. Towards that direction, useful information can be drawn from meteorological stations, and spread in a broader area. In this work, a time series clustering procedure composed of two levels is
[...] Read more.
Identifying regions with similar meteorological features is of both socioeconomic and ecological importance. Towards that direction, useful information can be drawn from meteorological stations, and spread in a broader area. In this work, a time series clustering procedure composed of two levels is proposed, focusing on clustering spatial units (meteorological stations) based on their temporal patterns, rather than clustering time periods. It is capable of handling univariate or multivariate time series, with missing data or different lengths but with a common seasonal time period. The first level involves the clustering of the dominant features of the time series (e.g., similar seasonal patterns) by employing K-means, while the second one produces clusters based on secondary features. Hierarchical clustering with Dynamic Time Warping for the univariate case and multivariate Dynamic Time Warping for the multivariate scenario are employed for the second level. Principal component analysis or Classic Multidimensional Scaling is applied before the first level, while an imputation technique is applied to the raw data in the second level to address missing values in the dataset. This step is particularly important given that missing data is a frequent issue in measurements obtained from meteorological stations. The method is subsequently applied to the available precipitation time series and then also to a time series of mean temperature obtained by the automated weather stations network in Greece. Further, both of the characteristics are employed to cover the multivariate scenario.
Full article
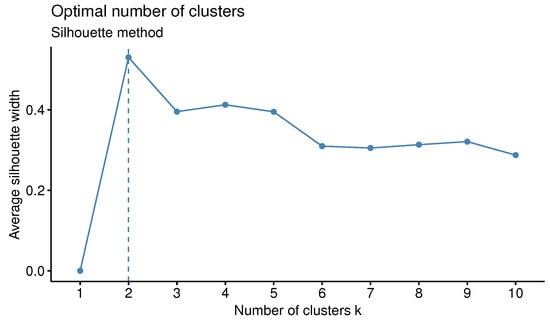
Figure 1
Open AccessArticle
Reliability Assessment via Combining Data from Similar Systems
by
Jianping Hao and Mochao Pei
Stats 2025, 8(2), 35; https://doi.org/10.3390/stats8020035 - 8 May 2025
Abstract
►▼
Show Figures
In operational testing contexts, testers face dual challenges of constrained timeframes and limited resources, both of which impede the generation of reliability test data. To address this issue, integrating data from similar systems with test data can effectively expand data sources. This study
[...] Read more.
In operational testing contexts, testers face dual challenges of constrained timeframes and limited resources, both of which impede the generation of reliability test data. To address this issue, integrating data from similar systems with test data can effectively expand data sources. This study proposes a systematic approach wherein the mission of the system under test (SUT) is decomposed to identify candidate subsystems for data combination. A phylogenetic tree representation is constructed for subsystem analysis and subsequently mapped to a mixed-integer programming (MIP) model, enabling efficient computation of similarity factors. A reliability assessment model that combines data from similar subsystems is established. The similarity factor is regarded as a covariate, and the regression relationship between it and the subsystem failure-time distribution is established. The joint posterior distribution of regression coefficients is derived using Bayesian theory, which are then sampled via the No-U-Turn Sampler (NUTS) algorithm to obtain reliability estimates. Numerical case studies demonstrate that the proposed method outperforms existing approaches, yielding more robust similarity factors and higher accuracy in reliability assessments.
Full article
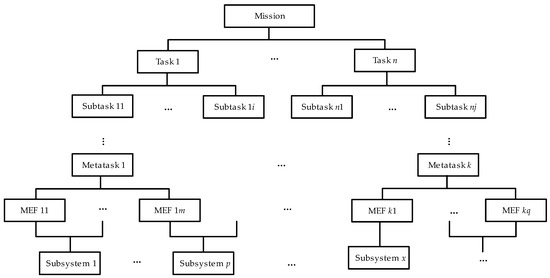
Figure 1
Open AccessArticle
Estimation of Weighted Extropy Under the α-Mixing Dependence Condition
by
Radhakumari Maya, Archana Krishnakumar, Muhammed Rasheed Irshad and Christophe Chesneau
Stats 2025, 8(2), 34; https://doi.org/10.3390/stats8020034 - 1 May 2025
Abstract
►▼
Show Figures
Introduced as a complementary concept to Shannon entropy, extropy provides an alternative perspective for measuring uncertainty. While useful in areas such as reliability theory and scoring rules, extropy in its original form treats all outcomes equally, which can limit its applicability in real-world
[...] Read more.
Introduced as a complementary concept to Shannon entropy, extropy provides an alternative perspective for measuring uncertainty. While useful in areas such as reliability theory and scoring rules, extropy in its original form treats all outcomes equally, which can limit its applicability in real-world settings where different outcomes have varying degrees of importance. To address this, the weighted extropy measure incorporates a weight function that reflects the relative significance of outcomes, thereby increasing the flexibility and sensitivity of uncertainty quantification. In this paper, we propose a novel recursive non-parametric kernel estimator for weighted extropy based on -mixing dependent observations, a common setting in time series and stochastic processes. The recursive formulation allows for efficient updating with sequential data, making it particularly suitable for real-time analysis. We establish several theoretical properties of the estimator, including its recursive structure, consistency, and asymptotic behavior under mild regularity conditions. A comprehensive simulation study and data application demonstrate the practical performance of the estimator and validate its superiority over the non-recursive kernel estimator in terms of accuracy and computational efficiency. The results confirm the relevance of the method for dynamic, dependent, and weighted systems.
Full article
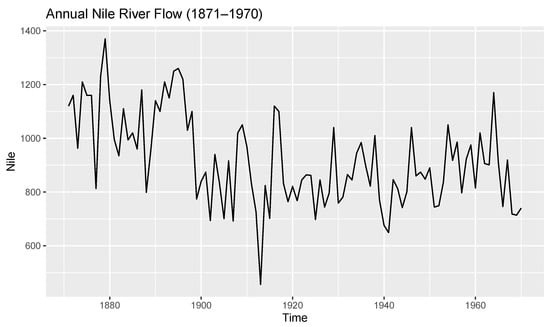
Figure 1
Open AccessArticle
A Smoothed Three-Part Redescending M-Estimator
by
Alistair J. Martin and Brenton R. Clarke
Stats 2025, 8(2), 33; https://doi.org/10.3390/stats8020033 - 30 Apr 2025
Abstract
A smoothed M-estimator is derived from Hampel’s three-part redescending estimator for location and scale. The estimator is shown to be weakly continuous and Fréchet differentiable in the neighbourhood of the normal distribution. Asymptotic assessment is conducted at asymmetric contaminating distributions, where smoothing is
[...] Read more.
A smoothed M-estimator is derived from Hampel’s three-part redescending estimator for location and scale. The estimator is shown to be weakly continuous and Fréchet differentiable in the neighbourhood of the normal distribution. Asymptotic assessment is conducted at asymmetric contaminating distributions, where smoothing is shown to improve variance and change-of-variance sensitivity. Other robust metrics compared are largely unchanged, and therefore, the smoothed functions represent an improvement for asymmetric contamination near the rejection point with little downside.
Full article
(This article belongs to the Section Statistical Methods)
►▼
Show Figures
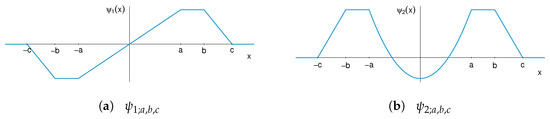
Figure 1
Open AccessArticle
Longitudinal Survival Analysis Using First Hitting Time Threshold Regression: With Applications to Wiener Processes
by
Ya-Shan Cheng, Yiming Chen and Mei-Ling Ting Lee
Stats 2025, 8(2), 32; https://doi.org/10.3390/stats8020032 - 28 Apr 2025
Abstract
►▼
Show Figures
First-hitting time threshold regression (TR) is well-known for analyzing event time data without the proportional hazards assumption. To date, most applications and software are developed for cross-sectional data. In this paper, using the Markov property of processes with stationary independent increments, we present
[...] Read more.
First-hitting time threshold regression (TR) is well-known for analyzing event time data without the proportional hazards assumption. To date, most applications and software are developed for cross-sectional data. In this paper, using the Markov property of processes with stationary independent increments, we present methods and procedures for conducting longitudinal threshold regression (LTR) for event time data with or without covariates. We demonstrate the usage of LTR in two case scenarios, namely, analyzing laser reliability data without covariates, and cardiovascular health data with time-dependent covariates. Moreover, we provide a simple-to-use R function for LTR estimation for applications using Wiener processes.
Full article
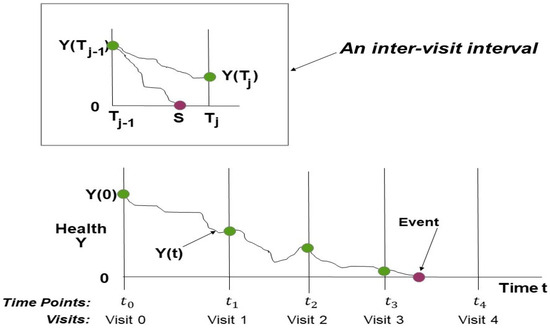
Figure 1
Open AccessArticle
Adaptive Clinical Trials and Sample Size Determination in the Presence of Measurement Error and Heterogeneity
by
Hassan Farooq, Sajid Ali, Ismail Shah, Ibrahim A. Nafisah and Mohammed M. A. Almazah
Stats 2025, 8(2), 31; https://doi.org/10.3390/stats8020031 - 25 Apr 2025
Abstract
Adaptive clinical trials offer a flexible approach for refining sample sizes during ongoing research to enhance their efficiency. This study delves into improving sample size recalculation through resampling techniques, employing measurement error and mixed distribution models. The research employs diverse sample size-recalculation strategies
[...] Read more.
Adaptive clinical trials offer a flexible approach for refining sample sizes during ongoing research to enhance their efficiency. This study delves into improving sample size recalculation through resampling techniques, employing measurement error and mixed distribution models. The research employs diverse sample size-recalculation strategies standard simulation, R1 and R2 approaches where R1 considers the mean and R2 employs both mean and standard deviation as summary locations. These strategies are tested against observed conditional power (OCP), restricted observed conditional power (ROCP), promising zone (PZ) and group sequential design (GSD). The key findings indicate that the R1 approach, capitalizing on mean as a summary location, outperforms standard recalculations without resampling as it mitigates variability in recalculated sample sizes across effect sizes. The OCP exhibits superior performance within the R1 approach compared to ROCP, PZ and GSD due to enhanced conditional power. However, a tendency to inflate the initial stage’s sample size is observed in the R1 approach, prompting the development of the R2 approach that considers mean and standard deviation. The ROCP in the R2 approach demonstrates robust performance across most effect sizes, although GSD retains superiority within the R2 approach due to its sample size boundary. Notably, sample size-recalculation designs perform worse than R1 for specific effect sizes, attributed to inefficiencies in approaching target sample sizes. The resampling-based approaches, particularly R1 and R2, offer improved sample size recalculation over conventional methods. The R1 approach excels in minimizing recalculated sample size variability, while the R2 approach presents a refined alternative.
Full article
Open AccessCommunication
An Integrated Hybrid-Stochastic Framework for Agro-Meteorological Prediction Under Environmental Uncertainty
by
Mohsen Pourmohammad Shahvar, Davide Valenti, Alfonso Collura, Salvatore Micciche, Vittorio Farina and Giovanni Marsella
Stats 2025, 8(2), 30; https://doi.org/10.3390/stats8020030 - 25 Apr 2025
Abstract
This study presents a comprehensive framework for agro-meteorological prediction, combining stochastic modeling, machine learning techniques, and environmental feature engineering to address challenges in yield prediction and wind behavior modeling. Focused on mango cultivation in the Mediterranean region, the workflow integrates diverse datasets, including
[...] Read more.
This study presents a comprehensive framework for agro-meteorological prediction, combining stochastic modeling, machine learning techniques, and environmental feature engineering to address challenges in yield prediction and wind behavior modeling. Focused on mango cultivation in the Mediterranean region, the workflow integrates diverse datasets, including satellite-derived variables such as NDVI, soil moisture, and land surface temperature (LST), along with meteorological features like wind speed and direction. Stochastic modeling was employed to capture environmental variability, while a proxy yield was defined using key environmental factors in the absence of direct field yield measurements. Machine learning models, including random forest and multi-layer perceptron (MLP), were hybridized to improve the prediction accuracy for both proxy yield and wind components (U and V that represent the east–west and north–south wind movement). The hybrid model achieved mean squared error (MSE) values of 0.333 for U and 0.181 for V, with corresponding R2 values of 0.8939 and 0.9339, respectively, outperforming the individual models and demonstrating reliable generalization in the 2022 test set. Additionally, although NDVI is traditionally important in crop monitoring, its low temporal variability across the observation period resulted in minimal contribution to the final prediction, as confirmed by feature importance analysis. Furthermore, the analysis revealed the significant influence of environmental factors such as LST, precipitable water, and soil moisture on yield dynamics, while wind visualization over digital elevation models (DEMs) highlighted the impact of terrain features on the wind patterns. The results demonstrate the effectiveness of combining stochastic and machine learning approaches in agricultural modeling, offering valuable insights for crop management and climate adaptation strategies.
Full article
(This article belongs to the Section Applied Statistics and Machine Learning Methods)
►▼
Show Figures
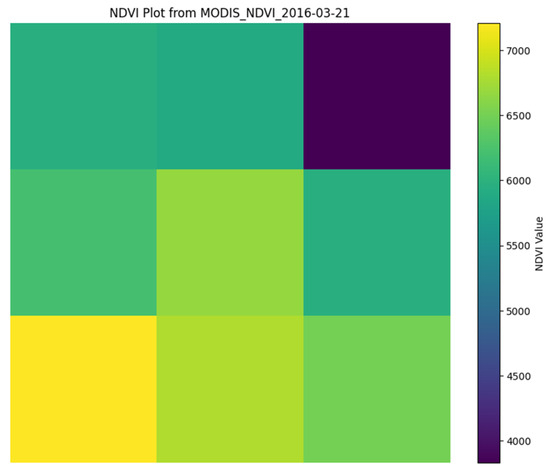
Figure 1
Open AccessArticle
Bias-Corrected Fixed Item Parameter Calibration, with an Application to PISA Data
by
Alexander Robitzsch
Stats 2025, 8(2), 29; https://doi.org/10.3390/stats8020029 - 24 Apr 2025
Abstract
►▼
Show Figures
Fixed item parameter calibration (FIPC) is commonly used to compare groups or countries using an item response theory model with a common set of fixed item parameters. However, FIPC has been shown to produce biased estimates of group means and standard deviations in
[...] Read more.
Fixed item parameter calibration (FIPC) is commonly used to compare groups or countries using an item response theory model with a common set of fixed item parameters. However, FIPC has been shown to produce biased estimates of group means and standard deviations in the presence of random differential item functioning (DIF). To address this, a bias-corrected variant of FIPC, called BCFIPC, is introduced in this article. BCFIPC eliminated the bias of FIPC with only minor efficiency losses in certain simulation conditions, but substantial precision gains in many others, particularly for estimating group standard deviations. Finally, a comparison of both methods using the PISA 2006 dataset revealed relatively large differences in country means and standard deviations.
Full article
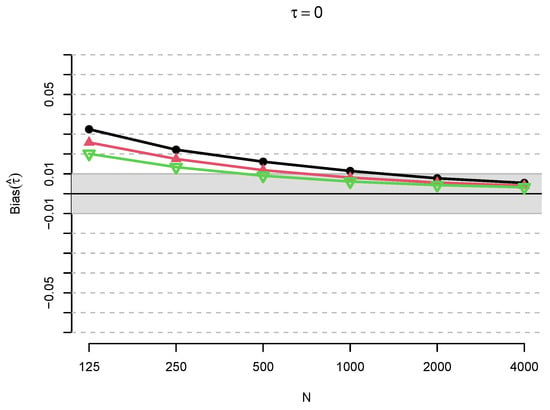
Figure 1
Open AccessFeature PaperArticle
Inferences About Two-Parameter Multicollinear Gaussian Linear Regression Models: An Empirical Type I Error and Power Comparison
by
Md Ariful Hoque, Zoran Bursac and B. M. Golam Kibria
Stats 2025, 8(2), 28; https://doi.org/10.3390/stats8020028 - 23 Apr 2025
Abstract
In linear regression analysis, the independence assumption is crucial and the ordinary least square (OLS) estimator generally regarded as the Best Linear Unbiased Estimator (BLUE) is applied. However, multicollinearity can complicate the estimation of the effect of individual variables, leading to potential inaccurate
[...] Read more.
In linear regression analysis, the independence assumption is crucial and the ordinary least square (OLS) estimator generally regarded as the Best Linear Unbiased Estimator (BLUE) is applied. However, multicollinearity can complicate the estimation of the effect of individual variables, leading to potential inaccurate statistical inferences. Because of this issue, different types of two-parameter estimators have been explored. This paper compares t-tests for assessing the significance of regression coefficients, including several two-parameter estimators. We conduct a Monte Carlo study to evaluate these methods by examining their empirical type I error and power characteristics, based on established protocols. The simulation results indicate that some two-parameter estimators achieve better power gains while preserving the nominal size at 5%. Real-life data are analyzed to illustrate the findings of this paper.
Full article
(This article belongs to the Section Statistical Methods)
►▼
Show Figures
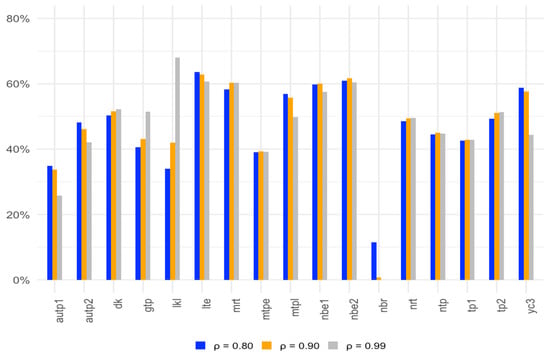
Figure 1
Open AccessArticle
Detailed Command vs. Mission Command: A Cancer-Stage Model of Institutional Decision-Making
by
Rodrick Wallace
Stats 2025, 8(2), 27; https://doi.org/10.3390/stats8020027 - 19 Apr 2025
Abstract
►▼
Show Figures
Those accustomed to acting within ‘normal’ bureaucracies will have experienced the degradation, distortion, and stunting imposed by inordinate levels of hierarchical ‘decision structure’, particularly under the critical time constraints so fondly exploited by John Boyd and his followers. Here, via an approach based
[...] Read more.
Those accustomed to acting within ‘normal’ bureaucracies will have experienced the degradation, distortion, and stunting imposed by inordinate levels of hierarchical ‘decision structure’, particularly under the critical time constraints so fondly exploited by John Boyd and his followers. Here, via an approach based on the asymptotic limit theorems of information and control theories, we explore this dynamic in detail, abducting ideas from the theory of carcinogenesis. The resulting probability models can, with some effort, be converted into new statistical tools for analysis of real time, real world data involving cognitive phenomena and their dysfunctions across a considerable range of scales and levels of organization.
Full article
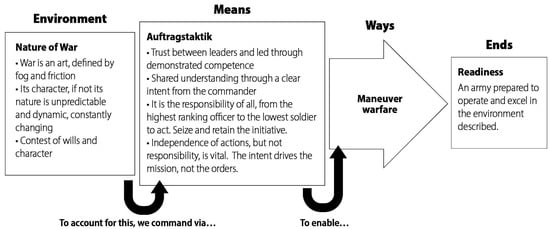
Figure 1
Open AccessArticle
The New Marshall–Olkin–Type II Exponentiated Half-Logistic–Odd Burr X-G Family of Distributions with Properties and Applications
by
Broderick Oluyede, Thatayaone Moakofi and Gomolemo Lekono
Stats 2025, 8(2), 26; https://doi.org/10.3390/stats8020026 - 4 Apr 2025
Abstract
►▼
Show Figures
We develop a novel family of distributions named the Marshall–Olkin type II exponentiated half-logistic–odd Burr X-G distribution. Several mathematical properties including linear representation of the density function, Rényi entropy, probability-weighted moments, and distribution of order statistics are obtained. Different estimation methods are employed
[...] Read more.
We develop a novel family of distributions named the Marshall–Olkin type II exponentiated half-logistic–odd Burr X-G distribution. Several mathematical properties including linear representation of the density function, Rényi entropy, probability-weighted moments, and distribution of order statistics are obtained. Different estimation methods are employed to estimate the unknown parameters of the new distribution. A simulation study is conducted to assess the effectiveness of the estimation methods. A special model of the new distribution is used to show its usefulness in various disciplines.
Full article
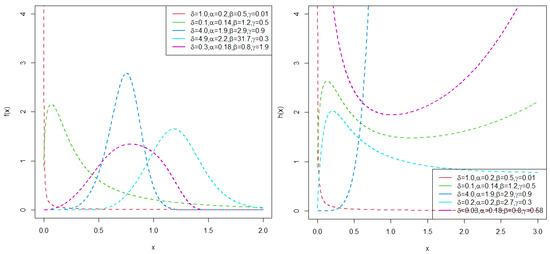
Figure 1
Open AccessArticle
Affine Calculus for Constrained Minima of the Kullback–Leibler Divergence
by
Giovanni Pistone
Stats 2025, 8(2), 25; https://doi.org/10.3390/stats8020025 - 21 Mar 2025
Abstract
The non-parametric version of Amari’s dually affine Information Geometry provides a practical calculus to perform computations of interest in statistical machine learning. The method uses the notion of a statistical bundle, a mathematical structure that includes both probability densities and random variables to
[...] Read more.
The non-parametric version of Amari’s dually affine Information Geometry provides a practical calculus to perform computations of interest in statistical machine learning. The method uses the notion of a statistical bundle, a mathematical structure that includes both probability densities and random variables to capture the spirit of Fisherian statistics. We focus on computations involving a constrained minimization of the Kullback–Leibler divergence. We show how to obtain neat and principled versions of known computations in applications such as mean-field approximation, adversarial generative models, and variational Bayes.
Full article
Open AccessArticle
Optimal ANOVA-Based Emulators of Models With(out) Derivatives
by
Matieyendou Lamboni
Stats 2025, 8(1), 24; https://doi.org/10.3390/stats8010024 - 17 Mar 2025
Abstract
This paper proposes new ANOVA-based approximations of functions and emulators of high-dimensional models using either available derivatives or local stochastic evaluations of such models. Our approach makes use of sensitivity indices to design adequate structures of emulators. For high-dimensional models with available derivatives,
[...] Read more.
This paper proposes new ANOVA-based approximations of functions and emulators of high-dimensional models using either available derivatives or local stochastic evaluations of such models. Our approach makes use of sensitivity indices to design adequate structures of emulators. For high-dimensional models with available derivatives, our derivative-based emulators reach dimension-free mean squared errors (MSEs) and a parametric rate of convergence (i.e., ). This approach is extended to cope with every model (without available derivatives) by deriving global emulators that account for the local properties of models or simulators. Such generic emulators enjoy dimension-free biases, parametric rates of convergence, and MSEs that depend on the dimensionality. Dimension-free MSEs are obtained for high-dimensional models with particular distributions from the input. Our emulators are also competitive in dealing with different distributions of the input variables and selecting inputs and interactions. Simulations show the efficiency of our approach.
Full article
(This article belongs to the Section Statistical Methods)
►▼
Show Figures
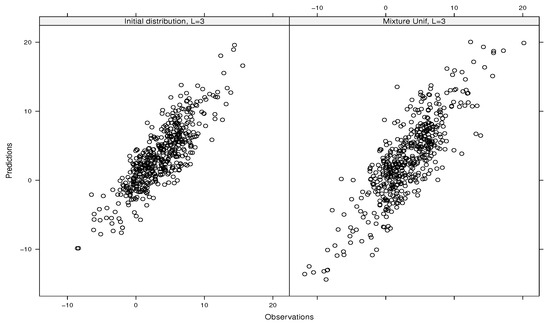
Figure 1
Open AccessArticle
Statistical Gravity and Entropy of Spacetime
by
Riccardo Fantoni
Stats 2025, 8(1), 23; https://doi.org/10.3390/stats8010023 - 13 Mar 2025
Abstract
We discuss the foundations of the statistical gravity theory we proposed in a recent publication [Riccardo Fantoni, Quantum Reports, 6, 706 (2024)].
Full article
Open AccessArticle
A Flexible Bivariate Integer-Valued Autoregressive of Order (1) Model for Over- and Under-Dispersed Time Series Applications
by
Naushad Mamode Khan and Yuvraj Sunecher
Stats 2025, 8(1), 22; https://doi.org/10.3390/stats8010022 - 12 Mar 2025
Abstract
►▼
Show Figures
In real-life inter-related time series, the counting responses of different entities are commonly influenced by some time-dependent covariates, while the individual counting series may exhibit different levels of mutual over- or under-dispersion or mixed levels of over- and under-dispersion. In the current literature,
[...] Read more.
In real-life inter-related time series, the counting responses of different entities are commonly influenced by some time-dependent covariates, while the individual counting series may exhibit different levels of mutual over- or under-dispersion or mixed levels of over- and under-dispersion. In the current literature, there is still no flexible bivariate time series process that can model series of data of such types. This paper introduces a bivariate integer-valued autoregressive of order 1 (BINAR(1)) model with COM-Poisson innovations under time-dependent moments that can accommodate different levels of over- and under-dispersion. Another particularity of the proposed model is that the cross-correlation between the series is induced locally by relating the current observation of one series with the previous-lagged observation of the other series. The estimation of the model parameters is conducted via a Generalized Quasi-Likelihood (GQL) approach. The proposed model is applied to different real-life series problems in Mauritius, including transport, finance, and socio-economic sectors.
Full article
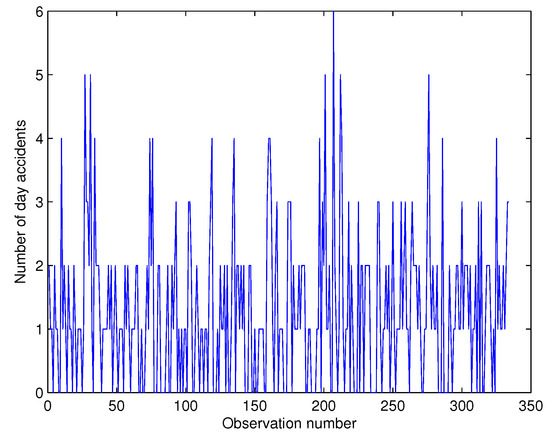
Figure 1
Highly Accessed Articles
Latest Books
E-Mail Alert
News
Topics
Topic in
JPM, Mathematics, Applied Sciences, Stats, Healthcare
Application of Biostatistics in Medical Sciences and Global Health
Topic Editors: Bogdan Oancea, Adrian Pană, Cǎtǎlina Liliana AndreiDeadline: 31 October 2026

Conferences
Special Issues
Special Issue in
Stats
Machine Learning and Natural Language Processing (ML & NLP)
Guest Editor: Stéphane MussardDeadline: 30 June 2025
Special Issue in
Stats
Benford's Law(s) and Applications (Second Edition)
Guest Editors: Marcel Ausloos, Roy Cerqueti, Claudio LupiDeadline: 31 October 2025
Special Issue in
Stats
Nonparametric Inference: Methods and Applications
Guest Editor: Stefano BonniniDeadline: 28 November 2025