Topic Menu
► Topic MenuTopic Editors
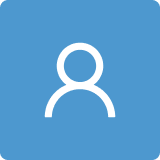
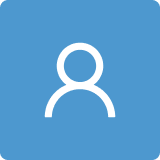
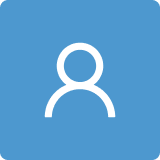
Short-Term Load Forecasting
Topic Information
Dear Colleagues,
It is well known that short-term load forecasting (STLF) plays a key role in the formulation of economic, reliable, and secure operating strategies for power systems (planning, scheduling, maintenance, and control processes, among others), and this topic has been an important issue for several decades. However, there is still much progress to be made in this field. The deployment of enabling technologies (e.g., smart meters and sub-metering) has made high granular data available for many customer segments and many tasks—for instance, it has made load forecasting tasks feasible at several demand aggregation levels. The first challenge in this area is the improvement of STLF models and their performance at new demand aggregation levels. Specifically, individual level demand forecasting, which is more challenging than aggregated demand, should be addressed in a comprehensive manner, helping customers in decision making. Moreover, the increasing inclusion of renewable energies (wind and solar power) in the power system, and the necessity of including more flexibility through demand response initiatives, have introduced greater uncertainties, creating new challenges for STLF in future power systems. Other relevant issues are net demand forecasting in “prosumers” (i.e., the integrated or disaggregated forecast of demand and renewable generation), and demand forecasting by end-uses in large or aggregated customers.
Many techniques have been proposed for STLF, including traditional statistical models (such as SARIMA, ARMAX, exponential smoothing, linear and non-linear models, etc.) and artificial intelligence techniques (such as fuzzy regression, artificial neural networks, support vector regression, tree-based regression, ensemble methods, stacked methods, etc.). In the case of individual loads, the techniques for peak detection and extreme values are of great importance. Furthermore, distribution planning needs, as well as grid modernization, have initiated the development of hierarchical load forecasting. Analogously, the need to face new uncertainty sources in the power system has given more importance to probabilistic load forecasting in recent years.
This Topic is concerned with both fundamental research on STLF methodologies and its practical application to power systems, aiming at exploring the challenges that will be faced by a more distributed power system in the future.
All submitted contributions must be based on the rigorous examination of the mentioned approaches and demonstrate a theoretically sound framework; submissions lacking such a scientific approach are discouraged. It is recommended that existing/presented approaches are validated using real practical applications.
Prof. Dr. Antonio Gabaldón
Prof. Dr. María Carmen Ruiz-Abellón
Prof. Dr. Luis Alfredo Fernández-Jiménez
Topic Editors
Keywords
- short-term load forecasting and distributed energy resources
- short-term load forecasting and demand aggregation levels
- statistical forecasting models (SARIMA, ARMAX, exponential smoothing, linear and non-linear regression, etc.)
- artificial neural networks (ANNs)
- fuzzy regression models
- tree-based regression methods
- stacked and ensemble methods
- evolutionary algorithms
- deep learning architectures
- support vector regression (SVR)
- robust load forecasting
- hierarchical and probabilistic forecasting
- hybrid and combined models
- renewable generation forecasting
- short-term net demand forecasting
- inference on extreme and rare events
- end-use demand forecasting
Participating Journals
Journal Name | Impact Factor | CiteScore | Launched Year | First Decision (median) | APC |
---|---|---|---|---|---|
![]()
Algorithms
|
1.8 | 4.1 | 2008 | 18.9 Days | CHF 1600 |
![]()
Applied Sciences
|
2.5 | 5.3 | 2011 | 18.4 Days | CHF 2400 |
![]()
Energies
|
3.0 | 6.2 | 2008 | 16.8 Days | CHF 2600 |
![]()
Forecasting
|
2.3 | 5.8 | 2019 | 18.5 Days | CHF 1800 |
![]()
Sustainability
|
3.3 | 6.8 | 2009 | 19.7 Days | CHF 2400 |
Preprints.org is a multidisciplinary platform offering a preprint service designed to facilitate the early sharing of your research. It supports and empowers your research journey from the very beginning.
MDPI Topics is collaborating with Preprints.org and has established a direct connection between MDPI journals and the platform. Authors are encouraged to take advantage of this opportunity by posting their preprints at Preprints.org prior to publication:
- Share your research immediately: disseminate your ideas prior to publication and establish priority for your work.
- Safeguard your intellectual contribution: Protect your ideas with a time-stamped preprint that serves as proof of your research timeline.
- Boost visibility and impact: Increase the reach and influence of your research by making it accessible to a global audience.
- Gain early feedback: Receive valuable input and insights from peers before submitting to a journal.
- Ensure broad indexing: Web of Science (Preprint Citation Index), Google Scholar, Crossref, SHARE, PrePubMed, Scilit and Europe PMC.