Topic Menu
► Topic MenuTopic Editors

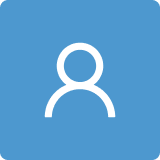
AI for Natural Disasters Detection, Prediction and Modeling
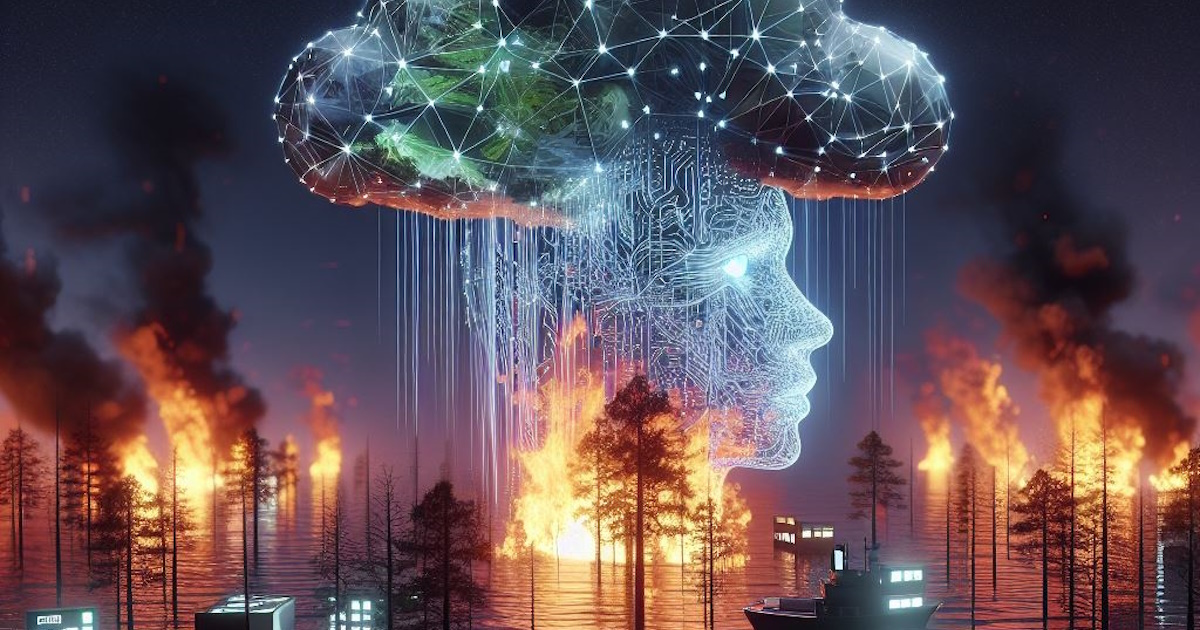
Topic Information
Dear Colleagues,
In recent years, we have witnessed escalating climate change and its increasing impact on global ecosystems, human lives, and the world economy. This situation calls for advanced tools that can leverage artificial intelligence (AI) for the early detection, prediction, and modeling of natural disasters. The increasing frequency and intensity of events such as wildfires, flooding, storms, and other catastrophic incidents necessitate innovative approaches for mitigation and response. This call for papers invites contributions that address the critical aspects of this interesting field, focusing on the integration of AI methodologies with remote sensing data. We encourage submissions that span a wide range of topics, including reviews of state-of-the-art AI applications for natural disaster management, risk assessment and hazard prediction; the use of AI to detect and track specific events; modeling techniques employing AI; and the development of advanced forecasting models utilizing AI methodologies.
The aim of this call is to bring together researchers and experts from various areas to foster collaborative efforts in developing cutting-edge solutions that will enhance our ability to anticipate, understand, and respond to the increasing challenges posed by natural disasters in an era of climate change.
Dr. Moulay A. Akhloufi
Dr. Mozhdeh Shahbazi
Topic Editors
Keywords
- AI for natural disasters
- forest fires, flooding, storms, earthquakes
- forest monitoring, environmental monitoring, natural risks
- forecasting models, mitigation, and response
- earth observation, remote sensing
- multispectral, hyperspectral, LiDAR, photogrammetry
- machine learning, deep learning, data fusion, image processing
- mapping, modelling, digital twins
Participating Journals
Journal Name | Impact Factor | CiteScore | Launched Year | First Decision (median) | APC | |
---|---|---|---|---|---|---|
![]()
AI
|
5.0 | 6.9 | 2020 | 20.7 Days | CHF 1600 | Submit |
![]()
Big Data and Cognitive Computing
|
4.4 | 9.8 | 2017 | 24.5 Days | CHF 1800 | Submit |
![]()
Fire
|
2.7 | 3.9 | 2018 | 16.5 Days | CHF 2400 | Submit |
![]()
GeoHazards
|
1.6 | 2.2 | 2020 | 17.2 Days | CHF 1400 | Submit |
![]()
Remote Sensing
|
4.1 | 8.6 | 2009 | 24.9 Days | CHF 2700 | Submit |
Preprints.org is a multidisciplinary platform offering a preprint service designed to facilitate the early sharing of your research. It supports and empowers your research journey from the very beginning.
MDPI Topics is collaborating with Preprints.org and has established a direct connection between MDPI journals and the platform. Authors are encouraged to take advantage of this opportunity by posting their preprints at Preprints.org prior to publication:
- Share your research immediately: disseminate your ideas prior to publication and establish priority for your work.
- Safeguard your intellectual contribution: Protect your ideas with a time-stamped preprint that serves as proof of your research timeline.
- Boost visibility and impact: Increase the reach and influence of your research by making it accessible to a global audience.
- Gain early feedback: Receive valuable input and insights from peers before submitting to a journal.
- Ensure broad indexing: Web of Science (Preprint Citation Index), Google Scholar, Crossref, SHARE, PrePubMed, Scilit and Europe PMC.