Journal Description
Journal of Cybersecurity and Privacy
Journal of Cybersecurity and Privacy
is an international, peer-reviewed, open access journal on all aspects of computer, systems, and information security, published quarterly online by MDPI.
- Open Access— free for readers, with article processing charges (APC) paid by authors or their institutions.
- High Visibility: indexed within Scopus, EBSCO, and other databases.
- Rapid Publication: manuscripts are peer-reviewed and a first decision is provided to authors approximately 23.5 days after submission; acceptance to publication is undertaken in 9.8 days (median values for papers published in this journal in the second half of 2023).
- Recognition of Reviewers: APC discount vouchers, optional signed peer review, and reviewer names published annually in the journal.
- Companion journal: Sensors.
Latest Articles
Passwordless Authentication Using a Combination of Cryptography, Steganography, and Biometrics
J. Cybersecur. Priv. 2024, 4(2), 278-297; https://doi.org/10.3390/jcp4020014 (registering DOI) - 01 May 2024
Abstract
User-generated passwords often pose a security risk in authentication systems. However, providing a comparative substitute poses a challenge, given the common tradeoff between security and user experience. This paper integrates cryptographic methods (both asymmetric and symmetric), steganography, and a combination of physiological and
[...] Read more.
User-generated passwords often pose a security risk in authentication systems. However, providing a comparative substitute poses a challenge, given the common tradeoff between security and user experience. This paper integrates cryptographic methods (both asymmetric and symmetric), steganography, and a combination of physiological and behavioural biometrics to construct a prototype for a passwordless authentication system. We demonstrate the feasibility of scalable passwordless authentication while maintaining a balance between usability and security. We employ threat modeling techniques to pinpoint the security prerequisites for the system, along with choosing appropriate cryptographic protocols. In addition, a comparative analysis is conducted, examining the security impacts of the proposed system in contrast to that of traditional password-based systems. The results from the prototype indicate that authentication is possible within a timeframe similar to passwords (within 2 s), without imposing additional hardware costs on users to enhance security or compromising usability. Given the scalable nature of the system design and the elimination of shared secrets, the financial and efficiency burdens associated with password resets are alleviated. Furthermore, the risk of breaches is mitigated as there is no longer a need to store passwords and/or their hashes. Differing from prior research, our study presents a pragmatic design and prototype that deserves consideration as a viable alternative for both password-based and passwordless authentication systems.
Full article
(This article belongs to the Special Issue Cyber Security and Digital Forensics)
►
Show Figures
Open AccessFeature PaperArticle
Facilitating the Integrative Use of Security Knowledge Bases within a Modelling Environment
by
Avi Shaked
J. Cybersecur. Priv. 2024, 4(2), 264-277; https://doi.org/10.3390/jcp4020013 - 20 Apr 2024
Abstract
►▼
Show Figures
Security threat and risk assessment of systems requires the integrated use of information from multiple knowledge bases. Such use is typically carried out ad-hoc by security experts in an unstructured manner. Also, this ad-hoc use of information often lacks foundations that allow for
[...] Read more.
Security threat and risk assessment of systems requires the integrated use of information from multiple knowledge bases. Such use is typically carried out ad-hoc by security experts in an unstructured manner. Also, this ad-hoc use of information often lacks foundations that allow for rigorous, disciplined applications of policy enforcement and the establishment of a well-integrated body of knowledge. This hinders organisational learning as well as the maturation of the threat modelling discipline. In this article, we uncover a newly developed extension of a state-of-the-art modelling tool that allows users to integrate and curate security-related information from multiple knowledge bases. Specifically, we provide catalogues of threats and security controls based on information from CAPEC, ATT&CK, and NIST SP800-53. We demonstrate the ability to curate security information using the designed solution. We highlight the contribution to improving the communication of security information, including the systematic mapping between user-defined security guidance and information derived from knowledge bases. The solution is open source and relies on model-to-model transformations and extendable threat and security control catalogues. Accordingly, the solution allows prospective users to adapt the modelling environment to their needs as well as keep it current with respect to evolving knowledge bases.
Full article
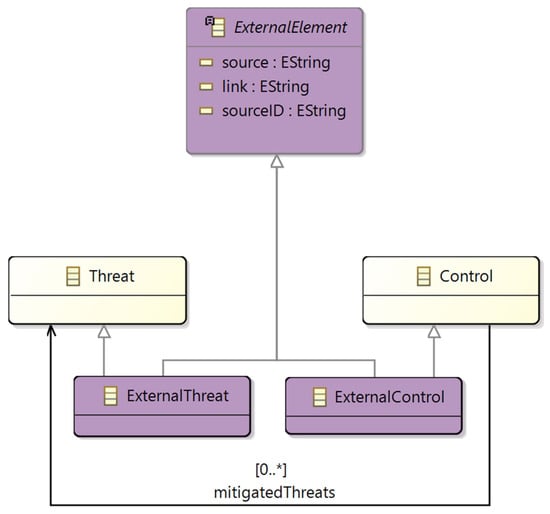
Figure 1
Open AccessArticle
Data-Driven Network Anomaly Detection with Cyber Attack and Defense Visualization
by
Eric Muhati and Danda Rawat
J. Cybersecur. Priv. 2024, 4(2), 241-263; https://doi.org/10.3390/jcp4020012 - 09 Apr 2024
Abstract
The exponential growth in data volumes, combined with the inherent complexity of network algorithms, has drastically affected network security. Data activities are producing voluminous network logs that often mask critical vulnerabilities. Although there are efforts to address these hidden vulnerabilities, the solutions often
[...] Read more.
The exponential growth in data volumes, combined with the inherent complexity of network algorithms, has drastically affected network security. Data activities are producing voluminous network logs that often mask critical vulnerabilities. Although there are efforts to address these hidden vulnerabilities, the solutions often come at high costs or increased complexities. In contrast, the potential of open-source tools, recognized for their security analysis capabilities, remains under-researched. These tools have the potential for detailed extraction of essential network components, and they strengthen network security. Addressing this gap, our paper proposes a data analytics-driven network anomaly detection model, which is uniquely complemented with a visualization layer, making the dynamics of cyberattacks and their subsequent defenses distinctive in near real-time. Our novel approach, based on network scanning tools and network discovery services, allows us to visualize the network based on how many IP-based networking devices are live, then we implement a data analytics-based intrusion detection system that scrutinizes all network connections. We then initiate mitigation measures, visually distinguishing malicious from benign connections using red and blue hues, respectively. Our experimental evaluation shows an score of and a minimal false positive rate of in our model, demonstrating a marked improvement over existing research in this domain.
Full article
(This article belongs to the Special Issue Intrusion, Malware Detection and Prevention in Networks)
►▼
Show Figures
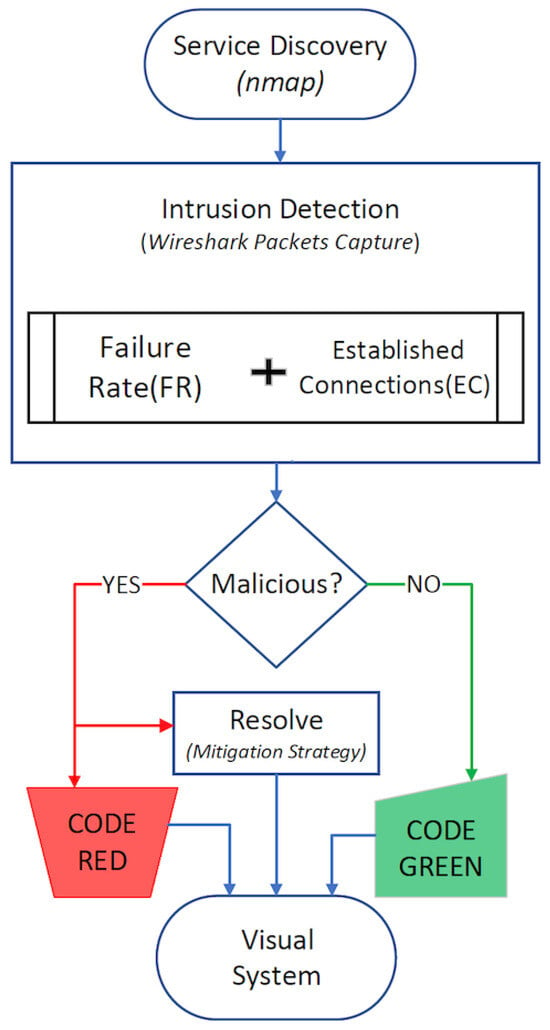
Figure 1
Open AccessArticle
Trustworthiness of Situational Awareness: Significance and Quantification
by
Arslan Munir, Alexander Aved, Khanh Pham and Joonho Kong
J. Cybersecur. Priv. 2024, 4(2), 223-240; https://doi.org/10.3390/jcp4020011 - 08 Apr 2024
Abstract
Situational awareness (SA) is of tremendous significance for successful operations in many domains, such as surveillance, humanitarian, search, and rescue missions, and national security. SA is particularly important for the defense sector, and is regarded as the decisive factor in military and air
[...] Read more.
Situational awareness (SA) is of tremendous significance for successful operations in many domains, such as surveillance, humanitarian, search, and rescue missions, and national security. SA is particularly important for the defense sector, and is regarded as the decisive factor in military and air combat engagements. Commanders and operators rely on the accuracy and fidelity of SA for comprehending the environment, decision-making, and carrying out actions based on these decisions for accomplishing a mission. SA, however, is susceptible to adversarial attacks that can compromise the security and trust of SA systems. In this paper, we discuss the significance of security and trust of SA from an air force perspective. We then propose a model for quantifying the trustworthiness of an SA system. We further present numerical examples that demonstrate the quantification of trustworthiness of an SA system using our proposed model. Finally, we conclude this paper with future research directions for quantifying the security of SA systems.
Full article
(This article belongs to the Section Security Engineering & Applications)
►▼
Show Figures
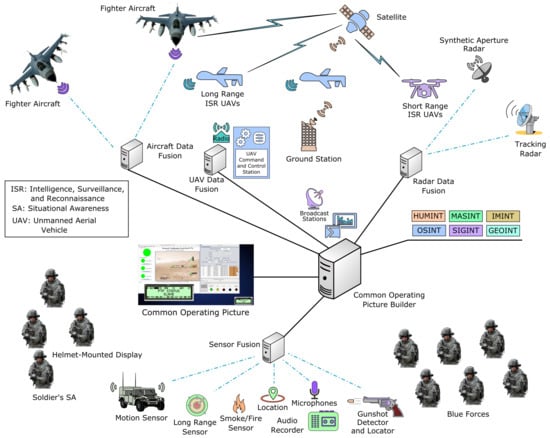
Figure 1
Open AccessArticle
A Blockchain-Based Decentralized Public Key Infrastructure Using the Web of Trust
by
Ratna Halder, Dipanjan Das Roy and Dongwan Shin
J. Cybersecur. Priv. 2024, 4(2), 196-222; https://doi.org/10.3390/jcp4020010 - 31 Mar 2024
Abstract
Internet applications rely on Secure Socket Layer (SSL)/Transport Security Layer (TSL) certifications to establish secure communication. However, the centralized nature of certificate authorities (CAs) poses a risk, as malicious third parties could exploit the CA to issue fake certificates to malicious web servers,
[...] Read more.
Internet applications rely on Secure Socket Layer (SSL)/Transport Security Layer (TSL) certifications to establish secure communication. However, the centralized nature of certificate authorities (CAs) poses a risk, as malicious third parties could exploit the CA to issue fake certificates to malicious web servers, potentially compromising the privacy and integrity of user data. In this paper, we demonstrate how the utilization of decentralized certificate verification with blockchain technology can effectively address and mitigate such attacks. We present a decentralized public key infrastructure (PKI) based on a distributed trust model, e.g., Web of Trust (WoT) and blockchain technologies, to overcome vulnerabilities like single points of failure and to prevent tampering with existing certificates. In addition, our infrastructure establishes a trusted key-ring network that decouples the authentication process from CAs in order to enhance secure certificate issuance and accelerate the revocation process. Furthermore, as a proof of concept, we present the implementation of our proposed system in the Ethereum blockchain, confirming that the proposed framework meets the five identified requirements. Our experimental results demonstrate the effectiveness of our proposed system in practice, albeit with additional overhead compared to conventional PKIs.
Full article
(This article belongs to the Special Issue Intrusion, Malware Detection and Prevention in Networks)
►▼
Show Figures
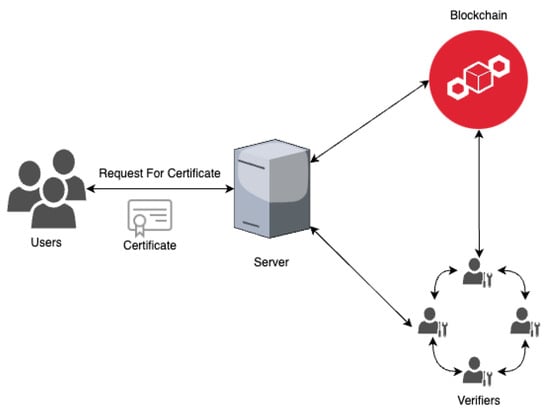
Figure 1
Open AccessArticle
On Data Leakage Prevention Maturity: Adapting the C2M2 Framework
by
Jan Domnik and Alexander Holland
J. Cybersecur. Priv. 2024, 4(2), 167-195; https://doi.org/10.3390/jcp4020009 - 30 Mar 2024
Abstract
In an evolving cybersecurity landscape marked by escalating data breaches and regulatory demands, data leakage prevention (DLP) has emerged as one of several defense mechanisms. This study underscores unresolved foundational issues within DLP, revealing that it remains a significant challenge in large organizations.
[...] Read more.
In an evolving cybersecurity landscape marked by escalating data breaches and regulatory demands, data leakage prevention (DLP) has emerged as one of several defense mechanisms. This study underscores unresolved foundational issues within DLP, revealing that it remains a significant challenge in large organizations. This highlights the necessity for a holistic approach to DLP to effectively address these persistent challenges. By developing a DLP Maturity Model, adapted from the renowned C2M2 framework, this research provides a comprehensive tool for assessing organizational DLP capabilities and pinpointing critical gaps. Applying the DLP Maturity Model within the financial sector as demonstrated through a banking scenario showcases its relevance and added value. This application illuminates the model’s effectiveness in securing sensitive data and adhering to essential regulatory standards, highlighting its adaptability across various compliance landscapes. Implementing this DLP Maturity Model in a banking scenario showcases its applicability, highlighting its ability to formulate a strategy to secure sensitive data and comply with regulatory standards. This approach aligns with the concept of a continuous risk-based strategy, merging the holistic model to identify and address critical insider risks within organizations. The study addresses a specific gap in DLP research, notably the lack of a holistic framework for assessing and enhancing DLP strategies across organizations. It equips practitioners with a foundational tool to determine current DLP maturity and devise strategies for mitigating insider-driven data breach risks, thereby bolstering organizational cybersecurity resilience.
Full article
(This article belongs to the Special Issue Cyber Security and Digital Forensics)
►▼
Show Figures
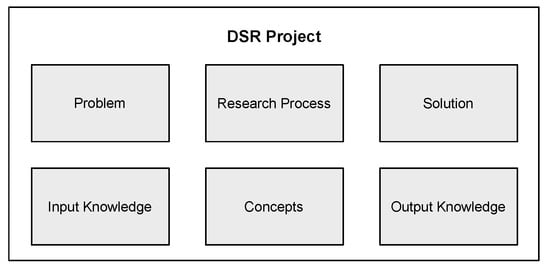
Figure 1
Open AccessArticle
ScriptBlock Smuggling: Uncovering Stealthy Evasion Techniques in PowerShell and .NET Environments
by
Anthony J. Rose, Scott R. Graham, Christine M. Schubert Kabban, Jacob J. Krasnov and Wayne C. Henry
J. Cybersecur. Priv. 2024, 4(2), 153-166; https://doi.org/10.3390/jcp4020008 - 25 Mar 2024
Abstract
The Antimalware Scan Interface (AMSI) plays a crucial role in detecting malware within Windows operating systems. This paper presents ScriptBlock Smuggling, a novel evasion and log spoofing technique exploiting PowerShell and .NET environments to circumvent the AMSI. By focusing on the manipulation of
[...] Read more.
The Antimalware Scan Interface (AMSI) plays a crucial role in detecting malware within Windows operating systems. This paper presents ScriptBlock Smuggling, a novel evasion and log spoofing technique exploiting PowerShell and .NET environments to circumvent the AMSI. By focusing on the manipulation of ScriptBlocks within the Abstract Syntax Tree (AST), this method creates dual AST representations, one for compiler execution and another for antivirus and log analysis, enabling the evasion of AMSI detection and challenging traditional memory patching bypass methods. This research provides a detailed analysis of PowerShell’s ScriptBlock creation and its inherent security features and pinpoints critical limitations in the AMSI’s capabilities to scrutinize ScriptBlocks and the implications of log spoofing as part of this evasion method. The findings highlight potential avenues for attackers to exploit these vulnerabilities, suggesting the possibility of a new class of AMSI bypasses and their use for log spoofing. In response, this paper proposes a synchronization strategy for ASTs, intended to unify the compilation and malware scanning processes to reduce the threat surfaces in PowerShell and .NET environments.
Full article
(This article belongs to the Special Issue Intrusion, Malware Detection and Prevention in Networks)
►▼
Show Figures
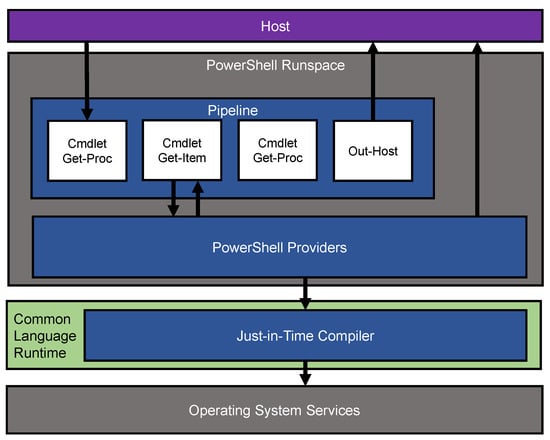
Figure 1
Open AccessReview
Image Encryption Algorithms: A Survey of Design and Evaluation Metrics
by
Yousef Alghamdi and Arslan Munir
J. Cybersecur. Priv. 2024, 4(1), 126-152; https://doi.org/10.3390/jcp4010007 - 23 Feb 2024
Abstract
Ensuring confidentiality and privacy is critical when it comes to sharing images over unsecured networks such as the internet. Since widely used and secure encryption methods, such as AES, Twofish, and RSA, are not suitable for real-time image encryption due to their slow
[...] Read more.
Ensuring confidentiality and privacy is critical when it comes to sharing images over unsecured networks such as the internet. Since widely used and secure encryption methods, such as AES, Twofish, and RSA, are not suitable for real-time image encryption due to their slow encryption speeds and high computational requirements, researchers have proposed specialized algorithms for image encryption. This paper provides an introduction and overview of the image encryption algorithms and metrics used, aiming to evaluate them and help researchers and practitioners starting in this field obtain adequate information to understand the current state of image encryption algorithms. This paper classifies image encryption into seven different approaches based on the techniques used and analyzes the strengths and weaknesses of each approach. Furthermore, this paper provides a detailed review of a comprehensive set of security, quality, and efficiency evaluation metrics for image encryption algorithms, and provides upper and lower bounds for these evaluation metrics. Finally, this paper discusses the pros and cons of different image encryption approaches as well as the suitability of different image encryption approaches for different applications.
Full article
(This article belongs to the Special Issue Multimedia Security and Privacy)
►▼
Show Figures
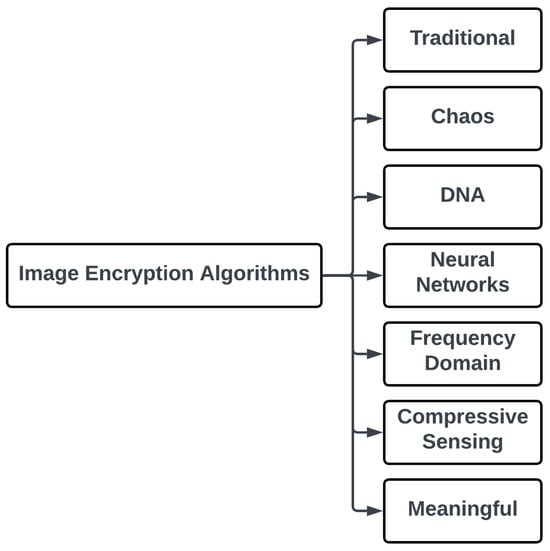
Figure 1
Open AccessArticle
Incidental Data: A Survey towards Awareness on Privacy-Compromising Data Incidentally Shared on Social Media
by
Stefan Kutschera, Wolfgang Slany, Patrick Ratschiller, Sarina Gursch, Patrick Deininger and Håvard Dagenborg
J. Cybersecur. Priv. 2024, 4(1), 105-125; https://doi.org/10.3390/jcp4010006 - 23 Feb 2024
Abstract
Sharing information with the public is becoming easier than ever before through the usage of the numerous social media platforms readily available today. Once posted online and released to the public, information is almost impossible to withdraw or delete. More alarmingly, postings may
[...] Read more.
Sharing information with the public is becoming easier than ever before through the usage of the numerous social media platforms readily available today. Once posted online and released to the public, information is almost impossible to withdraw or delete. More alarmingly, postings may carry sensitive information far beyond what was intended to be released, so-called incidental data, which raises various additional security and privacy concerns. To improve our understanding of the awareness of incidental data, we conducted a survey where we asked 192 students for their opinions on publishing selected postings on social media. We found that up to 21.88% of all participants would publish a posting that contained incidental data that two-thirds of them found privacy-compromising. Our results show that continued efforts are needed to increase our awareness of incidental data posted on social media.
Full article
(This article belongs to the Special Issue Data Protection and Privacy)
►▼
Show Figures
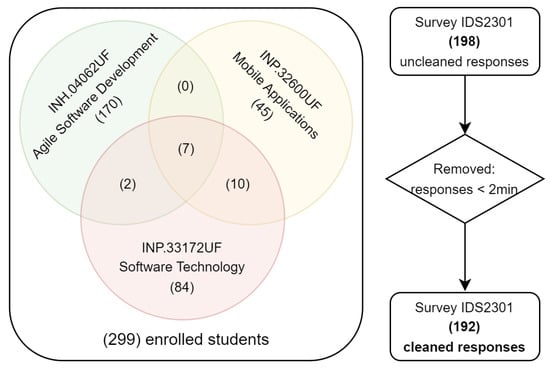
Figure 1
Open AccessArticle
Experiential Transformation in Privacy Behavior: A New Framework for Privacy Behavior Enhancement
by
Ioannis Paspatis and Aggeliki Tsohou
J. Cybersecur. Priv. 2024, 4(1), 76-104; https://doi.org/10.3390/jcp4010005 - 07 Feb 2024
Abstract
Multiple studies have demonstrated that the conventional method of learning is suboptimal when our goal is to enhance individuals’ genuine privacy behavior. This study introduces a framework for transforming privacy behavior, with the objective of enhancing individuals’ privacy practices to a higher level
[...] Read more.
Multiple studies have demonstrated that the conventional method of learning is suboptimal when our goal is to enhance individuals’ genuine privacy behavior. This study introduces a framework for transforming privacy behavior, with the objective of enhancing individuals’ privacy practices to a higher level of confidentiality. We performed an experiment on a limited number of people to validate the efficacy of our suggested transformation framework. This framework combined determining aspects of privacy behavior with experiential behavior modification methodologies such as neutral stimuli (e.g., cognitive behavioral transformation—CBTx), practical assessments and motivational interviews from other disciplines. While these methods have proven effective in fields like psychology and sociology, they have not yet been applied to the realm of Information Computer and Technology (ICT). In this study, we have effectively demonstrated the efficacy of the proposed framework through a five-phase experiment. The suggested framework has the potential to be advantageous for educational institutions, including both public and private schools as well as universities, to construct new frameworks or develop new methodologies regarding individuals’ privacy behavior transformation to a more protective one. Furthermore, our framework offers a conducive environment for further investigation into privacy behavior transformation methodologies.
Full article
(This article belongs to the Special Issue Privacy-Enhancing Technologies for User Systems to Foster a More Ethical E-society)
►▼
Show Figures
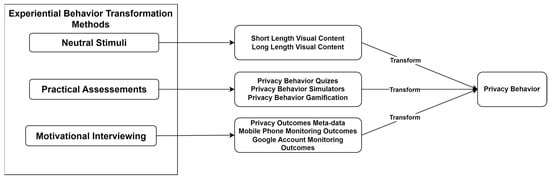
Figure 1
Open AccessArticle
Security Attack Behavioural Pattern Analysis for Critical Service Providers
by
Elias Seid, Oliver Popov and Fredrik Blix
J. Cybersecur. Priv. 2024, 4(1), 55-75; https://doi.org/10.3390/jcp4010004 - 10 Jan 2024
Abstract
Identifying potential system attacks that define security requirements is crucial to building secure cyber systems. Moreover, the attack frequency makes their subsequent analysis challenging and arduous in cyber–physical systems (CPS). Since CPS include people, organisations, software, and infrastructure, a thorough security attack analysis
[...] Read more.
Identifying potential system attacks that define security requirements is crucial to building secure cyber systems. Moreover, the attack frequency makes their subsequent analysis challenging and arduous in cyber–physical systems (CPS). Since CPS include people, organisations, software, and infrastructure, a thorough security attack analysis must consider both strategic (social and organisational) aspects and technical (software and physical infrastructure) aspects. Studying cyberattacks and their potential impact on internal and external assets in cyberspace is essential for maintaining cyber security. The importance is reflected in the work of the Swedish Civil Contingencies Agency (MSB), which receives IT incident reports from essential service providers mandated by the NIS directive of the European Union and Swedish government agencies. To tackle this problem, a multi-realm security attack event monitoring framework was proposed to monitor, model, and analyse security events in social(business process), cyber, and physical infrastructure components of cyber–physical systems. This paper scrutinises security attack patterns and the corresponding security solutions for Swedish government agencies and organisations within the EU’s NIS directive. A pattern analysis was conducted on 254 security incident reports submitted by critical service providers. A total of five critical security attacks, seven vulnerabilities (commonly known as threats), ten attack patterns, and ten parallel attack patterns were identified. Moreover, we employed standard mitigation techniques obtained from recognised repositories of cyberattack knowledge, namely, CAPEC and Mitre, in order to conduct an analysis of the behavioural patterns
Full article
(This article belongs to the Special Issue Secure Software Engineering)
►▼
Show Figures
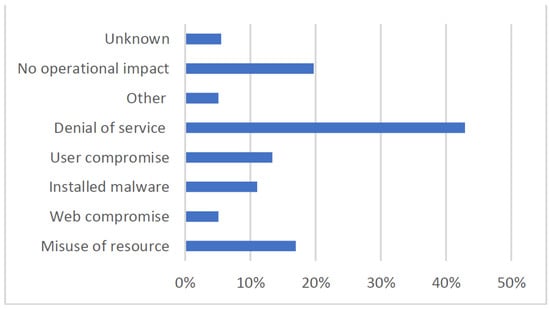
Figure 1
Open AccessArticle
Continued Fractions Applied to the One Line Factoring Algorithm for Breaking RSA
by
Anthony Overmars and Sitalakshmi Venkatraman
J. Cybersecur. Priv. 2024, 4(1), 41-54; https://doi.org/10.3390/jcp4010003 - 10 Jan 2024
Abstract
The RSA (Rivest–Shamir–Adleman) cryptosystem is an asymmetric public key cryptosystem popular for its use in encryptions and digital signatures. However, the Wiener’s attack on the RSA cryptosystem utilizes continued fractions, which has generated much interest in developing competitive factoring algorithms. A general-purpose integer
[...] Read more.
The RSA (Rivest–Shamir–Adleman) cryptosystem is an asymmetric public key cryptosystem popular for its use in encryptions and digital signatures. However, the Wiener’s attack on the RSA cryptosystem utilizes continued fractions, which has generated much interest in developing competitive factoring algorithms. A general-purpose integer factorization method first proposed by Lehmer and Powers formed the basis of the well-known Continued Fraction Factorization (CFRAC) method. Recent work on the one line factoring algorithm by Hart and its connection with Lehman’s factoring method have motivated this paper. The emphasis of this paper is to explore the representations of as continued fractions and the suitability of lower ordered convergences as representations of . These simpler convergences are then prescribed to Hart’s one line factoring algorithm. As an illustration, we demonstrate the working of our approach with two numbers: one smaller number and another larger number occupying 95 bits. Using our method, the fourth convergence finds the factors as the solution for the smaller number, while the eleventh convergence finds the factors for the larger number. The security of the RSA public key cryptosystem relies on the computational difficulty of factoring large integers. Among the challenges in breaking RSA semi-primes, RSA250, which is an 829-bit semi-prime, continues to hold a research record. In this paper, we apply our method to factorize RSA250 and present the practical implementation of our algorithm. Our approach’s theoretical and experimental findings demonstrate the reduction of the search space and a faster solution to the semi-prime factorization problem, resulting in key contributions and practical implications. We identify further research to extend our approach by exploring limitations and additional considerations such as the difference of squares method, paving the way for further research in this direction.
Full article
(This article belongs to the Section Cryptography and Cryptology)
Open AccessArticle
Security Vulnerabilities in 5G Non-Stand-Alone Networks: A Systematic Analysis and Attack Taxonomy
by
Mohamad Saalim Wani, Michael Rademacher, Thorsten Horstmann and Mathias Kretschmer
J. Cybersecur. Priv. 2024, 4(1), 23-40; https://doi.org/10.3390/jcp4010002 - 02 Jan 2024
Abstract
►▼
Show Figures
5G networks, pivotal for our digital mobile societies, are transitioning from 4G to 5G Stand-Alone (SA) networks. However, during this transition, 5G Non-Stand-Alone (NSA) networks are widely used. This paper examines potential security vulnerabilities in 5G NSA networks. Through an extensive literature review,
[...] Read more.
5G networks, pivotal for our digital mobile societies, are transitioning from 4G to 5G Stand-Alone (SA) networks. However, during this transition, 5G Non-Stand-Alone (NSA) networks are widely used. This paper examines potential security vulnerabilities in 5G NSA networks. Through an extensive literature review, we identify known 4G attacks that can theoretically be applied to 5G NSA. We organize these attacks into a structured taxonomy. Our findings reveal that 5G NSA networks may offer a false sense of security, as most security and privacy improvements are concentrated in 5G SA networks. To underscore this concern, we implement three attacks with severe consequences and successfully validate them on various commercially available smartphones. Notably, one of these attacks, the IMSI Leak, consistently exposes user information with no apparent security mitigation in 5G NSA networks. This highlights the ease of tracking individuals on current 5G networks.
Full article
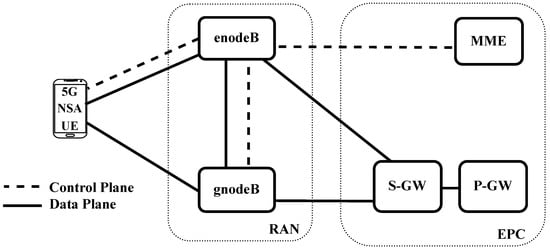
Figure 1
Open AccessArticle
How Close Is Existing C/C++ Code to a Safe Subset?
by
Christian DeLozier
J. Cybersecur. Priv. 2024, 4(1), 1-22; https://doi.org/10.3390/jcp4010001 - 28 Dec 2023
Abstract
Using a safe subset of C++ is a promising direction for increasing the safety of the programming language while maintaining its performance and productivity. In this paper, we examine how close existing C/C++ code is to conforming to a safe subset of C++.
[...] Read more.
Using a safe subset of C++ is a promising direction for increasing the safety of the programming language while maintaining its performance and productivity. In this paper, we examine how close existing C/C++ code is to conforming to a safe subset of C++. We examine the rules presented in existing safe C/C++ standards and safe C/C++ subsets. We analyze the code characteristics of 5.8 million code samples from the Exebench benchmark suite, two C/C++ benchmark suites, and five modern C++ applications using a static analysis tool. We find that raw pointers, unsafe casts, and unsafe library functions are used in both C/C++ code at large and in modern C++ applications. In general, C/C++ code at large does not differ much from modern C++ code, and continued work will be required to transition from existing C/C++ code to a safe subset of C++.
Full article
(This article belongs to the Special Issue Secure Software Engineering)
►▼
Show Figures

Figure 1
Open AccessArticle
Anomaly Detection for Modbus over TCP in Control Systems Using Entropy and Classification-Based Analysis
by
Tirthankar Ghosh, Sikha Bagui, Subhash Bagui, Martin Kadzis and Jackson Bare
J. Cybersecur. Priv. 2023, 3(4), 895-913; https://doi.org/10.3390/jcp3040041 - 01 Dec 2023
Abstract
This article presents a statistical approach using entropy and classification-based analysis to detect anomalies in industrial control systems traffic. Several statistical techniques have been proposed to create baselines and measure deviation to detect intrusion in enterprise networks with a centralized intrusion detection approach
[...] Read more.
This article presents a statistical approach using entropy and classification-based analysis to detect anomalies in industrial control systems traffic. Several statistical techniques have been proposed to create baselines and measure deviation to detect intrusion in enterprise networks with a centralized intrusion detection approach in mind. Looking at traffic volume alone to find anomalous deviation may not be enough—it may result in increased false positives. The near real-time communication requirements, coupled with the lack of centralized infrastructure in operations technology and limited resources of the sensor motes, require an efficient anomaly detection system characterized by these limitations. This paper presents extended results from our previous work by presenting a detailed cluster-based entropy analysis on selected network traffic features. It further extends the analysis using a classification-based approach. Our detailed entropy analysis corroborates with our earlier findings that, although some degree of anomaly may be detected using univariate and bivariate entropy analysis for Denial of Service (DOS) and Man-in-the-Middle (MITM) attacks, not much information may be obtained for the initial reconnaissance, thus preventing early stages of attack detection in the Cyber Kill Chain. Our classification-based analysis shows that, overall, the classification results of the DOS attacks were much higher than the MITM attacks using two Modbus features in addition to the three TCP/IP features. In terms of classifiers, J48 and random forest had the best classification results and can be considered comparable. For the DOS attack, no resampling with the 60–40 (training/testing split) had the best results (average accuracy of 97.87%), but for the MITM attack, the 80–20 non-attack vs. attack data with the 75–25 split (average accuracy of 82.81%) had the best results.
Full article
(This article belongs to the Special Issue Intrusion, Malware Detection and Prevention in Networks)
►▼
Show Figures
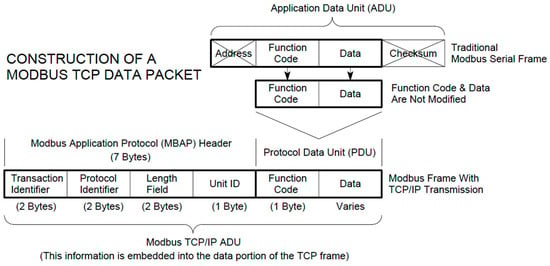
Figure 1
Open AccessArticle
Evaluating Cluster-Based Synthetic Data Generation for Blood-Transfusion Analysis
by
Shannon K. S. Kroes, Matthijs van Leeuwen, Rolf H. H. Groenwold and Mart P. Janssen
J. Cybersecur. Priv. 2023, 3(4), 882-894; https://doi.org/10.3390/jcp3040040 - 01 Dec 2023
Abstract
Synthetic data generation is becoming an increasingly popular approach to making privacy-sensitive data available for analysis. Recently, cluster-based synthetic data generation (CBSDG) has been proposed, which uses explainable and tractable techniques for privacy preservation. Although the algorithm demonstrated promising performance on simulated data,
[...] Read more.
Synthetic data generation is becoming an increasingly popular approach to making privacy-sensitive data available for analysis. Recently, cluster-based synthetic data generation (CBSDG) has been proposed, which uses explainable and tractable techniques for privacy preservation. Although the algorithm demonstrated promising performance on simulated data, CBSDG has not yet been applied to real, personal data. In this work, a published blood-transfusion analysis is replicated with synthetic data to assess whether CBSDG can reproduce more complex and intricate variable relations than previously evaluated. Data from the Dutch national blood bank, consisting of 250,729 donation records, were used to predict donor hemoglobin (Hb) levels by means of support vector machines (SVMs). Precision scores were equal to the original data results for both male (0.997) and female (0.987) donors, recall was 0.007 higher for male and 0.003 lower for female donors (original estimates 0.739 and 0.637, respectively). The impact of the variables on Hb predictions was similar, as quantified and visualized with Shapley additive explanation values. Opportunities for attribute disclosure were decreased for all but two variables; only the binary variables Deferral Status and Sex could still be inferred. Such inference was also possible for donors who were not used as input for the generator and may result from correlations in the data as opposed to overfitting in the synthetic-data-generation process. The high predictive performance obtained with the synthetic data shows potential of CBSDG for practical implementation.
Full article
(This article belongs to the Special Issue Data Protection and Privacy)
►▼
Show Figures
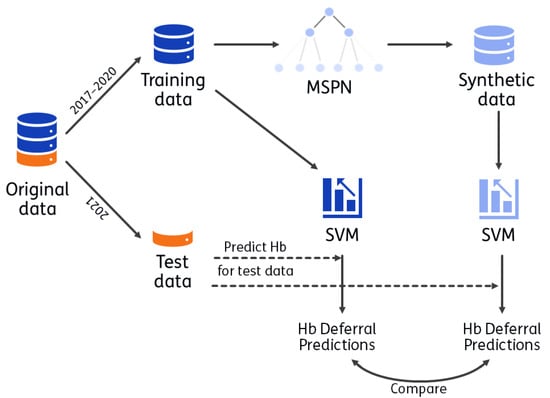
Figure 1
Open AccessArticle
Machine Learning Detection of Cloud Services Abuse as C&C Infrastructure
by
Turki Al lelah, George Theodorakopoulos, Amir Javed and Eirini Anthi
J. Cybersecur. Priv. 2023, 3(4), 858-881; https://doi.org/10.3390/jcp3040039 - 01 Dec 2023
Abstract
The proliferation of cloud and public legitimate services (CLS) on a global scale has resulted in increasingly sophisticated malware attacks that abuse these services as command-and-control (C&C) communication channels. Conventional security solutions are inadequate for detecting malicious C&C traffic because it blends with
[...] Read more.
The proliferation of cloud and public legitimate services (CLS) on a global scale has resulted in increasingly sophisticated malware attacks that abuse these services as command-and-control (C&C) communication channels. Conventional security solutions are inadequate for detecting malicious C&C traffic because it blends with legitimate traffic. This motivates the development of advanced detection techniques. We make the following contributions: First, we introduce a novel labeled dataset. This dataset serves as a valuable resource for training and evaluating detection techniques aimed at identifying malicious bots that abuse CLS as C&C channels. Second, we tailor our feature engineering to behaviors indicative of CLS abuse, such as connections to known CLS domains and potential C&C API calls. Third, to identify the most relevant features, we introduced a custom feature elimination (CFE) method designed to determine the exact number of features needed for filter selection approaches. Fourth, our approach focuses on both static and derivative features of Portable Executable (PE) files. After evaluating various machine learning (ML) classifiers, the random forest emerges as the most effective classifier, achieving a 98.26% detection rate. Fifth, we introduce the “Replace Misclassified Parameter (RMCP)” adversarial attack. This white-box strategy is designed to evaluate our system’s detection robustness. The RMCP attack modifies feature values in malicious samples to make them appear as benign samples, thereby bypassing the ML model’s classification while maintaining the malware’s malicious capabilities. The results of the robustness evaluation demonstrate that our proposed method successfully maintains a high accuracy level of 84%. In sum, our comprehensive approach offers a robust solution to the growing threat of malware abusing CLS as C&C infrastructure.
Full article
(This article belongs to the Special Issue Cloud Security and Privacy)
►▼
Show Figures
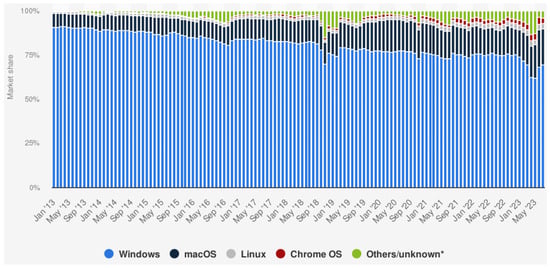
Figure 1
Open AccessArticle
Challenging Assumptions of Normality in AES s-Box Configurations under Side-Channel Analysis
by
Clay Carper, Stone Olguin, Jarek Brown, Caylie Charlton and Mike Borowczak
J. Cybersecur. Priv. 2023, 3(4), 844-857; https://doi.org/10.3390/jcp3040038 - 29 Nov 2023
Abstract
►▼
Show Figures
Power-based Side-Channel Analysis (SCA) began with visual-based examinations and has progressed to utilize data-driven statistical analysis. Two distinct classifications of these methods have emerged over the years; those focused on leakage exploitation and those dedicated to leakage detection. This work primarily focuses on
[...] Read more.
Power-based Side-Channel Analysis (SCA) began with visual-based examinations and has progressed to utilize data-driven statistical analysis. Two distinct classifications of these methods have emerged over the years; those focused on leakage exploitation and those dedicated to leakage detection. This work primarily focuses on a leakage detection-based schema that utilizes Welch’s t-test, known as Test Vector Leakage Assessment (TVLA). Both classes of methods process collected data using statistical frameworks that result in the successful exfiltration of information via SCA. Often, statistical testing used during analysis requires the assumption that collected power consumption data originates from a normal distribution. To date, this assumption has remained largely uncontested. This work seeks to demonstrate that while past studies have assumed the normality of collected power traces, this assumption should be properly evaluated. In order to evaluate this assumption, an implementation of Tiny-AES-c with nine unique substitution-box (s-box) configurations is conducted using TVLA to guide experimental design. By leveraging the complexity of the AES algorithm, a sufficiently diverse and complex dataset was developed. Under this dataset, statistical tests for normality such as the Shapiro-Wilk test and the Kolmogorov-Smirnov test provide significant evidence to reject the null hypothesis that the power consumption data is normally distributed. To address this observation, existing non-parametric equivalents such as the Wilcoxon Signed-Rank Test and the Kruskal-Wallis Test are discussed in relation to currently used parametric tests such as Welch’s t-test.
Full article
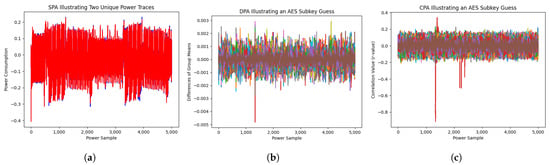
Figure 1
Open AccessArticle
A Hybrid Dimensionality Reduction for Network Intrusion Detection
by
Humera Ghani, Shahram Salekzamankhani and Bal Virdee
J. Cybersecur. Priv. 2023, 3(4), 830-843; https://doi.org/10.3390/jcp3040037 - 16 Nov 2023
Cited by 1
Abstract
Due to the wide variety of network services, many different types of protocols exist, producing various packet features. Some features contain irrelevant and redundant information. The presence of such features increases computational complexity and decreases accuracy. Therefore, this research is designed to reduce
[...] Read more.
Due to the wide variety of network services, many different types of protocols exist, producing various packet features. Some features contain irrelevant and redundant information. The presence of such features increases computational complexity and decreases accuracy. Therefore, this research is designed to reduce the data dimensionality and improve the classification accuracy in the UNSW-NB15 dataset. It proposes a hybrid dimensionality reduction system that does feature selection (FS) and feature extraction (FE). FS was performed using the Recursive Feature Elimination (RFE) technique, while FE was accomplished by transforming the features into principal components. This combined scheme reduced a total of 41 input features into 15 components. The proposed systems’ classification performance was determined using an ensemble of Support Vector Classifier (SVC), K-nearest Neighbor classifier (KNC), and Deep Neural Network classifier (DNN). The system was evaluated using accuracy, detection rate, false positive rate, f1-score, and area under the curve metrics. Comparing the voting ensemble results of the full feature set against the 15 principal components confirms that reduced and transformed features did not significantly decrease the classifier’s performance. We achieved 94.34% accuracy, a 93.92% detection rate, a 5.23% false positive rate, a 94.32% f1-score, and a 94.34% area under the curve when 15 components were input to the voting ensemble classifier.
Full article
(This article belongs to the Special Issue Intrusion, Malware Detection and Prevention in Networks)
►▼
Show Figures
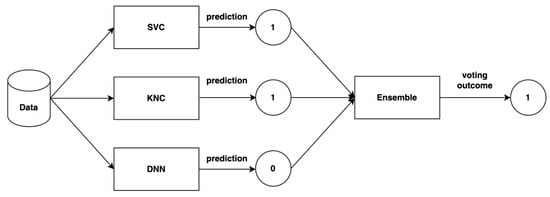
Figure 1
Open AccessArticle
D2WFP: A Novel Protocol for Forensically Identifying, Extracting, and Analysing Deep and Dark Web Browsing Activities
by
Mohamed Chahine Ghanem, Patrick Mulvihill, Karim Ouazzane, Ramzi Djemai and Dipo Dunsin
J. Cybersecur. Priv. 2023, 3(4), 808-829; https://doi.org/10.3390/jcp3040036 - 15 Nov 2023
Cited by 1
Abstract
The use of the unindexed web, commonly known as the deep web and dark web, to commit or facilitate criminal activity has drastically increased over the past decade. The dark web is a dangerous place where all kinds of criminal activities take place,
[...] Read more.
The use of the unindexed web, commonly known as the deep web and dark web, to commit or facilitate criminal activity has drastically increased over the past decade. The dark web is a dangerous place where all kinds of criminal activities take place, Despite advances in web forensic techniques, tools, and methodologies, few studies have formally tackled dark and deep web forensics and the technical differences in terms of investigative techniques and artefact identification and extraction. This study proposes a novel and comprehensive protocol to guide and assist digital forensic professionals in investigating crimes committed on or via the deep and dark web. The protocol, named D2WFP, establishes a new sequential approach for performing investigative activities by observing the order of volatility and implementing a systemic approach covering all browsing-related hives and artefacts which ultimately resulted in improving the accuracy and effectiveness. Rigorous quantitative and qualitative research has been conducted by assessing the D2WFP following a scientifically sound and comprehensive process in different scenarios and the obtained results show an apparent increase in the number of artefacts recovered when adopting the D2WFP which outperforms any current industry or opensource browsing forensic tools. The second contribution of the D2WFP is the robust formulation of artefact correlation and cross-validation within the D2WFP which enables digital forensic professionals to better document and structure their analysis of host-based deep and dark web browsing artefacts.
Full article
(This article belongs to the Special Issue Cyber Security and Digital Forensics)
►▼
Show Figures
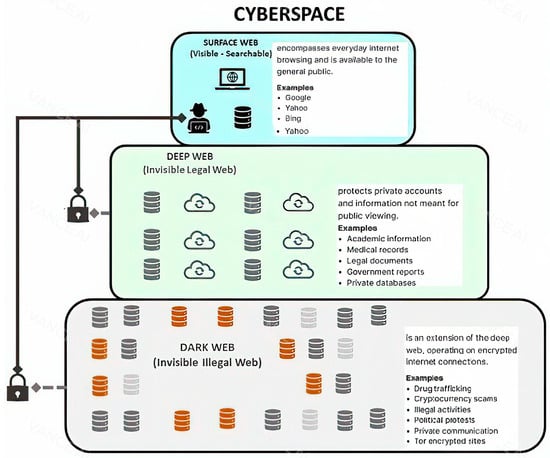
Figure 1
Highly Accessed Articles
Latest Books
E-Mail Alert
News
Topics
Topic in
Applied Sciences, Electronics, Future Internet, JCP, Sensors, Technologies
Cyber-Physical Security for IoT Systems
Topic Editors: Keping Yu, Chinmay ChakrabortyDeadline: 30 June 2024
Topic in
Applied Sciences, Cryptography, JCP, JSAN, Sci, Symmetry
Trends and Prospects in Security, Encryption and Encoding
Topic Editors: Ki-Hyun Jung, Luis Javier García VillalbaDeadline: 31 December 2024
Topic in
Electronics, JCP, Mathematics, Sustainability, Remote Sensing, IJGI
Recent Advances in Security, Privacy, and Trust
Topic Editors: Jun Feng, Changqing Luo, Mamoun AlazabDeadline: 31 December 2025

Conferences
Special Issues
Special Issue in
JCP
Intrusion, Malware Detection and Prevention in Networks
Guest Editors: Feng Wang, Yongning TangDeadline: 15 May 2024
Special Issue in
JCP
Security and Privacy of Intelligent Industrial Internet of Things
Guest Editors: Alan Briones, Ester Vidaña, Agustín Zaballos, Joan Navarro, Víctor CaballeroDeadline: 20 June 2024
Special Issue in
JCP
Data Protection and Privacy
Guest Editors: Kyriakos Stefanidis, Chrysostomos Stylios, Vasiliki LiagkouDeadline: 30 June 2024
Special Issue in
JCP
Privacy-Enhancing Technologies for User Systems to Foster a More Ethical E-society
Guest Editors: Thomas Hupperich, Martin Degeling, Luis Javier García Villalba, Maryline Laurent, Georgios KambourakisDeadline: 30 July 2024
Topical Collections
Topical Collection in
JCP
Intelligent Security and Privacy Approaches against Cyber Threats
Collection Editor: Nour Moustafa
Topical Collection in
JCP
Machine Learning and Data Analytics for Cyber Security
Collection Editors: Phil Legg, Giorgio Giacinto