Applications, Challenges and Promises of Computer Vision and Digital Imaging Processing
A special issue of Applied Sciences (ISSN 2076-3417). This special issue belongs to the section "Computing and Artificial Intelligence".
Deadline for manuscript submissions: closed (10 December 2024) | Viewed by 14616
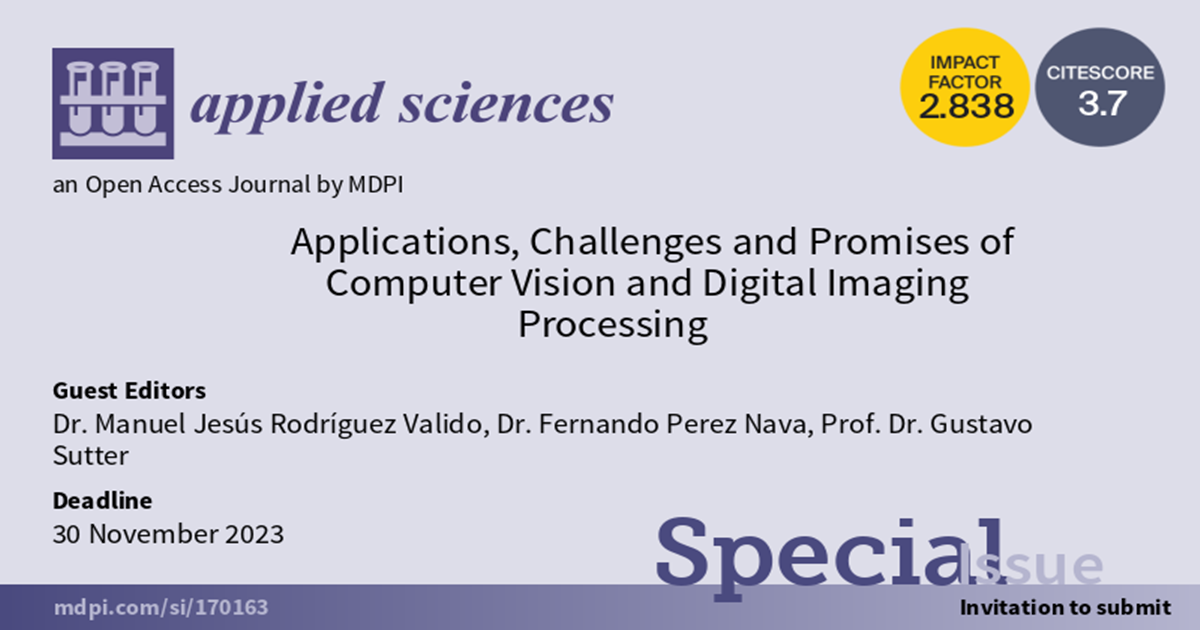
Special Issue Editors
Interests: smart sensor networks; FPGA image processing; Internet of Things; autonomous driving; sustainable electric mobility
Special Issues, Collections and Topics in MDPI journals
Interests: image and video processing; computer vision; virtual reality
Special Issues, Collections and Topics in MDPI journals
Interests: high-performance computing (HPC)
Special Issues, Collections and Topics in MDPI journals
Special Issue Information
Dear Colleagues,
Computer vision has recently experienced a significant increase in its overall adoption, ranging from facial recognition used in smartphones to navigation in self-driving vehicles. Part of this success can be attributed to the integration of techniques from other areas such as Artificial Intelligence (AI) within the field of Image Processing.
Real-world applications demand new ideas and techniques that solve practical problems. Consequently, this Special Issue is intended to present innovative solutions in this field, from their conception and analysis to their implementation.
Topics of interest for this Special Issue include but are not limited to the following:
- Low-Level Vision Techniques
- Detection, Recognition, Classification and Localization in 2D/3D
- Shape estimation
- 3D and Multiview processing and sensors
- Motion and Tracking
- Image and Video Understanding
- Image/Video Synthesis and Generative Models
- Integration of AI techniques
In addition, application areas of interest include but are not limited to:
- Agriculture
- Healthcare
- Manufacturing
- Remote Sensing
- Retail
- Robotics
- Security
- Sports
- Transport
- Virtual Reality
Dr. Manuel Jesús Rodríguez Valido
Dr. Fernando Perez Nava
Prof. Dr. Gustavo Sutter
Guest Editors
Manuscript Submission Information
Manuscripts should be submitted online at www.mdpi.com by registering and logging in to this website. Once you are registered, click here to go to the submission form. Manuscripts can be submitted until the deadline. All submissions that pass pre-check are peer-reviewed. Accepted papers will be published continuously in the journal (as soon as accepted) and will be listed together on the special issue website. Research articles, review articles as well as short communications are invited. For planned papers, a title and short abstract (about 100 words) can be sent to the Editorial Office for announcement on this website.
Submitted manuscripts should not have been published previously, nor be under consideration for publication elsewhere (except conference proceedings papers). All manuscripts are thoroughly refereed through a single-blind peer-review process. A guide for authors and other relevant information for submission of manuscripts is available on the Instructions for Authors page. Applied Sciences is an international peer-reviewed open access semimonthly journal published by MDPI.
Please visit the Instructions for Authors page before submitting a manuscript. The Article Processing Charge (APC) for publication in this open access journal is 2400 CHF (Swiss Francs). Submitted papers should be well formatted and use good English. Authors may use MDPI's English editing service prior to publication or during author revisions.
Keywords
- computer vision
- image and video processing
- image and video understanding
- computer vision applications
- machine learning
- artificial intelligence
Benefits of Publishing in a Special Issue
- Ease of navigation: Grouping papers by topic helps scholars navigate broad scope journals more efficiently.
- Greater discoverability: Special Issues support the reach and impact of scientific research. Articles in Special Issues are more discoverable and cited more frequently.
- Expansion of research network: Special Issues facilitate connections among authors, fostering scientific collaborations.
- External promotion: Articles in Special Issues are often promoted through the journal's social media, increasing their visibility.
- e-Book format: Special Issues with more than 10 articles can be published as dedicated e-books, ensuring wide and rapid dissemination.
Further information on MDPI's Special Issue policies can be found here.