Topic Menu
► Topic MenuTopic Editors
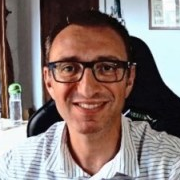


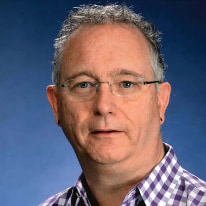
Google Earth Engine Applications for Monitoring Natural Ecosystems and Land Use
Topic Information
Dear Colleagues,
Global ecosystems play a major role in mitigating global warming, but climate change is increasing the number and the magnitude of stressors, making ecosystem monitoring more important than ever. In this context, remote sensing data and the Google Earth Engine cloud computing platform represent crucial tools for comprehensively and exhaustively monitoring ecosystems globally. Google Earth Engine provides access to the vast majority of freely available, public, multi-temporal remote sensing data and offers free cloud-based computational power to apply complex algorithms over large areas.
The Topic “Google Earth Engine Applications for Monitoring Natural Ecosystems and Land Use” welcomes high-quality studies that focus on applications exploiting GEE for monitoring natural ecosystems and land use. Relevant themes include, but are not limited to: (a) ecosystem disturbance near real-time prediction and monitoring, (b) carbon storage prediction, (c) forest species classification, (d) forest harvestings, wind damages, and fires prediction, (e) climate change impact on global ecosystems, (f) drought monitoring, (g) innovative time series analysis and machine learning approaches for ecosystem monitoring, (h) development and validation of ecosystem disturbance monitoring methods, (i) forest degradation monitoring, (j) natural disaster monitoring, (k) precision and accuracy estimation and modeling of forest structure and function parameters, (l) agroforestry ecosystem visualization and management, (m) land cover and land-use change monitoring, and (n) hydrological and eco-hydrological processes monitoring.
Prof. Dr. Gherardo Chirici
Dr. Saverio Francini
Dr. Noel Gorelick
Prof. Dr. Nicholas Coops
Topic Editors
Keywords
- forests
- ecosystems
- land-cover and land-use change
- Google Earth Engine (GEE)
- remote sensing
- hydrology
- artificial intelligence
- big data
- decision making
- carbon storage estimation
- sustainability
- biodiversity
Participating Journals
Journal Name | Impact Factor | CiteScore | Launched Year | First Decision (median) | APC |
---|---|---|---|---|---|
![]()
Agriculture
|
3.3 | 4.9 | 2011 | 19.2 Days | CHF 2600 |
![]()
Earth
|
2.1 | 3.3 | 2020 | 23.7 Days | CHF 1200 |
![]()
Forests
|
2.4 | 4.4 | 2010 | 16.2 Days | CHF 2600 |
![]()
Land
|
3.2 | 4.9 | 2012 | 16.9 Days | CHF 2600 |
![]()
Remote Sensing
|
4.2 | 8.3 | 2009 | 23.9 Days | CHF 2700 |
Preprints.org is a multidisciplinary platform offering a preprint service designed to facilitate the early sharing of your research. It supports and empowers your research journey from the very beginning.
MDPI Topics is collaborating with Preprints.org and has established a direct connection between MDPI journals and the platform. Authors are encouraged to take advantage of this opportunity by posting their preprints at Preprints.org prior to publication:
- Share your research immediately: disseminate your ideas prior to publication and establish priority for your work.
- Safeguard your intellectual contribution: Protect your ideas with a time-stamped preprint that serves as proof of your research timeline.
- Boost visibility and impact: Increase the reach and influence of your research by making it accessible to a global audience.
- Gain early feedback: Receive valuable input and insights from peers before submitting to a journal.
- Ensure broad indexing: Web of Science (Preprint Citation Index), Google Scholar, Crossref, SHARE, PrePubMed, Scilit and Europe PMC.