The Future of Cyber Security in Smart Grids
A special issue of Energies (ISSN 1996-1073). This special issue belongs to the section "A1: Smart Grids and Microgrids".
Deadline for manuscript submissions: closed (15 September 2023) | Viewed by 3192
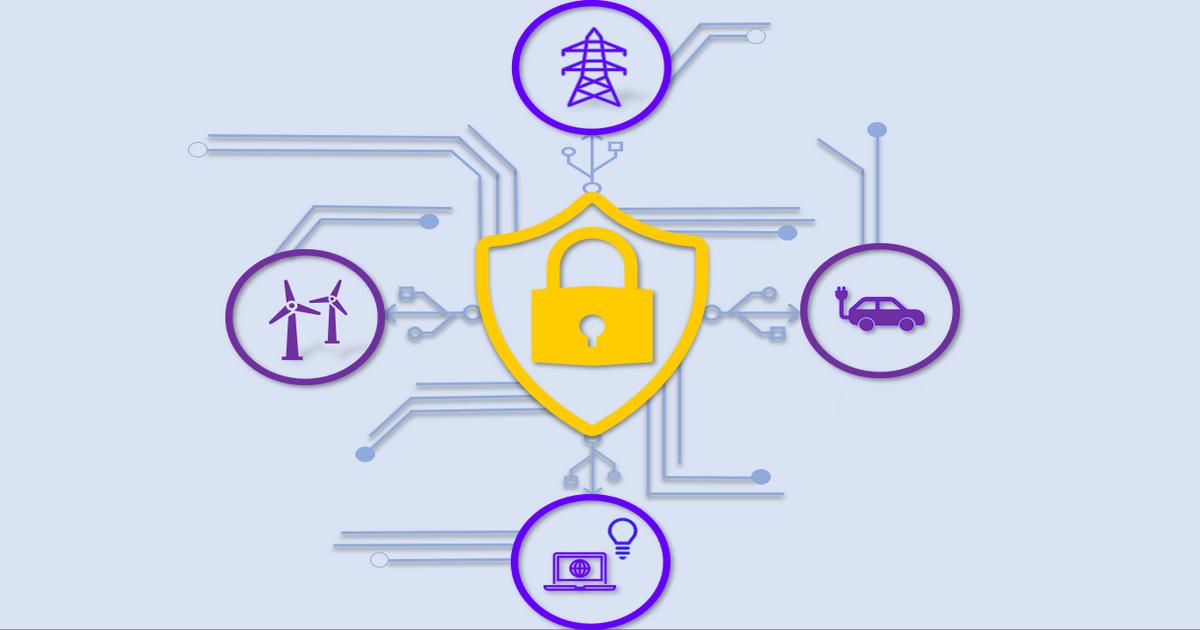
Special Issue Editors
Interests: cyber security; smart grids; energy system digitalisation; communication networks
Interests: applied cryptography; information security; data privacy; smart grid; P2P energy trading
Special Issue Information
Dear Colleagues,
Smart Grids are smart electrical networks that use digital technologies to service the power grid by enabling fine-grain data collection, management, and analytics. Digital technologies can support the decarbonization and decentralization of Smart Grids, and provide communication, interconnection, and interdependency between various critical infrastructures, increase grid visibility, enable proactive maintenance, and manage demand and supply. However, these digital technologies can introduce complex cybersecurity challenges in Smart Grids. For example, home appliances and products that were traditionally offline, are becoming online, so we need to protect these devices, applications, and data against cyber-attack and data privacy threats. These cyber-attacks have negative impacts of varying severity on Smart Grids, such as causing cascading failure, affecting the capability of the energy system to deliver a reliable service, manipulating the market, and misguiding the billing systems.
This Special Issue aims to present and disseminate the latest understanding and development on cyber security challenges in future smart grids and to demonstrate solutions to mitigate the potential cyber threats and data privacy risks that smart technologies can introduce. We strongly encourage interdisciplinary work to be submitted.
Topics of particular interest include but are not limited to cyber security in smart grids, data privacy in smart grids, cyber-physical systems, cyber mitigation techniques for energy systems, EV charging stations, smart metering, communication protocols, communication networks, peer-to-peer energy trading, transactive energy and community/collective self-consumption.
Dr. Zoya Pourmirza
Dr. Mustafa A. Mustafa
Dr. Roberto Metere
Guest Editors
Manuscript Submission Information
Manuscripts should be submitted online at www.mdpi.com by registering and logging in to this website. Once you are registered, click here to go to the submission form. Manuscripts can be submitted until the deadline. All submissions that pass pre-check are peer-reviewed. Accepted papers will be published continuously in the journal (as soon as accepted) and will be listed together on the special issue website. Research articles, review articles as well as short communications are invited. For planned papers, a title and short abstract (about 100 words) can be sent to the Editorial Office for announcement on this website.
Submitted manuscripts should not have been published previously, nor be under consideration for publication elsewhere (except conference proceedings papers). All manuscripts are thoroughly refereed through a single-blind peer-review process. A guide for authors and other relevant information for submission of manuscripts is available on the Instructions for Authors page. Energies is an international peer-reviewed open access semimonthly journal published by MDPI.
Please visit the Instructions for Authors page before submitting a manuscript. The Article Processing Charge (APC) for publication in this open access journal is 2600 CHF (Swiss Francs). Submitted papers should be well formatted and use good English. Authors may use MDPI's English editing service prior to publication or during author revisions.
Keywords
- cyber security in smart grids
- data privacy in smart grids
- cyber physical systems
- EV charging stations
- smart metering
- communication protocols
- communication networks
- peer-to-peer energy trading
- transactive energy
- community/collective self-consumption
Benefits of Publishing in a Special Issue
- Ease of navigation: Grouping papers by topic helps scholars navigate broad scope journals more efficiently.
- Greater discoverability: Special Issues support the reach and impact of scientific research. Articles in Special Issues are more discoverable and cited more frequently.
- Expansion of research network: Special Issues facilitate connections among authors, fostering scientific collaborations.
- External promotion: Articles in Special Issues are often promoted through the journal's social media, increasing their visibility.
- Reprint: MDPI Books provides the opportunity to republish successful Special Issues in book format, both online and in print.
Further information on MDPI's Special Issue policies can be found here.