Energy Efficiency and Energy Consumption Modelling Using Artificial Neural Networks
A special issue of Energies (ISSN 1996-1073). This special issue belongs to the section "F5: Artificial Intelligence and Smart Energy".
Deadline for manuscript submissions: closed (20 October 2022) | Viewed by 19065
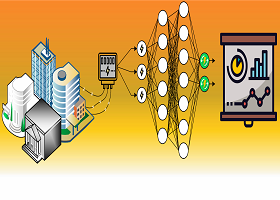
Special Issue Editors
Interests: machine learning; pattern recognition; computational intelligence; neural networks; deep learning; evolutionary algorithms; artificial intelligence; applied artificial intelligence; fuzzy logic; energy consumption modelling
Special Issues, Collections and Topics in MDPI journals
Interests: time series; data mining; artificial neural networks; energy efficiency; energy consumption modelling
Special Issues, Collections and Topics in MDPI journals
Special Issue Information
Dear Colleagues,
New technology and approaches are continuously and rapidly being introduced and implemented in today's energy systems. Machine Learning techniques play an increasingly important role in smart cities, ordinary buildings and renewable energy systems, amongst many others. As a consequence, assessing and modelling energy expenditure is a key task to improve energy efficiency in these systems. Recently, it has been noted that certain kinds of Machine Learning methods are growing in popularity when it comes to dealing with energy-related data for energy modelling and decision-making processes; these models are Artificial Neural Networks.
Traditionally, energy efficiency has mainly been handled using standard control methods in the energy industry. However, the application of intelligent techniques such as Artificial Neural Networks has led to new and sophisticated solutions for energy efficiency improvement. As a result, it is of paramount importance to develop and implement new intelligent techniques to address this problem.
This Special Issue aims to provide comprehensive coverage of energy efficiency and energy modelling using artificial neural networks. Therefore, we invite authors to contribute papers on innovative artificial intelligence applications for energy modelling, including reviews and case studies.
Prof. Dr. María del Carmen Pegalajar Jiménez
Dr. Luis G. Baca Ruiz
Guest Editors
Manuscript Submission Information
Manuscripts should be submitted online at www.mdpi.com by registering and logging in to this website. Once you are registered, click here to go to the submission form. Manuscripts can be submitted until the deadline. All submissions that pass pre-check are peer-reviewed. Accepted papers will be published continuously in the journal (as soon as accepted) and will be listed together on the special issue website. Research articles, review articles as well as short communications are invited. For planned papers, a title and short abstract (about 100 words) can be sent to the Editorial Office for announcement on this website.
Submitted manuscripts should not have been published previously, nor be under consideration for publication elsewhere (except conference proceedings papers). All manuscripts are thoroughly refereed through a single-blind peer-review process. A guide for authors and other relevant information for submission of manuscripts is available on the Instructions for Authors page. Energies is an international peer-reviewed open access semimonthly journal published by MDPI.
Please visit the Instructions for Authors page before submitting a manuscript. The Article Processing Charge (APC) for publication in this open access journal is 2600 CHF (Swiss Francs). Submitted papers should be well formatted and use good English. Authors may use MDPI's English editing service prior to publication or during author revisions.
Keywords
- Artificial Neural Networks
- Deep Learning
- Energy Consumption Modelling
- ANN model optimization applied to Energy Consumption
- Energy monitoring
- Energy modeling
- Machine Learning
- Energy optimization
- Energy systems
Benefits of Publishing in a Special Issue
- Ease of navigation: Grouping papers by topic helps scholars navigate broad scope journals more efficiently.
- Greater discoverability: Special Issues support the reach and impact of scientific research. Articles in Special Issues are more discoverable and cited more frequently.
- Expansion of research network: Special Issues facilitate connections among authors, fostering scientific collaborations.
- External promotion: Articles in Special Issues are often promoted through the journal's social media, increasing their visibility.
- Reprint: MDPI Books provides the opportunity to republish successful Special Issues in book format, both online and in print.
Further information on MDPI's Special Issue policies can be found here.