Topic Menu
► Topic MenuTopic Editors
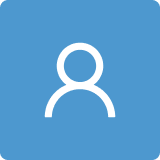
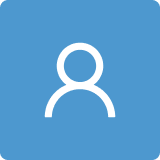
Computational Intelligence for Virus and Bacteria Detection in Multi Surface Environments
Topic Information
Dear Colleagues,
The year 2020 started with a global pandemic, with every nation having to manage the fallout of COVID-19’s spread. COVID-19, SARS, and several other viruses, fungi, and bacteria that are similarly dangerous for humans are present in our daily lives. We must work together to better understand and control them. Scientists are working in chemical laboratories to find a cure. Meanwhile, technologies to detect viruses, fungi, and bacteria on multi-surface environments are being developed. In certain conditions, the presence of biological threads, viruses, fungi, and bacteria can be recorded from metal, glass, paper, plastic, and several derivative materials and environments, whether porous or smooth, or soft or hard materials. Temperature and humidity are other factors with significant influence on the survival of viruses and bacteria.
During this pandemic, scientific methods that help detect viruses, fungi, and bacteria in image and data samples from multi surface environments are necessary. The aim of this Topic is to provide an open platform for scientists and professionals to present the latest achievements in detection methods and sensory apparatuses, by which analysis of information from images or other data samples can quickly and efficiently provide accurate detection to prevent the uncontrolled spread of biological threads, viruses, fungi, and bacteria that are dangerous both for humans and animals..
It is my pleasure to invite you to contribute your innovative research on computational intelligence to this Topic. This issue creates opportunities for dissemination of your research results and cooperation for further innovation.
Topics of interest:
- Bio-inspired methods, deep learning, convolutional neural networks, fuzzy systems, cognitive analysis, and hybrid architecture;
- Time series, gradient field methods, and surface reconstruction, as well as other mathematical models for intelligent feature detection, extraction, and recognition;
- Embedded intelligent computer vision algorithms for microscopic and infrared models;
- Human–nature technology and object activity recognition models;
- Hyper-parameter learning, transfer learning, automatic calibration, and hybrid and surrogate learning for computational intelligence in vision systems;
- Intelligent video and image acquisition techniques applied to medical, transportation, shopping, and delivery;
- Sensoric aparatures, devices, electronics, and various equipment to detect potential threads for humans and animals.
Prof. Dr. Marcin Woźniak
Prof. Dr. Muhammad Fazal Ijaz
Topic Editors
Keywords
- viruses
- fungi
- bacteria
- image samples
- data samples
- sensory apparatuses
- computational intelligence
- bio-inspired methods
- deep learning
- convolutional neural networks
Participating Journals
Journal Name | Impact Factor | CiteScore | Launched Year | First Decision (median) | APC |
---|---|---|---|---|---|
![]()
Sensors
|
3.4 | 7.3 | 2001 | 16.8 Days | CHF 2600 |
![]()
Viruses
|
3.8 | 7.3 | 2009 | 16.1 Days | CHF 2600 |
![]()
Diseases
|
2.9 | 0.8 | 2013 | 18.9 Days | CHF 1800 |
![]()
Algorithms
|
1.8 | 4.1 | 2008 | 15 Days | CHF 1600 |
![]()
Methods and Protocols
|
2.3 | 3.6 | 2018 | 24.9 Days | CHF 1800 |
![]()
Symmetry
|
2.2 | 5.4 | 2009 | 16.8 Days | CHF 2400 |
MDPI Topics is cooperating with Preprints.org and has built a direct connection between MDPI journals and Preprints.org. Authors are encouraged to enjoy the benefits by posting a preprint at Preprints.org prior to publication:
- Immediately share your ideas ahead of publication and establish your research priority;
- Protect your idea from being stolen with this time-stamped preprint article;
- Enhance the exposure and impact of your research;
- Receive feedback from your peers in advance;
- Have it indexed in Web of Science (Preprint Citation Index), Google Scholar, Crossref, SHARE, PrePubMed, Scilit and Europe PMC.