Topic Menu
► Topic MenuTopic Editors

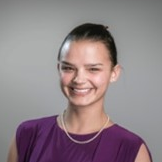
Machine Learning for Pharmacogenomics and Precision Medicine
Topic Information
Dear Colleagues,
Delivering the correct drug to the correct patient at the right time while avoiding toxic side effects remains a major limitation of modern medicine across disease modalities. The corpus of available disease-relevant omics data is ever-expanding alongside the increasing panoply of imaging and other relevant data types. Concurrent with this expansion, various machine learning algorithms are becoming increasingly suitable for application using these diverse data types and are even able to integrate multiple types. Together, these advances present the opportunity to ask and answer important questions around pharmacogenomics and precision medicine.
This Topic serves as a compendium for global leaders to present their most recent findings to educate and enhance the work of the broader community. In order to be relevant to experts and newcomers alike, both review and original research articles are invited to this Topic.
Prof. Dr. Weida Tong
Dr. Rebecca Kusko
Topic Editors
Keywords
- machine learning
- artificial intelligence
- genomics and genetics
- pharmacogenomics
- drug response
- diagnosis and prognosis
- precision and personalized medicine
- biomarker development
- translational informatics
- image analysis
Participating Journals
Journal Name | Impact Factor | CiteScore | Launched Year | First Decision (median) | APC |
---|---|---|---|---|---|
![]()
Biomedicines
|
3.9 | 5.2 | 2013 | 14.6 Days | CHF 2600 |
![]()
International Journal of Molecular Sciences
|
4.9 | 8.1 | 2000 | 16.8 Days | CHF 2900 |
![]()
Journal of Clinical Medicine
|
3.0 | 5.7 | 2012 | 16 Days | CHF 2600 |
![]()
Journal of Personalized Medicine
|
- | 4.1 | 2011 | 17.4 Days | CHF 2600 |
![]()
Pharmaceuticals
|
4.3 | 6.1 | 2004 | 13.9 Days | CHF 2900 |
![]()
Pharmacy
|
2.0 | - | 2013 | 24.6 Days | CHF 1800 |
![]()
Pharmaceutics
|
4.9 | 7.9 | 2009 | 15.5 Days | CHF 2900 |
Preprints.org is a multidisciplinary platform offering a preprint service designed to facilitate the early sharing of your research. It supports and empowers your research journey from the very beginning.
MDPI Topics is collaborating with Preprints.org and has established a direct connection between MDPI journals and the platform. Authors are encouraged to take advantage of this opportunity by posting their preprints at Preprints.org prior to publication:
- Share your research immediately: disseminate your ideas prior to publication and establish priority for your work.
- Safeguard your intellectual contribution: Protect your ideas with a time-stamped preprint that serves as proof of your research timeline.
- Boost visibility and impact: Increase the reach and influence of your research by making it accessible to a global audience.
- Gain early feedback: Receive valuable input and insights from peers before submitting to a journal.
- Ensure broad indexing: Web of Science (Preprint Citation Index), Google Scholar, Crossref, SHARE, PrePubMed, Scilit and Europe PMC.