Journal Description
Computers
Computers
is an international, scientific, peer-reviewed, open access journal of computer science, including computer and network architecture and computer–human interaction as its main foci, published monthly online by MDPI.
- Open Access— free for readers, with article processing charges (APC) paid by authors or their institutions.
- High Visibility: indexed within Scopus, ESCI (Web of Science), dblp, Inspec, Ei Compendex, and other databases.
- Journal Rank: JCR - Q2 (Computer Science, Interdisciplinary Applications) / CiteScore - Q1 (Computer Science (miscellaneous))
- Rapid Publication: manuscripts are peer-reviewed and a first decision is provided to authors approximately 15.5 days after submission; acceptance to publication is undertaken in 3.8 days (median values for papers published in this journal in the second half of 2024).
- Recognition of Reviewers: reviewers who provide timely, thorough peer-review reports receive vouchers entitling them to a discount on the APC of their next publication in any MDPI journal, in appreciation of the work done.
Impact Factor:
2.6 (2023);
5-Year Impact Factor:
2.4 (2023)
Latest Articles
Exploring the Factors Influencing AI Adoption Intentions in Higher Education: An Integrated Model of DOI, TOE, and TAM
Computers 2025, 14(6), 230; https://doi.org/10.3390/computers14060230 - 11 Jun 2025
Abstract
This study investigates the primary technological and socio-environmental factors influencing the adoption intentions of AI-powered technology at the corporate level within higher education institutions. A conceptual model based on the Diffusion of Innovation Theory (DOI), the Technology–Organization–Environment (TOE), and the Technology Acceptance Model
[...] Read more.
This study investigates the primary technological and socio-environmental factors influencing the adoption intentions of AI-powered technology at the corporate level within higher education institutions. A conceptual model based on the Diffusion of Innovation Theory (DOI), the Technology–Organization–Environment (TOE), and the Technology Acceptance Model (TAM) combined framework were proposed and tested using data collected from 367 higher education students, faculty members, and employees. SPSS Amos 24 was used for CB-SEM to choose the best-fitting model, which proved more efficient than traditional multiple regression analysis to examine the relationships among the proposed constructs, ensuring model fit and statistical robustness. The findings reveal that Compatibility “C”, Complexity “CX”, User Interface “UX”, Perceived Ease of Use “PEOU”, User Satisfaction “US”, Performance Expectation “PE”, Artificial intelligence “AI” introducing new tools “AINT”, AI Strategic Alignment “AIS”, Availability of Resources “AVR”, Technological Support “TS”, and Facilitating Conditions “FC” significantly impact AI adoption intentions. At the same time, Competitive Pressure “COP” and Government Regulations “GOR” do not. Demographic factors, including major and years of experience, moderated these associations, and there were large differences across educational backgrounds and experience.
Full article
(This article belongs to the Special Issue Transformative Approaches in Education: Harnessing AI, Augmented Reality, and Virtual Reality for Innovative Teaching and Learning)
►
Show Figures
Open AccessArticle
LSTM-Based Music Generation Technologies
by
Yi-Jen Mon
Computers 2025, 14(6), 229; https://doi.org/10.3390/computers14060229 - 11 Jun 2025
Abstract
In deep learning, Long Short-Term Memory (LSTM) is a well-established and widely used approach for music generation. Nevertheless, creating musical compositions that match the quality of those created by human composers remains a formidable challenge. The intricate nature of musical components, including pitch,
[...] Read more.
In deep learning, Long Short-Term Memory (LSTM) is a well-established and widely used approach for music generation. Nevertheless, creating musical compositions that match the quality of those created by human composers remains a formidable challenge. The intricate nature of musical components, including pitch, intensity, rhythm, notes, chords, and more, necessitates the extraction of these elements from extensive datasets, making the preliminary work arduous. To address this, we employed various tools to deconstruct the musical structure, conduct step-by-step learning, and then reconstruct it. This article primarily presents the techniques for dissecting musical components in the preliminary phase. Subsequently, it introduces the use of LSTM to build a deep learning network architecture, enabling the learning of musical features and temporal coherence. Finally, through in-depth analysis and comparative studies, this paper validates the efficacy of the proposed research methodology, demonstrating its ability to capture musical coherence and generate compositions with similar styles.
Full article
(This article belongs to the Special Issue Machine Learning: Techniques, Industry Applications, Code Sharing, and Future Trends)
►▼
Show Figures
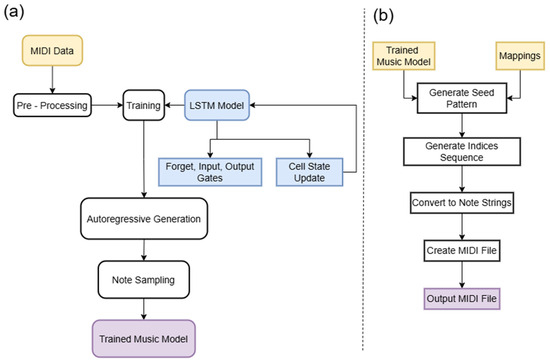
Figure 1
Open AccessArticle
Quantum Classification Outside the Promised Class
by
Theodore Andronikos, Constantinos Bitsakos, Konstantinos Nikas, Georgios I. Goumas and Nectarios Koziris
Computers 2025, 14(6), 228; https://doi.org/10.3390/computers14060228 - 10 Jun 2025
Abstract
This paper studies the important problem of quantum classification of Boolean functions from an entirely novel perspective. Typically, quantum classification algorithms allow us to classify functions with a probability of , if we are promised that they meet specific unique
[...] Read more.
This paper studies the important problem of quantum classification of Boolean functions from an entirely novel perspective. Typically, quantum classification algorithms allow us to classify functions with a probability of , if we are promised that they meet specific unique properties. The primary objective of this study is to explore whether it is feasible to obtain any insights when the input function deviates from the promised class. For concreteness, we use a recently introduced quantum algorithm that is designed to classify a large class of imbalanced Boolean functions with probability using just a single oracular query. First, we establish a completely new concept characterizing “nearness” between Boolean functions. Utilizing this concept, we show that, as long as the unknown function is close enough to the promised class, it is still possible to obtain useful information about its behavioral pattern from the classification algorithm. In this regard, the current study is among the first to provide evidence that shows how useful it is to apply quantum classification algorithms to functions outside the promised class in order to get a glimpse of important information.
Full article
Open AccessArticle
Deploying a Mental Health Chatbot in Higher Education: The Development and Evaluation of Luna, an AI-Based Mental Health Support System
by
Phillip Olla, Ashlee Barnes, Lauren Elliott, Mustafa Abumeeiz, Venus Olla and Joseph Tan
Computers 2025, 14(6), 227; https://doi.org/10.3390/computers14060227 (registering DOI) - 10 Jun 2025
Abstract
Rising mental health challenges among postsecondary students have increased the demand for scalable, ethical solutions. This paper presents the design, development, and safety evaluation of Luna, a GPT-4-based mental health chatbot. Built using a modular PHP architecture, Luna integrates multi-layered prompt engineering, safety
[...] Read more.
Rising mental health challenges among postsecondary students have increased the demand for scalable, ethical solutions. This paper presents the design, development, and safety evaluation of Luna, a GPT-4-based mental health chatbot. Built using a modular PHP architecture, Luna integrates multi-layered prompt engineering, safety guardrails, and referral logic. The Institutional Review Board (IRB) at the University of Detroit Mercy (Protocol #23-24-38) reviewed the proposed study and deferred full human subject approval, requesting technical validation prior to deployment. In response, we conducted a pilot test with a variety of users—including clinicians and students who simulated at-risk student scenarios. Results indicated that 96% of expert interactions were deemed safe, and 90.4% of prompts were considered useful. This paper describes Luna’s architecture, prompt strategy, and expert feedback, concluding with recommendations for future human research trials.
Full article
(This article belongs to the Special Issue Transformative Approaches in Education: Harnessing AI, Augmented Reality, and Virtual Reality for Innovative Teaching and Learning)
►▼
Show Figures
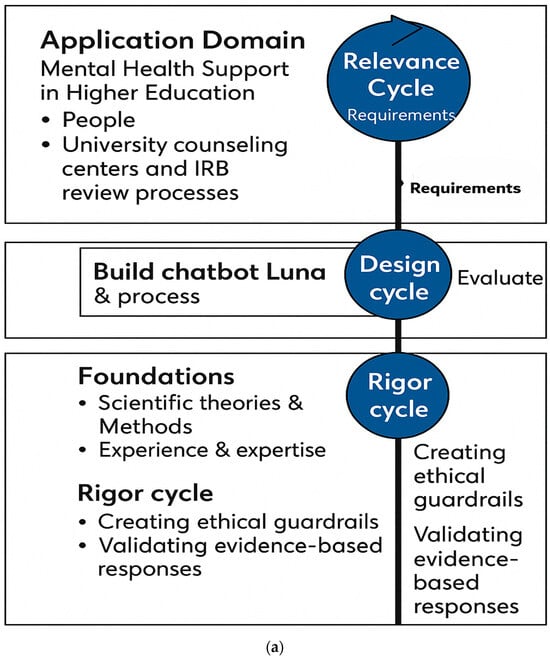
Figure 1
Open AccessSystematic Review
Ethereum Smart Contracts Under Scrutiny: A Survey of Security Verification Tools, Techniques, and Challenges
by
Mounira Kezadri Hamiaz and Maha Driss
Computers 2025, 14(6), 226; https://doi.org/10.3390/computers14060226 - 9 Jun 2025
Abstract
Smart contracts are self-executing programs that facilitate trustless transactions between multiple parties, most commonly deployed on the Ethereum blockchain. They have become integral to decentralized applications in areas such as voting, digital agreements, and financial systems. However, the immutable and transparent nature of
[...] Read more.
Smart contracts are self-executing programs that facilitate trustless transactions between multiple parties, most commonly deployed on the Ethereum blockchain. They have become integral to decentralized applications in areas such as voting, digital agreements, and financial systems. However, the immutable and transparent nature of smart contracts makes security vulnerabilities especially critical, as deployed contracts cannot be modified. Security flaws have led to substantial financial losses, underscoring the need for robust verification before deployment. This survey presents a comprehensive review of the state of the art in smart contract security verification, with a focus on Ethereum. We analyze a wide range of verification methods, including static and dynamic analysis, formal verification, and machine learning, and evaluate 62 open-source tools across their detection accuracy, efficiency, and usability. In addition, we highlight emerging trends, challenges, and the need for cross-methodological integration and benchmarking. Our findings aim to guide researchers, developers, and security auditors in selecting and advancing effective verification approaches for building secure and reliable smart contracts.
Full article
(This article belongs to the Special Issue Blockchain Technology—a Breakthrough Innovation for Modern Industries (2nd Edition))
►▼
Show Figures
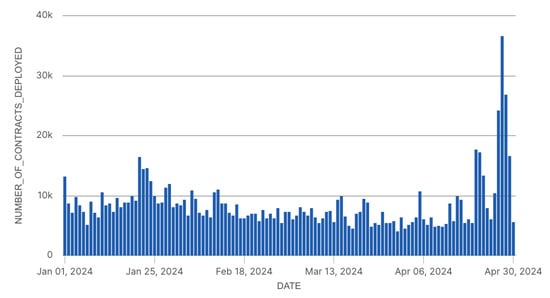
Figure 1
Open AccessArticle
Ensemble-Based Biometric Verification: Defending Against Multi-Strategy Deepfake Image Generation
by
Hilary Zen, Rohan Wagh, Miguel Wanderley, Gustavo Bicalho, Rachel Park, Megan Sun, Rafael Palacios, Lucas Carvalho, Guilherme Rinaldo and Amar Gupta
Computers 2025, 14(6), 225; https://doi.org/10.3390/computers14060225 - 9 Jun 2025
Abstract
►▼
Show Figures
Deepfake images, synthetic images created using digital software, continue to present a serious threat to online platforms. This is especially relevant for biometric verification systems, as deepfakes that attempt to bypass such measures increase the risk of impersonation, identity theft and scams. Although
[...] Read more.
Deepfake images, synthetic images created using digital software, continue to present a serious threat to online platforms. This is especially relevant for biometric verification systems, as deepfakes that attempt to bypass such measures increase the risk of impersonation, identity theft and scams. Although research on deepfake image detection has provided many high-performing classifiers, many of these commonly used detection models lack generalizability across different methods of deepfake generation. For companies and governments fighting identify fraud, a lack of generalization is challenging, as malicious actors may use a variety of deepfake image-generation methods available through online wrappers. This work explores if combining multiple classifiers into an ensemble model can improve generalization without losing performance across different generation methods. It also considers current methods of deepfake image generation, with a focus on publicly available and easily accessible methods. We compare our framework against its underlying models to show how companies can better respond to emerging deepfake generation methods.
Full article
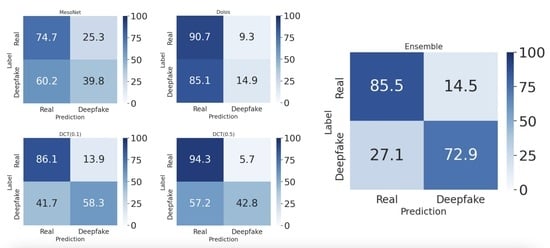
Graphical abstract
Open AccessArticle
Gamifying Sociological Surveys Through Serious Games—A Data Analysis Approach Applied to Multiple-Choice Question Responses Datasets
by
Alexandros Gazis and Eleftheria Katsiri
Computers 2025, 14(6), 224; https://doi.org/10.3390/computers14060224 - 7 Jun 2025
Abstract
►▼
Show Figures
E-polis is a serious digital game designed to gamify sociological surveys studying young people’s political opinions. In this platform game, players navigate a digital world, encountering quests posing sociological questions. Players’ answers shape the city-game world, altering building structures based on their choices.
[...] Read more.
E-polis is a serious digital game designed to gamify sociological surveys studying young people’s political opinions. In this platform game, players navigate a digital world, encountering quests posing sociological questions. Players’ answers shape the city-game world, altering building structures based on their choices. E-polis is a serious game, not a government simulation, aiming to understand players’ behaviors and opinions; thus, we do not train the players but rather understand them and help them visualize their choices in shaping a city’s future. Also, it is noticed that no correct or incorrect answers apply. Moreover, our game utilizes a novel middleware architecture for development, diverging from typical asset-prefab-scene and script segregation. This article presents the data layer of our game’s middleware, specifically focusing on data analysis based on respondents’ gameplay answers. E-polis represents an innovative approach to gamifying sociological research, providing a unique platform for gathering and analyzing data on political opinions among youth and contributing to the broader field of serious games.
Full article
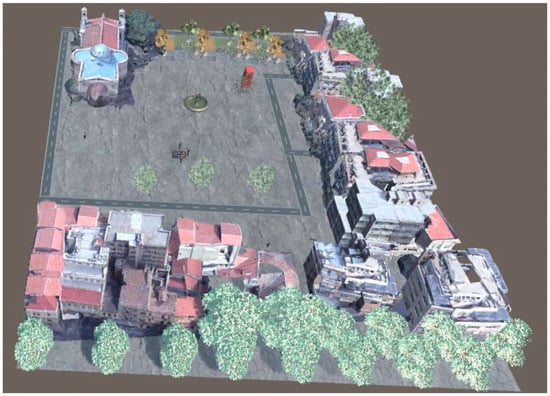
Figure 1
Open AccessArticle
An Efficient Hybrid 3D Computer-Aided Cephalometric Analysis for Lateral Cephalometric and Cone-Beam Computed Tomography (CBCT) Systems
by
Laurine A. Ashame, Sherin M. Youssef, Mazen Nabil Elagamy and Sahar M. El-Sheikh
Computers 2025, 14(6), 223; https://doi.org/10.3390/computers14060223 - 7 Jun 2025
Abstract
Lateral cephalometric analysis is commonly used in orthodontics for skeletal classification to ensure an accurate and reliable diagnosis for treatment planning. However, most current research depends on analyzing different type of radiographs, which requires more computational time than 3D analysis. Consequently, this study
[...] Read more.
Lateral cephalometric analysis is commonly used in orthodontics for skeletal classification to ensure an accurate and reliable diagnosis for treatment planning. However, most current research depends on analyzing different type of radiographs, which requires more computational time than 3D analysis. Consequently, this study addresses fully automatic orthodontics tracing based on the usage of artificial intelligence (AI) applied to 2D and 3D images, by designing a cephalometric system that analyzes the significant landmarks and regions of interest (ROI) needed in orthodontics tracing, especially for the mandible and maxilla teeth. In this research, a computerized system is developed to automate the tasks of orthodontics evaluation during 2D and Cone-Beam Computed Tomography (CBCT or 3D) systems measurements. This work was tested on a dataset that contains images of males and females obtained from dental hospitals with patient-informed consent. The dataset consists of 2D lateral cephalometric, panorama and CBCT radiographs. Many scenarios were applied to test the proposed system in landmark prediction and detection. Moreover, this study integrates the Grad-CAM (Gradient-Weighted Class Activation Mapping) technique to generate heat maps, providing transparent visualization of the regions the model focuses on during its decision-making process. By enhancing the interpretability of deep learning predictions, Grad-CAM strengthens clinical confidence in the system’s outputs, ensuring that ROI detection aligns with orthodontic diagnostic standards. This explainability is crucial in medical AI applications, where understanding model behavior is as important as achieving high accuracy. The experimental results achieved an accuracy exceeding 98.9%. This research evaluates and differentiates between the two-dimensional and the three-dimensional tracing analyses applied to measurements based on the practices of the European Board of Orthodontics. The results demonstrate the proposed methodology’s robustness when applied to cephalometric images. Furthermore, the evaluation of 3D analysis usage provides a clear understanding of the significance of integrated deep-learning techniques in orthodontics.
Full article
(This article belongs to the Special Issue Machine Learning Applications in Pattern Recognition)
►▼
Show Figures
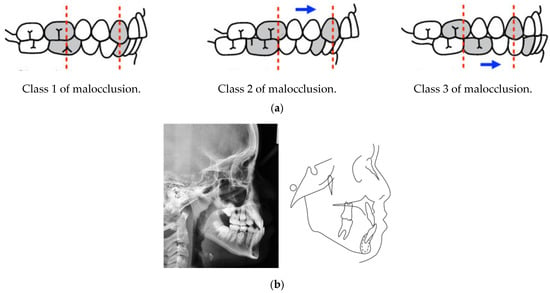
Figure 1
Open AccessArticle
Evaluating the Predictive Power of Software Metrics for Fault Localization
by
Issar Arab, Kenneth Magel and Mohammed Akour
Computers 2025, 14(6), 222; https://doi.org/10.3390/computers14060222 - 6 Jun 2025
Abstract
Fault localization remains a critical challenge in software engineering, directly impacting debugging efficiency and software quality. This study investigates the predictive power of various software metrics for fault localization by framing the task as a multi-class classification problem and evaluating it using the
[...] Read more.
Fault localization remains a critical challenge in software engineering, directly impacting debugging efficiency and software quality. This study investigates the predictive power of various software metrics for fault localization by framing the task as a multi-class classification problem and evaluating it using the Defects4J dataset. We fitted thousands of models and benchmarked different algorithms—including deep learning, Random Forest, XGBoost, and LightGBM—to choose the best-performing model. To enhance model transparency, we applied explainable AI techniques to analyze feature importance. The results revealed that test suite metrics consistently outperform static and dynamic metrics, making them the most effective predictors for identifying faulty classes. These findings underscore the critical role of test quality and coverage in automated fault localization. By combining machine learning with transparent feature analysis, this work delivers practical insights to support more efficient debugging workflows. It lays the groundwork for an iterative process that integrates metric-based predictive models with large language models (LLMs), enabling future systems to automatically generate targeted test cases for the most fault-prone components, which further enhances the automation and precision of software testing.
Full article
(This article belongs to the Special Issue Best Practices, Challenges and Opportunities in Software Engineering)
►▼
Show Figures
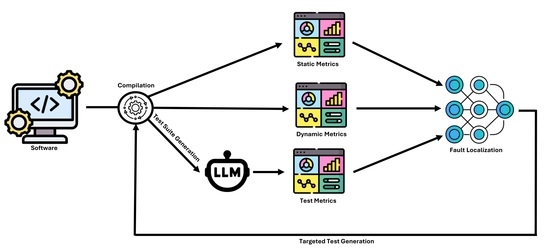
Graphical abstract
Open AccessArticle
Reducing Delivery Times by Utilising On-Site Wire Arc Additive Manufacturing with Digital-Twin Methods
by
Stefanie Sell, Kevin Villani and Marc Stautner
Computers 2025, 14(6), 221; https://doi.org/10.3390/computers14060221 - 6 Jun 2025
Abstract
The increasing demand for smaller batch sizes and mass customisation in production poses considerable challenges to logistics and manufacturing efficiency. Conventional methodologies are unable to address the need for expeditious, cost-effective distribution of premium-quality products tailored to individual specifications. Additionally, the reliability and
[...] Read more.
The increasing demand for smaller batch sizes and mass customisation in production poses considerable challenges to logistics and manufacturing efficiency. Conventional methodologies are unable to address the need for expeditious, cost-effective distribution of premium-quality products tailored to individual specifications. Additionally, the reliability and resilience of global logistics chains are increasingly under pressure. Additive manufacturing is regarded as a potentially viable solution to these problems, as it enables on-demand, on-site production, with reduced resource usage in production. Nevertheless, there are still significant challenges to be addressed, including the assurance of product quality and the optimisation of production processes with respect to time and resource efficiency. This article examines the potential of integrating digital twin methodologies to establish a fully digital and efficient process chain for on-site additive manufacturing. This study focuses on wire arc additive manufacturing (WAAM), a technology that has been successfully implemented in the on-site production of naval ship propellers and excavator parts. The proposed approach aims to enhance process planning efficiency, reduce material and energy consumption, and minimise the expertise required for operational deployment by leveraging digital twin methodologies. The present paper details the current state of research in this domain and outlines a vision for a fully virtualised process chain, highlighting the transformative potential of digital twin technologies in advancing on-site additive manufacturing. In this context, various aspects and components of a digital twin framework for wire arc additive manufacturing are examined regarding their necessity and applicability. The overarching objective of this paper is to conduct a preliminary investigation for the implementation and further development of a comprehensive DT framework for WAAM. Utilising a real-world sample, current already available process steps are validated and actual missing technical solutions are pointed out.
Full article
(This article belongs to the Section Internet of Things (IoT) and Industrial IoT)
►▼
Show Figures
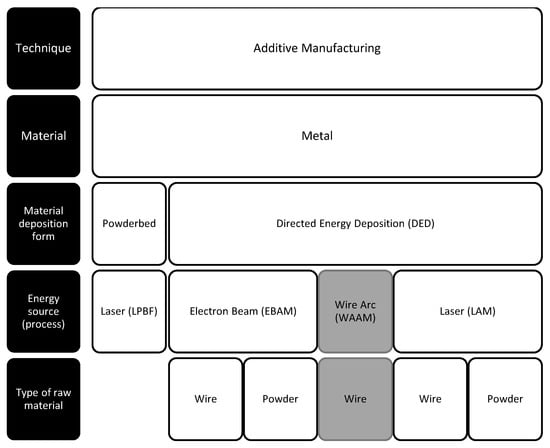
Figure 1
Open AccessArticle
GARMT: Grouping-Based Association Rule Mining to Predict Future Tables in Database Queries
by
Peixiong He, Libo Sun, Xian Gao, Yi Zhou and Xiao Qin
Computers 2025, 14(6), 220; https://doi.org/10.3390/computers14060220 - 6 Jun 2025
Abstract
In modern data management systems, structured query language (SQL) databases, as a mature and stable technology, have become the standard for processing structured data. These databases ensure data integrity through strongly typed schema definitions and support complex transaction management and efficient query processing
[...] Read more.
In modern data management systems, structured query language (SQL) databases, as a mature and stable technology, have become the standard for processing structured data. These databases ensure data integrity through strongly typed schema definitions and support complex transaction management and efficient query processing capabilities. However, data sparsity—where most fields in large table sets remain unused by most queries—leads to inefficiencies in access optimization. We propose a grouping-based approach (GARMT) that partitions SQL queries into fixed-size groups and applies a modified FP-Growth algorithm (GFP-Growth) to identify frequent table access patterns. Experiments on a real-world dataset show that grouping significantly reduces runtime—by up to 40%—compared to the ungrouped baseline while preserving rule relevance. These results highlight the practical value of query grouping for efficient pattern discovery in sparse database environments.
Full article
(This article belongs to the Special Issue Cloud Computing and Big Data Mining)
►▼
Show Figures
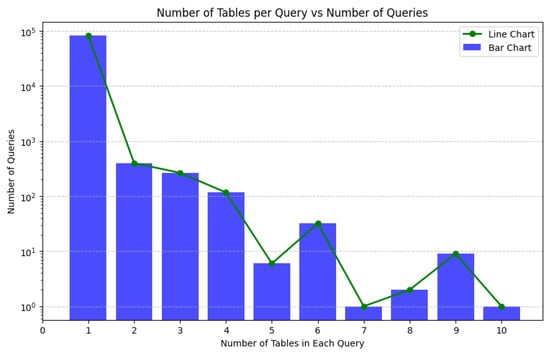
Figure 1
Open AccessArticle
Threats to the Digital Ecosystem: Can Information Security Management Frameworks, Guided by Criminological Literature, Effectively Prevent Cybercrime and Protect Public Data?
by
Shahrukh Mushtaq and Mahmood Shah
Computers 2025, 14(6), 219; https://doi.org/10.3390/computers14060219 - 4 Jun 2025
Abstract
As cyber threats escalate in scale and sophistication, the imperative to secure public data through theoretically grounded and practically viable frameworks becomes increasingly urgent. This review investigates whether and how criminology theories have effectively informed the development and implementation of information security management
[...] Read more.
As cyber threats escalate in scale and sophistication, the imperative to secure public data through theoretically grounded and practically viable frameworks becomes increasingly urgent. This review investigates whether and how criminology theories have effectively informed the development and implementation of information security management frameworks (ISMFs) to prevent cybercrime and fortify the digital ecosystem’s resilience. Anchored in a comprehensive bibliometric analysis of 617 peer-reviewed records extracted from Scopus and Web of Science, the study employs Multiple Correspondence Analysis (MCA), conceptual co-word mapping, and citation coupling to systematically chart the intellectual landscape bridging criminology and cybersecurity. The review reveals those foundational criminology theories—particularly routine activity theory, rational choice theory, and deterrence theory—have been progressively adapted to cyber contexts, offering novel insights into offender behaviour, target vulnerability, and systemic guardianship. In parallel, the study critically engages with global cybersecurity standards such as National Institute of Standards and Technology (NIST) and ISO, to evaluate how criminological principles are embedded in practice. Using data from the Global Cybersecurity Index (GCI), the paper introduces an innovative visual mapping of the divergence between cybersecurity preparedness and digital development across 170+ countries, revealing strategic gaps and overperformers. This paper ultimately argues for an interdisciplinary convergence between criminology and cybersecurity governance, proposing that the integration of criminological logic into cybersecurity frameworks can enhance risk anticipation, attacker deterrence, and the overall security posture of digital public infrastructures.
Full article
(This article belongs to the Special Issue Using New Technologies in Cyber Security Solutions (2nd Edition))
►▼
Show Figures
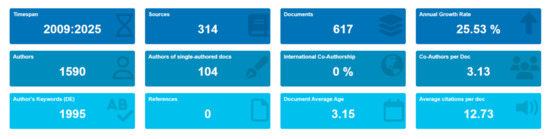
Figure 1
Open AccessArticle
Exploring the Potential of the Bicameral Mind Theory in Reinforcement Learning Algorithms
by
Munavvarkhon Mukhitdinova and Mariana Petrova
Computers 2025, 14(6), 218; https://doi.org/10.3390/computers14060218 - 3 Jun 2025
Abstract
This study explores the potential of Julian Jaynes’ bicameral mind theory in enhancing reinforcement learning (RL) algorithms and large language models (LLMs) for artificial intelligence (AI) systems. By drawing parallels between the dual-process structure of the bicameral mind, the observation–action cycle in RL,
[...] Read more.
This study explores the potential of Julian Jaynes’ bicameral mind theory in enhancing reinforcement learning (RL) algorithms and large language models (LLMs) for artificial intelligence (AI) systems. By drawing parallels between the dual-process structure of the bicameral mind, the observation–action cycle in RL, and the “thinking”/”writing” processes in LLMs, we hypothesize that incorporating principles from this theory could lead to more efficient and adaptive AI. Empirical evidence from OpenAI’s CoinRun and RainMazes models, together with analysis of Claude, Gemini, and ChatGPT functioning, supports our hypothesis, demonstrating the universality of the dual-component structure across different types of AI systems. We propose a conceptual model for integrating bicameral mind principles into AI architectures capable of guiding the development of systems that effectively generalize knowledge across various tasks and environments.
Full article
(This article belongs to the Special Issue Emerging Trends in Machine Learning and Artificial Intelligence)
►▼
Show Figures
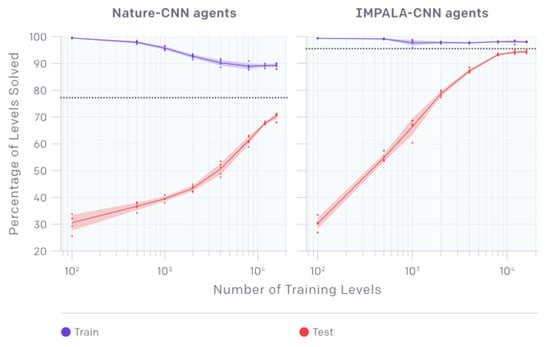
Figure 1
Open AccessSystematic Review
A Systematic Literature Review on Load-Balancing Techniques in Fog Computing: Architectures, Strategies, and Emerging Trends
by
Danah Aldossary, Ezaz Aldahasi, Taghreed Balharith and Tarek Helmy
Computers 2025, 14(6), 217; https://doi.org/10.3390/computers14060217 - 2 Jun 2025
Abstract
Fog computing has emerged as a promising paradigm to extend cloud services toward the edge of the network, enabling low-latency processing and real-time responsiveness for Internet of Things (IoT) applications. However, the distributed, heterogeneous, and resource-constrained nature of fog environments introduces significant challenges
[...] Read more.
Fog computing has emerged as a promising paradigm to extend cloud services toward the edge of the network, enabling low-latency processing and real-time responsiveness for Internet of Things (IoT) applications. However, the distributed, heterogeneous, and resource-constrained nature of fog environments introduces significant challenges in balancing workloads efficiently. This study presents a systematic literature review (SLR) of 113 peer-reviewed articles published between 2020 and 2024, aiming to provide a comprehensive overview of load-balancing strategies in fog computing. This review categorizes fog computing architectures, load-balancing algorithms, scheduling and offloading techniques, fault-tolerance mechanisms, security models, and evaluation metrics. The analysis reveals that three-layer (IoT–Fog–Cloud) architectures remain predominant, with dynamic clustering and virtualization commonly employed to enhance adaptability. Heuristic and hybrid load-balancing approaches are most widely adopted due to their scalability and flexibility. Evaluation frequently centers on latency, energy consumption, and resource utilization, while simulation is primarily conducted using tools such as iFogSim and YAFS. Despite considerable progress, key challenges persist, including workload diversity, security enforcement, and real-time decision-making under dynamic conditions. Emerging trends highlight the growing use of artificial intelligence, software-defined networking, and blockchain to support intelligent, secure, and autonomous load balancing. This review synthesizes current research directions, identifies critical gaps, and offers recommendations for designing efficient and resilient fog-based load-balancing systems.
Full article
(This article belongs to the Special Issue Edge and Fog Computing for Internet of Things Systems (2nd Edition))
►▼
Show Figures
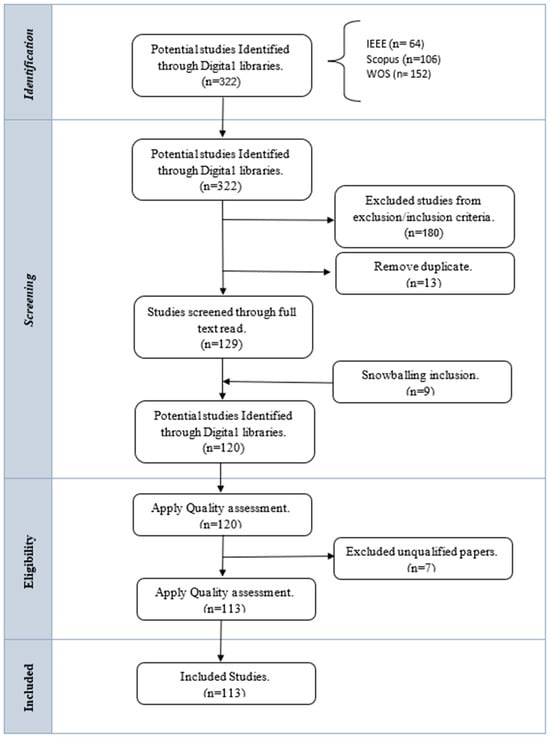
Figure 1
Open AccessArticle
Towards Trustworthy Energy Efficient P2P Networks: A New Method for Validating Computing Results in Decentralized Networks
by
Fernando Rodríguez-Sela and Borja Bordel
Computers 2025, 14(6), 216; https://doi.org/10.3390/computers14060216 - 2 Jun 2025
Abstract
Decentralized P2P networks have emerged as robust instruments to execute computing tasks, with enhanced security and transparency. Solutions such as Blockchain have proved to be successful in a large catalog of critical applications such as cryptocurrency, intellectual property, etc. However, although executions are
[...] Read more.
Decentralized P2P networks have emerged as robust instruments to execute computing tasks, with enhanced security and transparency. Solutions such as Blockchain have proved to be successful in a large catalog of critical applications such as cryptocurrency, intellectual property, etc. However, although executions are transparent and P2P are resistant against common cyberattacks, they tend to be untrustworthy. P2P nodes typically do not offer any evidence about the quality of their resolution of the delegated computing tasks, so trustworthiness of results is threatened. To mitigate this challenge, in usual P2P networks, many different replicas of the same computing task are delegated to different nodes. The final result is the one most nodes reached. But this approach is very resource consuming, especially in terms of energy, as many unnecessary computing tasks are executed. Therefore, new solutions to achieve trustworthy P2P networks, but with an energy efficiency perspective, are needed. This study addresses this challenge. The purpose of the research is to evaluate the effectiveness of an audit-based and score-based approach is assigned to each node instead of performing identical tasks redundantly on different nodes in the network. The proposed solution employs probabilistic methods to detect the malicious nodes taking into account parameters like number of executed tasks and number of audited ones giving a value to the node, and game theory which consider that all nodes play with the same rules. Qualitative and quantitative experimental methods are used to evaluate its impact. The results reveal a significant reduction in network energy consumption, minimum a 50% comparing to networks in which each task is delivered to different nodes considering the task is delivered to a pair of nodes, supporting the effectiveness of the proposed approach.
Full article
(This article belongs to the Section Blockchain Infrastructures and Enabled Applications)
►▼
Show Figures
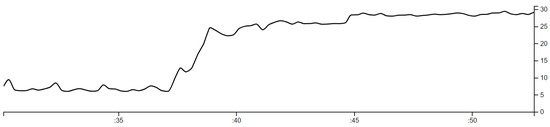
Figure 1
Open AccessArticle
Enhancing Injector Performance Through CFD Optimization: Focus on Cavitation Reduction
by
Jose Villagomez-Moreno, Aurelio Dominguez-Gonzalez, Carlos Gustavo Manriquez-Padilla, Juan Jose Saucedo-Dorantes and Angel Perez-Cruz
Computers 2025, 14(6), 215; https://doi.org/10.3390/computers14060215 - 2 Jun 2025
Abstract
►▼
Show Figures
The use of computer-aided engineering (CAE) tools has become essential in modern design processes, significantly streamlining mechanical design tasks. The integration of optimization algorithms further enhances these processes by facilitating studies on mechanical behavior and accelerating iterative operations. A key focus lies in
[...] Read more.
The use of computer-aided engineering (CAE) tools has become essential in modern design processes, significantly streamlining mechanical design tasks. The integration of optimization algorithms further enhances these processes by facilitating studies on mechanical behavior and accelerating iterative operations. A key focus lies in understanding and mitigating the detrimental effects of cavitation on injector surfaces, as it can reduce the injector lifespan and induce material degradation. By combining advanced numerical finite element tools with algorithmic optimization, these adverse effects can be effectively mitigated. The incorporation of computational tools enables efficient numerical analyses and rapid, automated modifications of injector designs, significantly enhancing the ability to explore and refine geometries. The primary goal remains the minimization of cavitation phenomena and the improvement in injector performance, while the collaborative use of specialized software environments ensures a more robust and streamlined design process. Specifically, using the simulated annealing algorithm (SA) helps identify the optimal configuration that minimizes cavitation-induced effects. The proposed approach provides a robust set of tools for engineers and researchers to enhance injector performance and effectively address cavitation-related challenges. The results derived from this integrated framework illustrate the effectiveness of the optimization methodology in facilitating the development of more efficient and reliable injector systems.
Full article
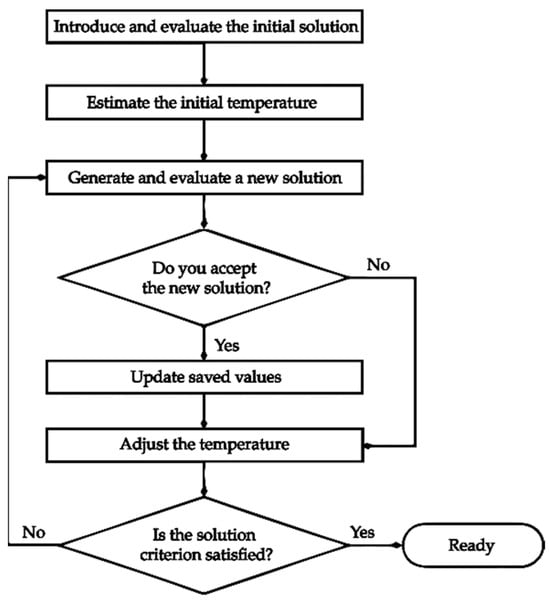
Figure 1
Open AccessArticle
Improved Big Data Security Using Quantum Chaotic Map of Key Sequence
by
Archana Kotangale, Meesala Sudhir Kumar and Amol P. Bhagat
Computers 2025, 14(6), 214; https://doi.org/10.3390/computers14060214 - 1 Jun 2025
Abstract
In the era of ubiquitous big data, ensuring secure storage, transmission, and processing has become a paramount concern. Classical cryptographic methods face increasing vulnerabilities in the face of quantum computing advancements. This research proposes an enhanced big data security framework integrating a quantum
[...] Read more.
In the era of ubiquitous big data, ensuring secure storage, transmission, and processing has become a paramount concern. Classical cryptographic methods face increasing vulnerabilities in the face of quantum computing advancements. This research proposes an enhanced big data security framework integrating a quantum chaotic map of key sequence (QCMKS), which synergizes the principles of quantum mechanics and chaos theory to generate highly unpredictable and non-repetitive key sequences. The system incorporates quantum random number generation (QRNG) for true entropy sources, quantum key distribution (QKD) for secure key exchange immune to eavesdropping, and quantum error correction (QEC) to maintain integrity against quantum noise. Additionally, quantum optical elements transformation (QOET) is employed to implement state transformations on photonic qubits, ensuring robustness during transmission across quantum networks. The integration of QCMKS with QRNG, QKD, QEC, and QOET significantly enhances the confidentiality, integrity, and availability of big data systems, laying the groundwork for a quantum-resilient data security paradigm. While the proposed framework demonstrates strong theoretical potential for improving big data security, its practical robustness and performance are subject to current quantum hardware limitations, noise sensitivity, and integration complexities.
Full article
(This article belongs to the Section ICT Infrastructures for Cybersecurity)
►▼
Show Figures
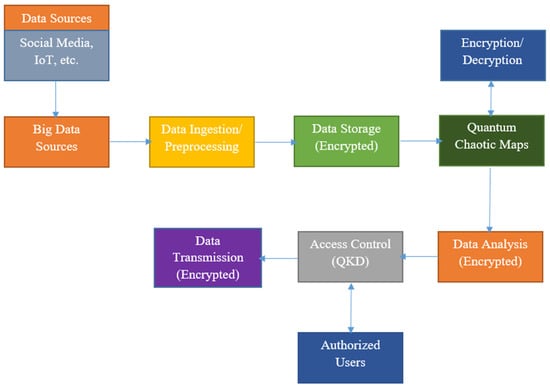
Figure 1
Open AccessArticle
Generating Accessible Webpages from Models
by
Karla Ordoñez-Briceño, José R. Hilera, Luis De-Marcos and Rodrigo Saraguro-Bravo
Computers 2025, 14(6), 213; https://doi.org/10.3390/computers14060213 - 31 May 2025
Abstract
►▼
Show Figures
Despite significant efforts to promote web accessibility through the adoption of various standards and tools, the web remains inaccessible to many users. One of the main barriers is the limited knowledge of accessibility issues among website designers. This gap in expertise results in
[...] Read more.
Despite significant efforts to promote web accessibility through the adoption of various standards and tools, the web remains inaccessible to many users. One of the main barriers is the limited knowledge of accessibility issues among website designers. This gap in expertise results in the development of websites that fail to meet accessibility standards, hindering access for people with diverse abilities and needs. In response to this challenge, this paper presents the ACG WebAcc prototype, which enables the automatic generation of accessible HTML code using a model-driven development (MDD) approach. The tool takes as input a Unified Modeling Language (UML) model, with a specific profile, and incorporates predefined Object Constraint Language (OCL) rules to ensure compliance with accessibility guidelines. By automating this process, ACG WebAcc reduces the need for extensive knowledge of accessibility standards, making it easier for designers to create accessible websites.
Full article
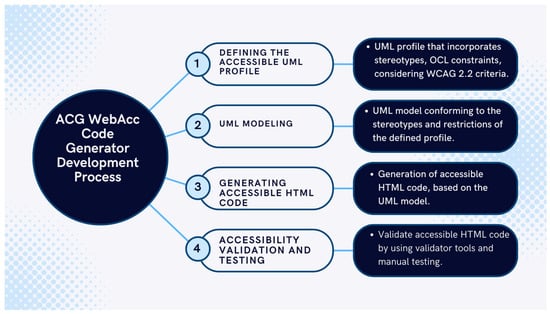
Figure 1
Open AccessArticle
Pain Level Classification Using Eye-Tracking Metrics and Machine Learning Models
by
Oussama El Othmani and Sami Naouali
Computers 2025, 14(6), 212; https://doi.org/10.3390/computers14060212 - 30 May 2025
Abstract
Pain estimation is a critical aspect of healthcare, particularly for patients who are unable to communicate discomfort effectively. The traditional methods, such as self-reporting or observational scales, are subjective and prone to bias. This study proposes a novel system for non-invasive pain estimation
[...] Read more.
Pain estimation is a critical aspect of healthcare, particularly for patients who are unable to communicate discomfort effectively. The traditional methods, such as self-reporting or observational scales, are subjective and prone to bias. This study proposes a novel system for non-invasive pain estimation using eye-tracking technology and advanced machine learning models. The methodology begins with preprocessing steps, including resizing, normalization, and data augmentation, to prepare high-quality input face images. DeepLabV3+ is employed for the precise segmentation of the eye and face regions, achieving 95% accuracy. Feature extraction is performed using VGG16, capturing key metrics such as pupil size, blink rate, and saccade velocity. Multiple machine learning models, including Random Forest, SVM, MLP, XGBoost, and NGBoost, are trained on the extracted features. XGBoost achieves the highest classification accuracy of 99.5%, demonstrating its robustness for pain level classification on a scale from 0 to 5. The feature analysis using SHAP values reveals that pupil size and blink rate contribute most to the predictions, with SHAP contribution scores of 0.42 and 0.35, respectively. The loss curves for DeepLabV3+ confirm rapid convergence during training, ensuring reliable segmentation. This work highlights the transformative potential of combining eye-tracking data with machine learning for non-invasive pain estimation, with significant applications in healthcare, human–computer interaction, and assistive technologies.
Full article
(This article belongs to the Topic Visual Computing and Understanding: New Developments and Trends)
►▼
Show Figures
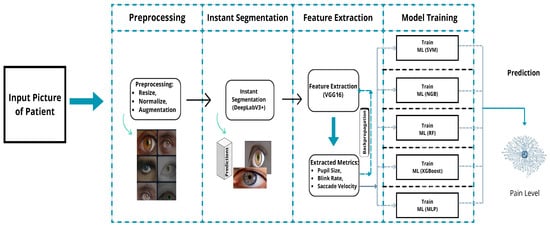
Figure 1
Open AccessArticle
Enhancing User Experiences in Digital Marketing Through Machine Learning: Cases, Trends, and Challenges
by
Alexios Kaponis, Manolis Maragoudakis and Konstantinos Chrysanthos Sofianos
Computers 2025, 14(6), 211; https://doi.org/10.3390/computers14060211 - 29 May 2025
Abstract
Online marketing environments are rapidly being transformed by Artificial Intelligence (AI). This represents the implementation of Machine Learning (ML) that has significant potential in content personalization, enhanced usability, and hyper-targeted marketing, and it will reconfigure how businesses reach and serve customers. This systematic
[...] Read more.
Online marketing environments are rapidly being transformed by Artificial Intelligence (AI). This represents the implementation of Machine Learning (ML) that has significant potential in content personalization, enhanced usability, and hyper-targeted marketing, and it will reconfigure how businesses reach and serve customers. This systematic examination of machine learning in the Digital Marketing (DM) industry is also closely examined, focusing on its effect on human–computer interaction (HCI). This research methodically elucidates how machine learning can be applied to the automation of strategies for user engagement that increase user experience (UX) and customer retention, and how to optimize recommendations from consumer behavior. The objective of the present study is to critically analyze the functional and ethical considerations of ML integration in DM and to evaluate its implications on data-driven personalization. Through selected case studies, the investigation also provides empirical evidence of the implications of ML applications on UX/customer loyalty as well as associated ethical aspects. These include algorithmic bias, concerns about the privacy of the data, and the need for greater transparency of ML-based decision-making processes. This research also contributes to the field by delivering actionable, data-driven strategies for marketing professionals and offering them frameworks to deal with the evolving responsibilities and tasks that accompany the introduction of ML technologies into DM.
Full article
(This article belongs to the Special Issue Harnessing Artificial Intelligence for Social and Semantic Understanding)
►▼
Show Figures
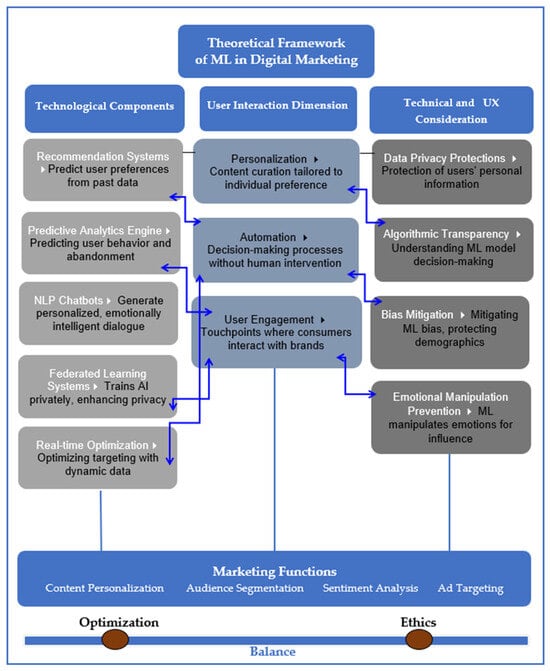
Figure 1
Highly Accessed Articles
Latest Books
E-Mail Alert
News
Topics
Topic in
Applied Sciences, Computers, Electronics, Sensors, Virtual Worlds, IJGI
Simulations and Applications of Augmented and Virtual Reality, 2nd Edition
Topic Editors: Radu Comes, Dorin-Mircea Popovici, Calin Gheorghe Dan Neamtu, Jing-Jing FangDeadline: 20 June 2025
Topic in
Applied Sciences, Automation, Computers, Electronics, Sensors, JCP, Mathematics
Intelligent Optimization, Decision-Making and Privacy Preservation in Cyber–Physical Systems
Topic Editors: Lijuan Zha, Jinliang Liu, Jian LiuDeadline: 31 August 2025
Topic in
Animals, Computers, Information, J. Imaging, Veterinary Sciences
AI, Deep Learning, and Machine Learning in Veterinary Science Imaging
Topic Editors: Vitor Filipe, Lio Gonçalves, Mário GinjaDeadline: 31 October 2025
Topic in
Applied Sciences, Computers, Electronics, JSAN, Technologies
Emerging AI+X Technologies and Applications
Topic Editors: Byung-Seo Kim, Hyunsik Ahn, Kyu-Tae LeeDeadline: 31 December 2025

Conferences
Special Issues
Special Issue in
Computers
Harnessing the Blockchain Technology in Unveiling Futuristic Applications
Guest Editors: Raman Singh, Shantanu PalDeadline: 15 June 2025
Special Issue in
Computers
When Natural Language Processing Meets Machine Learning—Opportunities, Challenges and Solutions
Guest Editors: Lu Bai, Huiru Zheng, Zhibao WangDeadline: 30 June 2025
Special Issue in
Computers
Intelligent Edge: When AI Meets Edge Computing
Guest Editor: Riduan AbidDeadline: 30 June 2025
Special Issue in
Computers
Future Systems Based on Healthcare 5.0 for Pandemic Preparedness 2024
Guest Editor: Xuhui ChenDeadline: 30 June 2025