Topic Menu
► Topic MenuTopic Editors
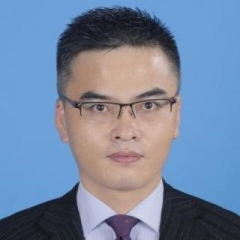
Robotic Intelligent Machining System
Topic Information
Dear Colleagues,
Robotic intelligent machining systems have been used for various applications in production systems because they have many advantages, such as large workspaces, a high degree of freedom, flexibility, and cost-effectiveness. Compared to dedicated machine tools, industrial robots have large workspaces that can easily be extended by the addition of stages or mobile platforms. Robotic machining can be highly flexible because robots can be adjusted for a variety of machining processes by changing the end-effectors or tools attached to the manipulator. As robotic machining systems have a higher DOF than machine tools, more complex parts can be machined. Robots can also form work cells with other robots or machine tools. Therefore, the use of robots is increasing as the machining paradigm shifts from mass production to mass customization. Furthermore, the total costs of robotic machining systems are less than those of dedicated machine tools. However, despite the advantages, the implementation of robotic machining systems is still in its infancy due to their low machining accuracy. Although most industrial robots are used for welding and material handling processes, the adoption of robots for other machining processes has increased. Thus, substantial research has been carried out to analyze and reduce robotic machining errors and improve the performance of robotic machining systems.
Robotic intelligent machining systems, which use the industrial robot as the actuator, multi-source sensors (such as vision, force, and vibration) as the perception system, and machine learning and intelligent cloud platform as the decision system, can complete anthropomorphic manufacturing tasks according to incomplete, inaccurate information on the working condition without certainty, prior knowledge, or a prediction model. In addition, the intelligent robot machining system can combine the monitoring data with the theoretical model to achieve higher accuracy and efficiency in manufacturing tasks.
The aim of this topic is to present an overview of the current state of recent research on robotic intelligent machining systems, namely machining process planning and control techniques including the analysis of the robot-workspace, robot trajectory planning, vibration monitoring and control, deformation monitoring and compensation, as well as the principles of these technologies such as robot stiffness characteristics, dynamic characteristics, chatter mechanisms, and deformation mechanisms.
Suggested topics include, but are not limited to:
- Multi-robot collaborative processing of large and complex parts
- Intelligent robot machining of multi-variety and small batch parts
- Robot stiffness and pose planning
- Robot dynamics and trajectory planning
- Robotic milling chatter and suppression
- Improving the machining accuracy of robotic machining systems
- Robot stiffness model and identification method
- Analysis of robot workspace based on stiffness
Dr. Fangchen Yin
Prof. Dr. Changcai Cui
Prof. Dr. Guoqin Huang
Dr. Jingqi Zhang
Topic Editors
Keywords
- robotic machining
- control system design
- intelligent control theory
- industrial robot
- robot dynamics
- intelligent machining
Participating Journals
Journal Name | Impact Factor | CiteScore | Launched Year | First Decision (median) | APC |
---|---|---|---|---|---|
![]()
Applied Sciences
|
2.5 | 5.3 | 2011 | 18.4 Days | CHF 2400 |
![]()
Automation
|
- | 2.9 | 2020 | 24.1 Days | CHF 1000 |
![]()
Machines
|
2.1 | 3.0 | 2013 | 15.5 Days | CHF 2400 |
![]()
Materials
|
3.1 | 5.8 | 2008 | 13.9 Days | CHF 2600 |
![]()
Robotics
|
2.9 | 6.7 | 2012 | 21 Days | CHF 1800 |
![]()
Actuators
|
2.2 | 3.9 | 2012 | 17.7 Days | CHF 2400 |
Preprints.org is a multidisciplinary platform offering a preprint service designed to facilitate the early sharing of your research. It supports and empowers your research journey from the very beginning.
MDPI Topics is collaborating with Preprints.org and has established a direct connection between MDPI journals and the platform. Authors are encouraged to take advantage of this opportunity by posting their preprints at Preprints.org prior to publication:
- Share your research immediately: disseminate your ideas prior to publication and establish priority for your work.
- Safeguard your intellectual contribution: Protect your ideas with a time-stamped preprint that serves as proof of your research timeline.
- Boost visibility and impact: Increase the reach and influence of your research by making it accessible to a global audience.
- Gain early feedback: Receive valuable input and insights from peers before submitting to a journal.
- Ensure broad indexing: Web of Science (Preprint Citation Index), Google Scholar, Crossref, SHARE, PrePubMed, Scilit and Europe PMC.