Topic Menu
► Topic MenuTopic Editors
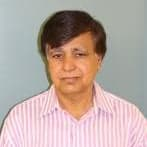
Advances in Data Analytics with Applications to Health Care
Topic Information
Dear Colleagues,
As we embark on the second quarter of the 21st century, the landscape of health care data as well as relevant analytics tools are rapidly changing. In the early years of statistical learning methodologies, data sets were limited to relatively small samples, and randomized clinical trials were the gold standard in knowledge extraction. Administrative data sets were often dismissed by statisticians as observational data with a plenty of biases. With the availability of large administrative data sets (streamed from all sorts of channels such as Electronic Medical Records (EMR), wearable sensors, disease control and public health organizations, etc.), the data analytics communities (statisticians, computer scientists, epidemiologists, etc.) were forced to develop new tools for the analysis of such data sets and meaningful information to the public and to the concerned decision makers. These advances have touched all areas of the health care data analytics, from mimicking clinical trials via causal inferences to modifying random forests and neural networks to accommodate data with special features such as censored event history data and going through models that describe an entire distribution such as quantile regressions. Applications of these new advanced methodologies have benefited a variety of health care domains leading to, for instance, better personalized health care (e.g., personalized medicine), better telemedicine and automated remote diagnoses, better predictions of supply chain and product demand in health care (specially during pandemics) and many more.
This Topic is an attempt to provide a glimpse of these advanced tools, especially during the past two years, in which a global pandemic presented an opportunity to learn more about the deficiencies of the existing data analytics tools. The topics to be covered include but are not limited to the following:
- New and advanced statistical learning (SL) tools for the analysis of health care data;
- New and advanced machine learning (ML) tools for the analysis of health care data;
- Novel ways of applying existing SL and/or ML tools to health care data.
Prof. Dr. S. Ejaz Ahmed
Prof. Dr. Abdulkadir Hussein
Dr. Abbas Khalili
Topic Editors
Participating Journals
Journal Name | Impact Factor | CiteScore | Launched Year | First Decision (median) | APC |
---|---|---|---|---|---|
![]()
Entropy
|
2.0 | 5.2 | 1999 | 21.8 Days | CHF 2600 |
![]()
Information
|
2.9 | 6.5 | 2010 | 18.6 Days | CHF 1800 |
![]()
Data
|
2.0 | 5.0 | 2016 | 25.2 Days | CHF 1600 |
![]()
International Journal of Environmental Research and Public Health
|
- | 8.5 | 2004 | 27.8 Days | CHF 2500 |
![]()
Machine Learning and Knowledge Extraction
|
6.0 | 9.9 | 2019 | 25.5 Days | CHF 1800 |
Preprints.org is a multidisciplinary platform offering a preprint service designed to facilitate the early sharing of your research. It supports and empowers your research journey from the very beginning.
MDPI Topics is collaborating with Preprints.org and has established a direct connection between MDPI journals and the platform. Authors are encouraged to take advantage of this opportunity by posting their preprints at Preprints.org prior to publication:
- Share your research immediately: disseminate your ideas prior to publication and establish priority for your work.
- Safeguard your intellectual contribution: Protect your ideas with a time-stamped preprint that serves as proof of your research timeline.
- Boost visibility and impact: Increase the reach and influence of your research by making it accessible to a global audience.
- Gain early feedback: Receive valuable input and insights from peers before submitting to a journal.
- Ensure broad indexing: Web of Science (Preprint Citation Index), Google Scholar, Crossref, SHARE, PrePubMed, Scilit and Europe PMC.