Advances in the Use of Artificial Intelligence (AI)/Machine Learning (ML) and IoT in the Primary Sector
A special issue of Electronics (ISSN 2079-9292). This special issue belongs to the section "Artificial Intelligence".
Deadline for manuscript submissions: closed (31 July 2024) | Viewed by 18748
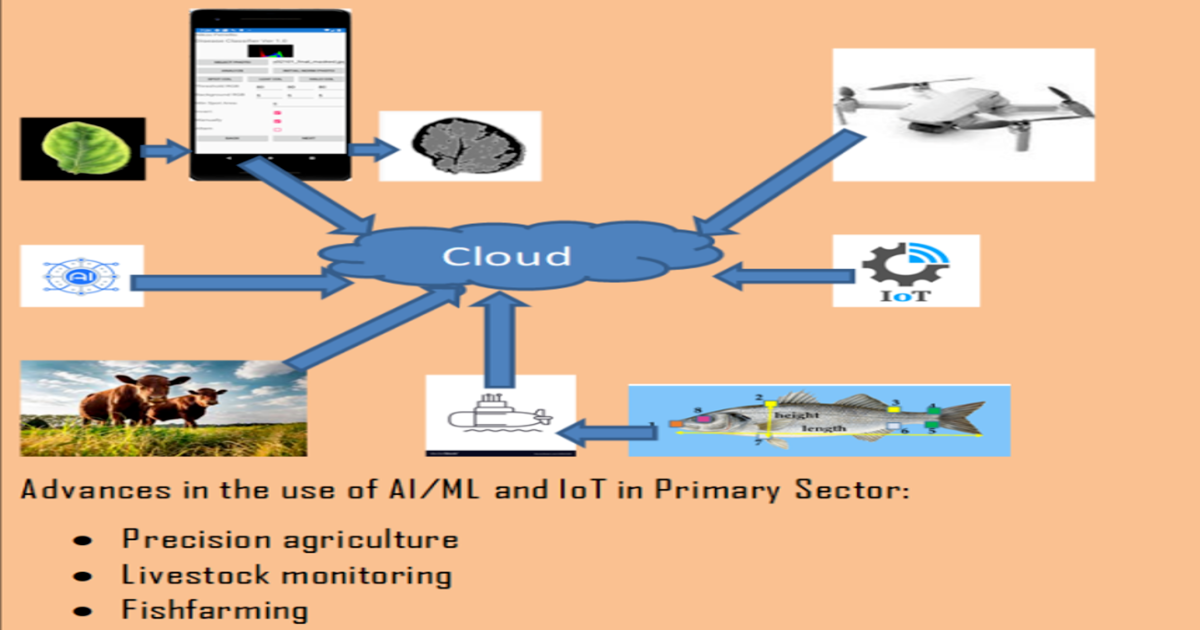
Special Issue Editors
Interests: image processing; classification; segmentation; disease diagnosis; plant disease; image filtering; Embedded systems; mixed signal; Signal Processing
Special Issues, Collections and Topics in MDPI journals
Interests: embedded systems; cyber-physical systems
Interests: wireless sensor networks architectures and performance; cross-layer commination protocols; power optimization for wireless sensor networks; cyber physical systems; internet of things; embedded systems; network simulation; performance evaluation
Special Issues, Collections and Topics in MDPI journals
Special Issue Information
Dear Colleagues,
Artificial Intelligence (AI), and Machine Learning (ML) more generally, have been used in a variety of fields of application. Images, as well as other signals generated by IoT sensors, can be efficiently processed using AI or ML techniques. In the primary sector, AI and ML can be used to process signals from cameras and IoT sensors for crop, livestock, as well as fish culture monitoring. Diseases can be diagnosed early, and harvesting time can be optimally scheduled to achieve the highest production efficiency with the lowest cost and the lowest environmental impact.
This Special Issue of Electronics will provide a forum for presenting novel AI and ML approaches on data captured by IoT sensors and cameras in primary sector applications. The images can be captured from mobile devices, UAVs, or underwater cameras. Original research articles and review papers are both welcome.
Topics of Interest of this Special Issue include, but are not limited to:
- AI/ML in precision agriculture applications:
- Crop monitoring;
- Exploiting UAVs and aerial photographs in crop monitoring;
- Maturity of fruit selection;
- Plant disease recognition;
- Robotics in precision agriculture;
- Irrigation control;
- Pollution monitoring.
- AI/ML in livestock monitoring:
- Animal activity;
- Health monitoring;
- Animal health certification;
- Sensors for livestock.
- AI/ML in fish cultures:
- Fish biometric feature measurement;
- Fish health monitoring;
- Fish waste management;
- Fish culture pollution monitoring;
- Submarine vehicles for fish culture monitoring.
Dr. Nikos Petrellis
Prof. Dr. Nikolaos Voros
Dr. Christos P. Antonopoulos
Guest Editors
Manuscript Submission Information
Manuscripts should be submitted online at www.mdpi.com by registering and logging in to this website. Once you are registered, click here to go to the submission form. Manuscripts can be submitted until the deadline. All submissions that pass pre-check are peer-reviewed. Accepted papers will be published continuously in the journal (as soon as accepted) and will be listed together on the special issue website. Research articles, review articles as well as short communications are invited. For planned papers, a title and short abstract (about 100 words) can be sent to the Editorial Office for announcement on this website.
Submitted manuscripts should not have been published previously, nor be under consideration for publication elsewhere (except conference proceedings papers). All manuscripts are thoroughly refereed through a single-blind peer-review process. A guide for authors and other relevant information for submission of manuscripts is available on the Instructions for Authors page. Electronics is an international peer-reviewed open access semimonthly journal published by MDPI.
Please visit the Instructions for Authors page before submitting a manuscript. The Article Processing Charge (APC) for publication in this open access journal is 2400 CHF (Swiss Francs). Submitted papers should be well formatted and use good English. Authors may use MDPI's English editing service prior to publication or during author revisions.
Keywords
- precision agriculture
- plant disease
- irregation
- crop monitoring
- livestock monitoring
- fish monitoring
- image processing
- AI/ML for object recognition
- shape alignment
- pattern recognition
Benefits of Publishing in a Special Issue
- Ease of navigation: Grouping papers by topic helps scholars navigate broad scope journals more efficiently.
- Greater discoverability: Special Issues support the reach and impact of scientific research. Articles in Special Issues are more discoverable and cited more frequently.
- Expansion of research network: Special Issues facilitate connections among authors, fostering scientific collaborations.
- External promotion: Articles in Special Issues are often promoted through the journal's social media, increasing their visibility.
- e-Book format: Special Issues with more than 10 articles can be published as dedicated e-books, ensuring wide and rapid dissemination.
Further information on MDPI's Special Issue policies can be found here.