Emerging Applications of Deep Learning in Industry
A special issue of Electronics (ISSN 2079-9292). This special issue belongs to the section "Computer Science & Engineering".
Deadline for manuscript submissions: closed (31 January 2022) | Viewed by 12101
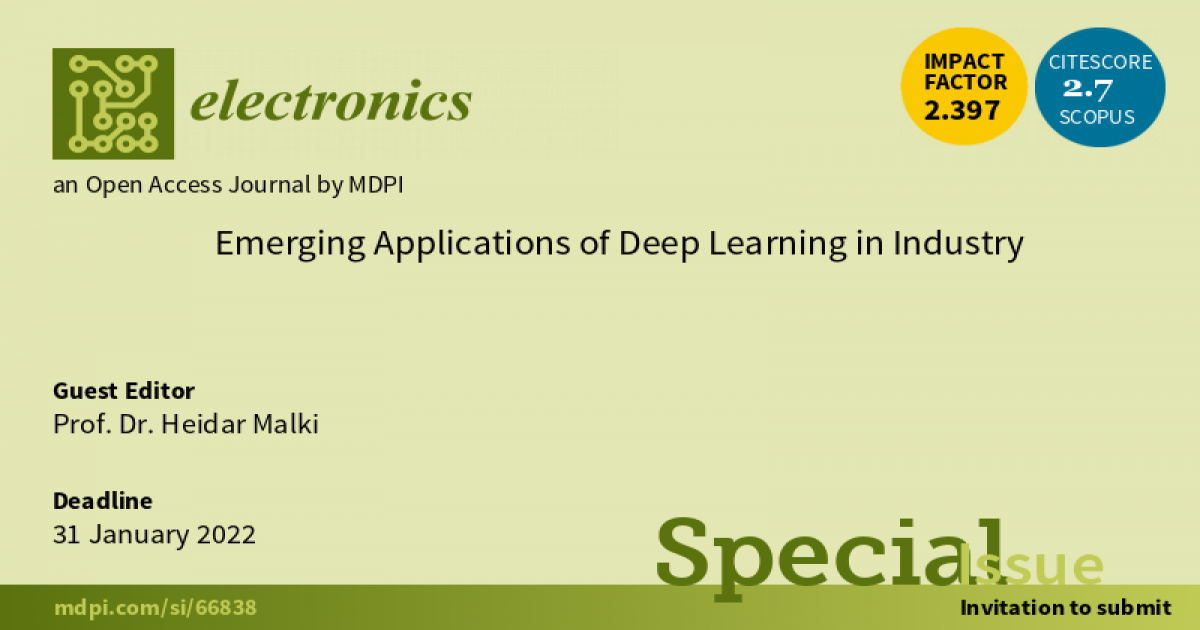
Special Issue Editor
Special Issue Information
Dear Colleagues,
The resurgence of multilayer neural network training algorithms in 1980s provided new tools to solve many complex and nonlinear systems. The resurgence of deep learning methods in recent years has allowed scientists to analyze and understand big data in all fields. Deep learning networks allows researchers and scientists to capture details and the complex behavior of physical systems. To this end, in this issue we invite scientists to submit their latest research on the applications of deep learning on all engineering fields including but not limited to image processing, recognition, classification, forecasting, and data analytics.
Prof. Dr. Heidar Malki
Guest Editor
Manuscript Submission Information
Manuscripts should be submitted online at www.mdpi.com by registering and logging in to this website. Once you are registered, click here to go to the submission form. Manuscripts can be submitted until the deadline. All submissions that pass pre-check are peer-reviewed. Accepted papers will be published continuously in the journal (as soon as accepted) and will be listed together on the special issue website. Research articles, review articles as well as short communications are invited. For planned papers, a title and short abstract (about 100 words) can be sent to the Editorial Office for announcement on this website.
Submitted manuscripts should not have been published previously, nor be under consideration for publication elsewhere (except conference proceedings papers). All manuscripts are thoroughly refereed through a single-blind peer-review process. A guide for authors and other relevant information for submission of manuscripts is available on the Instructions for Authors page. Electronics is an international peer-reviewed open access semimonthly journal published by MDPI.
Please visit the Instructions for Authors page before submitting a manuscript. The Article Processing Charge (APC) for publication in this open access journal is 2400 CHF (Swiss Francs). Submitted papers should be well formatted and use good English. Authors may use MDPI's English editing service prior to publication or during author revisions.
Keywords
- Neural networks
- deep learning
- classification
- recognitions
- data analytics
Benefits of Publishing in a Special Issue
- Ease of navigation: Grouping papers by topic helps scholars navigate broad scope journals more efficiently.
- Greater discoverability: Special Issues support the reach and impact of scientific research. Articles in Special Issues are more discoverable and cited more frequently.
- Expansion of research network: Special Issues facilitate connections among authors, fostering scientific collaborations.
- External promotion: Articles in Special Issues are often promoted through the journal's social media, increasing their visibility.
- Reprint: MDPI Books provides the opportunity to republish successful Special Issues in book format, both online and in print.
Further information on MDPI's Special Issue policies can be found here.