Edge and Cloud Computing Systems and Applications
A special issue of Applied Sciences (ISSN 2076-3417). This special issue belongs to the section "Computing and Artificial Intelligence".
Deadline for manuscript submissions: closed (20 November 2023) | Viewed by 24631
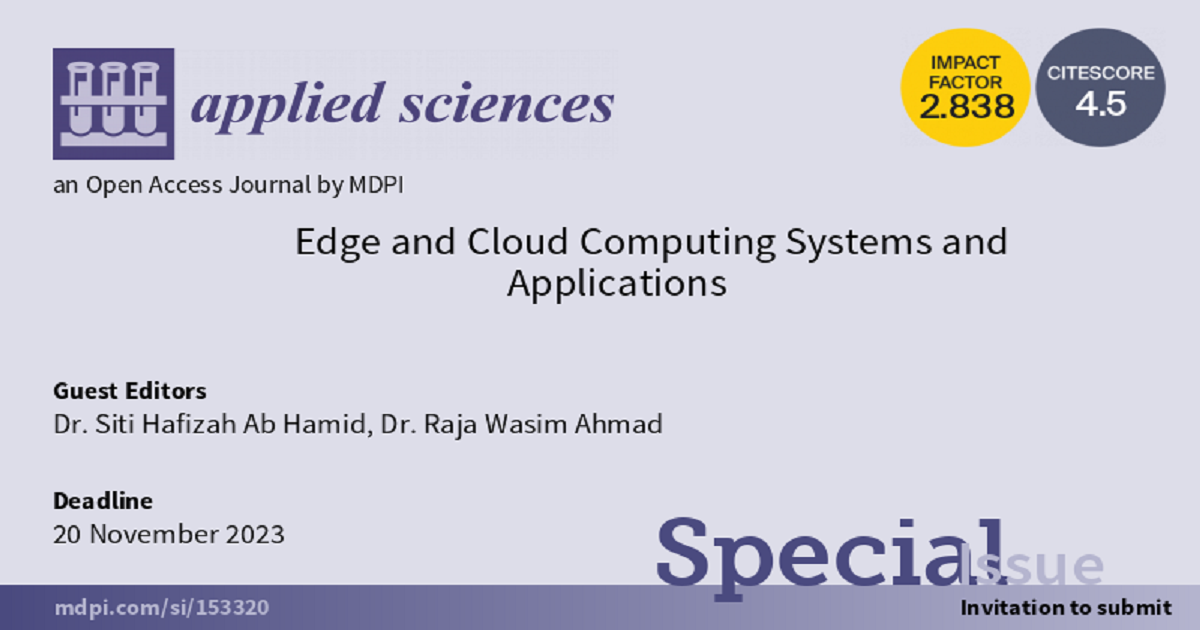
Special Issue Editors
Interests: software application reliability; software efficiency; machine and deep learning; reinforcement learning; multithreading communication.
Special Issue Information
Dear Colleagues,
With the recent need for digital integration across sectors and industries, from automobile to healthcare, banking, finance, retail, agriculture and manufacturing industries, edge and cloud computing ecosystems must be reliable and effective. Edge and cloud computing converge computation, communications, and storage services through systems and applications in a distributed manner. Hence, they open nearly unlimited research and application opportunities and raise unforeseen technological, theoretical, and societal issues and challenges that are not yet properly specified.
We are pleased to invite you to contribute your valuable work of experimental and application-oriented results to this Special Issue, which aims to disseminate recent developments in edge and cloud reliability, edge and cloud management, edge and cloud resources, edge and cloud services, as well as efficiency and security in edge and cloud systems. This Special Issue will highlight case studies and industrial applications in edge/cloud storage, edge/cloud connections, edge/cloud analytics, edge/cloud artificial intelligence, and edge/cloud processing engines.
This Special Issue aims to welcome both original research articles and reviews. Research areas may include (but are not limited to) the following:
- Edge and cloud technologies in software systems;
- Edge and cloud technologies for the Metaverse;
- Edge and cloud application innovations;
- Edge and cloud in blockchain;
- Industry-specific edges;
- Risk analysis of edge and cloud applications;
- Trust computing in edge and cloud applications
- Quality of Service in edge and cloud applications;
- Artificial intelligence for edge and cloud applications;
- Requirements and use cases for edge and cloud applications;
- Fault tolerance and recovery in edge and cloud technologies;
- Application regulations and standards with edge and cloud technologies.
Dr. Siti Hafizah Ab Hamid
Dr. Raja Wasim Ahmad
Guest Editors
Manuscript Submission Information
Manuscripts should be submitted online at www.mdpi.com by registering and logging in to this website. Once you are registered, click here to go to the submission form. Manuscripts can be submitted until the deadline. All submissions that pass pre-check are peer-reviewed. Accepted papers will be published continuously in the journal (as soon as accepted) and will be listed together on the special issue website. Research articles, review articles as well as short communications are invited. For planned papers, a title and short abstract (about 100 words) can be sent to the Editorial Office for announcement on this website.
Submitted manuscripts should not have been published previously, nor be under consideration for publication elsewhere (except conference proceedings papers). All manuscripts are thoroughly refereed through a single-blind peer-review process. A guide for authors and other relevant information for submission of manuscripts is available on the Instructions for Authors page. Applied Sciences is an international peer-reviewed open access semimonthly journal published by MDPI.
Please visit the Instructions for Authors page before submitting a manuscript. The Article Processing Charge (APC) for publication in this open access journal is 2400 CHF (Swiss Francs). Submitted papers should be well formatted and use good English. Authors may use MDPI's English editing service prior to publication or during author revisions.
Keywords
- edge computing
- cloud computing
- machine learning
- deep learning
- reinforcement learning
- collaborative computing
- performance evaluation
- computation analytics
- edge-based application
- cloud-based application
Benefits of Publishing in a Special Issue
- Ease of navigation: Grouping papers by topic helps scholars navigate broad scope journals more efficiently.
- Greater discoverability: Special Issues support the reach and impact of scientific research. Articles in Special Issues are more discoverable and cited more frequently.
- Expansion of research network: Special Issues facilitate connections among authors, fostering scientific collaborations.
- External promotion: Articles in Special Issues are often promoted through the journal's social media, increasing their visibility.
- Reprint: MDPI Books provides the opportunity to republish successful Special Issues in book format, both online and in print.
Further information on MDPI's Special Issue policies can be found here.