Hardware-Aware Deep Learning
A special issue of Applied Sciences (ISSN 2076-3417). This special issue belongs to the section "Computing and Artificial Intelligence".
Deadline for manuscript submissions: closed (20 May 2023) | Viewed by 41494
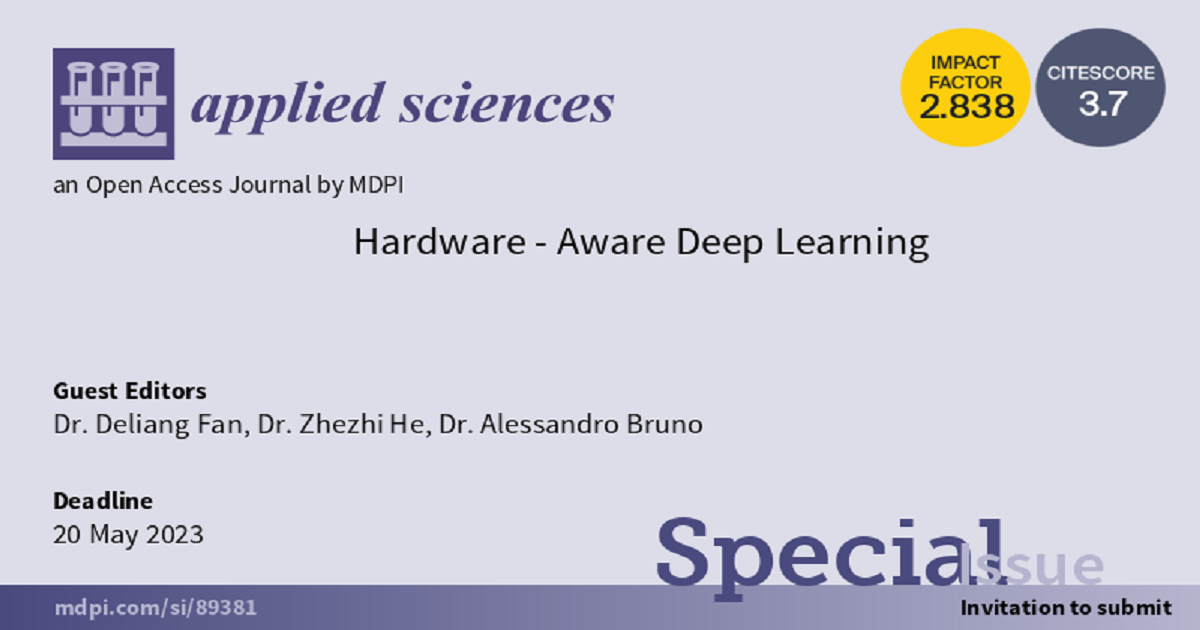
Special Issue Editors
Interests: hardware-aware deep learning; in-memory computing; emerging post-CMOS non-volatile memory; trustworthy AI
Interests: neuromorphic computing; secure and efficient deep learning; electronic design automation
Interests: computer vision; artificial intelligence; deep learning; image analysis and processing; visual saliency; biomedical engineering
Special Issues, Collections and Topics in MDPI journals
Special Issue Information
Dear Colleagues,
One of the main factors that contributes to the success of deep learning (DL) is the mighty computing power provided by modern hardware, spanning from high-performance server systems to resource-limited edge devices. The edge side (e.g., embedded systems, IoT) demands not only extreme energy-efficiency but also real-time inference capability, which requires cross-stack techniques, including model compression, compilation, architecture and circuit design of AI chips, emerging devices, etc. Beyond that, recent investigations, such federated learning, also bring model training to the edge side, with the data-security and computing limitations of mobile devices taken into consideration. On the cloud side, as the DL model size grows exponentially in the last two years (e.g., OpenAI GPT3, Google switch-transformer, etc.), how to efficiently support the training and inference of those immerse models is also an emerging research direction. Without lowering their hardware cost, however, incorporating them into the paradigm of machine learning as a service (MLaaS) will be infeasible. Moreover, the security and fault tolerant capability of DL also leads to a coherent of research, such as DL against error and non-ideal effects of the target hardware (e.g., bit-error of memory system). Therefore, the aforementioned concerns motivate the research of hardware-aware deep learning, for optimized energy, latency, and even security.
Dr. Deliang Fan
Dr. Zhezhi He
Dr. Alessandro Bruno
Guest Editors
Manuscript Submission Information
Manuscripts should be submitted online at www.mdpi.com by registering and logging in to this website. Once you are registered, click here to go to the submission form. Manuscripts can be submitted until the deadline. All submissions that pass pre-check are peer-reviewed. Accepted papers will be published continuously in the journal (as soon as accepted) and will be listed together on the special issue website. Research articles, review articles as well as short communications are invited. For planned papers, a title and short abstract (about 100 words) can be sent to the Editorial Office for announcement on this website.
Submitted manuscripts should not have been published previously, nor be under consideration for publication elsewhere (except conference proceedings papers). All manuscripts are thoroughly refereed through a single-blind peer-review process. A guide for authors and other relevant information for submission of manuscripts is available on the Instructions for Authors page. Applied Sciences is an international peer-reviewed open access semimonthly journal published by MDPI.
Please visit the Instructions for Authors page before submitting a manuscript. The Article Processing Charge (APC) for publication in this open access journal is 2400 CHF (Swiss Francs). Submitted papers should be well formatted and use good English. Authors may use MDPI's English editing service prior to publication or during author revisions.
Keywords
- acceleration of deep learning
- artificial intelligence of things (AIoT)
- model compression
- algorithm and hardware co-design for deep learning
- neural architecture search
- security issues associated with deep learning on hardware
- near-sensor intelligence
- hardware-aware compilation techniques of deep learning
- federated learning and split learning
Benefits of Publishing in a Special Issue
- Ease of navigation: Grouping papers by topic helps scholars navigate broad scope journals more efficiently.
- Greater discoverability: Special Issues support the reach and impact of scientific research. Articles in Special Issues are more discoverable and cited more frequently.
- Expansion of research network: Special Issues facilitate connections among authors, fostering scientific collaborations.
- External promotion: Articles in Special Issues are often promoted through the journal's social media, increasing their visibility.
- Reprint: MDPI Books provides the opportunity to republish successful Special Issues in book format, both online and in print.
Further information on MDPI's Special Issue policies can be found here.