Topic Menu
► Topic MenuTopic Editors
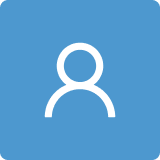
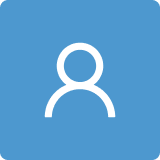
2. Biomedical Engineering Institute, Chiang Mai University, Chiang Mai 50200, Thailand
Artificial Intelligence in Healthcare - 2nd Volume
Topic Information
Dear Colleagues,
The complexity and rise of data in healthcare means that artificial intelligence (AI), databases, and related technologies will be increasingly applied in the field. Interdisciplinary approaches involving these data and their applications are a challenging new field in artificial intelligence (AI), databases, bio- and medical research, etc. playing a growing role in society today. As such, research in these fields represents a promising and an important technical field with the potential to improve our health and quality of life. Therefore, the goal of this Special Issue is to explore how emerging technology solutions and real-world applications in daily life, disease, cancer, healthcare, and hospitals can help us to lead heathy lives as well as improve wellbeing. This issue seeks to present not only solutions that combine state-of-the-art devices, computer software, and model-based approaches for exploiting the huge health and bio data, as well as the Internet of Things resources available (while ensuring that these systems are explainable to domain experts), but also new methods that more generally describe the successful application of emerging technologies and spectra, and science and engineering to issues such as disease, cancer, databases, sensor device and user interfaces, software design, and system implementation in healthcare, as well as the medical domain, biology, and wellbeing. The main goal is to cover the applications of artificial intelligence (AI) and related technologies and engineering issues addressing all facets of solutions in the real world in databases, disease, and human health technology from a wellbeing and healthy life perspective. This issue welcomes the submission of papers on technical, experimental, methodological, and data analytical approaches that develop and implement solutions focused on real-world problems and systems, as well as on general applications of AI and data mining. It also invites papers on emerging technology solutions and real-world applications related to life, disease, cancer, healthcare, and hospitals that can help humans to lead heathy lives. The topics of interest include but are not limited to the following:
- Data mining in healthcare;
- Machine and deep learning approaches for disease, and health data;
- Decision support systems for healthcare and wellbeing;
- Regression and forecasting for medical and/or biomedical signals;
- Healthcare and wellness information systems;
- Medical signal and image processing and techniques;
- Applications of AI techniques in healthcare and wellbeing systems;
- Intelligent computing and platforms in medicine and healthcare;
- Biomedical applications;
- Biomedical text mining;
- Deep learning and methods to explain disease prediction;
- Big data frameworks and architectures for applied medical and health data;
- Visualization and interactive interfaces related to healthcare systems;
- Recommending and decision-making models and systems based on AI and data mining technologies;
- Machine learning and deep learning applications for life, disease, cancer, healthcare, and hospitals;
- Querying and filtering on heterogeneous, multi-source streaming life, and health data;
- Internet of things and data management for human life;
- Data and applications for human life; data and applications for technology improvement;
- Emerging technologies and applications of data, database, big data, and data mining, AI, models.
Prof. Dr. Keun Ho Ryu
Prof. Dr. Nipon Theera-Umpon
Topic Editors
Keywords
- emerging and interdisciplinary technology
- artificial intelligence
- database and big data
- disease
- healthcare
- biomedicine/biomedical
- human life
Participating Journals
Journal Name | Impact Factor | CiteScore | Launched Year | First Decision (median) | APC |
---|---|---|---|---|---|
![]()
Applied Sciences
|
2.5 | 5.3 | 2011 | 18.4 Days | CHF 2400 |
![]()
Diagnostics
|
3.0 | 4.7 | 2011 | 20.3 Days | CHF 2600 |
![]()
Healthcare
|
2.4 | 3.5 | 2013 | 20.3 Days | CHF 2700 |
![]()
International Journal of Environmental Research and Public Health
|
- | 7.3 | 2004 | 25.8 Days | CHF 2500 |
![]()
Symmetry
|
2.2 | 5.4 | 2009 | 17.3 Days | CHF 2400 |
Preprints.org is a multidisciplinary platform offering a preprint service designed to facilitate the early sharing of your research. It supports and empowers your research journey from the very beginning.
MDPI Topics is collaborating with Preprints.org and has established a direct connection between MDPI journals and the platform. Authors are encouraged to take advantage of this opportunity by posting their preprints at Preprints.org prior to publication:
- Share your research immediately: disseminate your ideas prior to publication and establish priority for your work.
- Safeguard your intellectual contribution: Protect your ideas with a time-stamped preprint that serves as proof of your research timeline.
- Boost visibility and impact: Increase the reach and influence of your research by making it accessible to a global audience.
- Gain early feedback: Receive valuable input and insights from peers before submitting to a journal.
- Ensure broad indexing: Web of Science (Preprint Citation Index), Google Scholar, Crossref, SHARE, PrePubMed, Scilit and Europe PMC.