Predictive Analytics and Fault Diagnosis of Machines with Machine Learning Techniques
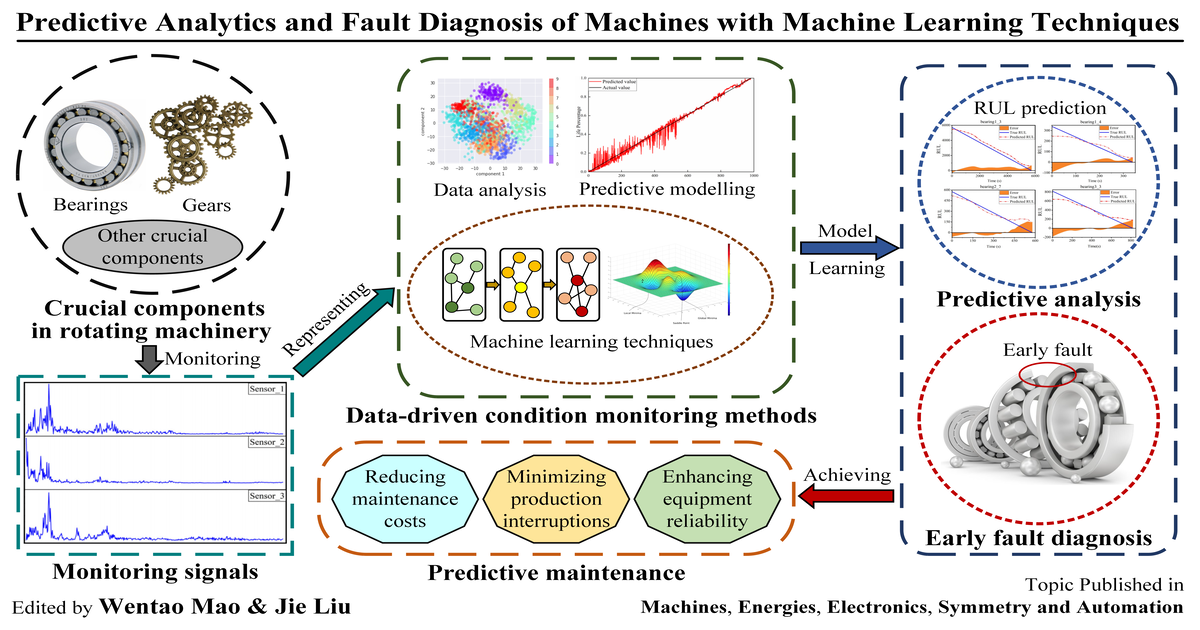
Topic Information
Dear Colleagues,
This Topic focuses on cutting-edge topics in the industrial sector, exploring how predictive analytics and machine fault diagnosis can enhance production efficiency and equipment reliability. With the emergence of Industry 4.0, data-driven decision-making and innovative maintenance strategies have become the cornerstone of the industry. This Topic delves into the methods of employing data analysis, predictive modelling, and machine learning techniques to achieve machine health monitoring and early fault diagnosis, ultimately reducing maintenance costs, minimizing production interruptions, and enhancing equipment reliability. We pay particular attention to critical components in rotating machinery, such as bearings, which play a pivotal role in industrial manufacturing. Through state monitoring and fault diagnosis, alongside emerging technologies like deep learning, we accurately diagnose machine conditions and proactively engage in predictive maintenance. This Topic is designed to foster collaboration between industry and academia, driving innovation in methods and applications to meet the demands of modern industrial production. We eagerly anticipate research findings in this critical field, which will provide the industry with more efficient and reliable production methods.
Prof. Dr. Wentao Mao
Dr. Jie Liu
Topic Editors
Keywords
- fault diagnostics
- remaining useful life prediction
- predictive maintenance
- condition monitoring
- real-time
- machine learning
- deep learning
Participating Journals
Journal Name | Impact Factor | CiteScore | Launched Year | First Decision (median) | APC | |
---|---|---|---|---|---|---|
![]()
Automation
|
- | 2.9 | 2020 | 24.1 Days | CHF 1000 | Submit |
![]()
Electronics
|
2.6 | 5.3 | 2012 | 16.4 Days | CHF 2400 | Submit |
![]()
Energies
|
3.0 | 6.2 | 2008 | 16.8 Days | CHF 2600 | Submit |
![]()
Machines
|
2.1 | 3.0 | 2013 | 15.5 Days | CHF 2400 | Submit |
Preprints.org is a multidisciplinary platform offering a preprint service designed to facilitate the early sharing of your research. It supports and empowers your research journey from the very beginning.
MDPI Topics is collaborating with Preprints.org and has established a direct connection between MDPI journals and the platform. Authors are encouraged to take advantage of this opportunity by posting their preprints at Preprints.org prior to publication:
- Share your research immediately: disseminate your ideas prior to publication and establish priority for your work.
- Safeguard your intellectual contribution: Protect your ideas with a time-stamped preprint that serves as proof of your research timeline.
- Boost visibility and impact: Increase the reach and influence of your research by making it accessible to a global audience.
- Gain early feedback: Receive valuable input and insights from peers before submitting to a journal.
- Ensure broad indexing: Web of Science (Preprint Citation Index), Google Scholar, Crossref, SHARE, PrePubMed, Scilit and Europe PMC.