Advances in Robotics and Mechatronics for Agriculture
A special issue of Applied Sciences (ISSN 2076-3417). This special issue belongs to the section "Agricultural Science and Technology".
Deadline for manuscript submissions: closed (20 December 2022) | Viewed by 22970
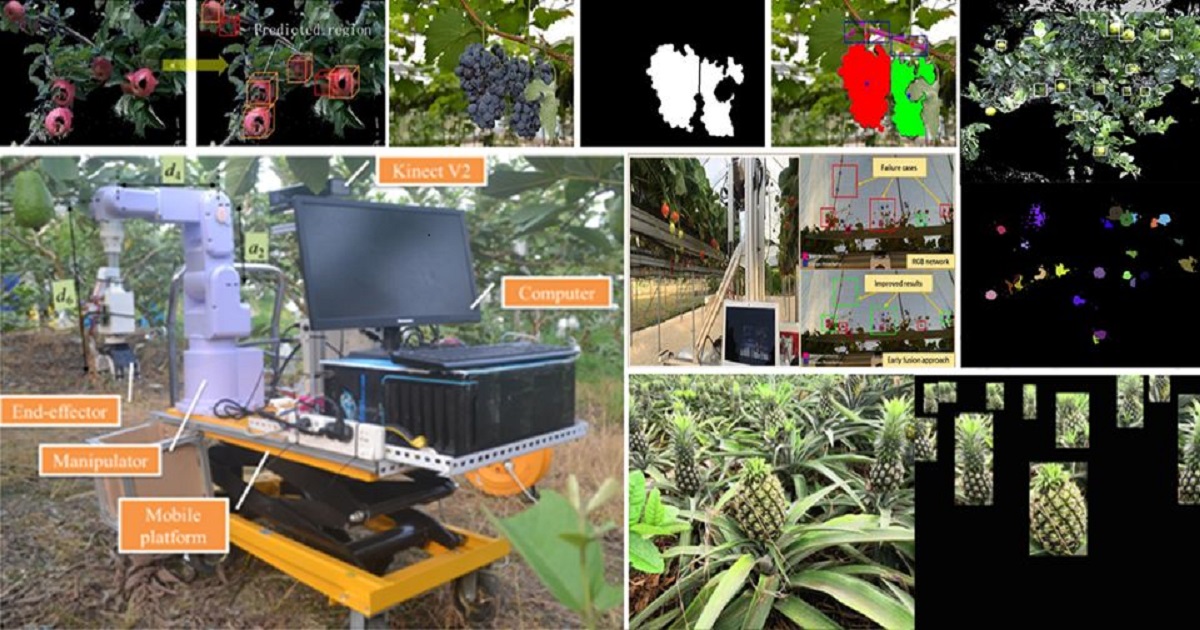
Special Issue Editors
Interests: computer vision; field robotics; frp composites; recycled concrete; mechanical performance of steel structures; computer vision; 3d reconstruction
Special Issues, Collections and Topics in MDPI journals
Interests: agricultural machinery; agricultural automation; field robotics
Interests: agricultural robots; machine vision and image processing; simulation technology
Special Issues, Collections and Topics in MDPI journals
Interests: intelligent perception; control of robots; agricultural robots; intelligent production lines; digital twins
Special Issues, Collections and Topics in MDPI journals
Special Issue Information
Dear Colleagues,
The operation scene of agricultural equipment is usually a complex, dynamic, and unstructured environment, with many links in current agricultural production still relying on labor-intensive operation. Flexibility, intelligence, and automation of smart agricultural machine have become a hotspot of modern agriculture at home and abroad. With the sharp development of artificial intelligence, robotics, intelligent sensing, flexible control, and other technologies, these advanced technologies have injected strong power support to improve the intelligent level of agricultural equipment. However, the development of precision agriculture and unmanned farms requires agricultural robots to have a series of intelligent behavior capabilities, including autonomous perception, cognition, autonomous path planning, and flexible adaptive operation.
The main aims of this focused section in Applied Sciences are to present the current state of the art in Robotics and Mechatronics for Precision Agriculture and to illustrate new results in several emerging research areas. Submissions can present theoretical and experimental aspects in these areas. The topics of interest within the scope of this focused section include but are not limited to:
- Advanced autonomy for unmanned mechatronics systems;
- Advanced machines or robotics for precision agriculture;
- Advanced approaches for high-throughput phenotyping and remote sensing;
- Advancement of cooperative mechatronics systems for precision agriculture;
- Soft-grasping/soft-robotics manipulators;
- Fruit/vegetable detection and localization for automatic harvesting robots;
- Crop yield estimation.
Dr. Yunchan Tang
Prof. Dr. Chengliang Liu
Prof. Dr. Xiangjun Zou
Dr. Lufeng Luo
Guest Editors
Manuscript Submission Information
Manuscripts should be submitted online at www.mdpi.com by registering and logging in to this website. Once you are registered, click here to go to the submission form. Manuscripts can be submitted until the deadline. All submissions that pass pre-check are peer-reviewed. Accepted papers will be published continuously in the journal (as soon as accepted) and will be listed together on the special issue website. Research articles, review articles as well as short communications are invited. For planned papers, a title and short abstract (about 100 words) can be sent to the Editorial Office for announcement on this website.
Submitted manuscripts should not have been published previously, nor be under consideration for publication elsewhere (except conference proceedings papers). All manuscripts are thoroughly refereed through a single-blind peer-review process. A guide for authors and other relevant information for submission of manuscripts is available on the Instructions for Authors page. Applied Sciences is an international peer-reviewed open access semimonthly journal published by MDPI.
Please visit the Instructions for Authors page before submitting a manuscript. The Article Processing Charge (APC) for publication in this open access journal is 2400 CHF (Swiss Francs). Submitted papers should be well formatted and use good English. Authors may use MDPI's English editing service prior to publication or during author revisions.
Keywords
- robotics
- precision agriculture
- digital agriculture
- UAV
- remote sensing
Benefits of Publishing in a Special Issue
- Ease of navigation: Grouping papers by topic helps scholars navigate broad scope journals more efficiently.
- Greater discoverability: Special Issues support the reach and impact of scientific research. Articles in Special Issues are more discoverable and cited more frequently.
- Expansion of research network: Special Issues facilitate connections among authors, fostering scientific collaborations.
- External promotion: Articles in Special Issues are often promoted through the journal's social media, increasing their visibility.
- Reprint: MDPI Books provides the opportunity to republish successful Special Issues in book format, both online and in print.
Further information on MDPI's Special Issue policies can be found here.