Topic Editors
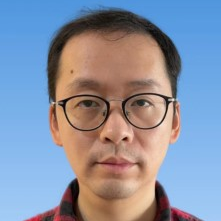

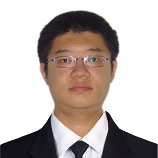
Bioinformatics in Drug Design and Discovery, 2nd Volume
Topic Information
Dear Colleagues,
With the development of modern sequencing technology, this decade has witnessed the expansion of huge biomedical data advances which has opened a new window for the clinical diagnoses and therapeutics of complex disease. Bioinformatics can extract, analyze, and communicate hidden information from sequences and structures as well as functional knowledge of nucleic acids and proteins in order to discover and identify new drug targets. This can potentially guide the design of therapeutic drugs that can activate or block the biological functions of biomolecules and help to construct various prediction models to aid virtual bioactive screening. This will, in turn, help to design and discover safer and more efficient therapeutic drugs that can either activate or block the biological functions of biomolecules.
Thus, there is a need to fundamentally address all of the above-mentioned issues in the application of bioinformatics techniques and the development of novel drugs. Here, we seek original research papers and reviews for a Special Issue on the theme of bioinformatics in drug design and discovery. Dr. Bing Niu Dr. Suren Rao Sooranna Dr. Pufeng Du Topic Editors
Dr. Bing Niu
Dr. Suren Rao Sooranna
Dr. Pufeng Du
Topic Editors
Keywords
- machine learning
- molecule simulation
- deep learning
- sequencing analysis
- drug–target interaction
- virtual screening
- de novo drug design
- benchmark databases
- big data
- artificial intelligent techniques
- pharmacophore technology
- quantitative structure-activity relationships
Participating Journals
Journal Name | Impact Factor | CiteScore | Launched Year | First Decision (median) | APC | |
---|---|---|---|---|---|---|
![]()
Biomolecules
|
5.5 | 8.3 | 2011 | 16.9 Days | CHF 2700 | Submit |
![]()
International Journal of Molecular Sciences
|
5.6 | 7.8 | 2000 | 16.3 Days | CHF 2900 | Submit |
![]()
Marine Drugs
|
5.4 | 9.6 | 2003 | 14 Days | CHF 2900 | Submit |
![]()
Molecules
|
4.6 | 6.7 | 1996 | 14.6 Days | CHF 2700 | Submit |
![]()
Scientia Pharmaceutica
|
2.5 | 6.4 | 1930 | 22.7 Days | CHF 1000 | Submit |
![]()
Genes
|
3.5 | 5.1 | 2010 | 16.5 Days | CHF 2600 | Submit |
![]()
Pharmaceutics
|
5.4 | 6.9 | 2009 | 14.2 Days | CHF 2900 | Submit |
![]()
Crystals
|
2.7 | 3.6 | 2011 | 10.6 Days | CHF 2600 | Submit |
MDPI Topics is cooperating with Preprints.org and has built a direct connection between MDPI journals and Preprints.org. Authors are encouraged to enjoy the benefits by posting a preprint at Preprints.org prior to publication:
- Immediately share your ideas ahead of publication and establish your research priority;
- Protect your idea from being stolen with this time-stamped preprint article;
- Enhance the exposure and impact of your research;
- Receive feedback from your peers in advance;
- Have it indexed in Web of Science (Preprint Citation Index), Google Scholar, Crossref, SHARE, PrePubMed, Scilit and Europe PMC.
Related Topic
- Bioinformatics in Drug Design and Discovery (15 articles)