Recent Advances of Ultrasonic Testing in Materials
A special issue of Applied Sciences (ISSN 2076-3417). This special issue belongs to the section "Materials Science and Engineering".
Deadline for manuscript submissions: closed (31 January 2024) | Viewed by 7877
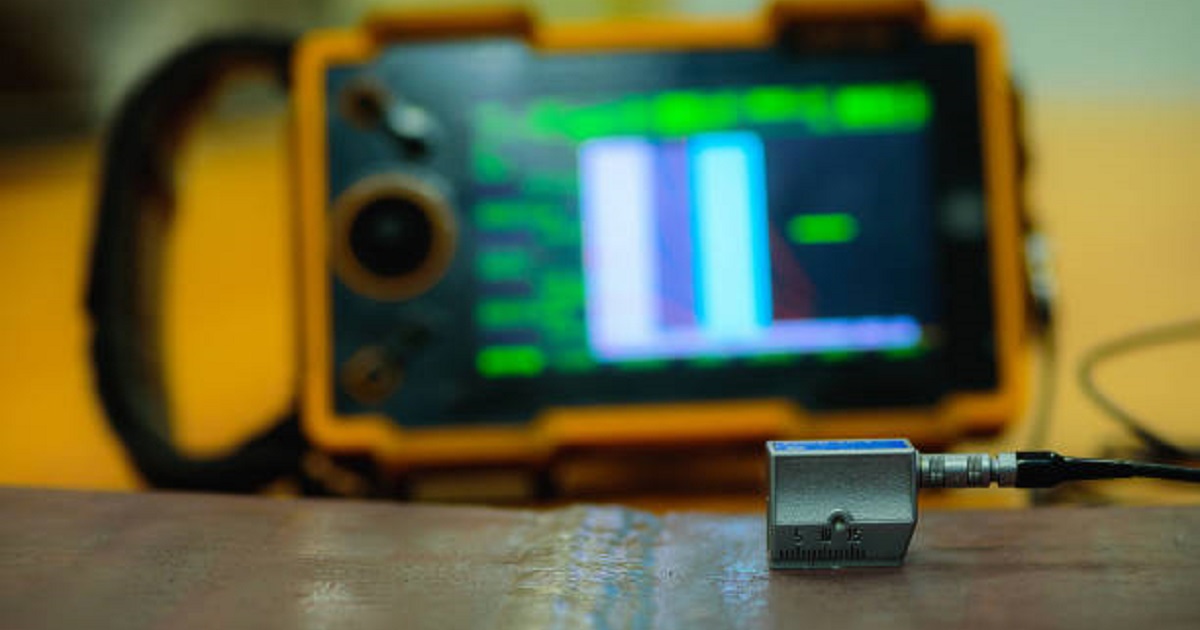
Special Issue Editors
Interests: modeling and simulating of nondestructive evaluation; automated NDE Systems; intelligent inversion of NDE signals; nondestructive materials characterization
Interests: modeling and mimulating of nondestructive evaluation; signal processing of NDE; machine learning of NDE; nuclear safety diagnosis;
Special Issue Information
Dear Colleagues,
We invite you to contribute to a Special Issue on the topic of recent advances of ultrasonic testing in materials. This Special Issue is about ultrasonic non-destructive testing and the evaluation applied in various research areas and practical fields. In particular, the Special Issue is to share the recent advances in finding defects in high-engineering application materials, such as titanium, Inconel, composite, dissimilar metal welds, TBC coating, etc. In addition, this Special Issue also intends to share the recent advances in ultrasonic nondestructive evaluation of the properies of those materials.
Especially, we encourage you to share your new approaches for inspecting defects that are difficult to detect using currently available techniques in the industrial fields. Those problematic defects would include surface micro cracks and IGSCC (intergranular stress corrosion cracking), etc.
We certainly welcome your new approaches using advanced sensors, such as IDT Sensor, EMAT, and air-cupplied transducer. We especially welcome your cutting-edge techniques using robust signal processing and interpretation tools, such as neural networks and export system.
We look forward to your participation and your expertise from academic and industrial fields.
Prof. Dr. Sung-Jin Song
Prof. Dr. Hak-Joon Kim
Guest Editors
Manuscript Submission Information
Manuscripts should be submitted online at www.mdpi.com by registering and logging in to this website. Once you are registered, click here to go to the submission form. Manuscripts can be submitted until the deadline. All submissions that pass pre-check are peer-reviewed. Accepted papers will be published continuously in the journal (as soon as accepted) and will be listed together on the special issue website. Research articles, review articles as well as short communications are invited. For planned papers, a title and short abstract (about 100 words) can be sent to the Editorial Office for announcement on this website.
Submitted manuscripts should not have been published previously, nor be under consideration for publication elsewhere (except conference proceedings papers). All manuscripts are thoroughly refereed through a single-blind peer-review process. A guide for authors and other relevant information for submission of manuscripts is available on the Instructions for Authors page. Applied Sciences is an international peer-reviewed open access semimonthly journal published by MDPI.
Please visit the Instructions for Authors page before submitting a manuscript. The Article Processing Charge (APC) for publication in this open access journal is 2400 CHF (Swiss Francs). Submitted papers should be well formatted and use good English. Authors may use MDPI's English editing service prior to publication or during author revisions.
Keywords
- ultrasonic non-destructive testing
- ultrasonic non-destructive evaluation
- defect characterization in materials
- material property characterization
- advanced ultrasonic sensors
- artificial intelligence
- advanced signal processing and interpretation